Deep Learning Optics For Computational Microscopy And Diffractive Computing
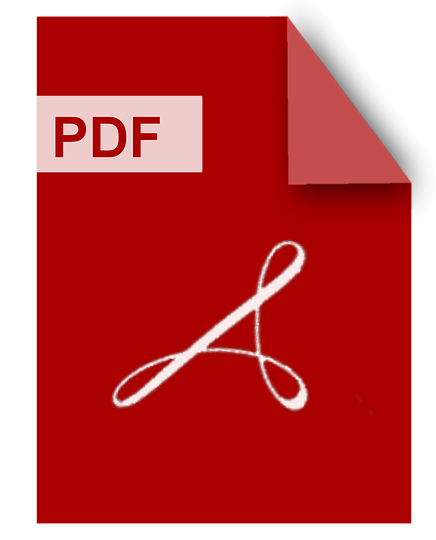
DOWNLOAD
Download Deep Learning Optics For Computational Microscopy And Diffractive Computing PDF/ePub or read online books in Mobi eBooks. Click Download or Read Online button to get Deep Learning Optics For Computational Microscopy And Diffractive Computing book now. This website allows unlimited access to, at the time of writing, more than 1.5 million titles, including hundreds of thousands of titles in various foreign languages. If the content not found or just blank you must refresh this page
Deep Learning Optics For Computational Microscopy And Diffractive Computing
DOWNLOAD
Author : Bijie Bai
language : en
Publisher:
Release Date : 2023
Deep Learning Optics For Computational Microscopy And Diffractive Computing written by Bijie Bai and has been published by this book supported file pdf, txt, epub, kindle and other format this book has been release on 2023 with categories.
The rapid development of machine learning has transformed conventional optical imaging processes, setting new benchmarks in computational imaging tasks. In this dissertation, we delve into the transformative impact of recent advancements in machine learning on optical imaging processes, focusing on how these technologies revolutionize computational imaging tasks. Specifically, this dissertation centers on two major topics: deep learning-enabled computational microscopy and the all-optical diffractive networks. Optical microscopy has long served as the benchmark technique for diagnosing various diseases over centuries. However, its reliance on high-end optical components and accessories, necessary to adapt to various imaging samples and conditions, often limits its applicability and throughput. Recent advancements in computational imaging techniques utilizing deep learning methods have transformed conventional microscopic imaging modalities, delivering both enhanced speed and superior image quality without introducing extra complexity of the optical systems. In the first topic of this dissertation, we demonstrate that deep learning-enabled image translation approach can significantly benefit a wide range of applications for microscopic imaging. We start with introducing a customized system for single-shot quantitative polarization imaging, capable of reconstructing comprehensive birefringent maps from a single image capture, which offers enhanced sensitivity and specificity in diagnosing crystal-induced diseases. Utilizing these quantitative birefringent maps as a baseline, we employ deep learning tools to convert phase-recovered holograms into quantitative birefringence maps, thereby improving the throughput of crystal detection with simplified system complexity. Extending this concept of deep learning-enabled image translation, we also explore its applications in histopathology. Our technique, termed as "virtual histological staining", transforms unstained biological samples into visually rich, stained-like images without the need for chemical agents. This innovation minimizes costs, labor, and diagnostic delays as well as opens up new possibilities in histopathology workflow. The evolution of deep learning tools not only facilities the optical image analysis and processing, but also provides guidance in design and enhancement of optical systems. The second topic of this dissertation is the development and application of diffractive deep neural networks (D2NN). Developed with deep learning, D2NNs execute given computational tasks by manipulating light diffraction through a series of engineered surfaces, which is completed at the speed of light propagation with negligible power consumption. Based on this framework, a lot of novel computational tasks can be executed in an all-optical way, which is beyond the capabilities of the traditional optics design approaches. We introduce several all-optical computational imaging applications based on D2NN, including class-specific imaging, class-specific image encryption, and unidirectional image magnification and demagnification, demonstrating the versatility of this promising framework.
Deep Learning Enabled Computational Imaging In Optical Microscopy And Air Quality Monitoring
DOWNLOAD
Author : Yichen Wu
language : en
Publisher:
Release Date : 2019
Deep Learning Enabled Computational Imaging In Optical Microscopy And Air Quality Monitoring written by Yichen Wu and has been published by this book supported file pdf, txt, epub, kindle and other format this book has been release on 2019 with categories.
