Data Driven Methods For Improved Estimation And Control Of An Urban Arterial Traffic Network
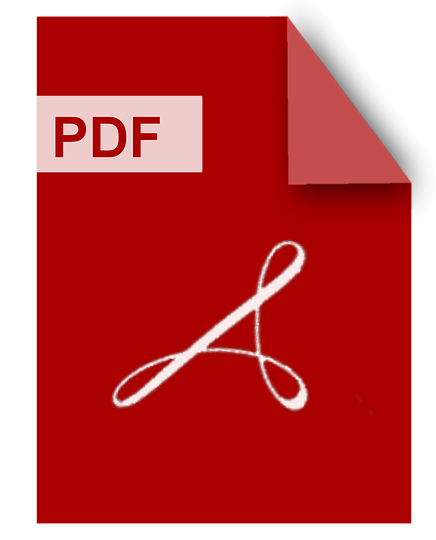
DOWNLOAD
Download Data Driven Methods For Improved Estimation And Control Of An Urban Arterial Traffic Network PDF/ePub or read online books in Mobi eBooks. Click Download or Read Online button to get Data Driven Methods For Improved Estimation And Control Of An Urban Arterial Traffic Network book now. This website allows unlimited access to, at the time of writing, more than 1.5 million titles, including hundreds of thousands of titles in various foreign languages. If the content not found or just blank you must refresh this page
Data Driven Methods For Improved Estimation And Control Of An Urban Arterial Traffic Network
DOWNLOAD
Author : Leah Adrian Anderson
language : en
Publisher:
Release Date : 2015
Data Driven Methods For Improved Estimation And Control Of An Urban Arterial Traffic Network written by Leah Adrian Anderson and has been published by this book supported file pdf, txt, epub, kindle and other format this book has been release on 2015 with categories.
Transportation is a field which is universal in our society: people from every country, culture or background are familiar with the challenges of getting around in our built environment. Yet what is not always so obvious to the average traveler is how the techniques and tools of designing, observing, and controlling our modern transportation networks are derived. In fact, the theory of traffic engineering has many gaps and unknowns that are the topic of ongoing research efforts in the academic community. This work presents a collection of theoretical and practical methodologies to advance the study of traffic flow modeling, state estimation, and control of signalized roadways in particular. It uses theory from traditional transportation engineering, but also demonstrates the application of new tools from control theory and computer science to the specific application of signalized traffic networks. First, two numerical modeling dynamics representing traffic flows on signalized arterials are presented: the well-known Cell Transmission Model, a discretization of the physical hydrodynamic laws believed to govern vehicle flows, and a new Vertical Cell Model which resembles classical "store-and-forward" models with the addition of transit delays and finite buffer capacities. Each of these models is implemented in a common software framework, which provides an ideal experimental platform for direct comparison of the competing dynamics. A chapter in this dissertation contributes a validation and comparison of the two models against real vehicle trajectory data on an existing signalized road network. Accuracy and confidence in such traffic models requires complimentary methods of observing true traffic conditions to provide initial conditions and real-time state estimates. Yet there are many technological deficiencies in existing urban roadway detection systems that prevent the acquisition of a real-time estimate of arterial link state (or queue length) at signalized intersections. Hence this thesis also contains methodology to improve the estimates obtained from existing hardware by combining data from typical infrastructure sensors with new sources of Lagrangian probe measurements. These are then assimilated into a detailed model of flow dynamics. This technique was previously proposed for continuous-flow (freeway) networks, but required novel adaptions to be applied to an interrupted-flow setting. This dissertation next explores advancements in theoretically optimal control algorithms for statistically-modeled signalized queueing networks. In the context of a large body of previous work on flow-impeding control for vertical queueing networks, the practical challenges of traffic signal control are highlighted. Some of these challenges are tackled in the specific case of the max pressure controller, an algorithm derived from the field of communications networks that has been shown to optimize through-flow in an idealized network model. The lack of adequate measurements or demand-volume data has historically been a major limitation in advancing research on signalized arterial road networks. Yet the current revolution of inexpensive storage and processing of "big data" shows promise for improving daily operations of existing roadways without the need for expensive new hardware systems. One example of this potential appears is the case of traffic signal control. Existing traffic signals are capable of operating more efficiently by changing signal plans based on real-time demand measurements through a traffic responsive plan selection (TRPS) mode of operation (rather than depending on a rigid schedule for plan changes). However, this mode is rarely used in practice because its calibration process is not accessible or intuitive to traffic technicians. This dissertation presents an application of statistical learning techniques to improve the process of calibrating and implementing an existing TRPS mechanism. A proof-of-concept implementation using historical sensor data from a busy urban intersection demonstrates that real operational improvements may be immediately achievable using existing sensing infrastructure.
