A Comparative Study Of Short Term Electric Vehicle Load Forecasting Using Data Driven Multivariate Probabilistic Deepar Approach
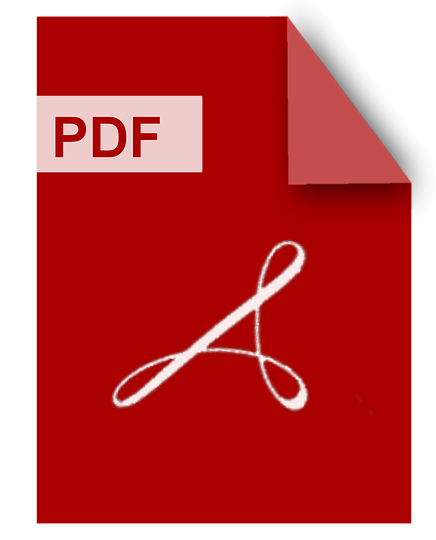
DOWNLOAD
Download A Comparative Study Of Short Term Electric Vehicle Load Forecasting Using Data Driven Multivariate Probabilistic Deepar Approach PDF/ePub or read online books in Mobi eBooks. Click Download or Read Online button to get A Comparative Study Of Short Term Electric Vehicle Load Forecasting Using Data Driven Multivariate Probabilistic Deepar Approach book now. This website allows unlimited access to, at the time of writing, more than 1.5 million titles, including hundreds of thousands of titles in various foreign languages. If the content not found or just blank you must refresh this page
A Comparative Study Of Short Term Electric Vehicle Load Forecasting Using Data Driven Multivariate Probabilistic Deepar Approach
DOWNLOAD
Author : Aidin Vahidmohammadi
language : en
Publisher:
Release Date : 2021
A Comparative Study Of Short Term Electric Vehicle Load Forecasting Using Data Driven Multivariate Probabilistic Deepar Approach written by Aidin Vahidmohammadi and has been published by this book supported file pdf, txt, epub, kindle and other format this book has been release on 2021 with categories.
With the surge of electric vehicles (EVs) and consequently the increase in power consumption, the power grid is facing many new challenges. Charging load forecasting remains one of the key challenges, that if not effectively scheduled, it may result in instability and quality-related issues in power systems. In recent years, numerous load forecasting techniques using machine learning and deep learning were proposed for predictions covering both commercial and household demands. However, there are very few studies that employed these methods to predict EV charging load behavior. This thesis proposes a multivariate RNN-based deep learning framework to forecast the short-term data-driven EV charging loads on two specific datasets for residential and workplace usage. In this research, a few popular deep learning models have been comparatively investigated to evaluate the forecasting performance of the proposed multivariate DeepAR model, a recurrent neural network-based model, as well as its univariate model on the historical charging data with exogenous variables. The 5-tuples input data used in this research include charging start time, duration of charging, charging load, time of use electricity price, and weekdays/weekends that were collected from three different locations and categorized into residential and workplace/parking lot scenarios. The short-term load forecasting algorithm in this study has been utilized multi-step daily horizons as one, three, seven and fifteens days ahead for the prediction window. Numerical results show that the multivariate DeepAR algorithm persists with manifestly higher stability and accuracy over multi-step daily prediction horizons. Its symmetric mean absolute percentage error (SMAPE) and mean absolute scaled error (MASE) are maintained at 1.9% and 4.95%, respectively, and outperform by a significant margin all other investigated deep learning and statistical models on the provided EV historical charging datasets. Eventually, the proposed framework can be further employed to formulate a more complex approach regarding charging load management at charging stations to maximize the load factor as well as balancing and flattening peak loads on the grid system.
Comparative Models For Electrical Load Forecasting
DOWNLOAD
Author : Derek W. Bunn
language : en
Publisher:
Release Date : 1985
Comparative Models For Electrical Load Forecasting written by Derek W. Bunn and has been published by this book supported file pdf, txt, epub, kindle and other format this book has been release on 1985 with Business & Economics categories.
