Cross Validation And Regression Analysis In High Dimensional Sparse Linear Models
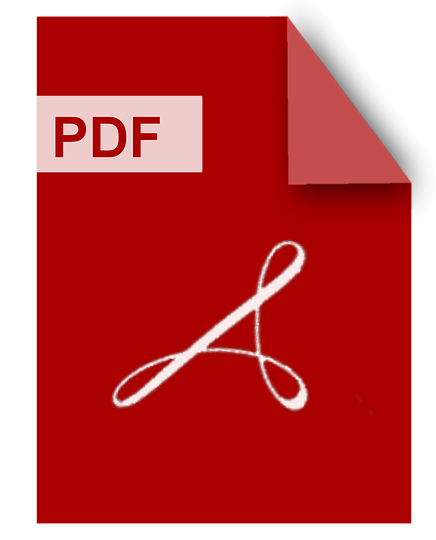
DOWNLOAD
Download Cross Validation And Regression Analysis In High Dimensional Sparse Linear Models PDF/ePub or read online books in Mobi eBooks. Click Download or Read Online button to get Cross Validation And Regression Analysis In High Dimensional Sparse Linear Models book now. This website allows unlimited access to, at the time of writing, more than 1.5 million titles, including hundreds of thousands of titles in various foreign languages. If the content not found or just blank you must refresh this page
Cross Validation And Regression Analysis In High Dimensional Sparse Linear Models
DOWNLOAD
Author : Feng Zhang
language : en
Publisher: Stanford University
Release Date : 2011
Cross Validation And Regression Analysis In High Dimensional Sparse Linear Models written by Feng Zhang and has been published by Stanford University this book supported file pdf, txt, epub, kindle and other format this book has been release on 2011 with categories.
Modern scientific research often involves experiments with at most hundreds of subjects but with tens of thousands of variables for every subject. The challenge of high dimensionality has reshaped statistical thinking and modeling. Variable selection plays a pivotal role in the high-dimensional data analysis, and the combination of sparsity and accuracy is crucial for statistical theory and practical applications. Regularization methods are attractive for tackling these sparsity and accuracy issues. The first part of this thesis studies two regularization methods. First, we consider the orthogonal greedy algorithm (OGA) used in conjunction with a high-dimensional information criterion introduced by Ing& Lai (2011). Although it has been shown to have excellent performance for weakly sparse regression models, one does not know a priori in practice that the actual model is weakly sparse, and we address this problem by developing a new cross-validation approach. OGA can be viewed as L0 regularization for weakly sparse regression models. When such sparsity fails, as revealed by the cross-validation analysis, we propose to use a new way to combine L1 and L2 penalties, which we show to have important advantages over previous regularization methods. The second part of the thesis develops a Monte Carlo Cross-Validation (MCCV) method to estimate the distribution of out-of-sample prediction errors when a training sample is used to build a regression model for prediction. Asymptotic theory and simulation studies show that the proposed MCCV method mimics the actual (but unknown) prediction error distribution even when the number of regressors exceeds the sample size. Therefore MCCV provides a useful tool for comparing the predictive performance of different regularization methods for real (rather than simulated) data sets.
Cross Validation And Regression Analysis In High Dimensional Sparse Linear Models
DOWNLOAD
Author : Feng Zhang
language : en
Publisher:
Release Date : 2011
Cross Validation And Regression Analysis In High Dimensional Sparse Linear Models written by Feng Zhang and has been published by this book supported file pdf, txt, epub, kindle and other format this book has been release on 2011 with categories.
Modern scientific research often involves experiments with at most hundreds of subjects but with tens of thousands of variables for every subject. The challenge of high dimensionality has reshaped statistical thinking and modeling. Variable selection plays a pivotal role in the high-dimensional data analysis, and the combination of sparsity and accuracy is crucial for statistical theory and practical applications. Regularization methods are attractive for tackling these sparsity and accuracy issues. The first part of this thesis studies two regularization methods. First, we consider the orthogonal greedy algorithm (OGA) used in conjunction with a high-dimensional information criterion introduced by Ing & Lai (2011). Although it has been shown to have excellent performance for weakly sparse regression models, one does not know a priori in practice that the actual model is weakly sparse, and we address this problem by developing a new cross-validation approach. OGA can be viewed as L0 regularization for weakly sparse regression models. When such sparsity fails, as revealed by the cross-validation analysis, we propose to use a new way to combine L1 and L2 penalties, which we show to have important advantages over previous regularization methods. The second part of the thesis develops a Monte Carlo Cross-Validation (MCCV) method to estimate the distribution of out-of-sample prediction errors when a training sample is used to build a regression model for prediction. Asymptotic theory and simulation studies show that the proposed MCCV method mimics the actual (but unknown) prediction error distribution even when the number of regressors exceeds the sample size. Therefore MCCV provides a useful tool for comparing the predictive performance of different regularization methods for real (rather than simulated) data sets.
