Development Of Deep Learning Methods For Magnetic Resonance Imaging Reconstruction And Analysis
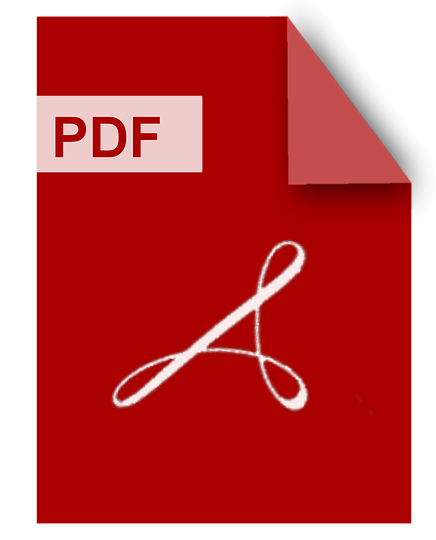
DOWNLOAD
Download Development Of Deep Learning Methods For Magnetic Resonance Imaging Reconstruction And Analysis PDF/ePub or read online books in Mobi eBooks. Click Download or Read Online button to get Development Of Deep Learning Methods For Magnetic Resonance Imaging Reconstruction And Analysis book now. This website allows unlimited access to, at the time of writing, more than 1.5 million titles, including hundreds of thousands of titles in various foreign languages. If the content not found or just blank you must refresh this page
Development Of Deep Learning Methods For Magnetic Resonance Imaging Reconstruction And Analysis
DOWNLOAD
Author : Yuhua Chen
language : en
Publisher:
Release Date : 2021
Development Of Deep Learning Methods For Magnetic Resonance Imaging Reconstruction And Analysis written by Yuhua Chen and has been published by this book supported file pdf, txt, epub, kindle and other format this book has been release on 2021 with categories.
Magnetic resonance image (MRI) is a widely used non-invasive radiation-free imaging technique that uniquely provides structural and functional information for disease detection, diagnosis, and treatment planning. However, the conventional MRI imaging techniques are typically slow and low in spatial or time resolution, resulting in long scan times and more susceptibility to motion artifacts. Moreover, a fast MRI scan usually comes in a low spatial resolution, making it less desirable for clinical application. A recently proposed technique, Multi-tasking MRI (MTMRI), significantly improves the scan efficiency with high temporal resolution. Nevertheless, the iterative reconstruction requires a lot of computational resources and takes a long time to process, making it challenging to fit in the clinical routine. Additionally, when doing image post-processing with MRI, despite MRI providing a good contrast of soft tissues, the variety in weighted contrast MRI's intensity values makes it challenging to extract image features compared with other quantitative imaging techniques. The most significant contribution of this dissertation's work is to address the three limitations above by developing a unified multi-purpose structure with deep-learning (DL) techniques. We achieved three primary goals in three different areas: 1) A general framework for highly accelerated MRI scanning without sacrificing spatial resolution, 2) reduce reconstruction time for motion-resolved free-breathing MRI technique, 3) accurately fully automated segmentation for abdominal MRI for fast image post-processing. All technical improvements utilize DL techniques to improve MRI in different aspects: to improve image quality in fast MRI scans, reduce reconstruction time in motion-resolved MRI, and reduce tedious human labors in abdominal MRI. First, a DL-based Super-Resolution (SR) technique is developed and evaluated in both brain MRI and coronary MR Angiography (MRA). SR can recover the image quality and structural details from a 4x and 16x low-resolution fast MRI scan. For brain MRI, several SR networks have been developed. The proposed network (mDCSRN) has successfully recovered the brain structural details from a 4x low-resolution fast scan. It is developed and evaluated on an open access high-resolution T1w brain MRI with 1131 healthy volunteers. Quantitative results show that it can achieve 4x acceleration in scan while keeping similar image quality. For coronary MRA, introducing a domain adaptive network (DRAGAN) jointly trained on both coronary and brain MRA to overcome catastrophic failures commonly in training a GAN in a small dataset, we successfully accelerated the MRA acquisition by a factor of 16. Second, DL networks are developed to accelerate the reconstruction of a 5-dimensional (5D) Multitasking MRI (MTMRI). The MTMRI is a respiratory and cardiac-motion-resolved, high-temporal-resolution technique that provides quantitative T1 mapping. However, the massive size of many dynamic MRI problems prevents deep learning networks from directly exploiting global temporal relationships. By applying deep neural networks inside a priori calculated temporal feature spaces, we enable deep learning reconstruction with global temporal modeling even for image sequences with >40,000 frames. One proposed variation of our approach using dilated multi-level Densely Connected Network (mDCN) speeds up feature space coordinate calculation by 3000x compared to conventional iterative methods, from 20 minutes to 0.39 seconds. Thus, the combination of low-rank tensor and deep learning models makes large-scale dynamic MRI feasible and practical for routine clinical application. Third, we developed Automated deep Learning-based Abdominal Multi-Organ segmentation (ALAMO) technique based on 2D U-net and a densely connected network structure with tailored design in data augmentation and training procedures. The model takes in multi-slice MR images and generates the output of segmentation results. 3.0-Tesla T1 VIBE (Volumetric Interpolated Breath-hold Examination) images of 102 subjects were used in our study. Ten OARs were studied, including the liver, spleen, pancreas, left/right kidneys, stomach, duodenum, small intestine, spinal cord, and vertebral bodies. ALAMO generated segmentation labels in good agreement with the manual results. Specifically, among the 10 OARs, 9 achieved high Dice Similarity Coefficients (DSCs) in the range of 0.87-0.96, except for the duodenum with a DSC of 0.80. Overall, the ALAMO model matched the state-of-the-art techniques in performance.
