Detect Spammers In Online Social Networks
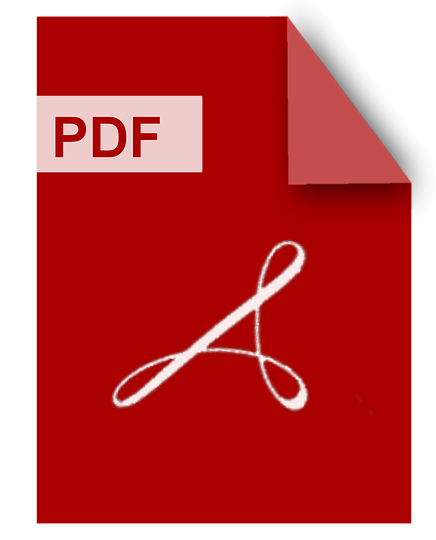
DOWNLOAD
Download Detect Spammers In Online Social Networks PDF/ePub or read online books in Mobi eBooks. Click Download or Read Online button to get Detect Spammers In Online Social Networks book now. This website allows unlimited access to, at the time of writing, more than 1.5 million titles, including hundreds of thousands of titles in various foreign languages. If the content not found or just blank you must refresh this page
Spammer Detection On Online Social Networks
DOWNLOAD
Author : Amit Anand Amlesahwaram
language : en
Publisher:
Release Date : 2013
Spammer Detection On Online Social Networks written by Amit Anand Amlesahwaram and has been published by this book supported file pdf, txt, epub, kindle and other format this book has been release on 2013 with categories.
Twitter with its rising popularity as a micro-blogging website has inevitably attracted attention of spammers. Spammers use myriad of techniques to lure victims into clicking malicious URLs. In this thesis, we present several novel features capable of distinguishing spam accounts from legitimate accounts in real-time. The features exploit the behavioral and content entropy, bait-techniques, community-orientation, and profile characteristics of spammers. We then use supervised learning algorithms to generate models using the proposed features and show that our tool, spAmbush, can detect spammers in real-time. Our analysis reveals detection of more than 90% of spammers with less than five tweets and more than half with only a single tweet. Our feature computation has low latency and resource requirement. Our results show a 96% detection rate with only 0.01% false positive rate. We further cluster the unknown spammers to identify and understand the prevalent spam campaigns on Twitter. The electronic version of this dissertation is accessible from http://hdl.handle.net/1969.1/148426
Detect Spammers In Online Social Networks
DOWNLOAD
Author : Yi Zhang
language : en
Publisher:
Release Date :
Detect Spammers In Online Social Networks written by Yi Zhang and has been published by this book supported file pdf, txt, epub, kindle and other format this book has been release on with categories.
Efficient Spam Detection Across Online Social Networks
DOWNLOAD
Author : Hailu Xu
language : en
Publisher:
Release Date : 2016
Efficient Spam Detection Across Online Social Networks written by Hailu Xu and has been published by this book supported file pdf, txt, epub, kindle and other format this book has been release on 2016 with Online social networks categories.
Online Social Networks (OSNs) have become more and more popular in the whole world recently. People share their personal activities, views, and opinions among different OSNs. Simultaneously, social spam appears more frequently and in various formats throughout popular OSNs. As big data theory receives much more attention, it is expected that OSNs will have more interactions with each other shortly. This would enable a spam link, content or profile attack to easily move from one social network like Twitter to other social networks like Facebook. Therefore, efficient detection of spam has become a significant and popular problem. This paper focuses on spam detection across multiple OSNs by leveraging the knowledge of detecting similar spam within an OSN and using it in different OSNs. We chose Facebook and Twitter for our study targets, considering that they share the most similar features in posts, topics, and user activities, etc. We collected two datasets from them and performed analysis based on our proposed methodology. The results show that detection combined with spam in Facebook show a more than 50% decrease of spam tweets in Twitter, and detection combined with spam of Twitter shows a nearly 71.2% decrease of spam posts in Facebook. This means similar spam of one social network can significantly facilitate spam detection in other social networks. We proposed a new perspective of spam detection in OSNs.
Spam Detection In Online Social Networks Using Feed Forward Neural Network
DOWNLOAD
Author : munish sabharwal
language : en
Publisher:
Release Date : 2018-10-03
Spam Detection In Online Social Networks Using Feed Forward Neural Network written by munish sabharwal and has been published by this book supported file pdf, txt, epub, kindle and other format this book has been release on 2018-10-03 with categories.
