Applying Machine Learning For Automated Classification Of Biomedical Data In Subject Independent Settings
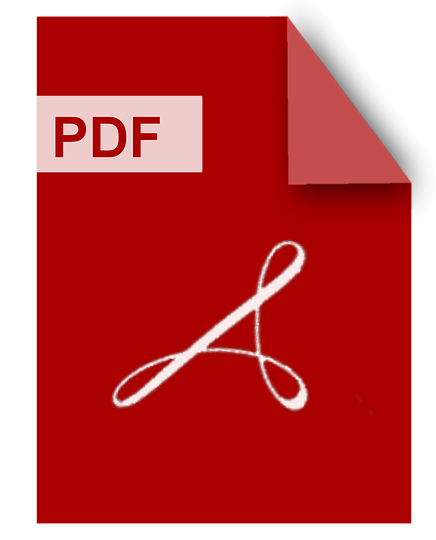
DOWNLOAD
Download Applying Machine Learning For Automated Classification Of Biomedical Data In Subject Independent Settings PDF/ePub or read online books in Mobi eBooks. Click Download or Read Online button to get Applying Machine Learning For Automated Classification Of Biomedical Data In Subject Independent Settings book now. This website allows unlimited access to, at the time of writing, more than 1.5 million titles, including hundreds of thousands of titles in various foreign languages. If the content not found or just blank you must refresh this page
Applying Machine Learning For Automated Classification Of Biomedical Data In Subject Independent Settings
DOWNLOAD
Author : Thuy T. Pham
language : en
Publisher: Springer
Release Date : 2018-08-23
Applying Machine Learning For Automated Classification Of Biomedical Data In Subject Independent Settings written by Thuy T. Pham and has been published by Springer this book supported file pdf, txt, epub, kindle and other format this book has been release on 2018-08-23 with Technology & Engineering categories.
This book describes efforts to improve subject-independent automated classification techniques using a better feature extraction method and a more efficient model of classification. It evaluates three popular saliency criteria for feature selection, showing that they share common limitations, including time-consuming and subjective manual de-facto standard practice, and that existing automated efforts have been predominantly used for subject dependent setting. It then proposes a novel approach for anomaly detection, demonstrating its effectiveness and accuracy for automated classification of biomedical data, and arguing its applicability to a wider range of unsupervised machine learning applications in subject-independent settings.
Computational Science And Its Applications Iccsa 2019
DOWNLOAD
Author : Sanjay Misra
language : en
Publisher: Springer
Release Date : 2019-06-28
Computational Science And Its Applications Iccsa 2019 written by Sanjay Misra and has been published by Springer this book supported file pdf, txt, epub, kindle and other format this book has been release on 2019-06-28 with Computers categories.
The six volumes LNCS 11619-11624 constitute the refereed proceedings of the 19th International Conference on Computational Science and Its Applications, ICCSA 2019, held in Saint Petersburg, Russia, in July 2019. The 64 full papers, 10 short papers and 259 workshop papers presented were carefully reviewed and selected form numerous submissions. The 64 full papers are organized in the following five general tracks: computational methods, algorithms and scientific applications; high performance computing and networks; geometric modeling, graphics and visualization; advanced and emerging applications; and information systems and technologies. The 259 workshop papers were presented at 33 workshops in various areas of computational sciences, ranging from computational science technologies to specific areas of computational sciences, such as software engineering, security, artificial intelligence and blockchain technologies.
Applications Of Machine Learning And Deep Learning On Biological Data
DOWNLOAD
Author : Faheem Masoodi
language : en
Publisher: CRC Press
Release Date : 2023-03-13
Applications Of Machine Learning And Deep Learning On Biological Data written by Faheem Masoodi and has been published by CRC Press this book supported file pdf, txt, epub, kindle and other format this book has been release on 2023-03-13 with Computers categories.