Machine Learning In Mri
DOWNLOAD
Author : Ing Thomas Kuestner
language : en
Publisher: Academic Press
Release Date : 2025-09-01
Machine Learning In Mri written by Ing Thomas Kuestner and has been published by Academic Press this book supported file pdf, txt, epub, kindle and other format this book has been release on 2025-09-01 with Science categories.
Machine Learning in MRI: From Methods to Clinical Translation presents state-of-the-art machine learning methods in magnetic resonance imaging that can shape and impact the future of patient treatment and planning. Common methods and strategies along the processing chain of data acquisition, image reconstruction, image post-processing and image analysis of these imaging modalities are presented and illustrated. The book focuses on applications and anatomies for which machine learning methods can bring, or have already brought, important contributions. Ideas and concepts of how processing could be harmonized and used to provide deployable frameworks that integrate into the clinical workflows are considered. Pitfalls and current limitations are discussed in the context of how they could be overcome to cater for clinical needs. Machine Learning in MRI: From Methods to Clinical Translation is an ideal reference for medical imaging researchers, industry scientists and engineers, advanced undergraduate and graduate students, and clinicians. By giving an interdisciplinary presentation and discussion on the obstacles and possible solutions for the clinical translation of machine learning methods, this book enables the evolution of machine learning in medical imaging for the next decade.
Machine Learning For Medical Image Reconstruction
DOWNLOAD
Author : Nandinee Haq
language : en
Publisher: Springer Nature
Release Date : 2021-09-29
Machine Learning For Medical Image Reconstruction written by Nandinee Haq and has been published by Springer Nature this book supported file pdf, txt, epub, kindle and other format this book has been release on 2021-09-29 with Computers categories.
This book constitutes the refereed proceedings of the 4th International Workshop on Machine Learning for Medical Reconstruction, MLMIR 2021, held in conjunction with MICCAI 2021, in October 2021. The workshop was planned to take place in Strasbourg, France, but was held virtually due to the COVID-19 pandemic. The 13 papers presented were carefully reviewed and selected from 20 submissions. The papers are organized in the following topical sections: deep learning for magnetic resonance imaging and deep learning for general image reconstruction.
Machine Learning And Medical Imaging
DOWNLOAD
Author : Guorong Wu
language : en
Publisher: Academic Press
Release Date : 2016-08-11
Machine Learning And Medical Imaging written by Guorong Wu and has been published by Academic Press this book supported file pdf, txt, epub, kindle and other format this book has been release on 2016-08-11 with Computers categories.
