Essays On Robust Model Selection And Model Averaging For
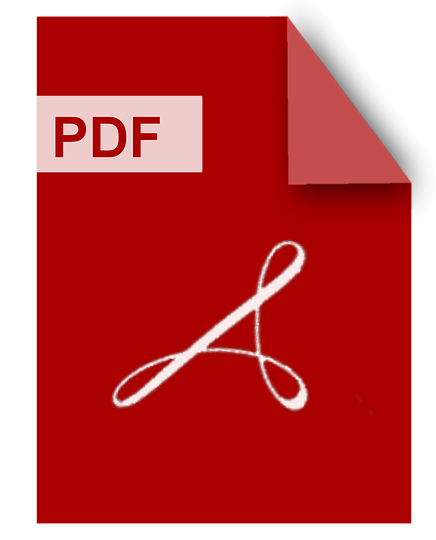
DOWNLOAD
Download Essays On Robust Model Selection And Model Averaging For PDF/ePub or read online books in Mobi eBooks. Click Download or Read Online button to get Essays On Robust Model Selection And Model Averaging For book now. This website allows unlimited access to, at the time of writing, more than 1.5 million titles, including hundreds of thousands of titles in various foreign languages. If the content not found or just blank you must refresh this page
Essays On Robust Model Selection And Model Averaging For Linear Models
DOWNLOAD
Author : Le Chang
language : en
Publisher:
Release Date : 2017
Essays On Robust Model Selection And Model Averaging For Linear Models written by Le Chang and has been published by this book supported file pdf, txt, epub, kindle and other format this book has been release on 2017 with categories.
Model selection is central to all applied statistical work. Selecting the variables for use in a regression model is one important example of model selection. This thesis is a collection of essays on robust model selection procedures and model averaging for linear regression models. In the first essay, we propose robust Akaike information criteria (AIC) for MM-estimation and an adjusted robust scale based AIC for M and MM-estimation. Our proposed model selection criteria can maintain their robust properties in the presence of a high proportion of outliers and the outliers in the covariates. We compare our proposed criteria with other robust model selection criteria discussed in previous literature. Our simulation studies demonstrate a significant outperformance of robust AIC based on MM-estimation in the presence of outliers in the covariates. The real data example also shows a better performance of robust AIC based on MM-estimation. The second essay focuses on robust versions of the "Least Absolute Shrinkage and Selection Operator" (lasso). The adaptive lasso is a method for performing simultaneous parameter estimation and variable selection. The adaptive weights used in its penalty term mean that the adaptive lasso achieves the oracle property. In this essay, we propose an extension of the adaptive lasso named the Tukey-lasso. By using Tukey's biweight criterion, instead of squared loss, the Tukey-lasso is resistant to outliers in both the response and covariates. Importantly, we demonstrate that the Tukey-lasso also enjoys the oracle property. A fast accelerated proximal gradient (APG) algorithm is proposed and implemented for computing the Tukey-lasso. Our extensive simulations show that the Tukey-lasso, implemented with the APG algorithm, achieves very reliable results, including for high-dimensional data where p>n. In the presence of outliers, the Tukey-lasso is shown to offer substantial improvements in performance compared to the adaptive lasso and other robust implementations of the lasso. Real data examples further demonstrate the utility of the Tukey-lasso. In many statistical analyses, a single model is used for statistical inference, ignoring the process that leads to the model being selected. To account for this model uncertainty, many model averaging procedures have been proposed. In the last essay, we propose an extension of a bootstrap model averaging approach, called bootstrap lasso averaging (BLA). BLA utilizes the lasso for model selection. This is in contrast to other forms of bootstrap model averaging that use AIC or Bayesian information criteria (BIC). The use of the lasso improves the computation speed and allows BLA to be applied even when the number of variables p is larger than the sample size n. Extensive simulations confirm that BLA has outstanding finite sample performance, in terms of both variable and prediction accuracies, compared with traditional model selection and model averaging methods. Several real data examples further demonstrate an improved out-of-sample predictive performance of BLA.
Essays On Model Averaging
DOWNLOAD
Author :
language : en
Publisher:
Release Date : 2012
Essays On Model Averaging written by and has been published by this book supported file pdf, txt, epub, kindle and other format this book has been release on 2012 with categories.
This dissertation is a collection of three essays on model averaging, organized in the form of three chapters. The first chapter proposes a new model averaging estimator for the linear regression model with heteroskedastic errors. We address the issues of how to assign the weights for candidate models optimally and how to make inference based on the averaging estimator. We first derive the asymptotic distribution of the averaging estimator with fixed weights in a local asymptotic framework, which allows us to characterize the optimal weights. The optimal weights are obtained by minimizing the asymptotic mean squared error. Second, we propose a plug-in estimator of the optimal weights and use these estimated weights to construct a plug-in averaging estimator of the parameter of interest. We derive the asymptotic distribution of the proposed estimator. Third, we show that confidence intervals based on normal approximations lead to distorted inference in this context. We suggest a plug-in method to construct confidence intervals, which have good finite-sample coverage probabilities. The second chapter investigates model combination in a predictive regression. We derive the mean squared forecast error (MSFE) of the model averaging estimator in a local asymptotic framework. We show that the optimal model weights which minimize the MSFE depend on the local parameters and the covariance matrix of the predictive regression. We propose a plug-in estimator of the optimal weights and use these estimated weights to construct the forecast combination. The third chapter proposes a model averaging approach to reduce the mean squared error (MSE) and weighted integrated mean squared error (WIMSE) of kernel estimators of regression functions. At each point of estimation, we construct a weighted average of the local constant and local linear estimators. The optimal local and global weights for averaging are chosen to minimize the MSE and WIMSE of the averaging estimator, respectively. We propose two data-driven approaches for bandwidth and weight selection and derive the rate of convergence of the cross-validated weights to their optimal benchmark values.