Exponential advancements in computational resources and algorithms have given birth to the new paradigm in imaging that rely on computation to digitally reconstruct and enhance images. These computational imaging modalities have enabled higher resolution, larger throughput and/or automatic detection capabilities for optical microscopy. An example is lens-less digital holographic microscope, which enables snapshot imaging of volumetric samples over wide field-of-view without using imaging lenses. Recent developments in the field of deep learning have further opened up exciting avenues for computational imaging, which offer unprecedented performance thanks to their capability to robustly learn content-specific complex image priors. This dissertation introduces a novel and universal modeling framework of deep learning -based image reconstruction technique to tackle various challenges in optical microscopic imaging, including digital holography reconstructions and 3D fluorescence microscopy. Firstly, auto-focusing and phase recovery in holography reconstruction are conventionally challenging and time-consuming to digitally perform. A convolutional neural network (CNN) based approach was developed that solves both problems rapidly in parallel, enabling extended depth-of-field holographic reconstruction with significantly improved time complexity from O(mn) to O(1). Secondly, to fuse advantages of snapshot volumetric capability in digital holography and speckle- and artifact-free image contrast in bright-field microscopy, a CNN was used to transform across microscopy modalities from holographic image reconstructions to their equivalent high contrast bright-field microscopic images. Thirdly, 3D fluorescence microscopy generally requires axial scanning. A CNN was trained to learn defocuses of fluorescence and digitally refocusing a single 2D fluorescence image onto user-defined 3D surfaces within the sample volume, which extends depth-of-field of fluorescence microscopy by 20-fold without any axial scanning, additional hardware, or a trade-off of imaging resolution or speed. This enables high-speed volumetric imaging and digital aberration correction for live samples. Based on deep learning powered computational microscopy, a hand-held device was also developed to measure the particulate matters and bio-aerosols in the air using the lens-less digital holographic microscopic imaging geometry. This device, named c-Air, demonstrates accurate, high-throughput and automatic detection, sizing and classification of the particles in the air, which opens new opportunities in deep learning based environmental sensing and personalized and/or distributed air quality monitoring.
Computational Deep Learning Microscopy
DOWNLOAD
Author : Kevin De Haan
language : en
Publisher:
Release Date : 2022
Computational Deep Learning Microscopy written by Kevin De Haan and has been published by this book supported file pdf, txt, epub, kindle and other format this book has been release on 2022 with categories.
Over the past decade, deep learning has become one of the leading techniques used in the field of image processing. Beyond popular tasks in computer vision such as classification and segmentation, it has proven to be revolutionary in its applications for image enhancement and transformations. It has significantly changed the field of computational optics - and neural networks can now be used to accurately and rapidly solve a wide variety of inverse problems in microscopy. This dissertation discusses a few major classes of inverse imaging problems that can be solved using deep learning. The dissertation first presents, a framework that can be used to enhance microscopy images using single image super-resolution . This framework has been proven to be effective at super-resolving images captured with a holographic microscope that are resolution limited both by the number of pixels used for imaging, as well as by the numerical aperture (NA) of the microscope. The effectiveness of this same general framework beyond optical microscopy, will be further demonstrated by super resolving electron microscopy images. Next, the dissertation will show that a similar super-resolution framework can be extended to perform a transformation between two imaging modalities and improve the overall quality of images by using it to enhance images of thin blood smears captured by a cost-effective mobile-phone microscope. By enhancing mobile phone microscopy images to match the quality of a top-of-the-line benchtop microscope, the images are standardized, have their resolution improved and have aberrations removed, allowing the images to be used for screening of sickle cell disease. Using a deep learning based classification framework, 98% accuracy was achieved during blind tested of 96 human blood smear slides. Furthermore, by enhancing the images, the image quality is brought to a level which can be used by clinicians for further analysis if required. Finally, this same framework will be used to transform microscopy images and generate images from that are equivalent to those which have undergone chemical labeling and show some of the many applications of this technology. The technique was applied to virtual staining of label-free thin histological tissue sections which were used to generate multiple stains from a single tissue section, enabling different stains to be performed at the microscopic level, as well as blending of stains together - creating entirely new digital stains. This dissertation shows how multiple virtual stains can be used to generate synthetic datasets of perfectly matched stains, allowing downstream networks be trained to perform transformations between stains. The efficacy of three of these stain transformation networks - generating the Masson's trichrome, Jones silver stain, and periodic acid-Schiff stains from hematoxylin and eosin-stained kidney tissue are demonstrated in a diagnostic study, with the results showing the improvement that such technology can bring to patient care.
Deep Optics
DOWNLOAD
Author : Julie Chang
language : en
Publisher:
Release Date : 2019
Deep Optics written by Julie Chang and has been published by this book supported file pdf, txt, epub, kindle and other format this book has been release on 2019 with categories.