Machine Learning and Medical Imaging presents state-of- the-art machine learning methods in medical image analysis. It first summarizes cutting-edge machine learning algorithms in medical imaging, including not only classical probabilistic modeling and learning methods, but also recent breakthroughs in deep learning, sparse representation/coding, and big data hashing. In the second part leading research groups around the world present a wide spectrum of machine learning methods with application to different medical imaging modalities, clinical domains, and organs. The biomedical imaging modalities include ultrasound, magnetic resonance imaging (MRI), computed tomography (CT), histology, and microscopy images. The targeted organs span the lung, liver, brain, and prostate, while there is also a treatment of examining genetic associations. Machine Learning and Medical Imaging is an ideal reference for medical imaging researchers, industry scientists and engineers, advanced undergraduate and graduate students, and clinicians. Demonstrates the application of cutting-edge machine learning techniques to medical imaging problems Covers an array of medical imaging applications including computer assisted diagnosis, image guided radiation therapy, landmark detection, imaging genomics, and brain connectomics Features self-contained chapters with a thorough literature review Assesses the development of future machine learning techniques and the further application of existing techniques
Deep Learning For Medical Image Analysis
DOWNLOAD
Author : S. Kevin Zhou
language : en
Publisher: Academic Press
Release Date : 2017-01-18
Deep Learning For Medical Image Analysis written by S. Kevin Zhou and has been published by Academic Press this book supported file pdf, txt, epub, kindle and other format this book has been release on 2017-01-18 with Computers categories.
Deep learning is providing exciting solutions for medical image analysis problems and is seen as a key method for future applications. This book gives a clear understanding of the principles and methods of neural network and deep learning concepts, showing how the algorithms that integrate deep learning as a core component have been applied to medical image detection, segmentation and registration, and computer-aided analysis, using a wide variety of application areas. Deep Learning for Medical Image Analysis is a great learning resource for academic and industry researchers in medical imaging analysis, and for graduate students taking courses on machine learning and deep learning for computer vision and medical image computing and analysis. Covers common research problems in medical image analysis and their challenges Describes deep learning methods and the theories behind approaches for medical image analysis Teaches how algorithms are applied to a broad range of application areas, including Chest X-ray, breast CAD, lung and chest, microscopy and pathology, etc. Includes a Foreword written by Nicholas Ayache
Magnetic Resonance Image Reconstruction
DOWNLOAD
Author : Mehmet Akcakaya
language : en
Publisher: Academic Press
Release Date : 2022-11-04
Magnetic Resonance Image Reconstruction written by Mehmet Akcakaya and has been published by Academic Press this book supported file pdf, txt, epub, kindle and other format this book has been release on 2022-11-04 with Science categories.
Magnetic Resonance Image Reconstruction: Theory, Methods and Applications presents the fundamental concepts of MR image reconstruction, including its formulation as an inverse problem, as well as the most common models and optimization methods for reconstructing MR images. The book discusses approaches for specific applications such as non-Cartesian imaging, under sampled reconstruction, motion correction, dynamic imaging and quantitative MRI. This unique resource is suitable for physicists, engineers, technologists and clinicians with an interest in medical image reconstruction and MRI. Explains the underlying principles of MRI reconstruction, along with the latest research“/li> Gives example codes for some of the methods presented Includes updates on the latest developments, including compressed sensing, tensor-based reconstruction and machine learning based reconstruction
Applying Machine Learning And Deep Learning For Improved Acquisition Reconstruction And Quantification In Mri
DOWNLOAD
Author : Enhao Gong
language : en
Publisher:
Release Date : 2018
Applying Machine Learning And Deep Learning For Improved Acquisition Reconstruction And Quantification In Mri written by Enhao Gong and has been published by this book supported file pdf, txt, epub, kindle and other format this book has been release on 2018 with categories.