Takes a practical look at how short-term forecasting has actually been undertaken and is being developed in public utility organizations.
Short Term Load Forecasting By Artificial Intelligent Technologies
DOWNLOAD
Author : Wei-Chiang Hong
language : en
Publisher: MDPI
Release Date : 2019-01-29
Short Term Load Forecasting By Artificial Intelligent Technologies written by Wei-Chiang Hong and has been published by MDPI this book supported file pdf, txt, epub, kindle and other format this book has been release on 2019-01-29 with categories.
This book is a printed edition of the Special Issue "Short-Term Load Forecasting by Artificial Intelligent Technologies" that was published in Energies
Stochastic Range Estimation Algorithms For Electric Vehicles Using Data Driven Learning Models
DOWNLOAD
Author : Scheubner, Stefan
language : en
Publisher: KIT Scientific Publishing
Release Date : 2022-06-03
Stochastic Range Estimation Algorithms For Electric Vehicles Using Data Driven Learning Models written by Scheubner, Stefan and has been published by KIT Scientific Publishing this book supported file pdf, txt, epub, kindle and other format this book has been release on 2022-06-03 with Technology & Engineering categories.
This work aims at improving the energy consumption forecast of electric vehicles by enhancing the prediction with a notion of uncertainty. The algorithm itself learns from driver and traffic data in a training set to generate accurate, driver-individual energy consumption forecasts.
On Short Term Load Forecasting Using Machine Learning Techniques
DOWNLOAD
Author : Behnam Farsi
language : en
Publisher:
Release Date : 2021
On Short Term Load Forecasting Using Machine Learning Techniques written by Behnam Farsi and has been published by this book supported file pdf, txt, epub, kindle and other format this book has been release on 2021 with categories.
Since electricity plays a crucial role in industrial infrastructures of countries, power companies are trying to monitor and control infrastructures to improve energy management, scheduling and develop efficiency plans. Smart Grids are an example of critical infrastructure which can lead to huge advantages such as providing higher resilience and reducing maintenance cost. Due to the nonlinear nature of electric load data there are high levels of uncertainties in predicting future load. Accurate forecasting is a critical task for stable and efficient energy supply, where load and supply are matched. However, this non-linear nature of loads presents significant challenges for forecasting. Many studies have been carried out on different algorithms for electricity load forecasting including; Deep Neural Networks, Regression-based methods, ARIMA and seasonal ARIMA (SARIMA) which among the most popular ones. This thesis discusses various algorithms analyze their performance for short-term load forecasting. In addition, a new hybrid deep learning model which combines long short-term memory (LSTM) and a convolutional neural network (CNN) has been proposed to carry out load forecasting without using any exogenous variables. The difference between our proposed model and previously hybrid CNN-LSTM models is that in those models, CNN is usually used to extract features while our proposed model focuses on the existing connection between LSTM and CNN. This methodology helps to increase the model's accuracy since the trend analysis and feature extraction process are accomplished, respectively, and they have no effect on each other during these processes. Two real-world data sets, namely "hourly load consumption of Malaysia" as well as "daily power electric consumption of Germany", are used to test and compare the presented models. To evaluate the performance of the tested models, root mean squared error (RMSE), mean absolute percentage error (MAPE) and R-squared were used. The results show that deep neural networks models are good candidates for being used as short-term prediction tools. Moreover, the proposed model improved the accuracy from 83.17\% for LSTM to 91.18\% for the German data. Likewise, the proposed model's accuracy in Malaysian case is 98.23\% which is an excellent result in load forecasting. In total, this thesis is divided into two parts, first part tries to find the best technique for short-term load forecasting, and then in second part the performance of the best technique is discussed. Since the proposed model has the best performance in the first part, this model is challenged to predict the load data of next day, next two days and next 10 days of Malaysian data set as well as next 7 days, next 10 days and next 30 days of German data set. The results show that the proposed model also has performed well where the accuracy of 10 days ahead of Malaysian data is 94.16\% and 30 days ahead of German data is 82.19\%. Since both German and Malaysian data sets are highly aggregated data, a data set from a research building in France is used to challenge the proposed model's performance. The average accuracy from the French experiment is almost 77\% which is reasonable for such a complex data without using any auxiliary variables. However, as Malaysian data and French data includes hourly weather data, the performance of the model after adding weather is evaluated to compare them before using weather data. Results show that weather data can have a positive influence on the model. These results show the strength of the proposed model and how much it is stable in front of some challenging tasks such as forecasting in different time horizons using two different data sets and working with complex data.
