Development Of A Deep Learning Scheme For Unconstrained Face Recognition
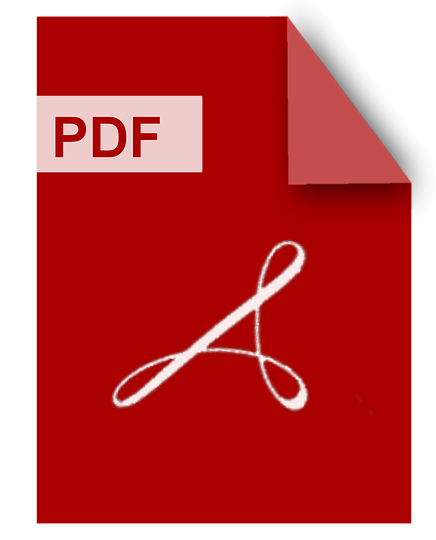
DOWNLOAD
Download Development Of A Deep Learning Scheme For Unconstrained Face Recognition PDF/ePub or read online books in Mobi eBooks. Click Download or Read Online button to get Development Of A Deep Learning Scheme For Unconstrained Face Recognition book now. This website allows unlimited access to, at the time of writing, more than 1.5 million titles, including hundreds of thousands of titles in various foreign languages. If the content not found or just blank you must refresh this page
Development Of A Deep Learning Scheme For Unconstrained Face Recognition
DOWNLOAD
Author : Marwa Yousif Hassan Said
language : en
Publisher:
Release Date : 2016
Development Of A Deep Learning Scheme For Unconstrained Face Recognition written by Marwa Yousif Hassan Said and has been published by this book supported file pdf, txt, epub, kindle and other format this book has been release on 2016 with categories.
Deep Learning Based Face Analytics
DOWNLOAD
Author : Nalini K Ratha
language : en
Publisher: Springer Nature
Release Date : 2021-08-16
Deep Learning Based Face Analytics written by Nalini K Ratha and has been published by Springer Nature this book supported file pdf, txt, epub, kindle and other format this book has been release on 2021-08-16 with Computers categories.
This book provides an overview of different deep learning-based methods for face recognition and related problems. Specifically, the authors present methods based on autoencoders, restricted Boltzmann machines, and deep convolutional neural networks for face detection, localization, tracking, recognition, etc. The authors also discuss merits and drawbacks of available approaches and identifies promising avenues of research in this rapidly evolving field. Even though there have been a number of different approaches proposed in the literature for face recognition based on deep learning methods, there is not a single book available in the literature that gives a complete overview of these methods. The proposed book captures the state of the art in face recognition using various deep learning methods, and it covers a variety of different topics related to face recognition. This book is aimed at graduate students studying electrical engineering and/or computer science. Biometrics is a course that is widely offered at both undergraduate and graduate levels at many institutions around the world: This book can be used as a textbook for teaching topics related to face recognition. In addition, the work is beneficial to practitioners in industry who are working on biometrics-related problems. The prerequisites for optimal use are the basic knowledge of pattern recognition, machine learning, probability theory, and linear algebra.
Towards A Robust Unconstrained Face Recognition Pipeline With Deep Neural Networks
DOWNLOAD
Author : Yichun Shi
language : en
Publisher:
Release Date : 2021
Towards A Robust Unconstrained Face Recognition Pipeline With Deep Neural Networks written by Yichun Shi and has been published by this book supported file pdf, txt, epub, kindle and other format this book has been release on 2021 with Electronic dissertations categories.
Face recognition is a classic problem in the field of computer vision and pattern recognition due to its wide applications in real-world problems such as access control, identity verification, physical security, surveillance, etc. Recent progress in deep learning techniques and the access to large-scale face databases has lead to a significant improvement of face recognition accuracy under constrained and semi-constrained scenarios. Deep neural networks are shown to surpass human performance on Labeled Face in the Wild (LFW), which consists of celebrity photos captured in the wild. However, in many applications, e.g. surveillance videos, where we cannot assume that the presented face is under controlled variations, the performance of current DNN-based methods drop significantly. The main challenges in such an unconstrained face recognition problem include, but are not limited to: lack of labeled data, robust face normalization, discriminative representation learning and the ambiguity of facial features caused by information loss.In this thesis, we propose a set of methods that attempt to address the above challenges in unconstrained face recognition systems. Starting from a classic deep face recognition pipeline, we review how each step in this pipeline could fail on low-quality uncontrolled input faces, what kind of solutions have been studied before, and then introduce our proposed methods. The various methods proposed in this thesis are independent but compatible with each other. Experiment on several challenging benchmarks, e.g. IJB-C and IJB-S show that the proposed methods are able to improve the robustness and reliability of deep unconstrained face recognition systems. Our solution achieves state-of-the-art performance, i.e. 95.0% TAR FAR=0.001% on IJB-C dataset and 61.98% Rank1 retrieval rate on the surveillance-to-booking protocol of IJB-S dataset.
