Data Assimilation With Gaussian Mixture Models Using The Dynamically Orthogonal Field Equations
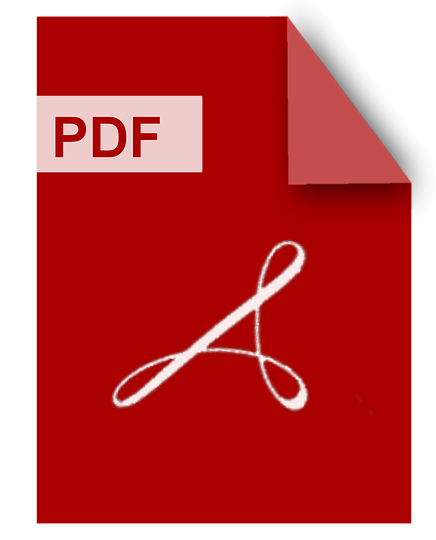
DOWNLOAD
Download Data Assimilation With Gaussian Mixture Models Using The Dynamically Orthogonal Field Equations PDF/ePub or read online books in Mobi eBooks. Click Download or Read Online button to get Data Assimilation With Gaussian Mixture Models Using The Dynamically Orthogonal Field Equations book now. This website allows unlimited access to, at the time of writing, more than 1.5 million titles, including hundreds of thousands of titles in various foreign languages. If the content not found or just blank you must refresh this page
Data Assimilation With Gaussian Mixture Models Using The Dynamically Orthogonal Field Equations
DOWNLOAD
Author : Thomas Sondergaard (S.M.)
language : en
Publisher:
Release Date : 2011
Data Assimilation With Gaussian Mixture Models Using The Dynamically Orthogonal Field Equations written by Thomas Sondergaard (S.M.) and has been published by this book supported file pdf, txt, epub, kindle and other format this book has been release on 2011 with categories.
Data assimilation, as presented in this thesis, is the statistical merging of sparse observational data with computational models so as to optimally improve the probabilistic description of the field of interest, thereby reducing uncertainties. The centerpiece of this thesis is the introduction of a novel such scheme that overcomes prior shortcomings observed within the community. Adopting techniques prevalent in Machine Learning and Pattern Recognition, and building on the foundations of classical assimilation schemes, we introduce the GMM-DO filter: Data Assimilation with Gaussian mixture models using the Dynamically Orthogonal field equations. We combine the use of Gaussian mixture models, the EM algorithm and the Bayesian Information Criterion to accurately approximate distributions based on Monte Carlo data in a framework that allows for efficient Bayesian inference. We give detailed descriptions of each of these techniques, supporting their application by recent literature. One novelty of the GMM-DO filter lies in coupling these concepts with an efficient representation of the evolving probabilistic description of the uncertain dynamical field: the Dynamically Orthogonal field equations. By limiting our attention to a dominant evolving stochastic subspace of the total state space, we bridge an important gap previously identified in the literature caused by the dimensionality of the state space. We successfully apply the GMM-DO filter to two test cases: (1) the Double Well Diffusion Experiment and (2) the Sudden Expansion fluid flow. With the former, we prove the validity of utilizing Gaussian mixture models, the EM algorithm and the Bayesian Information Criterion in a dynamical systems setting. With the application of the GMM-DO filter to the two-dimensional Sudden Expansion fluid flow, we further show its applicability to realistic test cases of non-trivial dimensionality. The GMMDO filter is shown to consistently capture and retain the far-from-Gaussian statistics that arise, both prior and posterior to the assimilation of data, resulting in its superior performance over contemporary filters. We present the GMM-DO filter as an efficient, data-driven assimilation scheme, focused on a dominant evolving stochastic subspace of the total state space, that respects nonlinear dynamics and captures non-Gaussian statistics, obviating the use of heuristic arguments.
Handbook Of Dynamic Data Driven Applications Systems
DOWNLOAD
Author : Erik P. Blasch
language : en
Publisher: Springer Nature
Release Date : 2022-05-11
Handbook Of Dynamic Data Driven Applications Systems written by Erik P. Blasch and has been published by Springer Nature this book supported file pdf, txt, epub, kindle and other format this book has been release on 2022-05-11 with Computers categories.