The automated learning of machines characterizes machine learning (ML). It focuses on making data-driven predictions using programmed algorithms. ML has several applications, including bioinformatics, which is a discipline of study and practice that deals with applying computational derivations to obtain biological data. It involves the collection, retrieval, storage, manipulation, and modeling of data for analysis or prediction made using customized software. Previously, comprehensive programming of bioinformatical algorithms was an extremely laborious task for such applications as predicting protein structures. Now, algorithms using ML and deep learning (DL) have increased the speed and efficacy of programming such algorithms. Applications of Machine Learning and Deep Learning on Biological Data is an examination of applying ML and DL to such areas as proteomics, genomics, microarrays, text mining, and systems biology. The key objective is to cover ML applications to biological science problems, focusing on problems related to bioinformatics. The book looks at cutting-edge research topics and methodologies in ML applied to the rapidly advancing discipline of bioinformatics. ML and DL applied to biological and neuroimaging data can open new frontiers for biomedical engineering, such as refining the understanding of complex diseases, including cancer and neurodegenerative and psychiatric disorders. Advances in this field could eventually lead to the development of precision medicine and automated diagnostic tools capable of tailoring medical treatments to individual lifestyles, variability, and the environment. Highlights include: Artificial Intelligence in treating and diagnosing schizophrenia An analysis of ML’s and DL’s financial effect on healthcare An XGBoost-based classification method for breast cancer classification Using ML to predict squamous diseases ML and DL applications in genomics and proteomics Applying ML and DL to biological data
Handbook Of Deep Learning In Biomedical Engineering And Health Informatics
DOWNLOAD
Author : E. Golden Julie
language : en
Publisher: CRC Press
Release Date : 2021-09-22
Handbook Of Deep Learning In Biomedical Engineering And Health Informatics written by E. Golden Julie and has been published by CRC Press this book supported file pdf, txt, epub, kindle and other format this book has been release on 2021-09-22 with Medical categories.
This new volume discusses state-of-the-art deep learning techniques and approaches that can be applied in biomedical systems and health informatics. Deep learning in the biomedical field is an effective method of collecting and analyzing data that can be used for the accurate diagnosis of disease. This volume delves into a variety of applications, techniques, algorithms, platforms, and tools used in this area, such as image segmentation, classification, registration, and computer-aided analysis. The editors proceed on the principle that accurate diagnosis of disease depends on image acquisition and interpretation. There are many methods to get high resolution radiological images, but we are still lacking in automated image interpretation. Currently deep learning techniques are providing a feasible solution for automatic diagnosis of disease with good accuracy. Analyzing clinical data using deep learning techniques enables clinicians to diagnose diseases at an early stage and treat patients more effectively. Chapters explore such approaches as deep learning algorithms, convolutional neural networks and recurrent neural network architecture, image stitching techniques, deep RNN architectures, and more. This volume also depicts how deep learning techniques can be applied for medical diagnostics of several specific health scenarios, such as cancer, COVID-19, acute neurocutaneous syndrome, cardiovascular and neuro diseases, skin lesions and skin cancer, etc. Key features: Introduces important recent technological advancements in the field Describes the various techniques, platforms, and tools used in biomedical deep learning systems Includes informative case studies that help to explain the new technologies Handbook of Deep Learning in Biomedical Engineering and Health Informatics provides a thorough exploration of biomedical systems applied with deep learning techniques and will provide valuable information for researchers, medical and industry practitioners, academicians, and students.
Practical Machine Learning For Data Analysis Using Python
DOWNLOAD
Author : Abdulhamit Subasi
language : en
Publisher: Academic Press
Release Date : 2020-06-05
Practical Machine Learning For Data Analysis Using Python written by Abdulhamit Subasi and has been published by Academic Press this book supported file pdf, txt, epub, kindle and other format this book has been release on 2020-06-05 with Computers categories.
