Data Driven Real Time Traffic Signal Optimization
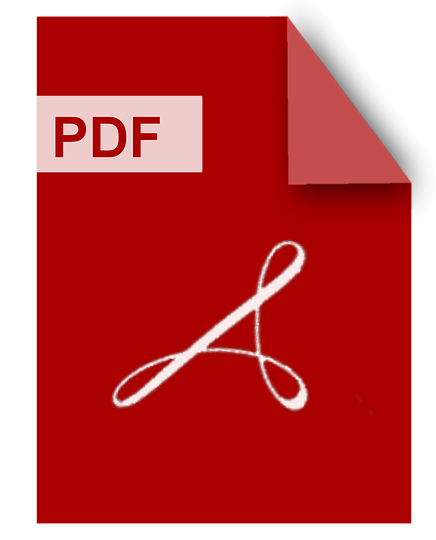
DOWNLOAD
Download Data Driven Real Time Traffic Signal Optimization PDF/ePub or read online books in Mobi eBooks. Click Download or Read Online button to get Data Driven Real Time Traffic Signal Optimization book now. This website allows unlimited access to, at the time of writing, more than 1.5 million titles, including hundreds of thousands of titles in various foreign languages. If the content not found or just blank you must refresh this page
Data Driven Real Time Traffic Signal Optimization
DOWNLOAD
Author : Stephen David Boyles
language : en
Publisher:
Release Date : 2020
Data Driven Real Time Traffic Signal Optimization written by Stephen David Boyles and has been published by this book supported file pdf, txt, epub, kindle and other format this book has been release on 2020 with categories.
Data Driven Adaptive Traffic Signal Control Via Deep Reinforcement Learning
DOWNLOAD
Author : Tian Tan
language : en
Publisher:
Release Date : 2020
Data Driven Adaptive Traffic Signal Control Via Deep Reinforcement Learning written by Tian Tan and has been published by this book supported file pdf, txt, epub, kindle and other format this book has been release on 2020 with categories.
Adaptive traffic signal control (ATSC) system serves a significant role for relieving urban traffic congestion. The system is capable of adjusting signal phases and timings of all traffic lights simultaneously according to real-time traffic sensor data, resulting in a better overall traffic management and an improved traffic condition on road. In recent years, deep reinforcement learning (DRL), one powerful paradigm in artificial intelligence (AI) for sequential decision-making, has drawn great attention from transportation researchers. The following three properties of DRL make it very attractive and ideal for the next generation ATSC system: (1) model-free: DRL reasons about the optimal control strategies directly from data without making additional assumptions on the underlying traffic distributions and traffic flows. Compared with traditional traffic optimization methods, DRL avoids the cumbersome formulation of traffic dynamics and modeling; (2) self-learning: DRL self-learns the signal control knowledge from traffic data with minimal human expertise; (3) simple data requirement: by using large nonlinear neural networks as function approximators, DRL has enough representation power to map directly from simple traffic measurements, e.g. queue length and waiting time, to signal control policies. This thesis focuses on building data-driven and adaptive controllers via deep reinforcement learning for large-scale traffic signal control systems. In particular, the thesis first proposes a hierarchical decentralized-to-centralized DRL framework for large-scale ATSC to better coordinate multiple signalized intersections in the traffic system. Second, the thesis introduces efficient DRL with efficient exploration for ATSC to greatly improve sample complexity of DRL algorithms, making them more suitable for real-world control systems. Furthermore, the thesis combines multi-agent system with efficient DRL to solve large-scale ATSC problems that have multiple intersections. Finally, the thesis presents several algorithmic extensions to handle complex topology and heterogeneous intersections in real-world traffic networks. To gauge the performance of the presented DRL algorithms, various experiments have been conducted and included in the thesis both on small-scale and on large-scale simulated traffic networks. The empirical results have demonstrated that the proposed DRL algorithms outperform both rule-based control policy and commonly-used off-the-shelf DRL algorithms by a significant margin. Moreover, the proposed efficient MARL algorithms have achieved the state-of-the-art performance with improved sample-complexity for large-scale ATSC.
Traffic Information And Control
DOWNLOAD
Author : Ruimin Li
language : en
Publisher: Institution of Engineering and Technology
Release Date : 2020-11-16
Traffic Information And Control written by Ruimin Li and has been published by Institution of Engineering and Technology this book supported file pdf, txt, epub, kindle and other format this book has been release on 2020-11-16 with Technology & Engineering categories.
Written by an international team of researchers, this book focuses on traffic information processing and signal control using emerging types of traffic data. It conveys advanced methods to estimate and predict traffic flows at different levels, including macroscopic, mesoscopic and microscopic. The aim of these predictions is to optimize traffic signal control for intersections and to mitigate ever-growing traffic congestion.
Application Of The Stochastic Optimization Method In Optimizing Traffic Signal Control Settings
DOWNLOAD
Author : Byungkyu Park
language : en
Publisher:
Release Date : 2008
Application Of The Stochastic Optimization Method In Optimizing Traffic Signal Control Settings written by Byungkyu Park and has been published by this book supported file pdf, txt, epub, kindle and other format this book has been release on 2008 with Stochastic programming categories.
