Deep Learning Image Synthesis For Mri
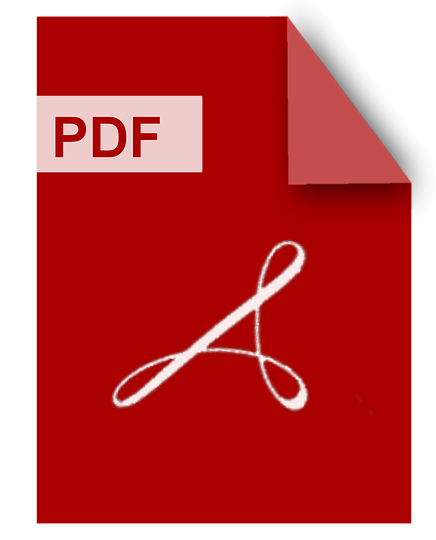
DOWNLOAD
Download Deep Learning Image Synthesis For Mri PDF/ePub or read online books in Mobi eBooks. Click Download or Read Online button to get Deep Learning Image Synthesis For Mri book now. This website allows unlimited access to, at the time of writing, more than 1.5 million titles, including hundreds of thousands of titles in various foreign languages. If the content not found or just blank you must refresh this page
Deep Learning Image Synthesis For Mri
DOWNLOAD
Author : Evan Masataka Masutani
language : en
Publisher:
Release Date : 2022
Deep Learning Image Synthesis For Mri written by Evan Masataka Masutani and has been published by this book supported file pdf, txt, epub, kindle and other format this book has been release on 2022 with categories.
Since its invention in the 1970s, magnetic resonance imaging (MRI) has contributed greatly to our understanding of the human body in health and disease. MRI images anatomy and physiology with high spatiotemporal resolution and without ionizing radiation. Due to these factors, MRI is particularly well suited to studying the heart, and cardiac MRI is considered the clinical gold-standard for assessment of cardiac morphology, flow, and function. However, interpretation of cardiac MRI is highly dependent on image quality and often requires extensive manual annotation and visual analysis. Convolutional neural networks (CNNs), a form of deep learning and artificial intelligence, have potential to revolutionize medical imaging. Broadly, CNNs comprise a series of trainable weights called layers, which iteratively learn the features required to perform a given task. Currently, CNNs are being explored for a variety of computer vision tasks, such as classification, localization, and segmentation, but have untapped potential. Specifically, their ability to perform image synthesis is unknown. Given these challenges in MRI and the untapped potential of CNNs, I asked: can we use deep learning to perform image synthesis for MRI? Using this question as the bedrock for my dissertation, I set out to solve progressively more challenging problems in cardiovascular MRI using CNNs, building towards the ultimate task of automatically quantifying cardiac function and biomechanics. In aim 1, I asked whether existing CNNs can enhance low-resolution cardiac images. That is, can CNNs perform image super-resolution of steady-state free precession (SSFP)? Specifically, I asked which CNN architectures are suitable for this task and how well they perform relative to conventional image upscaling methods. In aim 2, I asked whether I could upgrade the CNN architectures from aim 1 to isolate and remove background signal from 4D Flow MRI. That is, can CNNs perform phase-error correction of 4D Flow MRI acquisitions via synthesis of the background static vector field? To achieve this, I asked what architectural modifications are necessary to infer these multi-component volumetric vector fields. I then compared CNN-based phase error correction with existing manual segmentation-based methods. In aim 3, I asked whether I could further upgrade my phase-error correction CNN from aim 2 to predict intracardiac blood flow from videos of the beating heart. That is, can CNNs infer dynamic blood flow velocity fields from cardiac cine SSFP images? Specifically, I asked how I could incorporate spatiotemporal information and anatomical boundaries into this new architecture I call Triton-Net. I then measured the correlation between the synthesized flow fields and 4D Flow MRI measurements. Lastly, I asked whether I could use these flow values to detect left ventricular outflow obstruction. Finally, in aim 4, I asked whether I could refine Triton-Net to evaluate local myocardial function. That is, could I add explicit physical constraints into Triton-Net to infer dynamic myocardial velocity and strain tensor fields from cardiac cine SSFP images? Realizing that myocardial contraction is periodic, I explored how I may encode net-zero displacement and strain constraints into the Triton-Net architecture, resulting in a heavily modified deep learning synthetic strain (DLSS) CNN. I then characterized DLSS strain in a healthy population and asked whether I could use DLSS strain to identify wall motion abnormalities in an ischemic heart disease population. Lastly, I compared DLSS classification performance against the consensus visual assessment of four cardiothoracic radiologist readers.
