Anatomical Priors For Fully Automated Medical Image Segmentation
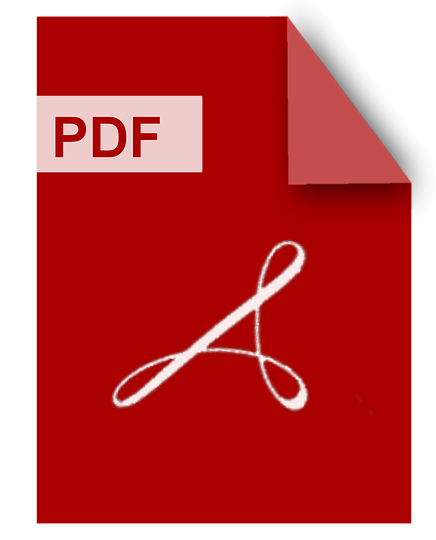
DOWNLOAD
Download Anatomical Priors For Fully Automated Medical Image Segmentation PDF/ePub or read online books in Mobi eBooks. Click Download or Read Online button to get Anatomical Priors For Fully Automated Medical Image Segmentation book now. This website allows unlimited access to, at the time of writing, more than 1.5 million titles, including hundreds of thousands of titles in various foreign languages. If the content not found or just blank you must refresh this page
Anatomical Priors For Fully Automated Medical Image Segmentation
DOWNLOAD
Author : Duc Duy Pham
language : en
Publisher:
Release Date : 2023
Anatomical Priors For Fully Automated Medical Image Segmentation written by Duc Duy Pham and has been published by this book supported file pdf, txt, epub, kindle and other format this book has been release on 2023 with categories.
Deformable Meshes For Medical Image Segmentation
DOWNLOAD
Author : Dagmar Kainmueller
language : en
Publisher: Springer
Release Date : 2014-08-18
Deformable Meshes For Medical Image Segmentation written by Dagmar Kainmueller and has been published by Springer this book supported file pdf, txt, epub, kindle and other format this book has been release on 2014-08-18 with Computers categories.
Segmentation of anatomical structures in medical image data is an essential task in clinical practice. Dagmar Kainmueller introduces methods for accurate fully automatic segmentation of anatomical structures in 3D medical image data. The author’s core methodological contribution is a novel deformation model that overcomes limitations of state-of-the-art Deformable Surface approaches, hence allowing for accurate segmentation of tip- and ridge-shaped features of anatomical structures. As for practical contributions, she proposes application-specific segmentation pipelines for a range of anatomical structures, together with thorough evaluations of segmentation accuracy on clinical image data. As compared to related work, these fully automatic pipelines allow for highly accurate segmentation of benchmark image data.
Information Processing In Medical Imaging
DOWNLOAD
Author : Jerry L. Prince
language : en
Publisher: Springer
Release Date : 2009-07-30
Information Processing In Medical Imaging written by Jerry L. Prince and has been published by Springer this book supported file pdf, txt, epub, kindle and other format this book has been release on 2009-07-30 with Computers categories.
This book constitutes the refeered proceedings of the 21st International Conference on Information Processing in Medical Imaging, IPMI 2009, held in Williamsburg, VA, USA, in July 2009 The 26 revised full papers and 33 revised poster papers presented were carefully reviewed and selected from 150 submissions. The papers are organized in topical sections on diffusion imaging, PET imaging, image registration, functional networks, space curves, tractography, microscopy, exploratory analyses, features and detection, image guided surgery, shape analysis, motion, and segmentation and validation.
Fully Automated Medical Image Analysis Facilitating Subsequent User Analysis
DOWNLOAD
Author : Shawn David Andrews
language : en
Publisher:
Release Date : 2014
Fully Automated Medical Image Analysis Facilitating Subsequent User Analysis written by Shawn David Andrews and has been published by this book supported file pdf, txt, epub, kindle and other format this book has been release on 2014 with categories.
