Applications Of Sparse Representation Compressive Sensing
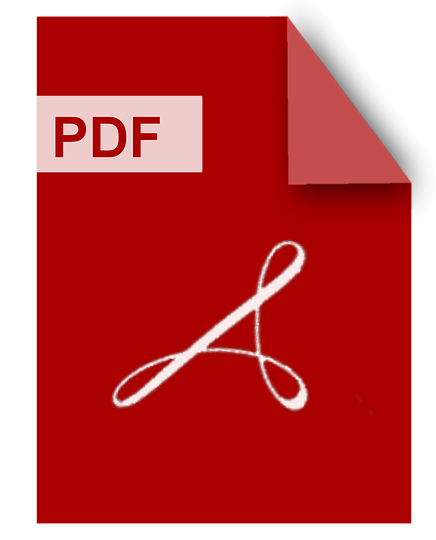
DOWNLOAD
Download Applications Of Sparse Representation Compressive Sensing PDF/ePub or read online books in Mobi eBooks. Click Download or Read Online button to get Applications Of Sparse Representation Compressive Sensing book now. This website allows unlimited access to, at the time of writing, more than 1.5 million titles, including hundreds of thousands of titles in various foreign languages. If the content not found or just blank you must refresh this page
Applications Of Sparse Representation Compressive Sensing
DOWNLOAD
Author : R. G. Baraniuk
language : en
Publisher:
Release Date : 2010
Applications Of Sparse Representation Compressive Sensing written by R. G. Baraniuk and has been published by this book supported file pdf, txt, epub, kindle and other format this book has been release on 2010 with categories.
Sparse Representation Of Visual Data For Compression And Compressed Sensing
DOWNLOAD
Author : Ehsan Miandji
language : en
Publisher: Linköping University Electronic Press
Release Date : 2018-11-23
Sparse Representation Of Visual Data For Compression And Compressed Sensing written by Ehsan Miandji and has been published by Linköping University Electronic Press this book supported file pdf, txt, epub, kindle and other format this book has been release on 2018-11-23 with categories.
The ongoing advances in computational photography have introduced a range of new imaging techniques for capturing multidimensional visual data such as light fields, BRDFs, BTFs, and more. A key challenge inherent to such imaging techniques is the large amount of high dimensional visual data that is produced, often requiring GBs, or even TBs, of storage. Moreover, the utilization of these datasets in real time applications poses many difficulties due to the large memory footprint. Furthermore, the acquisition of large-scale visual data is very challenging and expensive in most cases. This thesis makes several contributions with regards to acquisition, compression, and real time rendering of high dimensional visual data in computer graphics and imaging applications. Contributions of this thesis reside on the strong foundation of sparse representations. Numerous applications are presented that utilize sparse representations for compression and compressed sensing of visual data. Specifically, we present a single sensor light field camera design, a compressive rendering method, a real time precomputed photorealistic rendering technique, light field (video) compression and real time rendering, compressive BRDF capture, and more. Another key contribution of this thesis is a general framework for compression and compressed sensing of visual data, regardless of the dimensionality. As a result, any type of discrete visual data with arbitrary dimensionality can be captured, compressed, and rendered in real time. This thesis makes two theoretical contributions. In particular, uniqueness conditions for recovering a sparse signal under an ensemble of multidimensional dictionaries is presented. The theoretical results discussed here are useful for designing efficient capturing devices for multidimensional visual data. Moreover, we derive the probability of successful recovery of a noisy sparse signal using OMP, one of the most widely used algorithms for solving compressed sensing problems.
Compressed Sensing
DOWNLOAD
Author : Yonina C. Eldar
language : en
Publisher: Cambridge University Press
Release Date : 2012-05-17
Compressed Sensing written by Yonina C. Eldar and has been published by Cambridge University Press this book supported file pdf, txt, epub, kindle and other format this book has been release on 2012-05-17 with Mathematics categories.
A detailed presentation of compressed sensing by leading researchers, covering the most significant theoretical and application-oriented advances.
Sparse Representations And Compressive Sensing For Imaging And Vision
DOWNLOAD
Author : Vishal M. Patel
language : en
Publisher: Springer Science & Business Media
Release Date : 2013-02-11
Sparse Representations And Compressive Sensing For Imaging And Vision written by Vishal M. Patel and has been published by Springer Science & Business Media this book supported file pdf, txt, epub, kindle and other format this book has been release on 2013-02-11 with Technology & Engineering categories.