Unconstrained Face Recognition
DOWNLOAD
Author : Shaohua Kevin Zhou
language : en
Publisher: Springer Science & Business Media
Release Date : 2006-10-11
Unconstrained Face Recognition written by Shaohua Kevin Zhou and has been published by Springer Science & Business Media this book supported file pdf, txt, epub, kindle and other format this book has been release on 2006-10-11 with Computers categories.
Face recognition has been actively studied over the past decade and continues to be a big research challenge. Just recently, researchers have begun to investigate face recognition under unconstrained conditions. Unconstrained Face Recognition provides a comprehensive review of this biometric, especially face recognition from video, assembling a collection of novel approaches that are able to recognize human faces under various unconstrained situations. The underlying basis of these approaches is that, unlike conventional face recognition algorithms, they exploit the inherent characteristics of the unconstrained situation and thus improve the recognition performance when compared with conventional algorithms. Unconstrained Face Recognition is structured to meet the needs of a professional audience of researchers and practitioners in industry. This volume is also suitable for advanced-level students in computer science.
Texture Based 3d Face Recognition Using Deep Neural Networks For Unconstrained Human Machine Interaction
DOWNLOAD
Author : Michael Danner
language : en
Publisher:
Release Date : 2020
Texture Based 3d Face Recognition Using Deep Neural Networks For Unconstrained Human Machine Interaction written by Michael Danner and has been published by this book supported file pdf, txt, epub, kindle and other format this book has been release on 2020 with categories.
Deep Learning Face Recognition
DOWNLOAD
Author :
language : en
Publisher:
Release Date : 2018
Deep Learning Face Recognition written by and has been published by this book supported file pdf, txt, epub, kindle and other format this book has been release on 2018 with categories.
Learn how to develop a face recognition system by leveraging deep learning. Find out how to code for face detection, identification, and more.
Reliable Face Recognition Methods
DOWNLOAD
Author : Harry Wechsler
language : en
Publisher: Springer Science & Business Media
Release Date : 2009-04-05
Reliable Face Recognition Methods written by Harry Wechsler and has been published by Springer Science & Business Media this book supported file pdf, txt, epub, kindle and other format this book has been release on 2009-04-05 with Computers categories.
This book seeks to comprehensively address the face recognition problem while gaining new insights from complementary fields of endeavor. These include neurosciences, statistics, signal and image processing, computer vision, machine learning and data mining. The book examines the evolution of research surrounding the field to date, explores new directions, and offers specific guidance on the most promising venues for future research and development. The book’s focused approach and its clarity of presentation make this an excellent reference work.
Weakly Supervised Learning For Unconstrained Face Processing
DOWNLOAD
Author : Gary B. Huang
language : en
Publisher:
Release Date : 2012
Weakly Supervised Learning For Unconstrained Face Processing written by Gary B. Huang and has been published by this book supported file pdf, txt, epub, kindle and other format this book has been release on 2012 with Face perception categories.
Machine face recognition has traditionally been studied under the assumption of a carefully controlled image acquisition process. By controlling image acquisition, variation due to factors such as pose, lighting, and background can be either largely eliminated or specifically limited to a study over a discrete number of possibilities. Applications of face recognition have had mixed success when deployed in conditions where the assumption of controlled image acquisition no longer holds. This dissertation focuses on this unconstrained face recognition problem, where face images exhibit the same amount of variability that one would encounter in everyday life. We formalize unconstrained face recognition as a binary pair matching problem (verification), and present a data set for benchmarking performance on the unconstrained face verification task. We observe that it is comparatively much easier to obtain many examples of unlabeled face images than face images that have been labeled with identity or other higher level information, such as the position of the eyes and other facial features. We thus focus on improving unconstrained face verification by leveraging the information present in this source of weakly supervised data. We first show how unlabeled face images can be used to perform unsupervised face alignment, thereby reducing variability in pose and improving verification accuracy. Next, we demonstrate how deep learning can be used to perform unsupervised feature discovery, providing additional image representations that can be combined with representations from standard hand-crafted image descriptors, to further improve recognition performance. Finally, we combine unsupervised feature learning with joint face alignment, leading to an unsupervised alignment system that achieves gains in recognition performance matching that achieved by supervised alignment.