In a clinical setting, accuracy is paramount for medical image analysis tasks such as segmentation and registration. Since it is often required that results be manually verified by a human expert, computational techniques designed to aid clinicians in these image analysis tasks are usually interactive, requiring user input. However, these techniques cannot take advantage of the time between when an image is acquired and when a clinician is available to provide input. In this thesis, we will present novel techniques for automatically processing medical images, with the goal of facilitating later analysis by a human expert. These techniques fall into two classes. The first class involves leveraging prior anatomical information to automatically generate results that are robust and independent of initialization. The second class involves precomputing data that is used to greatly increase the speed and responsiveness of subsequent interactive techniques, saving clinicians valuable time. Each of the techniques presented focus on encoding meaningful uncertainty information, which can guide human experts to potential errors or pathologies.
Multi Modality State Of The Art Medical Image Segmentation And Registration Methodologies
DOWNLOAD
Author : Ayman S. El-Baz
language : en
Publisher: Springer Science & Business Media
Release Date : 2011-04-11
Multi Modality State Of The Art Medical Image Segmentation And Registration Methodologies written by Ayman S. El-Baz and has been published by Springer Science & Business Media this book supported file pdf, txt, epub, kindle and other format this book has been release on 2011-04-11 with Medical categories.
With the advances in image guided surgery for cancer treatment, the role of image segmentation and registration has become very critical. The central engine of any image guided surgery product is its ability to quantify the organ or segment the organ whether it is a magnetic resonance imaging (MRI) and computed tomography (CT), X-ray, PET, SPECT, Ultrasound, and Molecular imaging modality. Sophisticated segmentation algorithms can help the physicians delineate better the anatomical structures present in the input images, enhance the accuracy of medical diagnosis and facilitate the best treatment planning system designs. The focus of this book in towards the state of the art techniques in the area of image segmentation and registration.
From Fully Supervised Single Task To Semi Supervised Multi Task Deep Learning Architectures For Segmentation In Medical Imaging Applications
DOWNLOAD
Author : S. M. Kamrul Hasan
language : en
Publisher:
Release Date : 2023
From Fully Supervised Single Task To Semi Supervised Multi Task Deep Learning Architectures For Segmentation In Medical Imaging Applications written by S. M. Kamrul Hasan and has been published by this book supported file pdf, txt, epub, kindle and other format this book has been release on 2023 with Deep learning (Machine learning) categories.
"Medical imaging is routinely performed in clinics worldwide for the diagnosis and treatment of numerous medical conditions in children and adults. With the advent of these medical imaging modalities, radiologists can visualize both the structure of the body as well as the tissues within the body. However, analyzing these high-dimensional (2D/3D/4D) images demands a significant amount of time and effort from radiologists. Hence, there is an ever-growing need for medical image computing tools to extract relevant information from the image data to help radiologists perform efficiently. Image analysis based on machine learning has pivotal potential to improve the entire medical imaging pipeline, providing support for clinical decision-making and computer-aided diagnosis. To be effective in addressing challenging image analysis tasks such as classification, detection, registration, and segmentation, specifically for medical imaging applications, deep learning approaches have shown significant improvement in performance. While deep learning has shown its potential in a variety of medical image analysis problems including segmentation, motion estimation, etc., generalizability is still an unsolved problem and many of these successes are achieved at the cost of a large pool of datasets. For most practical applications, getting access to a copious dataset can be very difficult, often impossible. Annotation is tedious and time-consuming. This cost is further amplified when annotation must be done by a clinical expert in medical imaging applications. Additionally, the applications of deep learning in the real-world clinical setting are still limited due to the lack of reliability caused by the limited prediction capabilities of some deep learning models. Moreover, while using a CNN in an automated image analysis pipeline, it’s critical to understand which segmentation results are problematic and require further manual examination. To this extent, the estimation of uncertainty calibration in a semi-supervised setting for medical image segmentation is still rarely reported. This thesis focuses on developing and evaluating optimized machine learning models for a variety of medical imaging applications, ranging from fully-supervised, single-task learning to semi-supervised, multi-task learning that makes efficient use of annotated training data. The contributions of this dissertation are as follows: (1) developing a fully-supervised, single-task transfer learning for the surgical instrument segmentation from laparoscopic