Traffic congestion has greatly affected not only the nation's economy and environment but also every citizen's quality of life. A recent study shows that every American traveler spent an extra 38 hours and 26 gallons of fuel per year due to traffic congestion during the peak period. Of this congestion, 10% is attributable to improper operations of traffic signals. Surprisingly, more than a half of all signalized intersections in the United States needs to be re-optimized immediately to maintain peak efficiency. Even though many traffic signal control systems have been upgraded from pre-timed controllers to actuated and adaptive controllers, the traffic signal optimization software has not been kept current. For example, existing commercial traffic signal timing optimization programs including SYNCHRO and TRANSYT-7F do not optimize advanced controller settings available in the modern traffic controllers including minimum green time, extension time, and detector settings. This is in part because existing programs are based on macroscopic simulation tools that do not explicitly consider individual vehicular movements. To overcome such a shortcoming, a stochastic optimization method (SOM) was proposed and successfully applied to a signalized corridor in Northern Virginia. This study presents enhancements made in the SOM and case study results from an arterial network consisting of 16 signalized intersections. The proposed method employs a distributed computing environment (DCE) for faster computation time and uses a shuffled frog-leaping algorithm (SFLA) for better optimization. The case study results showed that the proposed enhanced SOM method, called SFLASOM, improved the total network travel times over field settings by 3.5% for Mid-Day and 2.1% for PM-Peak. In addition, corridor travel times were improved by 2.3% to 17.9% over field settings. However, when the new SOM timing plan was compared to the new field timing plan implemented in July 2008, the improvements were marginal, showing slightly over 2% reductions in individual vehicular delay.
Data Driven Approaches For Robust Signal Plans In Urban Transportation Networks
DOWNLOAD
Author : Zahra Amini
language : en
Publisher:
Release Date : 2018
Data Driven Approaches For Robust Signal Plans In Urban Transportation Networks written by Zahra Amini and has been published by this book supported file pdf, txt, epub, kindle and other format this book has been release on 2018 with categories.
In urban transportation networks with signalized intersections a robust pre-timed signal plan is a practical alternative to adaptive control strategies, since it has less complexity and an easier implementation process. Recent advances in technology are making data collection at traffic signals economical and data-driven approaches are likely to benefit from the large traffic data. Data-driven approaches are necessary for designing robust timing plans that can satisfy rapid traffic volume fluctuation and demand growth. This dissertation introduces four data-driven approaches for studying and improving traffic conditions at signalized intersections. Firstly, I discuss the development and testing of two algorithms for checking the quality of traffic data and for estimating performance measures at intersections. The first of these algorithms estimates the systematic error of the detector data at signalized intersections by using flow conservation. According to the ground truth data from a real-world network, the algorithm can reduce the error in the data up to 25%. The second algorithm helps in estimating intersection performance measures in real-time by measuring the number of the vehicles in each approach using high resolution(HR) data. An offset optimization algorithm was developed to adjust signal offsets so as to improve the delay in the system. The performance of three real-world networks using the offsets obtained by the algorithm and those obtained from the widely used Synchro optimization tool, are compared using the VISSIM microscopic simulation model. Simulation results show up to a 30% reduction in the average number of stops and total delay that vehicles experience along the major routes when using the proposed algorithms’ optimized offsets. The fourth algorithm estimates the appropriate switching time between designed timing plans during the day based on the traffic profile of the intersection by using the K-means clustering method. In conclusion, these four algorithms extract useful information from HR data about traffic at signalized intersections. Moreover, the algorithms assist in designing robust timing plans for satisfying demand fluctuations at signalized intersection. Lastly, simulation results from real-world networks illustrate the significant improvements that the proposed data-driven approaches can make in the control systems at urban transportation networks.
Improving Performance Of Coordinated Signal Control Systems Using Signal And Loop Data
DOWNLOAD
Author : Meng Li (Writer on traffic signs and signals)
language : en
Publisher:
Release Date : 2010
Improving Performance Of Coordinated Signal Control Systems Using Signal And Loop Data written by Meng Li (Writer on traffic signs and signals) and has been published by this book supported file pdf, txt, epub, kindle and other format this book has been release on 2010 with Automatic data collection systems categories.
Data Driven Solutions To Transportation Problems
DOWNLOAD
Author : Yinhai Wang
language : en
Publisher: Elsevier
Release Date : 2018-12-04
Data Driven Solutions To Transportation Problems written by Yinhai Wang and has been published by Elsevier this book supported file pdf, txt, epub, kindle and other format this book has been release on 2018-12-04 with Transportation categories.