Over the past decade, computational imaging has transformed the expectations we have for our cameras. At the fingertips of anyone with an iPhone X, for example, are panoramas, high dynamic range (HDR) photography, and Portrait mode--all classic examples of computational imaging. This integration of optical design and image processing has also enabled us to see around corners and behind walls, observe molecular dynamics during chemical reactions, and even to piece together the first image of a black hole. For each application though, the system needs to be reformulated and designed, and it is difficult to claim that the final solution is actually optimal. Concurrently, a tremendous amount of progress has been made in the field of deep learning. Convolutional neural networks (CNNs) have found success in a wide variety of computer vision tasks, including image classification, object detection, image generation, and more. However, this high performance comes at a high computational cost, and it remains difficult to deploy CNNs in embedded systems due to tight power budgets. Through earlier projects discussed in Section I, we gain an understanding of the ways computational imaging and deep learning could potentially enhance and benefit from the other. In section II, we present the concept of deep optics, in which the optical model is jointly optimized with the task-specific algorithm. Deep optics capitalizes on the recent successes of deep learning for computer vision and uses the same data-driven approach to optimize optical parameters together with subsequent neural network parameters. Not only could this improve computer vision performance over basic or heuristically designed cameras, but it could also relieve some of the computational cost by partially pre-processing the data in optics. We begin by building a hybrid optical-electronic convolutional neural network for image classification, specifically focusing on the concept of an optimizable optical convolutional layer to replace the standard electronic convolutional layer. Finally, we apply deep optics to the problems of monocular depth estimation and 3D object detection, demonstrating how these common computer vision tasks that typically ignore the image capture process can be improved by proper modeling and optimization of the optics.
Computational Optical Phase Imaging
DOWNLOAD
Author : Cheng Liu
language : en
Publisher: Springer Nature
Release Date : 2022-04-11
Computational Optical Phase Imaging written by Cheng Liu and has been published by Springer Nature this book supported file pdf, txt, epub, kindle and other format this book has been release on 2022-04-11 with Science categories.
In this book, computational optical phase imaging techniques are presented along with Matlab codes that allow the reader to run their own simulations and gain a thorough understanding of the current state-of-the-art. The book focuses on modern applications of computational optical phase imaging in engineering measurements and biomedical imaging. Additionally, it discusses the future of computational optical phase imaging, especially in terms of system miniaturization and deep learning-based phase retrieval.
Computational Methods And Deep Learning For Ophthalmology
DOWNLOAD
Author : D. Jude Hemanth
language : en
Publisher: Elsevier
Release Date : 2023-02-18
Computational Methods And Deep Learning For Ophthalmology written by D. Jude Hemanth and has been published by Elsevier this book supported file pdf, txt, epub, kindle and other format this book has been release on 2023-02-18 with Science categories.
Computational Methods and Deep Learning for Ophthalmology presents readers with the concepts and methods needed to design and use advanced computer-aided diagnosis systems for ophthalmologic abnormalities in the human eye. Chapters cover computational approaches for diagnosis and assessment of a variety of ophthalmologic abnormalities. Computational approaches include topics such as Deep Convolutional Neural Networks, Generative Adversarial Networks, Auto Encoders, Recurrent Neural Networks, and modified/hybrid Artificial Neural Networks. Ophthalmological abnormalities covered include Glaucoma, Diabetic Retinopathy, Macular Degeneration, Retinal Vein Occlusions, eye lesions, cataracts, and optical nerve disorders. This handbook provides biomedical engineers, computer scientists, and multidisciplinary researchers with a significant resource for addressing the increase in the prevalence of diseases such as Diabetic Retinopathy, Glaucoma, and Macular Degeneration. Presents the latest computational methods for designing and using Decision-Support Systems for ophthalmologic disorders in the human eye Conveys the role of a variety of computational methods and algorithms for efficient and effective diagnosis of ophthalmologic disorders, including Diabetic Retinopathy, Glaucoma, Macular Degeneration, Retinal Vein Occlusions, eye lesions, cataracts, and optical nerve disorders Explains how to develop and apply a variety of computational diagnosis systems and technologies, including medical image processing algorithms, bioinspired optimization, Deep Learning, computational intelligence systems, fuzzy-based segmentation methods, transfer learning approaches, and hybrid Artificial Neural Networks
Computer Design Of Diffractive Optics
DOWNLOAD
Author : V A Soifer
language : en
Publisher: Elsevier
Release Date : 2012-11-19
Computer Design Of Diffractive Optics written by V A Soifer and has been published by Elsevier this book supported file pdf, txt, epub, kindle and other format this book has been release on 2012-11-19 with Technology & Engineering categories.