Mobility Data Driven Urban Traffic Monitoring
DOWNLOAD
Author : Zhidan Liu
language : en
Publisher: Springer Nature
Release Date : 2021-05-18
Mobility Data Driven Urban Traffic Monitoring written by Zhidan Liu and has been published by Springer Nature this book supported file pdf, txt, epub, kindle and other format this book has been release on 2021-05-18 with Computers categories.
This book introduces the concepts of mobility data and data-driven urban traffic monitoring. A typical framework of mobility data-based urban traffic monitoring is also presented, and it describes the processes of mobility data collection, data processing, traffic modelling, and some practical issues of applying the models for urban traffic monitoring. This book presents three novel mobility data-driven urban traffic monitoring approaches. First, to attack the challenge of mobility data sparsity, the authors propose a compressive sensing-based urban traffic monitoring approach. This solution mines the traffic correlation at the road network scale and exploits the compressive sensing theory to recover traffic conditions of the whole road network from sparse traffic samplings. Second, the authors have compared the traffic estimation performances between linear and nonlinear traffic correlation models and proposed a dynamical non-linear traffic correlation modelling-based urban traffic monitoring approach. To address the challenge of involved huge computation overheads, the approach adapts the traffic modelling and estimations tasks to Apache Spark, a popular parallel computing framework. Third, in addition to mobility data collected by the public transit systems, the authors present a crowdsensing-based urban traffic monitoring approach. The proposal exploits the lightweight mobility data collected from participatory bus riders to recover traffic statuses through careful data processing and analysis. Last but not the least, the book points out some future research directions, which can further improve the accuracy and efficiency of mobility data-driven urban traffic monitoring at large scale. This book targets researchers, computer scientists, and engineers, who are interested in the research areas of intelligent transportation systems (ITS), urban computing, big data analytic, and Internet of Things (IoT). Advanced level students studying these topics benefit from this book as well.
Road Traffic Modeling And Management
DOWNLOAD
Author : Fouzi Harrou
language : en
Publisher: Elsevier
Release Date : 2021-10-05
Road Traffic Modeling And Management written by Fouzi Harrou and has been published by Elsevier this book supported file pdf, txt, epub, kindle and other format this book has been release on 2021-10-05 with Transportation categories.
Road Traffic Modeling and Management: Using Statistical Monitoring and Deep Learning provides a framework for understanding and enhancing road traffic monitoring and management. The book examines commonly used traffic analysis methodologies as well the emerging methods that use deep learning methods. Other sections discuss how to understand statistical models and machine learning algorithms and how to apply them to traffic modeling, estimation, forecasting and traffic congestion monitoring. Providing both a theoretical framework along with practical technical solutions, this book is ideal for researchers and practitioners who want to improve the performance of intelligent transportation systems. Provides integrated, up-to-date and complete coverage of the key components for intelligent transportation systems: traffic modeling, forecasting, estimation and monitoring Uses methods based on video and time series data for traffic modeling and forecasting Includes case studies, key processes guidance and comparisons of different methodologies
A Deep Learning Approach For Spatiotemporal Data Driven Traffic State Estimation
DOWNLOAD
Author : Amr Abdelraouf
language : en
Publisher:
Release Date : 2022
A Deep Learning Approach For Spatiotemporal Data Driven Traffic State Estimation written by Amr Abdelraouf and has been published by this book supported file pdf, txt, epub, kindle and other format this book has been release on 2022 with categories.