Practical Machine Learning for Data Analysis Using Python is a problem solver’s guide for creating real-world intelligent systems. It provides a comprehensive approach with concepts, practices, hands-on examples, and sample code. The book teaches readers the vital skills required to understand and solve different problems with machine learning. It teaches machine learning techniques necessary to become a successful practitioner, through the presentation of real-world case studies in Python machine learning ecosystems. The book also focuses on building a foundation of machine learning knowledge to solve different real-world case studies across various fields, including biomedical signal analysis, healthcare, security, economics, and finance. Moreover, it covers a wide range of machine learning models, including regression, classification, and forecasting. The goal of the book is to help a broad range of readers, including IT professionals, analysts, developers, data scientists, engineers, and graduate students, to solve their own real-world problems. Offers a comprehensive overview of the application of machine learning tools in data analysis across a wide range of subject areas Teaches readers how to apply machine learning techniques to biomedical signals, financial data, and healthcare data Explores important classification and regression algorithms as well as other machine learning techniques Explains how to use Python to handle data extraction, manipulation, and exploration techniques, as well as how to visualize data spread across multiple dimensions and extract useful features
Signal Processing And Machine Learning For Biomedical Big Data
DOWNLOAD
Author : Ervin Sejdic
language : en
Publisher: CRC Press
Release Date : 2018-07-04
Signal Processing And Machine Learning For Biomedical Big Data written by Ervin Sejdic and has been published by CRC Press this book supported file pdf, txt, epub, kindle and other format this book has been release on 2018-07-04 with Medical categories.
Within the healthcare domain, big data is defined as any ``high volume, high diversity biological, clinical, environmental, and lifestyle information collected from single individuals to large cohorts, in relation to their health and wellness status, at one or several time points.'' Such data is crucial because within it lies vast amounts of invaluable information that could potentially change a patient's life, opening doors to alternate therapies, drugs, and diagnostic tools. Signal Processing and Machine Learning for Biomedical Big Data thus discusses modalities; the numerous ways in which this data is captured via sensors; and various sample rates and dimensionalities. Capturing, analyzing, storing, and visualizing such massive data has required new shifts in signal processing paradigms and new ways of combining signal processing with machine learning tools. This book covers several of these aspects in two ways: firstly, through theoretical signal processing chapters where tools aimed at big data (be it biomedical or otherwise) are described; and, secondly, through application-driven chapters focusing on existing applications of signal processing and machine learning for big biomedical data. This text aimed at the curious researcher working in the field, as well as undergraduate and graduate students eager to learn how signal processing can help with big data analysis. It is the hope of Drs. Sejdic and Falk that this book will bring together signal processing and machine learning researchers to unlock existing bottlenecks within the healthcare field, thereby improving patient quality-of-life. Provides an overview of recent state-of-the-art signal processing and machine learning algorithms for biomedical big data, including applications in the neuroimaging, cardiac, retinal, genomic, sleep, patient outcome prediction, critical care, and rehabilitation domains. Provides contributed chapters from world leaders in the fields of big data and signal processing, covering topics such as data quality, data compression, statistical and graph signal processing techniques, and deep learning and their applications within the biomedical sphere. This book’s material covers how expert domain knowledge can be used to advance signal processing and machine learning for biomedical big data applications.
Deep Learning Machine Learning And Iot In Biomedical And Health Informatics
DOWNLOAD
Author : Sujata Dash
language : en
Publisher: CRC Press
Release Date : 2022-02-10
Deep Learning Machine Learning And Iot In Biomedical And Health Informatics written by Sujata Dash and has been published by CRC Press this book supported file pdf, txt, epub, kindle and other format this book has been release on 2022-02-10 with Computers categories.