Social Networks define a path for consumers to continue contact with their friends. Social Networks' is increasingly the popularity allows of them to accumulator huge amounts of PI (Personal Information) about their consumers. Unhappily, spam information wealth as-well-as its simple to access consumers information can attract attackers class concentration. That's why social networks have been attacked by spammers while there has been a various work to identify and repair them.
Enhancing Spammer Detection In Online Social Networks With Trust Based Metrics
DOWNLOAD
Author : Alexander J. Murmann
language : en
Publisher:
Release Date : 2009
Enhancing Spammer Detection In Online Social Networks With Trust Based Metrics written by Alexander J. Murmann and has been published by this book supported file pdf, txt, epub, kindle and other format this book has been release on 2009 with Online social networks categories.
Analyzing And Detecting Social Spammers With Robust Features
DOWNLOAD
Author : Jianan Yue
language : en
Publisher:
Release Date : 2017
Analyzing And Detecting Social Spammers With Robust Features written by Jianan Yue and has been published by this book supported file pdf, txt, epub, kindle and other format this book has been release on 2017 with categories.
"The rapid growth of online social networks has attracted an increasing number of social spammers. Spammers gain profits by posting various content such as rumors and malwares. These behaviors greatly compromise social networks' privacy and security, and endanger the whole network community. In the last few years, researchers have proposed a number of spam detection strategies. However, spammers become harder to be detected as they constantly evolve to evade detection by emulating legitimate users and hiding spam patterns. Many detection methods become ineffective. In this thesis, we aim to design spam detection methods using features that are resilient to evolving spammers. To achieve this goal, we first conduct an in-depth analysis on different properties of user accounts. We study the Twitter accounts and extract four kinds of features: profile-based, content-based, community-based and time-based features. By analyzing evasion techniques used by current spammers, we find that the commonly-used profile and content-based features are not effective enough to uncover cunning spammers. This is because these features can be easily emulated by spammers. To tackle this issue, we investigate the structural properties of Twitter network topologies and propose community-based features. These community-based features are more robust than profile-based and content-based features due to the fact that community structure is determined by multiple accounts collectively. Compared to a normal user, a spammer is more likely to connect with other spammers. Moreover, we find that spammers often need to fulfill a task in a short period of time to reduce costs. Based on this phenomenon, we design new time-based features to capture spam outbreaks. In addition to effectiveness, we also consider efficiency as another important factor. We select computation-efficient features as potential candidates by comparing the time of feature construction. Our data-driven evaluation demonstrates that our advanced features largely improve the performance of the spam detection system. While achieving an even lower false positive rate, the detection rate increases by 16% and f1 score increases by over 10% when applying our feature set. " --
Spam Analysis And Detection For User Generated Content In Online Social Networks
DOWNLOAD
Author : Enhua Tan
language : en
Publisher:
Release Date : 2013
Spam Analysis And Detection For User Generated Content In Online Social Networks written by Enhua Tan and has been published by this book supported file pdf, txt, epub, kindle and other format this book has been release on 2013 with categories.
With the access to three large OSN user activity logs, including Yahoo! Blogs, Yahoo! Answers, and Yahoo! Del.icio.us, for a duration of up to 4.5 years, we are able to well analyze the patterns of content generation patterns of social network users in detail. Our analysis consistently shows that users' posting behavior in these networks exhibits strong daily and weekly patterns, but the user active time in these OSNs does not follow commonly assumed exponential distributions. We also show that the user posting behavior in these OSNs follows stretched exponential distributions instead of widely accepted power law distributions. Our discovery lays a foundation for user behavior analysis in social networks, and serves as a ground truth for anomaly detection and anti-spam.
Spam Detection On Online Social Media Networks
DOWNLOAD
Author : Vijay Sharma
language : en
Publisher:
Release Date : 2023-01-12
Spam Detection On Online Social Media Networks written by Vijay Sharma and has been published by this book supported file pdf, txt, epub, kindle and other format this book has been release on 2023-01-12 with Health & Fitness categories.