The past decade witnessed rapid developments in traffic data sensing technologies in the form of roadside detector hardware, vehicle on-board units, and pedestrian wearable devices. The growing magnitude and complexity of the available traffic data has fueled the demand for data-driven models that can handle large scale inputs. In the recent past, deep-learning-powered algorithms have become the state-of-the-art for various data-driven applications. In this research, three applications of deep learning algorithms for traffic state estimation were investigated. Firstly, network-wide traffic parameters estimation was explored. An attention-based multi-encoder-decoder (Att-MED) neural network architecture was proposed and trained to predict freeway traffic speed up to 60 minutes ahead. Att-MED was designed to encode multiple traffic input sequences: short-term, daily, and weekly cyclic behavior. The proposed network produced an average prediction accuracy of 97.5%, which was superior to the compared baseline models. In addition to improving the output performance, the model’s attention weights enhanced the model interpretability. This research additionally explored the utility of low-penetration connected probe-vehicle data for network-wide traffic parameters estimation and prediction on freeways. A novel sequence-to-sequence recurrent graph networks (Seq2Se2 GCN-LSTM) was designed. It was then trained to estimate and predict traffic volume and speed for a 60-minute future time horizon. The proposed methodology generated volume and speed predictions with an average accuracy of 90.5% and 96.6%, respectively, outperforming the investigated baseline models. The proposed method demonstrated robustness against perturbations caused by the probe vehicle fleet’s low penetration rate. Secondly, the application of deep learning for road weather detection using roadside CCTVs were investigated. A Vision Transformer (ViT) was trained for simultaneous rain and road surface condition classification. Next, a Spatial Self-Attention (SSA) network was designed to consume the individual detection results, interpret the spatial context, and modify the collective detection output accordingly. The sequential module improved the accuracy of the stand-alone Vision Transformer as measured by the F1-score, raising the total accuracy for both tasks to 96.71% and 98.07%, respectively. Thirdly, a real-time video-based traffic incident detection algorithm was developed to enhance the utilization of the existing roadside CCTV network. The methodology automatically identified the main road regions in video scenes and investigated static vehicles around those areas. The developed algorithm was evaluated using a dataset of roadside videos. The incidents were detected with 85.71% sensitivity and 11.10% false alarm rate with an average delay of 27.53 seconds. In general, the research proposed in this dissertation maximizes the utility of pre-existing traffic infrastructure and emerging probe traffic data. It additionally demonstrated deep learning algorithms’ capability of modeling complex spatiotemporal traffic data. This research illustrates that advances in the deep learning field continue to have a high applicability potential in the traffic state estimation domain.
Data Driven Approaches For Robust Signal Plans In Urban Transportation Networks
DOWNLOAD
Author : Zahra Amini
language : en
Publisher:
Release Date : 2018
Data Driven Approaches For Robust Signal Plans In Urban Transportation Networks written by Zahra Amini and has been published by this book supported file pdf, txt, epub, kindle and other format this book has been release on 2018 with categories.
In urban transportation networks with signalized intersections a robust pre-timed signal plan is a practical alternative to adaptive control strategies, since it has less complexity and an easier implementation process. Recent advances in technology are making data collection at traffic signals economical and data-driven approaches are likely to benefit from the large traffic data. Data-driven approaches are necessary for designing robust timing plans that can satisfy rapid traffic volume fluctuation and demand growth. This dissertation introduces four data-driven approaches for studying and improving traffic conditions at signalized intersections. Firstly, I discuss the development and testing of two algorithms for checking the quality of traffic data and for estimating performance measures at intersections. The first of these algorithms estimates the systematic error of the detector data at signalized intersections by using flow conservation. According to the ground truth data from a real-world network, the algorithm can reduce the error in the data up to 25%. The second algorithm helps in estimating intersection performance measures in real-time by measuring the number of the vehicles in each approach using high resolution(HR) data. An offset optimization algorithm was developed to adjust signal offsets so as to improve the delay in the system. The performance of three real-world networks using the offsets obtained by the algorithm and those obtained from the widely used Synchro optimization tool, are compared using the VISSIM microscopic simulation model. Simulation results show up to a 30% reduction in the average number of stops and total delay that vehicles experience along the major routes when using the proposed algorithms’ optimized offsets. The fourth algorithm estimates the appropriate switching time between designed timing plans during the day based on the traffic profile of the intersection by using the K-means clustering method. In conclusion, these four algorithms extract useful information from HR data about traffic at signalized intersections. Moreover, the algorithms assist in designing robust timing plans for satisfying demand fluctuations at signalized intersection. Lastly, simulation results from real-world networks illustrate the significant improvements that the proposed data-driven approaches can make in the control systems at urban transportation networks.