Recurrent Neural Networks For Short Term Load Forecasting
DOWNLOAD
Author : Filippo Maria Bianchi
language : en
Publisher: Springer
Release Date : 2017-11-09
Recurrent Neural Networks For Short Term Load Forecasting written by Filippo Maria Bianchi and has been published by Springer this book supported file pdf, txt, epub, kindle and other format this book has been release on 2017-11-09 with Computers categories.
The key component in forecasting demand and consumption of resources in a supply network is an accurate prediction of real-valued time series. Indeed, both service interruptions and resource waste can be reduced with the implementation of an effective forecasting system. Significant research has thus been devoted to the design and development of methodologies for short term load forecasting over the past decades. A class of mathematical models, called Recurrent Neural Networks, are nowadays gaining renewed interest among researchers and they are replacing many practical implementations of the forecasting systems, previously based on static methods. Despite the undeniable expressive power of these architectures, their recurrent nature complicates their understanding and poses challenges in the training procedures. Recently, new important families of recurrent architectures have emerged and their applicability in the context of load forecasting has not been investigated completely yet. This work performs a comparative study on the problem of Short-Term Load Forecast, by using different classes of state-of-the-art Recurrent Neural Networks. The authors test the reviewed models first on controlled synthetic tasks and then on different real datasets, covering important practical cases of study. The text also provides a general overview of the most important architectures and defines guidelines for configuring the recurrent networks to predict real-valued time series.
Short Term Forecasting Of Electric Loads Using Nonlinear Autoregressive Artificial Neural Networks With Exogenous Multivariable Inputs
DOWNLOAD
Author : Jaime H. Buitrago
language : en
Publisher:
Release Date : 2017
Short Term Forecasting Of Electric Loads Using Nonlinear Autoregressive Artificial Neural Networks With Exogenous Multivariable Inputs written by Jaime H. Buitrago and has been published by this book supported file pdf, txt, epub, kindle and other format this book has been release on 2017 with categories.
Short-term load forecasting is crucial for the operations planning of an electrical grid. Forecasting the next 24 h of electrical load in a grid allows operators to plan and optimize their resources. The purpose of this study is to develop a more accurate short-term load forecasting method utilizing non-linear autoregressive artificial neural networks (ANN) with exogenous multi-variable input (NARX). The proposed implementation of the network is new: the neural network is trained in open-loop using actual load and weather data, and then, the network is placed in closed-loop to generate a forecast using the predicted load as the feedback input. Unlike the existing short-term load forecasting methods using ANNs, the proposed method uses its own output as the input in order to improve the accuracy, thus effectively implementing a feedback loop for the load, making it less dependent on external data. Using the proposed framework, mean absolute percent errors in the forecast in the order of 1\% have been achieved, which is a 30\% improvement on the average error using feedforward ANNs, ARMAX and state space methods, which can result in large savings by avoiding commissioning of unnecessary power plants. In addition, in order to improve the robustness of the forecast to variations in the number of neurons and other network parameters, the author proposes a method using an exponential decay of the error weights for training the neural network. The modification consists in giving higher error weight to more recent values and lower weight to older values of the training set. By doing this, mover recent values have a higher influence on the calculation of the synaptic weights and therefore the forecast produced by the NARX network is more accurate. This method, combined with the use of Bayesian regularization for training, results in improved forecast accuracy of up to 25\% and robustness to variation in parameter selection. The New England electrical load data are used to train and validate the forecast prediction.