images; and (2) utilizing supervised, single-task, transfer learning for segmenting and digitally removing the surgical instruments from endoscopic/laparoscopic videos to allow the visualization of the anatomy being obscured by the tool. The tool removal algorithms use a tool segmentation mask and either instrument-free reference frames or previous instrument-containing frames to fill in (inpaint) the instrument segmentation mask; (3) developing fully-supervised, single-task learning via efficient weight pruning and learned group convolution for accurate left ventricle (LV), right ventricle (RV) blood pool and myocardium localization and segmentation from 4D cine cardiac MR images; (4) demonstrating the use of our fully-supervised memory-efficient model to generate dynamic patient-specific right ventricle (RV) models from cine cardiac MRI dataset via an unsupervised learning-based deformable registration field; and (5) integrating a Monte Carlo dropout into our fully-supervised memory-efficient model with inherent uncertainty estimation, with the overall goal to estimate the uncertainty associated with the obtained segmentation and error, as a means to flag regions that feature less than optimal segmentation results; (6) developing semi-supervised, single-task learning via self-training (through meta pseudo-labeling) in concert with a Teacher network that instructs the Student network by generating pseudo-labels given unlabeled input data; (7) proposing largely-unsupervised, multi-task learning to demonstrate the power of a simple combination of a disentanglement block, variational autoencoder (VAE), generative adversarial network (GAN), and a conditioning layer-based reconstructor for performing two of the foremost critical tasks in medical imaging — segmentation of cardiac structures and reconstruction of the cine cardiac MR images; (8) demonstrating the use of 3D semi-supervised, multi-task learning for jointly learning multiple tasks in a single backbone module – uncertainty estimation, geometric shape generation, and cardiac anatomical structure segmentation of the left atrial cavity from 3D Gadolinium-enhanced magnetic resonance (GE-MR) images. This dissertation summarizes the impact of the contributions of our work in terms of demonstrating the adaptation and use of deep learning architectures featuring different levels of supervision to build a variety of image segmentation tools and techniques that can be used across a wide spectrum of medical image computing applications centered on facilitating and promoting the wide-spread computer-integrated diagnosis and therapy data science."--Abstract.
Prior Knowledge For Targeted Object Segmentation In Medical Images
DOWNLOAD
Author : Seyed Masoud Nosrati
language : en
Publisher:
Release Date : 2015
Prior Knowledge For Targeted Object Segmentation In Medical Images written by Seyed Masoud Nosrati and has been published by this book supported file pdf, txt, epub, kindle and other format this book has been release on 2015 with categories.
Medical image segmentation, the task of partitioning an image into meaningful parts, is an important step toward automating medical image analysis and is at the crux of a variety of medical imaging applications, such as computer aided diagnosis, therapy planning and delivery, and computer aided interventions. However, existence of noise, low contrast and objects' complexity in medical images preclude ideal segmentation. Incorporating prior knowledge into image segmentation algorithms has proven useful for obtaining more accurate and plausible results on targeted objects segmentation. In this thesis, we develop novel techniques to augment optimization-based segmentation frameworks with different types of prior knowledge to identify and delineate only those objects (targeted objects) that conform to specific geometrical, topological and appearance priors. These techniques include employing prior knowledge to segment multi-part objects with part-configuration constraints and encoding priors based on images acquired from different imaging equipment and of differing dimensions. Our objective is to satisfy two important aspects in optimization-based image segmentation: (1) fidelity-optimizability trade-off, and (2) space and time complexity.Particularly, in our first contribution, we adopt several prior information to build a faithful objective function unconcerned about its convexity to segment potentially overlapping cells with complex topology. In our second contribution, we improve the space and time complexity and augment the level sets framework with the ability to handle geometric constraints between boundaries of multi-region objects. In our first two contributions we opt for ensuring the objective function is flexible enough (even if it is non-convex) to accurately capture the intricacies of the segmentation problem. In our third contribution, we focus on optimizability. We propose a convex formulation to augment the popular Mumford-Shah model and develop a new regularization term to incorporate similar geometrical and distance prior as our second contribution while maintaining global optimality. Lastly, we efficiently incorporate different types of priors based on images acquired from different imaging equipment (different modalities) and of dissimilar dimensions to segment multiple objects in intraoperative multi-view endoscopic videos. We show how our technique allows for the inclusion of laparoscopic camera motion model to stabilize the segmentation.