Real Time Estimation Of Arterial Performance Measures Using A Data Driven Microscopic Traffic Simulation Technique
DOWNLOAD
Author : Dwayne Anthony Henclewood
language : en
Publisher:
Release Date : 2012
Real Time Estimation Of Arterial Performance Measures Using A Data Driven Microscopic Traffic Simulation Technique written by Dwayne Anthony Henclewood and has been published by this book supported file pdf, txt, epub, kindle and other format this book has been release on 2012 with Traffic congestion categories.
Traffic congestion is a one hundred billion dollar problem in the US. The cost of congestion has been trending upward over the last few decades, but has experienced slight decreases in recent years partly due to the impact of congestion reduction strategies. The impact of these strategies is however largely experienced on freeways and not arterials. This discrepancy in impact is partially linked to the lack of real-time, arterial traffic information. Toward this end, this research effort seeks to address the lack of arterial traffic information. :To address this dearth of information, this effort developed a methodology to provide accurate estimates of arterial performance measures to transportation facility managers and travelers in real-time. This methodology employs transmitted point sensor data to drive an online, microscopic traffic simulation model. The feasibility of this methodology was examined through a series of experiments that were built upon the successes of the previous, while addressing the necessary limitations. The results from each experiment were encouraging. They successfully demonstrated the method's likely feasibility, and the accuracy with which field estimates of performance measures may be obtained. In addition, the method's results support the viability of a "real-world" implementation of the method. An advanced calibration process was also developed as a means of improving the method's accuracy. This process will in turn serve to inform future calibration efforts as the need for more robust and accurate traffic simulation models are needed. :The success of this method provides a template for real-time traffic simulation modeling which is capable of adequately addressing the lack of available arterial traffic information. In providing such information, it is hoped that transportation facility managers and travelers will make more informed decisions regarding more efficient management and usage of the nation's transportation network.
Data Driven Traffic Engineering
DOWNLOAD
Author : Hubert Rehborn
language : en
Publisher: Elsevier
Release Date : 2020-11-08
Data Driven Traffic Engineering written by Hubert Rehborn and has been published by Elsevier this book supported file pdf, txt, epub, kindle and other format this book has been release on 2020-11-08 with Transportation categories.
Data-Driven Traffic Engineering: Understanding of Traffic and Applications Based on Three-Phase Traffic Theory shifts the current focus from using modeling and simulation data for traffic measurements to the use of actual data. The book uses real-world, empirically-derived data from a large fleet of connected vehicles, local observations and aerial observation to shed light on key traffic phenomena. Readers will learn how to develop an understanding of the empirical features of vehicular traffic networks and how to consider these features in emerging, intelligent transport systems. Topics cover congestion patterns, fuel consumption, the influence of weather, and much more. This book offers a unique, data-driven analysis of vehicular traffic in traffic networks, also considering how to apply data-driven insights to the intelligent transport systems of the future. Provides an empirically-driven analysis of traffic measurements/congestion based on real-world data collected from a global fleet of vehicles Applies Kerner's three-phase traffic theory to empirical data Offers a critical scientific understanding of the underlying concerns of traffic control in automated driving and intelligent transport systems
Automated Vehicle Delay And Travel Time Estimation Techniques For Improved Performance Measures Of Urban Network System
DOWNLOAD
Author : Ibrahem Mahmoud Shatnawi
language : en
Publisher:
Release Date : 2015
Automated Vehicle Delay And Travel Time Estimation Techniques For Improved Performance Measures Of Urban Network System written by Ibrahem Mahmoud Shatnawi and has been published by this book supported file pdf, txt, epub, kindle and other format this book has been release on 2015 with Roads categories.