The Handbook of Dynamic Data Driven Applications Systems establishes an authoritative reference of DDDAS, pioneered by Dr. Darema and the co-authors for researchers and practitioners developing DDDAS technologies. Beginning with general concepts and history of the paradigm, the text provides 32 chapters by leading experts in ten application areas to enable an accurate understanding, analysis, and control of complex systems; be they natural, engineered, or societal: The authors explain how DDDAS unifies the computational and instrumentation aspects of an application system, extends the notion of Smart Computing to span from the high-end to the real-time data acquisition and control, and manages Big Data exploitation with high-dimensional model coordination. The Dynamically Data Driven Applications Systems (DDDAS) paradigm inspired research regarding the prediction of severe storms. Specifically, the DDDAS concept allows atmospheric observing systems, computer forecast models, and cyberinfrastructure to dynamically configure themselves in optimal ways in direct response to current or anticipated weather conditions. In so doing, all resources are used in an optimal manner to maximize the quality and timeliness of information they provide. Kelvin Droegemeier, Regents’ Professor of Meteorology at the University of Oklahoma; former Director of the White House Office of Science and Technology Policy We may well be entering the golden age of data science, as society in general has come to appreciate the possibilities for organizational strategies that harness massive streams of data. The challenges and opportunities are even greater when the data or the underlying system are dynamic - and DDDAS is the time-tested paradigm for realizing this potential. Sangtae Kim, Distinguished Professor of Mechanical Engineering and Distinguished Professor of Chemical Engineering at Purdue University
Handbook Of Dynamic Data Driven Applications Systems
DOWNLOAD
Author : Erik Blasch
language : en
Publisher: Springer
Release Date : 2018-11-13
Handbook Of Dynamic Data Driven Applications Systems written by Erik Blasch and has been published by Springer this book supported file pdf, txt, epub, kindle and other format this book has been release on 2018-11-13 with Computers categories.
The Handbook of Dynamic Data Driven Applications Systems establishes an authoritative reference of DDDAS, pioneered by Dr. Darema and the co-authors for researchers and practitioners developing DDDAS technologies. Beginning with general concepts and history of the paradigm, the text provides 32 chapters by leading experts in10 application areas to enable an accurate understanding, analysis, and control of complex systems; be they natural, engineered, or societal: Earth and Space Data Assimilation Aircraft Systems Processing Structures Health Monitoring Biological Data Assessment Object and Activity Tracking Embedded Control and Coordination Energy-Aware Optimization Image and Video Computing Security and Policy Coding Systems Design The authors explain how DDDAS unifies the computational and instrumentation aspects of an application system, extends the notion of Smart Computing to span from the high-end to the real-time data acquisition and control, and manages Big Data exploitation with high-dimensional model coordination.
Dynamic Data Driven Environmental Systems Science
DOWNLOAD
Author : Sai Ravela
language : en
Publisher: Springer
Release Date : 2015-11-26
Dynamic Data Driven Environmental Systems Science written by Sai Ravela and has been published by Springer this book supported file pdf, txt, epub, kindle and other format this book has been release on 2015-11-26 with Computers categories.
This book constitutes the refereed proceedings of the First International Conference on Dynamic Data-Driven Environmental Systems Science, DyDESS 2014, held in Cambridge, MA, USA, in November 2014.The 24 revised full papers and 7 short papers were carefully reviewed and selected from 62 submissions and cover topics on sensing, imaging and retrieval for the oceans, atmosphere, space, land, earth and planets that is informed by the environmental context; algorithms for modeling and simulation, downscaling, model reduction, data assimilation, uncertainty quantification and statistical learning; methodologies for planning and control, sampling and adaptive observation, and efficient coupling of these algorithms into information-gathering and observing system designs; and applications of methodology to environmental estimation, analysis and prediction including climate, natural hazards, oceans, cryosphere, atmosphere, land, space, earth and planets.
Next Generation Earth System Prediction
DOWNLOAD
Author : National Academies of Sciences, Engineering, and Medicine
language : en
Publisher: National Academies Press
Release Date : 2016-08-22
Next Generation Earth System Prediction written by National Academies of Sciences, Engineering, and Medicine and has been published by National Academies Press this book supported file pdf, txt, epub, kindle and other format this book has been release on 2016-08-22 with Science categories.
As the nation's economic activities, security concerns, and stewardship of natural resources become increasingly complex and globally interrelated, they become ever more sensitive to adverse impacts from weather, climate, and other natural phenomena. For several decades, forecasts with lead times of a few days for weather and other environmental phenomena have yielded valuable information to improve decision-making across all sectors of society. Developing the capability to forecast environmental conditions and disruptive events several weeks and months in advance could dramatically increase the value and benefit of environmental predictions, saving lives, protecting property, increasing economic vitality, protecting the environment, and informing policy choices. Over the past decade, the ability to forecast weather and climate conditions on subseasonal to seasonal (S2S) timescales, i.e., two to fifty-two weeks in advance, has improved substantially. Although significant progress has been made, much work remains to make S2S predictions skillful enough, as well as optimally tailored and communicated, to enable widespread use. Next Generation Earth System Predictions presents a ten-year U.S. research agenda that increases the nation's S2S research and modeling capability, advances S2S forecasting, and aids in decision making at medium and extended lead times.