Deep Learning For Computer Vision
DOWNLOAD
Author : Jason Brownlee
language : en
Publisher: Machine Learning Mastery
Release Date : 2019-04-04
Deep Learning For Computer Vision written by Jason Brownlee and has been published by Machine Learning Mastery this book supported file pdf, txt, epub, kindle and other format this book has been release on 2019-04-04 with Computers categories.
Step-by-step tutorials on deep learning neural networks for computer vision in python with Keras.
Scale Aware Multi Path Deep Neural Networks For Unconstrained Face Detection
DOWNLOAD
Author : Yuguang Liu
language : en
Publisher:
Release Date : 2017
Scale Aware Multi Path Deep Neural Networks For Unconstrained Face Detection written by Yuguang Liu and has been published by this book supported file pdf, txt, epub, kindle and other format this book has been release on 2017 with categories.
"Unconstrained face detection is the task of robustly finding and locating faces in an image subject to possible variations in facial scale, blur, pose, illumination, occlusion, and facial expression. It is a critical first step towards a host of modern surveillance applications, including but not limited to face verification, face recognition, face tracking, and human-computer interaction. Though much progress has been made in unconstrained face detection during the past decade, the majority of work focuses on improving the detection robustness on variations caused by blur, pose, illumination, occlusion and facial expression. Facial scale, despite its immense influence on face detection accuracy, has received much less attention than have the above factors. This is partially due to the fact that most traditional face detection benchmark datasets tend to collect faces of relatively large size and with modest scale variation. Nonetheless, in real-world applications, such as surveillance systems, it is imperative to possess an equal ability to detect both big faces (close to camera) and tiny ones (far away from the camera) at the same time. To the best of our knowledge, no published face detection algorithm can detect a face as large as 1000 x 1000 pixels while simultaneously detecting another one as small as 10 x 10 pixels within a single image with similarly high accuracy.We introduce a Multi-Path Face Detection Network (MP-FDN) to filter an image for simultaneously proposing and verifying different sized faces in parallel paths. This is the first time that faces across a large span of scales are detected by a single network with forked detection paths. More importantly, the division of the paths are not handcrafted, but totally based on the scale sensitivity inherent in the convolutional networks that was also discovered in this thesis for the first time. MP-FDN consists of two stages. The first stage is a Multi-Path Face Proposal Network (MP-FPN) that suggests faces at three different scale ranges. This design is based on our observation that the hierarchical multi-scale layers of deep convolutional networks (ConvNet) can inherently represent face patterns at multiple scales. In particular, low-level ConvNet layers are more sensitive to tiny faces, while high-level ConvNet layers are more discriminative to big faces. To this end, MP-FPN utilizes three parallel outputs of the convolutional feature maps to simultaneously predict small, medium and large candidate face regions, respectively. The second stage is a Multi-Path Face Verification Network (MP-FVN) that further eliminates false positives while including false negatives. MP-FVN utilizes the same three parallel paths as MP-FPN. For each detection path, it pools features from both a face candidate region (provided by MP-FPN) and a larger contextual region (surrounding the face candidate region). These facial and contextual features are then concatenated to provide a more accurate "faceness" probability to the face candidate. Note that the network structure and hyper-parameters of MP-FPN and MP-FVN are completely based on controlled experiments, rather than being "handcrafted". To testify to the performance of MP-FDN on the basis its ability to perform face detection, we conducted comprehensive experiments on two challenging public face detection benchmark datasets: WIDER FACE and FDDB datasets. MP-FDN consistently achieves better than the state-of-the-art performance on both of them. Specifically, on the most challenging so-called "hard partition" of WIDER FACE test set that contains faces as small as about 9 pixels and as large as more than 1000 pixels in height, MP-FDN outperforms the former best result by 9.8% for the Average Precision. This demonstrates that MP-FDN is a viable and accurate face detector for unconstrained face detection, especially in the case of large scale variations." --