Vehicle delay and travel time are considered the most important measures of effectiveness (MOE) in urban arterial roads. They allow traffic engineers to evaluate the performance of a traffic system component or the effectiveness of the system wide control strategy. They are often utilized for real-time applications such as adaptive signal control, congestion management, and dynamic traffic assignment. However, obtaining intersection performance data in real-time, including average control delay and travel time, can be very time consuming and labor intensive. Three real-time logics: AVDET, AVTTET, and ANDET are proposed for estimating performance measures of isolated intersections, traffic corridors, and traffic networks. These approaches use the existing traffic detection system to estimate vehicle delay and travel time. By using a real-time traffic detection system as input, the algorithms used in real-time logics make use of information from both the detectors and the signal system of the intersection. By tracking each detector's status and traffic signal operation second by second, travel time, approach, and intersection delays can be estimated automatically. Results from the proposed algorithms are compared with those from simulation output, and statistical tests are conducted under varying traffic operation conditions. Also, the results are analyzed using different statistical techniques. The findings show that the proposed algorithms are able to yield reliable results in different traffic and signal control conditions and that the output is very stable. Future works are suggested to further examine and improve the performance of the proposed algorithms.
Annual Report
DOWNLOAD
Author : University of Minnesota. Intelligent Transportation Systems Institute
language : en
Publisher:
Release Date : 2005
Annual Report written by University of Minnesota. Intelligent Transportation Systems Institute and has been published by this book supported file pdf, txt, epub, kindle and other format this book has been release on 2005 with Intelligent Vehicle Highway Systems categories.
Data Driven Adaptive Traffic Signal Control Via Deep Reinforcement Learning
DOWNLOAD
Author : Tian Tan
language : en
Publisher:
Release Date : 2020
Data Driven Adaptive Traffic Signal Control Via Deep Reinforcement Learning written by Tian Tan and has been published by this book supported file pdf, txt, epub, kindle and other format this book has been release on 2020 with categories.
Adaptive traffic signal control (ATSC) system serves a significant role for relieving urban traffic congestion. The system is capable of adjusting signal phases and timings of all traffic lights simultaneously according to real-time traffic sensor data, resulting in a better overall traffic management and an improved traffic condition on road. In recent years, deep reinforcement learning (DRL), one powerful paradigm in artificial intelligence (AI) for sequential decision-making, has drawn great attention from transportation researchers. The following three properties of DRL make it very attractive and ideal for the next generation ATSC system: (1) model-free: DRL reasons about the optimal control strategies directly from data without making additional assumptions on the underlying traffic distributions and traffic flows. Compared with traditional traffic optimization methods, DRL avoids the cumbersome formulation of traffic dynamics and modeling; (2) self-learning: DRL self-learns the signal control knowledge from traffic data with minimal human expertise; (3) simple data requirement: by using large nonlinear neural networks as function approximators, DRL has enough representation power to map directly from simple traffic measurements, e.g. queue length and waiting time, to signal control policies. This thesis focuses on building data-driven and adaptive controllers via deep reinforcement learning for large-scale traffic signal control systems. In particular, the thesis first proposes a hierarchical decentralized-to-centralized DRL framework for large-scale ATSC to better coordinate multiple signalized intersections in the traffic system. Second, the thesis introduces efficient DRL with efficient exploration for ATSC to greatly improve sample complexity of DRL algorithms, making them more suitable for real-world control systems. Furthermore, the thesis combines multi-agent system with efficient DRL to solve large-scale ATSC problems that have multiple intersections. Finally, the thesis presents several algorithmic extensions to handle complex topology and heterogeneous intersections in real-world traffic networks. To gauge the performance of the presented DRL algorithms, various experiments have been conducted and included in the thesis both on small-scale and on large-scale simulated traffic networks. The empirical results have demonstrated that the proposed DRL algorithms outperform both rule-based control policy and commonly-used off-the-shelf DRL algorithms by a significant margin. Moreover, the proposed efficient MARL algorithms have achieved the state-of-the-art performance with improved sample-complexity for large-scale ATSC.