Deep Learning Based Ct Image Reconstruction
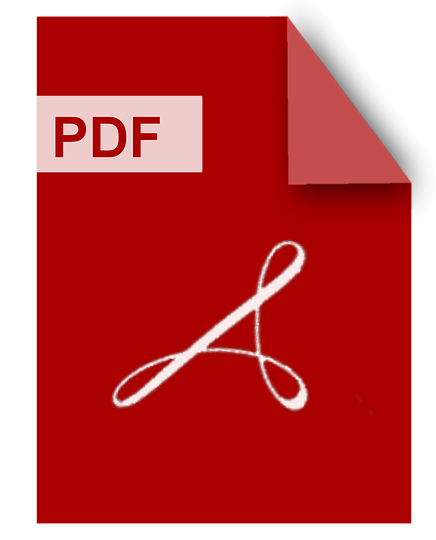
DOWNLOAD
Download Deep Learning Based Ct Image Reconstruction PDF/ePub or read online books in Mobi eBooks. Click Download or Read Online button to get Deep Learning Based Ct Image Reconstruction book now. This website allows unlimited access to, at the time of writing, more than 1.5 million titles, including hundreds of thousands of titles in various foreign languages. If the content not found or just blank you must refresh this page
Deep Learning Based Ct Image Reconstruction
DOWNLOAD
Author : Ruiwen Xing
language : en
Publisher:
Release Date : 2020
Deep Learning Based Ct Image Reconstruction written by Ruiwen Xing and has been published by this book supported file pdf, txt, epub, kindle and other format this book has been release on 2020 with Image reconstruction categories.
As a common medical imaging method, Computed Tomography (CT) can create tomographic images using X-ray data acquired from around the human body. However, high quality and adequately sampled X-ray measurement data are not always available. In this scenario, the tomographic image created by conventional reconstruction algorithms will be noisy, or contain artifacts. The goal of our study is to reconstruct high-quality tomographic images from noisy or incomplete scan data, including low-dose, sparse-view, and limited-angle scenarios, by utilizing novel deep learning techniques. In this project, we trained a Generative Adversarial Network (GAN) and used it as a signal prior in the Simultaneous Algebraic Reconstruction Technique (SART) iterative reconstruction algorithm. The GAN we trained includes a self-attention block to model long-range dependencies in the scan data. Compared with the state-of-the-art denoising cycle GAN, CIRCLE GAN, and a conventional mathematical reconstruction algorithm incorporating total variation minimization, our Self-Attention GAN for CT image reconstruction produces competitive results on solving limited-angle data reconstruction problems. On sparse view and low-dose scenarios, our method is not always the best among compared methods, but produces competitive results in some situations.
Deep Learning For Biomedical Image Reconstruction
DOWNLOAD
Author : Jong Chul Ye
language : en
Publisher: Cambridge University Press
Release Date : 2023-09-30
Deep Learning For Biomedical Image Reconstruction written by Jong Chul Ye and has been published by Cambridge University Press this book supported file pdf, txt, epub, kindle and other format this book has been release on 2023-09-30 with Technology & Engineering categories.
Discover the power of deep neural networks for image reconstruction with this state-of-the-art review of modern theories and applications. The background theory of deep learning is introduced step-by-step, and by incorporating modeling fundamentals this book explains how to implement deep learning in a variety of modalities, including X-ray, CT, MRI and others. Real-world examples demonstrate an interdisciplinary approach to medical image reconstruction processes, featuring numerous imaging applications. Recent clinical studies and innovative research activity in generative models and mathematical theory will inspire the reader towards new frontiers. This book is ideal for graduate students in Electrical or Biomedical Engineering or Medical Physics.
Deep Learning For Computed Tomography Reconstruction
DOWNLOAD
Author : Johannes Leuschner
language : en
Publisher:
Release Date : 2023
Deep Learning For Computed Tomography Reconstruction written by Johannes Leuschner and has been published by this book supported file pdf, txt, epub, kindle and other format this book has been release on 2023 with categories.