Biomedical and Health Informatics is an important field that brings tremendous opportunities and helps address challenges due to an abundance of available biomedical data. This book examines and demonstrates state-of-the-art approaches for IoT and Machine Learning based biomedical and health related applications. This book aims to provide computational methods for accumulating, updating and changing knowledge in intelligent systems and particularly learning mechanisms that help us to induce knowledge from the data. It is helpful in cases where direct algorithmic solutions are unavailable, there is lack of formal models, or the knowledge about the application domain is inadequately defined. In the future IoT has the impending capability to change the way we work and live. These computing methods also play a significant role in design and optimization in diverse engineering disciplines. With the influence and the development of the IoT concept, the need for AI (artificial intelligence) techniques has become more significant than ever. The aim of these techniques is to accept imprecision, uncertainties and approximations to get a rapid solution. However, recent advancements in representation of intelligent IoTsystems generate a more intelligent and robust system providing a human interpretable, low-cost, and approximate solution. Intelligent IoT systems have demonstrated great performance to a variety of areas including big data analytics, time series, biomedical and health informatics. This book will be very beneficial for the new researchers and practitioners working in the biomedical and healthcare fields to quickly know the best performing methods. It will also be suitable for a wide range of readers who may not be scientists but who are also interested in the practice of such areas as medical image retrieval, brain image segmentation, among others. • Discusses deep learning, IoT, machine learning, and biomedical data analysis with broad coverage of basic scientific applications • Presents deep learning and the tremendous improvement in accuracy, robustness, and cross- language generalizability it has over conventional approaches • Discusses various techniques of IoT systems for healthcare data analytics • Provides state-of-the-art methods of deep learning, machine learning and IoT in biomedical and health informatics • Focuses more on the application of algorithms in various real life biomedical and engineering problems
Predictive Modeling In Biomedical Data Mining And Analysis
DOWNLOAD
Author : Sudipta Roy
language : en
Publisher: Elsevier
Release Date : 2022-08-26
Predictive Modeling In Biomedical Data Mining And Analysis written by Sudipta Roy and has been published by Elsevier this book supported file pdf, txt, epub, kindle and other format this book has been release on 2022-08-26 with Science categories.
Predictive Modeling in Biomedical Data Mining and Analysis presents major technical advancements and research findings in the field of machine learning in biomedical image and data analysis. The book examines recent technologies and studies in preclinical and clinical practice in computational intelligence. The authors present leading-edge research in the science of processing, analyzing and utilizing all aspects of advanced computational machine learning in biomedical image and data analysis. As the application of machine learning is spreading to a variety of biomedical problems, including automatic image segmentation, image classification, disease classification, fundamental biological processes, and treatments, this is an ideal reference. Machine Learning techniques are used as predictive models for many types of applications, including biomedical applications. These techniques have shown impressive results across a variety of domains in biomedical engineering research. Biology and medicine are data-rich disciplines, but the data are complex and often ill-understood, hence the need for new resources and information. Includes predictive modeling algorithms for both Supervised Learning and Unsupervised Learning for medical diagnosis, data summarization and pattern identification Offers complete coverage of predictive modeling in biomedical applications, including data visualization, information retrieval, data mining, image pre-processing and segmentation, mathematical models and deep neural networks Provides readers with leading-edge coverage of biomedical data processing, including high dimension data, data reduction, clinical decision-making, deep machine learning in large data sets, multimodal, multi-task, and transfer learning, as well as machine learning with Internet of Biomedical Things applications
Animal Biomechanics Application Of The Biomedical Engineering To The Veterinary Sciences For The Animal Healthcare
DOWNLOAD
Author : Mauro Malvè
language : en
Publisher: Frontiers Media SA
Release Date : 2024-04-01
Animal Biomechanics Application Of The Biomedical Engineering To The Veterinary Sciences For The Animal Healthcare written by Mauro Malvè and has been published by Frontiers Media SA this book supported file pdf, txt, epub, kindle and other format this book has been release on 2024-04-01 with Medical categories.
Automated Machine Learning
DOWNLOAD
Author : Frank Hutter
language : en
Publisher: Springer
Release Date : 2019-05-17
Automated Machine Learning written by Frank Hutter and has been published by Springer this book supported file pdf, txt, epub, kindle and other format this book has been release on 2019-05-17 with Computers categories.
This open access book presents the first comprehensive overview of general methods in Automated Machine Learning (AutoML), collects descriptions of existing systems based on these methods, and discusses the first series of international challenges of AutoML systems. The recent success of commercial ML applications and the rapid growth of the field has created a high demand for off-the-shelf ML methods that can be used easily and without expert knowledge. However, many of the recent machine learning successes crucially rely on human experts, who manually select appropriate ML architectures (deep learning architectures or more traditional ML workflows) and their hyperparameters. To overcome this problem, the field of AutoML targets a progressive automation of machine learning, based on principles from optimization and machine learning itself. This book serves as a point of entry into this quickly-developing field for researchers and advanced students alike, as well as providing a reference for practitioners aiming to use AutoML in their work.