Magnetic Resonance Imaging, MRI, is a powerful imaging modality that is frequently used in both clinical and academic settings. With its advantages of flexibility in signal encoding, we can use MRI to non-invasively visualize various soft-tissue contrasts, showing not only anatomical but also metabolic and functional information. In addition, MRI is a radiation-free modality which makes it favorable in numbers of clinical applications because of the reduced radiation-risk compared with other radiology modalities such as X-ray, Computed Tomography (CT), Positron Emission Tomography (PET) etc. Despite the advantages of MRI techniques, there are still several challenges preventing MRI from becoming more efficient and accessible. First, the scan time for MRI is usually longer than other modalities such as X-ray and CT, since it requires enough measurements to resolve high-quality images for diagnostic tasks. In order to accelerate MRI, various fast-imaging techniques, such as Parallel Imaging (PI) and Compressed Sensing (CS) have been proposed to speed up MRI acquisition using under-sampling. However, it is still unclear what is the best approach to conduct the under-sampling as different under-sampling patterns may result in different reconstruction quality. Second, the reconstruction methods for under-sampled MRI need further improvement. The reconstruction algorithms are formed as nonlinear optimization problems using iterative optimization that can be time-consuming. Fixed and handcrafted penalty terms are usually used to regularize the optimization, which are hard to tune. There are often trade-offs between the speed of the algorithm and the quality of resulting images. In many cases, the imperfect artifact suppression or over-smoothing slows down the clinical adoption of these fast-imaging techniques. Third, MR images are typically not quantitative. Most clinical MRI protocols used nowadays are contrast-weighted sequences, which incorporate the tissue contrasts in qualitative ways. Therefore, the resulting MR images may vary a lot between different protocols and scanners, which makes it very difficult for radiologists to conduct quantitative analysis or longitudinal comparison. In this work, we propose to resolve these remaining challenges to further improve MRI technologies. We utilized state-of-the-art Machine Learning and Deep Learning algorithms to significantly improve these three essential components in MRI: faster acquisition, better reconstruction, and more accurate qualification. Specifically, we firstly propose a machine learning based method to optimize the undersampling pattern for accelerated acquisition. The results, validated on in-vivo multi-contrast brain and prostate MRI datasets, demonstrate that the proposed method can generalize well for different anatomy. It enables efficient (5sec-10sec) and adaptive under-sampling pattern optimization at per-subject/per-scan level, and achieves 30%-50% lower PI+CS reconstruction error at the same acceleration factor. To improve MRI acquisition with a safer protocol and lower contrast dose, a deep learning model is developed to enhance the MRI. The proposed Deep Learning method yielded significant (N=50, p 0.001) improvements over the low-dose (10%) images ( 5dB PSNR gains and > 11.0% SSIM). Ratings on image quality and contrast enhancement are significantly (N=20, p
Machine Learning For Medical Image Reconstruction
DOWNLOAD
Author : Florian Knoll
language : en
Publisher: Springer Nature
Release Date : 2019-10-24
Machine Learning For Medical Image Reconstruction written by Florian Knoll and has been published by Springer Nature this book supported file pdf, txt, epub, kindle and other format this book has been release on 2019-10-24 with Computers categories.
This book constitutes the refereed proceedings of the Second International Workshop on Machine Learning for Medical Reconstruction, MLMIR 2019, held in conjunction with MICCAI 2019, in Shenzhen, China, in October 2019. The 24 full papers presented were carefully reviewed and selected from 32 submissions. The papers are organized in the following topical sections: deep learning for magnetic resonance imaging; deep learning for computed tomography; and deep learning for general image reconstruction.
Machine Learning For Medical Image Reconstruction
DOWNLOAD
Author : Florian Knoll
language : en
Publisher: Springer
Release Date : 2018-09-11
Machine Learning For Medical Image Reconstruction written by Florian Knoll and has been published by Springer this book supported file pdf, txt, epub, kindle and other format this book has been release on 2018-09-11 with Computers categories.
This book constitutes the refereed proceedings of the First International Workshop on Machine Learning for Medical Reconstruction, MLMIR 2018, held in conjunction with MICCAI 2018, in Granada, Spain, in September 2018. The 17 full papers presented were carefully reviewed and selected from 21 submissions. The papers are organized in the following topical sections: deep learning for magnetic resonance imaging; deep learning for computed tomography, and deep learning for general image reconstruction.
Medical Image Reconstruction
DOWNLOAD
Author : Gengsheng Lawrence Zeng
language : en
Publisher: Walter de Gruyter GmbH & Co KG
Release Date : 2023-07-04
Medical Image Reconstruction written by Gengsheng Lawrence Zeng and has been published by Walter de Gruyter GmbH & Co KG this book supported file pdf, txt, epub, kindle and other format this book has been release on 2023-07-04 with Science categories.
This textbook introduces the essential concepts of tomography in the field of medical imaging. The medical imaging modalities include x-ray CT (computed tomography), PET (positron emission tomography), SPECT (single photon emission tomography) and MRI. In these modalities, the measurements are not in the image domain and the conversion from the measurements to the images is referred to as the image reconstruction. The work covers various image reconstruction methods, ranging from the classic analytical inversion methods to the optimization-based iterative image reconstruction methods. As machine learning methods have lately exhibited astonishing potentials in various areas including medical imaging the author devotes one chapter to applications of machine learning in image reconstruction. Based on college level in mathematics, physics, and engineering the textbook supports students in understanding the concepts. It is an essential reference for graduate students and engineers with electrical engineering and biomedical background due to its didactical structure and the balanced combination of methodologies and applications,