X-ray computed tomography (CT) is a highly relevant imaging technique with clinical and industrial applications. At its core, CT involves an image reconstruction task from detector measurements that are acquired from multiple projection angles. Improving CT reconstruction using deep learning, which is being explored and utilized in various fields, is a subject of recent and current research. This thesis comprises six papers, whose contributions can be summarized as two-fold. First, several deep learning approaches are compared quantitatively and qualitatively, involving the creation of a benchmark dataset as well as the realization and evaluation of challenges for learned low-dose and sparse-view CT reconstruction. Second, several extensions of the deep image prior (DIP)--an unsupervised deep learning image reconstruction framework--are investigated. This includes its application to CT using total-variation regularization, pretraining on synthetically generated data, and uncertainty estimation via a probabilistic model. These extensions benefit DIP-based CT reconstruction in several ways, such as an improved reconstruction quality, an accelerated reconstruction process, and the identification of potential errors in the reconstruction. Additionally, a Bayesian experimental design approach utilizing the uncertainty estimation is studied for the selection of scanning angles based on a pilot scan. Complementing the papers, which are included without any modifications in the second part of this thesis, the first part introduces relevant foundations, as well as a large overview of literature on deep learning for CT reconstruction.
Deep Learning Based Computed Tomography Image Processing
DOWNLOAD
Author : Setareh Dabiri
language : en
Publisher:
Release Date : 2022
Deep Learning Based Computed Tomography Image Processing written by Setareh Dabiri and has been published by this book supported file pdf, txt, epub, kindle and other format this book has been release on 2022 with categories.
Computed Tomography (CT) is one of the most common modalities of medical imaging. CT scan has become a useful screening tool for body composition analysis and spinal conditions. Body composition is an emerging biomarker for cancer diagnosis and treatment. The assessment of the body composition profile of cancer patients during treatment and survivorship revealed the critical role of skeletal muscle mass in drug toxicity, hospital stay, infection rate and survival outcome. In this thesis, we propose a set of automatic CT image analysis frameworks for skeletal muscle mass and adipose tissue segmentation. This pipeline includes middle cross-sectional slice classification at the third lumbar vertebra in a CT-scan volume and muscle and adipose tissue segmentation at the third and fourth lumbar vertebrae levels. A multi-class segmentation network is trained to generate the segmentation map of skeletal muscle, subcutaneous adipose tissue, visceral adipose tissue and intramuscular adipose tissue in the third lumbar vertebrae level CT images. The model is designed to translate the spatial resolution from the feature maps of the encoder section to the decoder layers and learn the data representations at various receptive fields. To develop a computer-aided detection technique for vertebral column metastases and other spine related diseases, an automatic vertebral column segmentation and identification method is essential. In the second part of this thesis, a deep-learning based method for accurate pixel-level labelling of vertebrae on CT images is proposed. This algorithm includes 3D vertebral column segmentation and localization on CT-scan volumes. A two step semantic segmentation model utilizing a pixel-link map is introduced to tackle the vertebrae identification task. The proposed methods leverage deep learning algorithms to learn the representation of the data and map the input images to the desired output. Several datasets of CT images from various clinical institutions were obtained to train and evaluate the proposed models. These methods, accelerate the CT image analysis process and provide the information required for physicians to make a diagnosis.
Machine Learning For Medical Image Reconstruction
DOWNLOAD
Author : Nandinee Haq
language : en
Publisher: Springer Nature
Release Date : 2022-09-22
Machine Learning For Medical Image Reconstruction written by Nandinee Haq and has been published by Springer Nature this book supported file pdf, txt, epub, kindle and other format this book has been release on 2022-09-22 with Computers categories.
This book constitutes the refereed proceedings of the 5th International Workshop on Machine Learning for Medical Reconstruction, MLMIR 2022, held in conjunction with MICCAI 2022, in September 2022, held in Singapore. The 15 papers presented were carefully reviewed and selected from 19 submissions. The papers are organized in the following topical sections: deep learning for magnetic resonance imaging and deep learning for general image reconstruction.
Machine Learning For Tomographic Imaging
DOWNLOAD
Author : Ge Wang
language : en
Publisher: Programme: Iop Expanding Physi
Release Date : 2019-12-30
Machine Learning For Tomographic Imaging written by Ge Wang and has been published by Programme: Iop Expanding Physi this book supported file pdf, txt, epub, kindle and other format this book has been release on 2019-12-30 with Technology & Engineering categories.