Data-Driven Solutions to Transportation Problems explores the fundamental principle of analyzing different types of transportation-related data using methodologies such as the data fusion model, the big data mining approach, computer vision-enabled traffic sensing data analysis, and machine learning. The book examines the state-of-the-art in data-enabled methodologies, technologies and applications in transportation. Readers will learn how to solve problems relating to energy efficiency under connected vehicle environments, urban travel behavior, trajectory data-based travel pattern identification, public transportation analysis, traffic signal control efficiency, optimizing traffic networks network, and much more. Synthesizes the newest developments in data-driven transportation science Includes case studies and examples in each chapter that illustrate the application of methodologies and technologies employed Useful for both theoretical and technically-oriented researchers
Traffic Signal Optimization Programs
DOWNLOAD
Author : Chris Claterbos
language : en
Publisher:
Release Date : 1984
Traffic Signal Optimization Programs written by Chris Claterbos and has been published by this book supported file pdf, txt, epub, kindle and other format this book has been release on 1984 with Electronic traffic controls categories.
Test of MAXBAND, SIGOP-III, and TRANSYT-7F on arterials in Lawrence, Kansas, Overland Park, Kansas, and Davenport, Iowa.
Investigation Of Schedules For Traffic Signal Timing Optimization
DOWNLOAD
Author : Byungkyu Park
language : en
Publisher:
Release Date : 2005
Investigation Of Schedules For Traffic Signal Timing Optimization written by Byungkyu Park and has been published by this book supported file pdf, txt, epub, kindle and other format this book has been release on 2005 with Electronic traffic controls categories.
Traffic signal optimization is recognized as one of the most cost-effective ways to improve urban mobility; however the extent of the benefits realized could significantly depend on how often traffic signal re-optimization occurs. Using a case study from the Northern Virginia Smart Traffic Signal System (NVSTSS), this project sought to determine how often traffic signals need to be re-optimized to provide the greatest benefits. This project developed a new traffic signal timing plan evaluation and optimization program by combining the Integrated SYNCHRO and Platoon Dispersion (ISAPD) model and the OptQuest optimization program. Based on 2001 (base scenario) and 2004 traffic data, five scenarios of re-optimization time intervals (i.e., 2 weeks, 4 weeks, 8 weeks, 16 weeks, and 1 year) were investigated. Study results indicate that (1) determining time intervals for re-optimization in the NVSTSS is feasible; (2) among the various re-optimization time intervals investigated for the Route 50 case study network, the time interval of 1 year was the best for both midday and the PM peak; and (3) the annual net savings from implementing this 1-year re-optimization time interval could be as high as $107,340 and $254,436, respectively, given the assumptions used in the study. The report recommends (1) the annual re-optimization of the Route 50 corridor traffic signal system; (2) the NVSTSS implementation of the combined ISAPS and OptQuest program for measuring "regrets" of not maintaining the optimal timing plan; (3) the adoption by VDOT traffic engineers of the methodology developed in this study, which is based on the combined ISAPD and OptQuest program, for making decisions regarding traffic signal re-optimization; and (4) a future study to investigate the impact of traffic volume growth rates and changes in turning movements as a means of assisting with determinations about traffic signal timing plan re-optimization.
Traffic Signal Timing Optimization With Connected Vehicles
DOWNLOAD
Author : Wan Li
language : en
Publisher:
Release Date : 2019
Traffic Signal Timing Optimization With Connected Vehicles written by Wan Li and has been published by this book supported file pdf, txt, epub, kindle and other format this book has been release on 2019 with Intelligent transportation systems categories.
The advent and deployment of Connected vehicle (CV) and Vehicle-to-everything (V2X) communications offer the potential to significantly improve the efficiency of traffic signal control systems. The knowledge of vehicle trajectories in the network allows for optimal signal setting and significant improvements in network performance compared to existing traffic signal control systems. This research aims to develop a framework, including modeling techniques, algorithms, and testing strategies, for urban traffic signal optimization with CVs. The objective is to improve the safety, mobility, and sustainability of all vehicles in the study areas utilizing CV data, i.e., real time information on vehicles' locations and speeds, as well as communications to the signal control systems. The proposed framework is able to optimize traffic signal timing for a single intersection and along a corridor under various market penetration of CVs. Under full penetration rate of CVs, the signal timing optimization and coordination problems are first formulated in a centralized scheme as a mixed-integer nonlinear programing (MINLP). Due to the complexity of the model, the problem is decomposed into two levels: an intersection level to optimize phase durations using dynamic programing (DP) and a corridor level to optimize the offsets of all intersections. Under medium-to-high penetration rates of CVs, Kalman filter methods are applied to estimate trajectories of unequipped vehicles given the available trajectories of CVs. The estimated trajectories combined with CV trajectories are utilized in the trajectory-based signal timing optimization process. Under relatively low penetration rates of CVs, a Deep Intersection Spatial Temporal Network (DISTN) is developed to predict short-term movement-based traffic volumes. The predicted volumes are used in a volume-based adaptive signal control method to calculate signal timing parameters. Comprehensive testing and validation of the proposed methods are conducted in traffic simulation and with real world CV (probe vehicle) data. The testing tasks aim to validate that the developed methods are computationally manageable and have the potential to be implemented in CV-based traffic signal applications in the real world.