Biomedical Image Synthesis And Simulation
DOWNLOAD
Author : Ninon Burgos
language : en
Publisher: Academic Press
Release Date : 2022-06-18
Biomedical Image Synthesis And Simulation written by Ninon Burgos and has been published by Academic Press this book supported file pdf, txt, epub, kindle and other format this book has been release on 2022-06-18 with Computers categories.
Biomedical Image Synthesis and Simulation: Methods and Applications presents the basic concepts and applications in image-based simulation and synthesis used in medical and biomedical imaging. The first part of the book introduces and describes the simulation and synthesis methods that were developed and successfully used within the last twenty years, from parametric to deep generative models. The second part gives examples of successful applications of these methods. Both parts together form a book that gives the reader insight into the technical background of image synthesis and how it is used, in the particular disciplines of medical and biomedical imaging. The book ends with several perspectives on the best practices to adopt when validating image synthesis approaches, the crucial role that uncertainty quantification plays in medical image synthesis, and research directions that should be worth exploring in the future. Gives state-of-the-art methods in (bio)medical image synthesis Explains the principles (background) of image synthesis methods Presents the main applications of biomedical image synthesis methods
Medical Image Synthesis
DOWNLOAD
Author : Xiaofeng Yang
language : en
Publisher: CRC Press
Release Date : 2024-02-06
Medical Image Synthesis written by Xiaofeng Yang and has been published by CRC Press this book supported file pdf, txt, epub, kindle and other format this book has been release on 2024-02-06 with Medical categories.
Image synthesis across and within medical imaging modalities is an active area of research with broad applications in radiology and radiation oncology. This book covers the principles and methods of medical image synthesis, along with state-of-the-art research. First, various traditional non-learning-based, traditional machine-learning-based, and recent deep-learning-based medical image synthesis methods are reviewed. Second, specific applications of different inter- and intra-modality image synthesis tasks and of synthetic image-aided segmentation and registration are introduced and summarized, listing and highlighting the proposed methods, study designs, and reported performances with the related clinical applications of representative studies. Third, the clinical usages of medical image synthesis, such as treatment planning and image-guided adaptive radiotherapy, are discussed. Last, the limitations and current challenges of various medical synthesis applications are explored, along with future trends and potential solutions to solve these difficulties. The benefits of medical image synthesis have sparked growing interest in a number of advanced clinical applications, such as magnetic resonance imaging (MRI)-only radiation therapy treatment planning and positron emission tomography (PET)/MRI scanning. This book will be a comprehensive and exciting resource for undergraduates, graduates, researchers, and practitioners.
Brain Tumor Mri Image Segmentation Using Deep Learning Techniques
DOWNLOAD
Author : Jyotismita Chaki
language : en
Publisher: Academic Press
Release Date : 2021-11-27
Brain Tumor Mri Image Segmentation Using Deep Learning Techniques written by Jyotismita Chaki and has been published by Academic Press this book supported file pdf, txt, epub, kindle and other format this book has been release on 2021-11-27 with Science categories.