Machine learning represents a paradigm shift in tomographic imaging, and image reconstruction is a new frontier of machine learning. This book will meet the needs of those who want to catch the wave of smart imaging. The book targets graduate students and researchers in the imaging community. Open network software, working datasets, and multimedia will be included. The first of its kind in the emerging field of deep reconstruction and deep imaging, Machine Learning for Tomographic Imaging presents the most essential elements, latest progresses and an in-depth perspective on this important topic.
Machine Learning For Medical Image Reconstruction
DOWNLOAD
Author : Florian Knoll
language : en
Publisher: Springer Nature
Release Date : 2019-10-24
Machine Learning For Medical Image Reconstruction written by Florian Knoll and has been published by Springer Nature this book supported file pdf, txt, epub, kindle and other format this book has been release on 2019-10-24 with Computers categories.
This book constitutes the refereed proceedings of the Second International Workshop on Machine Learning for Medical Reconstruction, MLMIR 2019, held in conjunction with MICCAI 2019, in Shenzhen, China, in October 2019. The 24 full papers presented were carefully reviewed and selected from 32 submissions. The papers are organized in the following topical sections: deep learning for magnetic resonance imaging; deep learning for computed tomography; and deep learning for general image reconstruction.
Machine Learning For Medical Image Reconstruction
DOWNLOAD
Author : Florian Knoll
language : en
Publisher: Springer
Release Date : 2018-09-11
Machine Learning For Medical Image Reconstruction written by Florian Knoll and has been published by Springer this book supported file pdf, txt, epub, kindle and other format this book has been release on 2018-09-11 with Computers categories.
This book constitutes the refereed proceedings of the First International Workshop on Machine Learning for Medical Reconstruction, MLMIR 2018, held in conjunction with MICCAI 2018, in Granada, Spain, in September 2018. The 17 full papers presented were carefully reviewed and selected from 21 submissions. The papers are organized in the following topical sections: deep learning for magnetic resonance imaging; deep learning for computed tomography, and deep learning for general image reconstruction.
Deep Learning Based Methods For Image Reconstruction In Cardiac Ct And Cardiac Cine Mri
DOWNLOAD
Author : Andreas Kofler
language : en
Publisher:
Release Date : 2021
Deep Learning Based Methods For Image Reconstruction In Cardiac Ct And Cardiac Cine Mri written by Andreas Kofler and has been published by this book supported file pdf, txt, epub, kindle and other format this book has been release on 2021 with categories.
Computed Tomography
DOWNLOAD
Author : Willi A. Kalender
language : en
Publisher: John Wiley & Sons
Release Date : 2011-07-07
Computed Tomography written by Willi A. Kalender and has been published by John Wiley & Sons this book supported file pdf, txt, epub, kindle and other format this book has been release on 2011-07-07 with Medical categories.
The book offers a comprehensive and user-oriented description of the theoretical and technical system fundamentals of computed tomography (CT) for a wide readership, from conventional single-slice acquisitions to volume acquisition with multi-slice and cone-beam spiral CT. It covers in detail all characteristic parameters relevant for image quality and all performance features significant for clinical application. Readers will thus be informed how to use a CT system to an optimum depending on the different diagnostic requirements. This includes a detailed discussion about the dose required and about dose measurements as well as how to reduce dose in CT. All considerations pay special attention to spiral CT and to new developments towards advanced multi-slice and cone-beam CT. For the third edition most of the contents have been updated and latest topics like dual source CT, dual energy CT, flat detector CT and interventional CT have been added. The enclosed CD-ROM again offers copies of all figures in the book and attractive case studies, including many examples from the most recent 64-slice acquisitions, and interactive exercises for image viewing and manipulation. This book is intended for all those who work daily, regularly or even only occasionally with CT: physicians, radiographers, engineers, technicians and physicists. A glossary describes all the important technical terms in alphabetical order. The enclosed DVD again offers attractive case studies, including many examples from the most recent 64-slice acquisitions, and interactive exercises for image viewing and manipulation. This book is intended for all those who work daily, regularly or even only occasionally with CT: physicians, radiographers, engineers, technicians and physicists. A glossary describes all the important technical terms in alphabetical order.