High Order Stochastic Transport And Lagrangian Data Assimilation
DOWNLOAD
Author : Arkopal Dutt
language : en
Publisher:
Release Date : 2018
High Order Stochastic Transport And Lagrangian Data Assimilation written by Arkopal Dutt and has been published by this book supported file pdf, txt, epub, kindle and other format this book has been release on 2018 with categories.
Ocean currents transport a variety of natural (e.g. water masses, phytoplankton, zooplankton, sediments, etc.) and man-made materials (e.g. pollutants, floating debris, particulate matter, etc.). Understanding such uncertain Lagrangian transport is imperative for reducing environmental damage due to natural hazards and for allowing rigorous risk analysis and effective search and rescue. While secondary variables and trajectories have classically been used for the analyses of such transports, Lagrangian Coherent Structures (LCSs) provide a robust and objective description of the important material lines. To ensure accurate and useful Lagrangian hazard scenario predictions and prevention, the first goal of this thesis is to obtain accurate probabilistic prediction of the underlying stochastic velocity fields using the Dynamically Orthogonal (DO) approach. The second goal is to merge data from both Eulerian and Lagrangian observations with predictions such that the whole information content of observations is utilized. In the first part of this thesis, we develop high-order numerical schemes for the DO equations that ensure efficiency, accuracy, stability, and consistency between the Monte Carlo (MC) and DO solutions. We discuss the numerical challenges in applying the DO equations to the unsteady stochastic Navier-Stokes equations. In order to maintain consistent evaluation of advection terms, we utilize linear centered advection schemes with fully explicit and linear Shapiro filters. We then discuss how to combine the semi-implicit projection method with new high order implicitexplicit (IMEX) linear multi-step and multistage IMEX-RK time marching schemes for the coupled DO equations to ensure further stability and accuracy. We also review efficient numerical re-orthonormalization strategies during time marching. We showcase our results with stochastic test cases of stochastic passive tracer advection in a deterministic swirl flow, stochastic flow past a cylinder, and stochastic lid-driven cavity flow. We show that our schemes improve the consistency between reconstructed DO realizations and the corresponding MC realizations, and that we achieve the expected order of accuracy. In the second part of the work, we first undertake a study of different Lagrangian instruments and outline how the DO methodology can be applied to obtain Lagrangian variables of stochastic flow maps and LCS in uncertain flows. We then review existing methods for Bayesian Lagrangian data assimilation (DA). Disadvantages of earlier methods include the use of approximate measurement models to directly link Lagrangian variables with Eulerian variables, the challenges in respecting the Lagrangian nature of variables, and the assumptions of linearity or of Gaussian statistics during prediction or assimilation. To overcome these, we discuss how the Gaussian Mixture Model (GMM) DO Filter can be extended to fully coupled Eulerian-Lagrangian data assimilation. We define an augmented state vector of the Eulerian and Lagrangian state variables that directly exploits the full mutual information and complete the Bayesian DA in the joint Eulerian-Lagrangian stochastic subspace. Results of such coupled Eulerian-Lagrangian DA are discussed using test cases based on a double gyre flow with random frequency.
Adaptive Stochastic Reduced Order Modeling For Autonomous Ocean Platforms
DOWNLOAD
Author : Young Hyun Ryu
language : en
Publisher:
Release Date : 2022
Adaptive Stochastic Reduced Order Modeling For Autonomous Ocean Platforms written by Young Hyun Ryu and has been published by this book supported file pdf, txt, epub, kindle and other format this book has been release on 2022 with categories.