Compressed sensing or compressive sensing is a new concept in signal processing where one measures a small number of non-adaptive linear combinations of the signal. These measurements are usually much smaller than the number of samples that define the signal. From these small numbers of measurements, the signal is then reconstructed by non-linear procedure. Compressed sensing has recently emerged as a powerful tool for efficiently processing data in non-traditional ways. In this book, we highlight some of the key mathematical insights underlying sparse representation and compressed sensing and illustrate the role of these theories in classical vision, imaging and biometrics problems.
Sparse Representation Modeling And Learning In Visual Recognition
DOWNLOAD
Author : Hong Cheng
language : en
Publisher: Springer
Release Date : 2015-05-25
Sparse Representation Modeling And Learning In Visual Recognition written by Hong Cheng and has been published by Springer this book supported file pdf, txt, epub, kindle and other format this book has been release on 2015-05-25 with Computers categories.
This unique text/reference presents a comprehensive review of the state of the art in sparse representations, modeling and learning. The book examines both the theoretical foundations and details of algorithm implementation, highlighting the practical application of compressed sensing research in visual recognition and computer vision. Topics and features: describes sparse recovery approaches, robust and efficient sparse representation, and large-scale visual recognition; covers feature representation and learning, sparsity induced similarity, and sparse representation and learning-based classifiers; discusses low-rank matrix approximation, graphical models in compressed sensing, collaborative representation-based classification, and high-dimensional nonlinear learning; includes appendices outlining additional computer programming resources, and explaining the essential mathematics required to understand the book.
Compressive Sensing For Computer Vision And Image Processing
DOWNLOAD
Author : Naveen Kulkarni
language : en
Publisher:
Release Date : 2011
Compressive Sensing For Computer Vision And Image Processing written by Naveen Kulkarni and has been published by this book supported file pdf, txt, epub, kindle and other format this book has been release on 2011 with Computer vision categories.
With the introduction of compressed sensing and sparse representation, many image processing and computer vision problems have been looked at in a new way. Recent trends indicate that many challenging computer vision and image processing problems are being solved using compressive sensing and sparse representation algorithms. This thesis assays some applications of compressive sensing and sparse representation with regards to image enhancement, restoration and classication. The first application deals with image Super-Resolution through compressive sensing based sparse representation. A novel framework is developed for understanding and analyzing some of the implications of compressive sensing in reconstruction and recovery of an image through raw-sampled and trained dictionaries. Properties of the projection operator and the dictionary are examined and the corresponding results presented. In the second application a novel technique for representing image classes uniquely in a high-dimensional space for image classification is presented. In this method, design and implementation strategy of the image classification system through unique affine sparse codes is presented, which leads to state of the art results. This further leads to analysis of some of the properties attributed to these unique sparse codes. In addition to obtaining these codes, a strong classier is designed and implemented to boost the results obtained. Evaluation with publicly available datasets shows that the proposed method outperforms other state of the art results in image classication. The final part of the thesis deals with image denoising with a novel approach towards obtaining high quality denoised image patches using only a single image. A new technique is proposed to obtain highly correlated image patches through sparse representation, which are then subjected to matrix completion to obtain high quality image patches. Experiments suggest that there may exist a structure within a noisy image which can be exploited for denoising through a low-rank constraint.
New Trends In Applied Harmonic Analysis
DOWNLOAD
Author : Akram Aldroubi
language : en
Publisher: Birkhäuser
Release Date : 2016-04-21
New Trends In Applied Harmonic Analysis written by Akram Aldroubi and has been published by Birkhäuser this book supported file pdf, txt, epub, kindle and other format this book has been release on 2016-04-21 with Mathematics categories.
This volume is a selection of written notes corresponding to courses taught at the CIMPA School: "New Trends in Applied Harmonic Analysis: Sparse Representations, Compressed Sensing and Multifractal Analysis". New interactions between harmonic analysis and signal and image processing have seen striking development in the last 10 years, and several technological deadlocks have been solved through the resolution of deep theoretical problems in harmonic analysis. New Trends in Applied Harmonic Analysis focuses on two particularly active areas that are representative of such advances: multifractal analysis, and sparse representation and compressed sensing. The contributions are written by leaders in these areas, and cover both theoretical aspects and applications. This work should prove useful not only to PhD students and postdocs in mathematics and signal and image processing, but also to researchers working in related topics.