Statistical Learning With Sparsity
DOWNLOAD
Author : Trevor Hastie
language : en
Publisher: CRC Press
Release Date : 2015-05-07
Statistical Learning With Sparsity written by Trevor Hastie and has been published by CRC Press this book supported file pdf, txt, epub, kindle and other format this book has been release on 2015-05-07 with Business & Economics categories.
Discover New Methods for Dealing with High-Dimensional DataA sparse statistical model has only a small number of nonzero parameters or weights; therefore, it is much easier to estimate and interpret than a dense model. Statistical Learning with Sparsity: The Lasso and Generalizations presents methods that exploit sparsity to help recover the underl
Statistical Analysis For High Dimensional Data
DOWNLOAD
Author : Arnoldo Frigessi
language : en
Publisher: Springer
Release Date : 2016-02-16
Statistical Analysis For High Dimensional Data written by Arnoldo Frigessi and has been published by Springer this book supported file pdf, txt, epub, kindle and other format this book has been release on 2016-02-16 with Mathematics categories.
This book features research contributions from The Abel Symposium on Statistical Analysis for High Dimensional Data, held in Nyvågar, Lofoten, Norway, in May 2014. The focus of the symposium was on statistical and machine learning methodologies specifically developed for inference in “big data” situations, with particular reference to genomic applications. The contributors, who are among the most prominent researchers on the theory of statistics for high dimensional inference, present new theories and methods, as well as challenging applications and computational solutions. Specific themes include, among others, variable selection and screening, penalised regression, sparsity, thresholding, low dimensional structures, computational challenges, non-convex situations, learning graphical models, sparse covariance and precision matrices, semi- and non-parametric formulations, multiple testing, classification, factor models, clustering, and preselection. Highlighting cutting-edge research and casting light on future research directions, the contributions will benefit graduate students and researchers in computational biology, statistics and the machine learning community.
Two Stepwise Regression Methods And Consistent Model Selection For Highly Correlated And High Dimensional Sparse Linear Models
DOWNLOAD
Author : Yuan-Yi Fu
language : en
Publisher:
Release Date : 2018
Two Stepwise Regression Methods And Consistent Model Selection For Highly Correlated And High Dimensional Sparse Linear Models written by Yuan-Yi Fu and has been published by this book supported file pdf, txt, epub, kindle and other format this book has been release on 2018 with categories.
Partial Least Squares Regression
DOWNLOAD
Author : R. Dennis Cook
language : en
Publisher: CRC Press
Release Date : 2024-07-17
Partial Least Squares Regression written by R. Dennis Cook and has been published by CRC Press this book supported file pdf, txt, epub, kindle and other format this book has been release on 2024-07-17 with Mathematics categories.
Partial least squares (PLS) regression is, at its historical core, a black-box algorithmic method for dimension reduction and prediction based on an underlying linear relationship between a possibly vector-valued response and a number of predictors. Through envelopes, much more has been learned about PLS regression, resulting in a mass of information that allows an envelope bridge that takes PLS regression from a black-box algorithm to a core statistical paradigm based on objective function optimization and, more generally, connects the applied sciences and statistics in the context of PLS. This book focuses on developing this bridge. It also covers uses of PLS outside of linear regression, including discriminant analysis, non-linear regression, generalized linear models and dimension reduction generally. Key Features: • Showcases the first serviceable method for studying high-dimensional regressions. • Provides necessary background on PLS and its origin. • R and Python programs are available for nearly all methods discussed in the book. This book can be used as a reference and as a course supplement at the Master's level in Statistics and beyond. It will be of interest to both statisticians and applied scientists.
Statistical Learning For Kernel Based Functional Linear Regression
DOWNLOAD
Author : Keli Guo
language : en
Publisher:
Release Date : 2022
Statistical Learning For Kernel Based Functional Linear Regression written by Keli Guo and has been published by this book supported file pdf, txt, epub, kindle and other format this book has been release on 2022 with Convergence categories.