Onboard forecasting and data assimilation are challenging but essential for unmanned autonomous ocean platforms. Due to the numerous operational constraints for these platforms, efficient adaptive reduced-order models (ROMs) are needed. In this thesis, we first review existing approaches and then develop a new adaptive Dynamic Mode Decomposition (DMD)-based, data-driven, reduced-order model framework that provides onboard forecasting and data assimilation capabilities for bandwidth-disadvantaged autonomous ocean platforms. We refer to the new adaptive ROM as the incremental, stochastic Low-Rank Dynamic Mode Decomposition (iLRDMD) algorithm. Given a set of high-fidelity and high-dimensional stochastic forecasts computed in remote centers, this framework enables i) efficient and accurate send and receive of the high-fidelity forecasts, ii) incremental update of the onboard reduced-order model, iii) data-driven onboard forecasting, and iv) onboard ROM data assimilation and learning. We analyze the computational costs for the compression, communications, incremental updates, and onboard forecasts. We evaluate the adaptive ROM using a simple 2D flow behind an island, both as a test case to develop the method, and to investigate the parameter sensitivity and algorithmic design choices. We develop the extension of deterministic iLRDMD to stochastic applications with uncertain ocean forecasts. We then demonstrate the adaptive ROM on more complex ocean fields ranging from univariate 2D, univariate 3D, and multivariate 3D fields from multi-resolution, data-assimilative Multidisciplinary Simulation, Estimation, and Assimilation Systems (MSEAS) reanalyses, specifically from the real-time exercises in the Middle Atlantic Bight region. We also highlight our results using the Navy's Hybrid Coordinate Ocean Model (HYCOM) forecasts in the North Atlantic region. We t hen apply the adaptive ROM onboard forecasting algorithm to interdisciplinary applications, showcasing adaptive reduced-order forecasts for onboard underwater acoustics computations and forecasts, as well as for exact time-optimal path-planning with autonomous surface vehicles. For stochastic forecasting and data assimilation onboard the unmanned autonomous ocean platforms, we combine the stochastic ensemble DMD method with the Gaussian Mixture Model - Dynamically Orthogonal equations (GMM-DO) filter. The autonomous platforms can then perform principled Bayesian data assimilation onboard and learn from the limited and gappy ocean observation data and improve onboard estimates. We extend the DMD with the GMM-DO filter further by incorporating incremental DMD algorithms so that the stochastic ensemble DMD model itself is updated with new measurements. To address some of the inefficiencies in the first combination of the stochastic ensemble DMD with the GMM-DO filter, we further introduce the GMM-DMD algorithm. This algorithm not only uses the stochastic ensemble DMD as a computationally efficient forward model, but also employs the existing decomposition to fit the GMM to and perform Bayesian updates on. We demonstrate this incremental stochastic ensemble DMD with GMM-DO and GMM-DMD using a real at-sea application in the Middle Atlantic Bight region. We employ a 300 member set of stochastic ensemble forecasts for the Positioning System for Deep Ocean Navigation - Precision Ocean Interrogation, Navigation, and Timing (POSYDON-POINT) sea experiment, and highlight the capabilities of reduced data assimilation using simulated twin experiments.
Nonlinear Data Assimilation
DOWNLOAD
Author : Peter Jan Van Leeuwen
language : en
Publisher: Springer
Release Date : 2015-07-22
Nonlinear Data Assimilation written by Peter Jan Van Leeuwen and has been published by Springer this book supported file pdf, txt, epub, kindle and other format this book has been release on 2015-07-22 with Mathematics categories.
This book contains two review articles on nonlinear data assimilation that deal with closely related topics but were written and can be read independently. Both contributions focus on so-called particle filters. The first contribution by Jan van Leeuwen focuses on the potential of proposal densities. It discusses the issues with present-day particle filters and explorers new ideas for proposal densities to solve them, converging to particle filters that work well in systems of any dimension, closing the contribution with a high-dimensional example. The second contribution by Cheng and Reich discusses a unified framework for ensemble-transform particle filters. This allows one to bridge successful ensemble Kalman filters with fully nonlinear particle filters, and allows a proper introduction of localization in particle filters, which has been lacking up to now.
Data Assimilation For Atmospheric Oceanic And Hydrologic Applications Vol Ii
DOWNLOAD
Author : Seon Ki Park
language : en
Publisher: Springer Science & Business Media
Release Date : 2013-05-22
Data Assimilation For Atmospheric Oceanic And Hydrologic Applications Vol Ii written by Seon Ki Park and has been published by Springer Science & Business Media this book supported file pdf, txt, epub, kindle and other format this book has been release on 2013-05-22 with Science categories.
This book contains the most recent progress in data assimilation in meteorology, oceanography and hydrology including land surface. It spans both theoretical and applicative aspects with various methodologies such as variational, Kalman filter, ensemble, Monte Carlo and artificial intelligence methods. Besides data assimilation, other important topics are also covered including targeting observation, sensitivity analysis, and parameter estimation. The book will be useful to individual researchers as well as graduate students for a reference in the field of data assimilation.
Data Assimilation Methods Algorithms And Applications
DOWNLOAD
Author : Mark Asch
language : en
Publisher: SIAM
Release Date : 2016-12-29
Data Assimilation Methods Algorithms And Applications written by Mark Asch and has been published by SIAM this book supported file pdf, txt, epub, kindle and other format this book has been release on 2016-12-29 with Mathematics categories.
Data assimilation is an approach that combines observations and model output, with the objective of improving the latter. This book places data assimilation into the broader context of inverse problems and the theory, methods, and algorithms that are used for their solution. It provides a framework for, and insight into, the inverse problem nature of data assimilation, emphasizing ?why? and not just ?how.? Methods and diagnostics are emphasized, enabling readers to readily apply them to their own field of study. Readers will find a comprehensive guide that is accessible to nonexperts; numerous examples and diverse applications from a broad range of domains, including geophysics and geophysical flows, environmental acoustics, medical imaging, mechanical and biomedical engineering, economics and finance, and traffic control and urban planning; and the latest methods for advanced data assimilation, combining variational and statistical approaches.