Image Understanding Using Sparse Representations
DOWNLOAD
Author : Jayaraman J. Thiagarajan
language : en
Publisher: Springer Nature
Release Date : 2022-06-01
Image Understanding Using Sparse Representations written by Jayaraman J. Thiagarajan and has been published by Springer Nature this book supported file pdf, txt, epub, kindle and other format this book has been release on 2022-06-01 with Technology & Engineering categories.
Image understanding has been playing an increasingly crucial role in several inverse problems and computer vision. Sparse models form an important component in image understanding, since they emulate the activity of neural receptors in the primary visual cortex of the human brain. Sparse methods have been utilized in several learning problems because of their ability to provide parsimonious, interpretable, and efficient models. Exploiting the sparsity of natural signals has led to advances in several application areas including image compression, denoising, inpainting, compressed sensing, blind source separation, super-resolution, and classification. The primary goal of this book is to present the theory and algorithmic considerations in using sparse models for image understanding and computer vision applications. To this end, algorithms for obtaining sparse representations and their performance guarantees are discussed in the initial chapters. Furthermore, approaches for designing overcomplete, data-adapted dictionaries to model natural images are described. The development of theory behind dictionary learning involves exploring its connection to unsupervised clustering and analyzing its generalization characteristics using principles from statistical learning theory. An exciting application area that has benefited extensively from the theory of sparse representations is compressed sensing of image and video data. Theory and algorithms pertinent to measurement design, recovery, and model-based compressed sensing are presented. The paradigm of sparse models, when suitably integrated with powerful machine learning frameworks, can lead to advances in computer vision applications such as object recognition, clustering, segmentation, and activity recognition. Frameworks that enhance the performance of sparse models in such applications by imposing constraints based on the prior discriminatory information and the underlying geometrical structure, and kernelizing the sparse coding and dictionary learning methods are presented. In addition to presenting theoretical fundamentals in sparse learning, this book provides a platform for interested readers to explore the vastly growing application domains of sparse representations.
Sparse Representations For Radar With Matlab Examples
DOWNLOAD
Author : Peter Knee
language : en
Publisher: Springer Nature
Release Date : 2022-05-31
Sparse Representations For Radar With Matlab Examples written by Peter Knee and has been published by Springer Nature this book supported file pdf, txt, epub, kindle and other format this book has been release on 2022-05-31 with Technology & Engineering categories.
Although the field of sparse representations is relatively new, research activities in academic and industrial research labs are already producing encouraging results. The sparse signal or parameter model motivated several researchers and practitioners to explore high complexity/wide bandwidth applications such as Digital TV, MRI processing, and certain defense applications. The potential signal processing advancements in this area may influence radar technologies. This book presents the basic mathematical concepts along with a number of useful MATLAB® examples to emphasize the practical implementations both inside and outside the radar field. Table of Contents: Radar Systems: A Signal Processing Perspective / Introduction to Sparse Representations / Dimensionality Reduction / Radar Signal Processing Fundamentals / Sparse Representations in Radar
Compressed Sensing Sparse Filtering
DOWNLOAD
Author : Avishy Y. Carmi
language : en
Publisher: Springer Science & Business Media
Release Date : 2013-09-13
Compressed Sensing Sparse Filtering written by Avishy Y. Carmi and has been published by Springer Science & Business Media this book supported file pdf, txt, epub, kindle and other format this book has been release on 2013-09-13 with Technology & Engineering categories.
This book is aimed at presenting concepts, methods and algorithms ableto cope with undersampled and limited data. One such trend that recently gained popularity and to some extent revolutionised signal processing is compressed sensing. Compressed sensing builds upon the observation that many signals in nature are nearly sparse (or compressible, as they are normally referred to) in some domain, and consequently they can be reconstructed to within high accuracy from far fewer observations than traditionally held to be necessary. Apart from compressed sensing this book contains other related approaches. Each methodology has its own formalities for dealing with such problems. As an example, in the Bayesian approach, sparseness promoting priors such as Laplace and Cauchy are normally used for penalising improbable model variables, thus promoting low complexity solutions. Compressed sensing techniques and homotopy-type solutions, such as the LASSO, utilise l1-norm penalties for obtaining sparse solutions using fewer observations than conventionally needed. The book emphasizes on the role of sparsity as a machinery for promoting low complexity representations and likewise its connections to variable selection and dimensionality reduction in various engineering problems. This book is intended for researchers, academics and practitioners with interest in various aspects and applications of sparse signal processing.