Marginal Space Learning For Medical Image Analysis
DOWNLOAD
Author : Yefeng Zheng
language : en
Publisher: Springer
Release Date : 2016-09-03
Marginal Space Learning For Medical Image Analysis written by Yefeng Zheng and has been published by Springer this book supported file pdf, txt, epub, kindle and other format this book has been release on 2016-09-03 with Computers categories.
Automatic detection and segmentation of anatomical structures in medical images are prerequisites to subsequent image measurements and disease quantification, and therefore have multiple clinical applications. This book presents an efficient object detection and segmentation framework, called Marginal Space Learning, which runs at a sub-second speed on a current desktop computer, faster than the state-of-the-art. Trained with a sufficient number of data sets, Marginal Space Learning is also robust under imaging artifacts, noise and anatomical variations. The book showcases 35 clinical applications of Marginal Space Learning and its extensions to detecting and segmenting various anatomical structures, such as the heart, liver, lymph nodes and prostate in major medical imaging modalities (CT, MRI, X-Ray and Ultrasound), demonstrating its efficiency and robustness.
Information Processing In Medical Imaging
DOWNLOAD
Author : Jerry L. Prince
language : en
Publisher: Springer Science & Business Media
Release Date : 2009-06-19
Information Processing In Medical Imaging written by Jerry L. Prince and has been published by Springer Science & Business Media this book supported file pdf, txt, epub, kindle and other format this book has been release on 2009-06-19 with Computers categories.
This book constitutes the refeered proceedings of the 21st International Conference on Information Processing in Medical Imaging, IPMI 2009, held in Williamsburg, VA, USA, in July 2009 The 26 revised full papers and 33 revised poster papers presented were carefully reviewed and selected from 150 submissions. The papers are organized in topical sections on diffusion imaging, PET imaging, image registration, functional networks, space curves, tractography, microscopy, exploratory analyses, features and detection, image guided surgery, shape analysis, motion, and segmentation and validation.
Robust Medical Image Recognition And Segmentation
DOWNLOAD
Author : Zhennan Yan
language : en
Publisher:
Release Date : 2016
Robust Medical Image Recognition And Segmentation written by Zhennan Yan and has been published by this book supported file pdf, txt, epub, kindle and other format this book has been release on 2016 with Computer vision categories.
In recent decades, with increasing amount of medical data, clinical trials are designed and conducted to explore whether a medical strategy, treatment, or device is safe and effective for humans. In clinical trials, due to the large variance of collected image data and limited golden standard training samples, designing a robust and automated algorithm or framework for quantitative medical image analysis is still challenging and active field of research. Many state-of-the-art algorithms are designed/trained for specific anatomies or tasks with corresponding prior knowledge. In this dissertation, we focus on robust and easy-to-use solutions for two fundamental and key modules in medical image analysis, specifically, anatomy recognition and segmentation. The medical image recognition is formulated as a classification problem to identify the body section from which the image is taken. The problem is solved by a patch-based convolutional neural network (CNN). The proposed method can utilize the image-level label to discover discriminative local patches without local annotations and train classifier using these local features. Its performance in our application is superior to conventional models using ad-hoc designed features as well as standard CNN. Accurate and efficient image recognition serves as a reliable initialization module for anatomy segmentation algorithms. In medical image segmentation, precise labeling usually relies on prior knowledge due to ambiguous visual clues of different anatomies and between-subject variance. We use Gaussian Mixture Model and Markov Random Field to model the appearances and spatial relationships of voxels in medical image. To finely utilize the prior knowledge from training atlases (medical image and its corresponding label image), we design an adaptive statistical atlas based method to segment new subjects which could be very different from the training samples. The method is shown robust and accurate in brain segmentation and can be easily applied in other applications.