Essays In Honor Of Subal Kumbhakar
DOWNLOAD
Author : Christopher F. Parmeter
language : en
Publisher: Emerald Group Publishing
Release Date : 2024-04-05
Essays In Honor Of Subal Kumbhakar written by Christopher F. Parmeter and has been published by Emerald Group Publishing this book supported file pdf, txt, epub, kindle and other format this book has been release on 2024-04-05 with Business & Economics categories.
It is the editor’s distinct privilege to gather this collection of papers that honors Subhal Kumbhakar’s many accomplishments, drawing further attention to the various areas of scholarship that he has touched.
Model Selection And Model Averaging
DOWNLOAD
Author : Gerda Claeskens
language : en
Publisher:
Release Date : 2008-07-28
Model Selection And Model Averaging written by Gerda Claeskens and has been published by this book supported file pdf, txt, epub, kindle and other format this book has been release on 2008-07-28 with Mathematics categories.
First book to synthesize the research and practice from the active field of model selection.
The Robustness Of Model Selection Rules
DOWNLOAD
Author : Jochen A. Jungeilges
language : en
Publisher: LIT Verlag Münster
Release Date : 1992
The Robustness Of Model Selection Rules written by Jochen A. Jungeilges and has been published by LIT Verlag Münster this book supported file pdf, txt, epub, kindle and other format this book has been release on 1992 with Business & Economics categories.
Essays In Honor Of M Hashem Pesaran
DOWNLOAD
Author : Alexander Chudik
language : en
Publisher: Emerald Group Publishing
Release Date : 2022-01-18
Essays In Honor Of M Hashem Pesaran written by Alexander Chudik and has been published by Emerald Group Publishing this book supported file pdf, txt, epub, kindle and other format this book has been release on 2022-01-18 with Business & Economics categories.
The collection of chapters in Volume 43 Part A of Advances in Econometrics serves as a tribute to one of the most innovative, influential, and productive econometricians of his generation, Professor M. Hashem Pesaran.
Essays In Honor Of Aman Ullah
DOWNLOAD
Author : R. Carter Hill
language : en
Publisher: Emerald Group Publishing
Release Date : 2016-06-29
Essays In Honor Of Aman Ullah written by R. Carter Hill and has been published by Emerald Group Publishing this book supported file pdf, txt, epub, kindle and other format this book has been release on 2016-06-29 with Business & Economics categories.
Volume 36 of Advances in Econometrics recognizes Aman Ullah's significant contributions in many areas of econometrics and celebrates his long productive career.
Model Selection And Model Averaging For Neural Networks
DOWNLOAD
Author : Herbert K. H. Lee
language : en
Publisher:
Release Date : 1998
Model Selection And Model Averaging For Neural Networks written by Herbert K. H. Lee and has been published by this book supported file pdf, txt, epub, kindle and other format this book has been release on 1998 with Bayesian statistical decision theory categories.
Improving Robust Model Selection Tests For Dynamic Models
DOWNLOAD
Author : Hwan-sik Choi
language : en
Publisher:
Release Date : 2010
Improving Robust Model Selection Tests For Dynamic Models written by Hwan-sik Choi and has been published by this book supported file pdf, txt, epub, kindle and other format this book has been release on 2010 with categories.
We propose an improved model selection test for dynamic models based on the method of Rivers and Vuong (2002) using a new asymptotic approximation to the sampling distribution of a new test statistic. The model selection test is applicable to dynamic models with very general selection criteria and estimation methods. Since our test statistic does not assume the exact form of a true model, the test is essentially nonparametric once competing models are estimated. For the unkown serial correlation in data, we use a Heteroskedasticity/Autocorrelation Consistent (HAC) variance estimator, and the sampling distribution of the test statistic is approximated by the fixed-b asymptotic approximation (Kiefer and Vogelsang (2005)). The asymptotic approximation depends on kernel functions and bandwidth parameters used in HAC estimators. We compare the finite sample performance of the new test with the bootstrap methods as well as with the standard normal approximations, and show that the fixed-b asymptotics and the bootstrap methods are markedly superior to the standard normal approximation for a moderate sample size for time series data. An empirical application for foreign exchange rate forecasting models is presented, and the result shows the normal approximation to the distribution of the test statistic considered appears to overstate the data's ability to distinguish between two competing models.
Algorithms For Statistical Model Selection And Robust Estimation
DOWNLOAD
Author : Marc Hofmann
language : en
Publisher:
Release Date : 2009
Algorithms For Statistical Model Selection And Robust Estimation written by Marc Hofmann and has been published by this book supported file pdf, txt, epub, kindle and other format this book has been release on 2009 with categories.