Brain Tumor MRI Image Segmentation Using Deep Learning Techniques offers a description of deep learning approaches used for the segmentation of brain tumors. The book demonstrates core concepts of deep learning algorithms by using diagrams, data tables and examples to illustrate brain tumor segmentation. After introducing basic concepts of deep learning-based brain tumor segmentation, sections cover techniques for modeling, segmentation and properties. A focus is placed on the application of different types of convolutional neural networks, like single path, multi path, fully convolutional network, cascade convolutional neural networks, Long Short-Term Memory - Recurrent Neural Network and Gated Recurrent Units, and more. The book also highlights how the use of deep neural networks can address new questions and protocols, as well as improve upon existing challenges in brain tumor segmentation. Provides readers with an understanding of deep learning-based approaches in the field of brain tumor segmentation, including preprocessing techniques Integrates recent advancements in the field, including the transformation of low-resolution brain tumor images into super-resolution images using deep learning-based methods, single path Convolutional Neural Network based brain tumor segmentation, and much more Includes coverage of Long Short-Term Memory (LSTM) based Recurrent Neural Network (RNN), Gated Recurrent Units (GRU) based Recurrent Neural Network (RNN), Generative Adversarial Networks (GAN), Auto Encoder based brain tumor segmentation, and Ensemble deep learning Model based brain tumor segmentation Covers research Issues and the future of deep learning-based brain tumor segmentation
Deep Learning For Biomedical Image Reconstruction
DOWNLOAD
Author : Jong Chul Ye
language : en
Publisher: Cambridge University Press
Release Date : 2023-09-30
Deep Learning For Biomedical Image Reconstruction written by Jong Chul Ye and has been published by Cambridge University Press this book supported file pdf, txt, epub, kindle and other format this book has been release on 2023-09-30 with Technology & Engineering categories.
Discover the power of deep neural networks for image reconstruction with this state-of-the-art review of modern theories and applications. Including interdisciplinary examples and a step-by-step background of deep learning, this book provides insight into the future of biomedical image reconstruction with clinical studies and mathematical theory.
Simulation And Synthesis In Medical Imaging
DOWNLOAD
Author : Ninon Burgos
language : en
Publisher: Springer Nature
Release Date : 2020-09-23
Simulation And Synthesis In Medical Imaging written by Ninon Burgos and has been published by Springer Nature this book supported file pdf, txt, epub, kindle and other format this book has been release on 2020-09-23 with Computers categories.
This book constitutes the refereed proceedings of the 5th International Workshop on Simulation and Synthesis in Medical Imaging, SASHIMI 2020, held in conjunction with MICCAI 2020, in Lima, Peru, in October 2020. The 19 full papers presented were carefully reviewed and selected from 27 submissions. The contributions span the following broad categories in alignment with the initial call-for-papers: methods based on generative models or adversarial learning for MRI/CT/PET/microscopy image synthesis, and several applications of image synthesis and simulation for data augmentation, image enhancement or segmentation.
Deep Learning And Convolutional Neural Networks For Medical Imaging And Clinical Informatics
DOWNLOAD
Author : Le Lu
language : en
Publisher: Springer Nature
Release Date : 2019-09-19
Deep Learning And Convolutional Neural Networks For Medical Imaging And Clinical Informatics written by Le Lu and has been published by Springer Nature this book supported file pdf, txt, epub, kindle and other format this book has been release on 2019-09-19 with Computers categories.
This book reviews the state of the art in deep learning approaches to high-performance robust disease detection, robust and accurate organ segmentation in medical image computing (radiological and pathological imaging modalities), and the construction and mining of large-scale radiology databases. It particularly focuses on the application of convolutional neural networks, and on recurrent neural networks like LSTM, using numerous practical examples to complement the theory. The book’s chief features are as follows: It highlights how deep neural networks can be used to address new questions and protocols, and to tackle current challenges in medical image computing; presents a comprehensive review of the latest research and literature; and describes a range of different methods that employ deep learning for object or landmark detection tasks in 2D and 3D medical imaging. In addition, the book examines a broad selection of techniques for semantic segmentation using deep learning principles in medical imaging; introduces a novel approach to text and image deep embedding for a large-scale chest x-ray image database; and discusses how deep learning relational graphs can be used to organize a sizable collection of radiology findings from real clinical practice, allowing semantic similarity-based retrieval. The intended reader of this edited book is a professional engineer, scientist or a graduate student who is able to comprehend general concepts of image processing, computer vision and medical image analysis. They can apply computer science and mathematical principles into problem solving practices. It may be necessary to have a certain level of familiarity with a number of more advanced subjects: image formation and enhancement, image understanding, visual recognition in medical applications, statistical learning, deep neural networks, structured prediction and image segmentation.