With the rise of social media, people started sharing opinions or experiences, exchanging ideas, providing suggestions etc. by using different means of online communication. We, the human beings, like to take the opinions or suggestions of our loved ones for taking decisions in our day-to-day activities. Nowadays, online opinions are playing a central role in people decision-making process. When it comes to online scenario, these opinions can be expressed in different forms on different online environments - people use online social networks like Facebook to express their feelings in the form of a post; micro-blogging websites like Twitter provide people a way to interact and share their thoughts globally in the form of a tweet; users write reviews about products or services on review platforms to share their experiences; people taking advice or leaving comment on a blog network; discussion forums provide rich content to people to have discussions on different topics of interest in the form of question/answer posts or comments;
Mining Content And Relations For Social Spammer Detection
DOWNLOAD
Author : Xia Hu (Ph.D.)
language : en
Publisher:
Release Date : 2015
Mining Content And Relations For Social Spammer Detection written by Xia Hu (Ph.D.) and has been published by this book supported file pdf, txt, epub, kindle and other format this book has been release on 2015 with Computer networks categories.
Social networking services have emerged as an important platform for large-scale information sharing and communication. With the growing popularity of social media, spamming has become rampant in the platforms. Complex network interactions and evolving content present great challenges for social spammer detection. Different from some existing well-studied platforms, distinct characteristics of newly emerged social media data present new challenges for social spammer detection. First, texts in social media are short and potentially linked with each other via user connections. Second, it is observed that abundant contextual information may play an important role in distinguishing social spammers and normal users. Third, not only the content information but also the social connections in social media evolve very fast. Fourth, it is easy to amass vast quantities of unlabeled data in social media, but would be costly to obtain labels, which are essential for many supervised algorithms. To tackle those challenges raise in social media data, I focused on developing effective and efficient machine learning algorithms for social spammer detection.I provide a novel and systematic study of social spammer detection in the dissertation. By analyzing the properties of social network and content information, I propose a unified framework for social spammer detection by collectively using the two types of information in social media. Motivated by psychological findings in physical world, I investigate whether sentiment analysis can help spammer detection in online social media. In particular, I conduct an exploratory study to analyze the sentiment differences between spammers and normal users; and present a novel method to incorporate sentiment information into social spammer detection framework. Given the rapidly evolving nature, I propose a novel framework to efficiently reflect the effect of newly emerging social spammers. To tackle the problem of lack of labeling data in social media, I study how to incorporate network information into text content modeling, and design strategies to select the most representative and informative instances from social media for labeling. Motivated by publicly available label information from other media platforms, I propose to make use of knowledge learned from cross-media to help spammer detection on social media.
Mining Content And Relations For Social Spammer Detection
DOWNLOAD
Author : Xia Hu
language : en
Publisher:
Release Date : 2015
Mining Content And Relations For Social Spammer Detection written by Xia Hu and has been published by this book supported file pdf, txt, epub, kindle and other format this book has been release on 2015 with Machine learning categories.
Social networking services have emerged as an important platform for large-scale information sharing and communication. With the growing popularity of social media, spamming has become rampant in the platforms. Complex network interactions and evolving content present great challenges for social spammer detection. Different from some existing well-studied platforms, distinct characteristics of newly emerged social media data present new challenges for social spammer detection. First, texts in social media are short and potentially linked with each other via user connections. Second, it is observed that abundant contextual information may play an important role in distinguishing social spammers and normal users. Third, not only the content information but also the social connections in social media evolve very fast. Fourth, it is easy to amass vast quantities of unlabeled data in social media, but would be costly to obtain labels, which are essential for many supervised algorithms. To tackle those challenges raise in social media data, I focused on developing effective and efficient machine learning algorithms for social spammer detection. I provide a novel and systematic study of social spammer detection in the dissertation. By analyzing the properties of social network and content information, I propose a unified framework for social spammer detection by collectively using the two types of information in social media. Motivated by psychological findings in physical world, I investigate whether sentiment analysis can help spammer detection in online social media. In particular, I conduct an exploratory study to analyze the sentiment differences between spammers and normal users; and present a novel method to incorporate sentiment information into social spammer detection framework. Given the rapidly evolving nature, I propose a novel framework to efficiently reflect the effect of newly emerging social spammers. To tackle the problem of lack of labeling data in social media, I study how to incorporate network information into text content modeling, and design strategies to select the most representative and informative instances from social media for labeling. Motivated by publicly available label information from other media platforms, I propose to make use of knowledge learned from cross-media to help spammer detection on social media.