Statistical Learning Tools For Electricity Load Forecasting
DOWNLOAD
Author : Anestis Antoniadis
language : en
Publisher: Birkhäuser
Release Date : 2024-09-21
Statistical Learning Tools For Electricity Load Forecasting written by Anestis Antoniadis and has been published by Birkhäuser this book supported file pdf, txt, epub, kindle and other format this book has been release on 2024-09-21 with Mathematics categories.
This monograph explores a set of statistical and machine learning tools that can be effectively utilized for applied data analysis in the context of electricity load forecasting. Drawing on their substantial research and experience with forecasting electricity demand in industrial settings, the authors guide readers through several modern forecasting methods and tools from both industrial and applied perspectives – generalized additive models (GAMs), probabilistic GAMs, functional time series and wavelets, random forests, aggregation of experts, and mixed effects models. A collection of case studies based on sizable high-resolution datasets, together with relevant R packages, then illustrate the implementation of these techniques. Five real datasets at three different levels of aggregation (nation-wide, region-wide, or individual) from four different countries (UK, France, Ireland, and the USA) are utilized to study five problems: short-term point-wise forecasting, selection of relevant variables for prediction, construction of prediction bands, peak demand prediction, and use of individual consumer data. This text is intended for practitioners, researchers, and post-graduate students working on electricity load forecasting; it may also be of interest to applied academics or scientists wanting to learn about cutting-edge forecasting tools for application in other areas. Readers are assumed to be familiar with standard statistical concepts such as random variables, probability density functions, and expected values, and to possess some minimal modeling experience.
Machine Learning For Public Ev Charging Station Forecasting
DOWNLOAD
Author : Alexander Orzechowski
language : en
Publisher:
Release Date : 2021
Machine Learning For Public Ev Charging Station Forecasting written by Alexander Orzechowski and has been published by this book supported file pdf, txt, epub, kindle and other format this book has been release on 2021 with categories.
"The year-after-year increasing popularity of electric vehicles will cause unprecedented issues in managing the supply of electricity and charging spaces. It is in the interest of utility providers and everyday consumers to be able to plan for peaks and congestion. While past work has been done for localized, short-term forecasting, it has not included longer term forecasting, spatial diversity, while also lacking an exploration of features. This thesis proposes a methodology to forecast demand at public EV charging stations. We explore the potential of data-driven models to predict demand for the medium term or up to one week in advance. Moreover, we take into account not only the temporal-spatial relationship between an EV and a station, but also between different stations. To the best of our knowledge, this is the first study to propose machine learning to forecast medium-term public EV charging demand, to exploit weather and other features, and to study different stations and networks. The method was validated using data from eleven stations over three years from Scotland, UK. The prediction horizon is seven days. Our method predicts demand of a whole network with a MAE of 124.7kWh and SMAPE of 5.9%"--
Energy Management Strategies And Evaluation For Plug In Electric Vehicles On And Off The Road
DOWNLOAD
Author : Guanchen Zhang
language : en
Publisher:
Release Date : 2017
Energy Management Strategies And Evaluation For Plug In Electric Vehicles On And Off The Road written by Guanchen Zhang and has been published by this book supported file pdf, txt, epub, kindle and other format this book has been release on 2017 with categories.
Electric vehicle (EV) industries are driven by new technologies in batteries and powertrains. This thesis studies the cutting-edge Formula E racing vehicles with vehicle simulation and optimization for energy efficiency. On the consumer side, a new challenge EVs introduce is the need for large-scale charging infrastructure with minimum grid impact. This thesis studies EV charging management on the daily basis, featuring practical smart charging solutions at public locations and bi-directional (dis)charging at workplace and residence. Techniques that support smart charging are also studied. A data-mining based load disaggregation approach is developed to evaluate the general energy usage in the residential context. A machine-learning based load forecasting model is proposed to predict short-term residential loads in ultra-small scales. Overall, this thesis anticipates every aspect of EVs' daily activities, whether it is on or off the road, and suggests solutions to maximizing EV utilization for both drivers and the smart grid.