Diffractive optics involves the manipulation of light using diffractive optical elements (DOEs). DOEs are being widely applied in such areas as telecommunications, electronics, laser technologies and biomedical engineering. Computer design of diffractive optics provides an authoritative guide to the principles and applications of computer-designed diffractive optics.The theoretical aspects underpinning diffractive optics are initially explored, including the main equations in diffraction theory and diffractive optical transformations. Application of electromagnetic field theory for calculating diffractive gratings and related methods in micro-optics are discussed, as is analysis of transverse modes of laser radiation and the formation of self-replicating multimode laser beams. Key applications of DOEs reviewed include geometrical optics approximation, scalar approximation and optical manipulation of micro objects, with additional consideration of multi-order DOEs and synthesis of DOEs on polycrystalline diamond films.With its distinguished editor and respected team of expert contributors, Computer design of diffractive optics is a comprehensive reference tool for professionals and academics working in the field of optical engineering and photonics. Explores the theoretical aspects underpinning diffractive optics Discusses key applications of diffractive optical elements A comprehensive reference for professionals and academics in optical engineering and photonics
Deep Learning In Optical Microscopy Holographic Imaging And Sensing
DOWNLOAD
Author : Tairan Liu
language : en
Publisher:
Release Date : 2022
Deep Learning In Optical Microscopy Holographic Imaging And Sensing written by Tairan Liu and has been published by this book supported file pdf, txt, epub, kindle and other format this book has been release on 2022 with categories.
The microscopy imaging technique has been employed as the gold-standard method for diagnosing numerous diseases for hundreds of years. However, the dependence on high-end optical components of traditional optical microscopes may often limit their usage in many applications. Recent developments in deep learning-enabled computational imaging techniques have revolutionized the field achieving both faster speed and higher image quality while maintaining the simplicity of the optical system.In the first part of this dissertation, a set of novel deep learning-enabled microscopy imaging techniques is introduced to perform super-resolution, color holography, and quantitative polarization imaging, which aims for improving the performance of the existing optical system. Firstly, deep learning was adopted to enhance the resolution of both pixel size-limited and diffraction-limited coherent imaging systems, providing a rapid, non-iterative method to improve the space-bandwidth product of coherent imaging systems. Then, a high-fidelity color image reconstruction method using a single hologram is presented, where deep learning was used to simultaneously eliminate the missing-phase-related artifacts and correct the color distortion. In addition, another deep learning-enabled holographic polarization microscope is demonstrated, which can obtain quantitative birefringence retardance and orientation information of the specimen from a phase-recovered hologram from one polarizer/analyzer pair. In the second part of this dissertation, deep learning is further applied to various biological imaging or sensing applications enabling these systems to perform virtual histology staining, cell classification, and pathogen detection. A digital staining technique is first demonstrated to transform the quantitative phase images (QPI) of label-free tissue sections into images equivalent to the brightfield microscopy images of the same tissue sections that are histologically stained. Next, using time-lapse lensless speckle imaging and a deep learning classifier, a computational cytometer is shown to rapidly detect magnetic bead-conjugated rare cells of interest in three dimensions (3D). Lastly, two deep learning-based pathogen detection frameworks are presented. A bacterial colony-forming-unit (CFU) detection system exploiting a thin-film-transistor (TFT)-based image sensor array is firstly shown which can save ~12 hours compared to the Environmental Protection Agency (EPA)-approved methods. Then, a stain-free quantitative viral plaque assay framework is presented which could automatically detect the first cell lysing events due to the viral replication as early as 5 hours after the incubation and achieved a >90% detection rate for the plaque-forming units (PFUs) with 100% specificity in
Fourier Ptychographic Imaging
DOWNLOAD
Author : Guoan Zheng
language : en
Publisher: Morgan & Claypool Publishers
Release Date : 2016-06-30
Fourier Ptychographic Imaging written by Guoan Zheng and has been published by Morgan & Claypool Publishers this book supported file pdf, txt, epub, kindle and other format this book has been release on 2016-06-30 with Science categories.
This book demonstrates the concept of Fourier ptychography, a new imaging technique that bypasses the resolution limit of the employed optics. In particular, it transforms the general challenge of high-throughput, high-resolution imaging from one that is coupled to the physical limitations of the optics to one that is solvable through computation. Demonstrated in a tutorial form and providing many MATLAB® simulation examples for the reader, it also discusses the experimental implementation and recent developments of Fourier ptychography. This book will be of interest to researchers and engineers learning simulation techniques for Fourier optics and the Fourier ptychography concept.
Deep Learning Assisted Phase Retrieval And Computational Methods In Coherent Diffractive Imaging
DOWNLOAD
Author : Alfredo Bellisario
language : en
Publisher:
Release Date : 2024
Deep Learning Assisted Phase Retrieval And Computational Methods In Coherent Diffractive Imaging written by Alfredo Bellisario and has been published by this book supported file pdf, txt, epub, kindle and other format this book has been release on 2024 with categories.