Over the last two decades, functional linear regression that relates a scalar response on a functional predictor has been extensively studied. In practice, however, apart from functional predictors, scalar predictors or outliers are frequently included in the dataset. To address this issue, we investigate three variants of the functional linear regression model within the framework of reproducing kernel Hilbert space (RKHS), respectively. First, we consider the semi-functional linear model that consists of a functional component and anonparametric component. A double-penalized least squares method is adopted to estimate both the functional and nonparametric components within the framework of reproducing kernel Hilbert space. By virtue of the representer theorem, an efficient algorithm that requires no iterations is proposed to solve the corresponding optimization problem, where the regularization parameters are selected by the generalized cross-validation criterion. Moreover, we establish minimax rates of convergence for prediction in the semi-functional linear model. Our results reveal that the functional component can be learned with the minimax optimal rate as if the nonparametric component were known. Numerical studies and real data analysis are provided to demonstrate the effectiveness of the method and to verify the theoretical f indings. Then we consider the partially functional linear regression model (PFLM) that consists of a functional linear regression component and a sparse high-dimensional linear regression component. We adopt a double-penalized least squares approach to estimate the functional component within the framework of reproducing kernel Hilbert space and the parametric component by sorted 1 penalized estimation (SLOPE). Moreover, we establish minimax rates of convergence for prediction in the PFLM. Our results suggest that the estimator obtained by SLOPE can achive the minimax optimal rate regardless of the functional component. In contrast, the learning rate for the functional component depends on both functional and parametric components. To solve the optimization problem, an efficient computing algorithm is proposed with the help of the representer theorem. Numerical studies are conducted to demonstrate the performance of the proposed method. Finally, we propose an outlier-resistant functional linear regression model so that we can perform robust regression and outlier detection simultaneously. The proposed model includes a subject-specific mean shift parameter in the functional linear regression model to indicate whether an observation is an outlier or not. We adopt a double-penalized least squares method to estimate the functional component within the framework of reproducing kernel Hilbert space and the mean shift parameter by 1 penalization or SLOPE. By virtue of the representer theorem, an efficient algorithm is proposed to solve the corresponding optimization problem. Moreover, we establish the minimax rates of convergence for prediction and estimation in the proposed model. Our results reveal that the convergence rate for estimation of the mean shift parameter is not affected by the functional component. The functional component can be learned with the minimax optimal rate as if there were no outliers. Numerical studies are provided to demonstrate the effectiveness of the proposed methods.
Machine Learning Techniques For Gait Biometric Recognition
DOWNLOAD
Author : James Eric Mason
language : en
Publisher: Springer
Release Date : 2016-02-04
Machine Learning Techniques For Gait Biometric Recognition written by James Eric Mason and has been published by Springer this book supported file pdf, txt, epub, kindle and other format this book has been release on 2016-02-04 with Technology & Engineering categories.
This book focuses on how machine learning techniques can be used to analyze and make use of one particular category of behavioral biometrics known as the gait biometric. A comprehensive Ground Reaction Force (GRF)-based Gait Biometrics Recognition framework is proposed and validated by experiments. In addition, an in-depth analysis of existing recognition techniques that are best suited for performing footstep GRF-based person recognition is also proposed, as well as a comparison of feature extractors, normalizers, and classifiers configurations that were never directly compared with one another in any previous GRF recognition research. Finally, a detailed theoretical overview of many existing machine learning techniques is presented, leading to a proposal of two novel data processing techniques developed specifically for the purpose of gait biometric recognition using GRF. This book · introduces novel machine-learning-based temporal normalization techniques · bridges research gaps concerning the effect of footwear and stepping speed on footstep GRF-based person recognition · provides detailed discussions of key research challenges and open research issues in gait biometrics recognition · compares biometrics systems trained and tested with the same footwear against those trained and tested with different footwear
Approximate Cross Validation For Sparse Generalized Linear Models
DOWNLOAD
Author : William Thomas Stephenson
language : en
Publisher:
Release Date : 2019
Approximate Cross Validation For Sparse Generalized Linear Models written by William Thomas Stephenson and has been published by this book supported file pdf, txt, epub, kindle and other format this book has been release on 2019 with categories.
Cross validation (CV) is an effective yet computationally expensive tool for assessing the out of sample error for many methods in machine learning and statistics. Previous work has shown that methods to approximate CV can be very accurate and computationally cheap, but only for low dimensional problems. In this thesis, a modification of existing methods is developed to extend the high accuracy of these techniques to high dimensional settings.
High Dimensional Data Analysis In Cancer Research
DOWNLOAD
Author : Xiaochun Li
language : en
Publisher: Springer Science & Business Media
Release Date : 2008-12-19
High Dimensional Data Analysis In Cancer Research written by Xiaochun Li and has been published by Springer Science & Business Media this book supported file pdf, txt, epub, kindle and other format this book has been release on 2008-12-19 with Medical categories.
Multivariate analysis is a mainstay of statistical tools in the analysis of biomedical data. It concerns with associating data matrices of n rows by p columns, with rows representing samples (or patients) and columns attributes of samples, to some response variables, e.g., patients outcome. Classically, the sample size n is much larger than p, the number of variables. The properties of statistical models have been mostly discussed under the assumption of fixed p and infinite n. The advance of biological sciences and technologies has revolutionized the process of investigations of cancer. The biomedical data collection has become more automatic and more extensive. We are in the era of p as a large fraction of n, and even much larger than n. Take proteomics as an example. Although proteomic techniques have been researched and developed for many decades to identify proteins or peptides uniquely associated with a given disease state, until recently this has been mostly a laborious process, carried out one protein at a time. The advent of high throughput proteome-wide technologies such as liquid chromatography-tandem mass spectroscopy make it possible to generate proteomic signatures that facilitate rapid development of new strategies for proteomics-based detection of disease. This poses new challenges and calls for scalable solutions to the analysis of such high dimensional data. In this volume, we will present the systematic and analytical approaches and strategies from both biostatistics and bioinformatics to the analysis of correlated and high-dimensional data.