Deep Learning In Medical Image Analysis
DOWNLOAD
Author : Gobert Lee
language : en
Publisher: Springer Nature
Release Date : 2020-02-06
Deep Learning In Medical Image Analysis written by Gobert Lee and has been published by Springer Nature this book supported file pdf, txt, epub, kindle and other format this book has been release on 2020-02-06 with Medical categories.
This book presents cutting-edge research and applications of deep learning in a broad range of medical imaging scenarios, such as computer-aided diagnosis, image segmentation, tissue recognition and classification, and other areas of medical and healthcare problems. Each of its chapters covers a topic in depth, ranging from medical image synthesis and techniques for muskuloskeletal analysis to diagnostic tools for breast lesions on digital mammograms and glaucoma on retinal fundus images. It also provides an overview of deep learning in medical image analysis and highlights issues and challenges encountered by researchers and clinicians, surveying and discussing practical approaches in general and in the context of specific problems. Academics, clinical and industry researchers, as well as young researchers and graduate students in medical imaging, computer-aided-diagnosis, biomedical engineering and computer vision will find this book a great reference and very useful learning resource.
Gans For Data Augmentation In Healthcare
DOWNLOAD
Author : Arun Solanki
language : en
Publisher: Springer Nature
Release Date : 2023-12-15
Gans For Data Augmentation In Healthcare written by Arun Solanki and has been published by Springer Nature this book supported file pdf, txt, epub, kindle and other format this book has been release on 2023-12-15 with Medical categories.
Computer-Assisted Diagnostics (CAD) using Convolutional Neural Network (CNN) model has become an important technology in the medical industry, improving the accuracy of diagnostics. However, the lack Magnetic Resonance Imaging (MRI) data leads to the failure of the depth study algorithm. Medical records often different because of the cost of obtaining information and the time-consuming information. In general, clinical data are unreliable, the training of neural network methods to distribute disease across classes does not yield the desired results. Data augmentation is often done by training data to solve problems caused by augmentation tasks such as scaling, cropping, flipping, padding, rotation, translation, affine transformation, and color augmentation techniques such as brightness, contrast, saturation, and hue. Data Augmentation and Segmentation imaging using GAN can be used to provide clear images of brain, liver, chest, abdomen, and liver on MRI. In addition, GAN shows strong promise in the field of clinical image synthesis. In many cases, clinical evaluation is limited by a lack of data and/or the cost of actual information. GAN can overcome these problems by enabling scientists and clinicians to work on beautiful and realistic images. This can improve diagnosis, prognosis, and disease. Finally, GAN highlights the potential for location of patient information with data. This is a beneficial clinical application of GAN because it can effectively protect patient confidentiality. This book covers the application of GANs on medical imaging augmentation and segmentation.
Simulation And Synthesis In Medical Imaging
DOWNLOAD
Author : Ali Gooya
language : en
Publisher: Springer
Release Date : 2018-09-11
Simulation And Synthesis In Medical Imaging written by Ali Gooya and has been published by Springer this book supported file pdf, txt, epub, kindle and other format this book has been release on 2018-09-11 with Computers categories.
This book constitutes the refereed proceedings of the Third International Workshop on Simulation and Synthesis in Medical Imaging, SASHIMI 2018, held in conjunction with MICCAI 2018, in Granada, Spain, in September 2018. The 14 full papers presented were carefully reviewed and selected from numerous submissions. This workshop continues to provide a state-of-the-art and integrative perspective on simulation and synthesis in medical imaging for the purpose of invigorating research and stimulating new ideas on how to build theoretical links, practical synergies, and best practices between these two research directions.