Domain Adaptation On Personalized Neural Netowrk Based Language Models
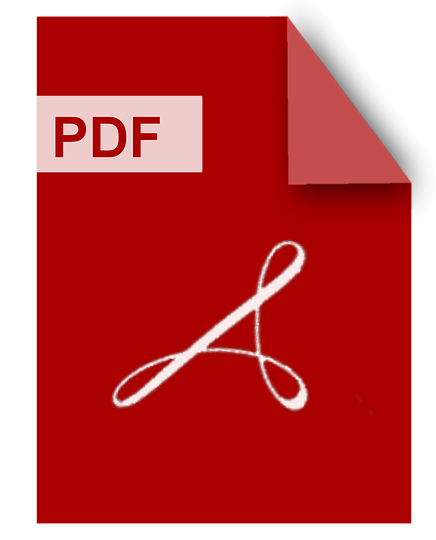
DOWNLOAD
Download Domain Adaptation On Personalized Neural Netowrk Based Language Models PDF/ePub or read online books in Mobi eBooks. Click Download or Read Online button to get Domain Adaptation On Personalized Neural Netowrk Based Language Models book now. This website allows unlimited access to, at the time of writing, more than 1.5 million titles, including hundreds of thousands of titles in various foreign languages. If the content not found or just blank you must refresh this page
Transfer Learning For Natural Language Processing
DOWNLOAD
Author : Paul Azunre
language : en
Publisher: Simon and Schuster
Release Date : 2021-08-31
Transfer Learning For Natural Language Processing written by Paul Azunre and has been published by Simon and Schuster this book supported file pdf, txt, epub, kindle and other format this book has been release on 2021-08-31 with Computers categories.
Build custom NLP models in record time by adapting pre-trained machine learning models to solve specialized problems. Summary In Transfer Learning for Natural Language Processing you will learn: Fine tuning pretrained models with new domain data Picking the right model to reduce resource usage Transfer learning for neural network architectures Generating text with generative pretrained transformers Cross-lingual transfer learning with BERT Foundations for exploring NLP academic literature Training deep learning NLP models from scratch is costly, time-consuming, and requires massive amounts of data. In Transfer Learning for Natural Language Processing, DARPA researcher Paul Azunre reveals cutting-edge transfer learning techniques that apply customizable pretrained models to your own NLP architectures. You’ll learn how to use transfer learning to deliver state-of-the-art results for language comprehension, even when working with limited label data. Best of all, you’ll save on training time and computational costs. Purchase of the print book includes a free eBook in PDF, Kindle, and ePub formats from Manning Publications. About the technology Build custom NLP models in record time, even with limited datasets! Transfer learning is a machine learning technique for adapting pretrained machine learning models to solve specialized problems. This powerful approach has revolutionized natural language processing, driving improvements in machine translation, business analytics, and natural language generation. About the book Transfer Learning for Natural Language Processing teaches you to create powerful NLP solutions quickly by building on existing pretrained models. This instantly useful book provides crystal-clear explanations of the concepts you need to grok transfer learning along with hands-on examples so you can practice your new skills immediately. As you go, you’ll apply state-of-the-art transfer learning methods to create a spam email classifier, a fact checker, and more real-world applications. What's inside Fine tuning pretrained models with new domain data Picking the right model to reduce resource use Transfer learning for neural network architectures Generating text with pretrained transformers About the reader For machine learning engineers and data scientists with some experience in NLP. About the author Paul Azunre holds a PhD in Computer Science from MIT and has served as a Principal Investigator on several DARPA research programs. Table of Contents PART 1 INTRODUCTION AND OVERVIEW 1 What is transfer learning? 2 Getting started with baselines: Data preprocessing 3 Getting started with baselines: Benchmarking and optimization PART 2 SHALLOW TRANSFER LEARNING AND DEEP TRANSFER LEARNING WITH RECURRENT NEURAL NETWORKS (RNNS) 4 Shallow transfer learning for NLP 5 Preprocessing data for recurrent neural network deep transfer learning experiments 6 Deep transfer learning for NLP with recurrent neural networks PART 3 DEEP TRANSFER LEARNING WITH TRANSFORMERS AND ADAPTATION STRATEGIES 7 Deep transfer learning for NLP with the transformer and GPT 8 Deep transfer learning for NLP with BERT and multilingual BERT 9 ULMFiT and knowledge distillation adaptation strategies 10 ALBERT, adapters, and multitask adaptation strategies 11 Conclusions
Generalized Domain Adaptation For Sequence Labeling In Natural Language Processing
DOWNLOAD
Author : Min Xiao
language : en
Publisher:
Release Date : 2016
Generalized Domain Adaptation For Sequence Labeling In Natural Language Processing written by Min Xiao and has been published by this book supported file pdf, txt, epub, kindle and other format this book has been release on 2016 with categories.
Sequence labeling tasks have been widely studied in the natural language processing area, such as part-of-speech tagging, syntactic chunking, dependency parsing, and etc. Most of those systems are developed on a large amount of labeled training data via supervised learning. However, manually collecting labeled training data is too time-consuming and expensive. As an alternative, to alleviate the issue of label scarcity, domain adaptation has recently been proposed to train a statistical machine learning model in a target domain where there is no enough labeled training data by exploiting existing free labeled training data in a different but related source domain. The natural language processing community has witnessed the success of domain adaptation in a variety of sequence labeling tasks. Though the labeled training data in the source domain are available and free, however, they are not exactly as and can be very different from the test data in the target domain. Thus, simply applying naive supervised machine learning algorithms without considering domain differences may not fulfill the purpose. In this dissertation, we developed several novel representation learning approaches to address domain adaptation for sequence labeling in natural language processing. Those representation learning techniques aim to induce latent generalizable features to bridge domain divergence to enable cross-domain prediction. We first tackle a semi-supervised domain adaptation scenario where the target domain has a small amount of labeled training data and propose a distributed representation learning approach based on a probabilistic neural language model. We then relax the assumption of the availability of labeled training data in the target domain and study an unsupervised domain adaptation scenario where the target domain has only unlabeled training data, and give a task-informative representation learning approach based on dynamic dependency networks. Both works are developed in the setting where different domains contain sentences in different genres. We then extend and generalize domain adaptation into a more challenging scenario where different domains contain sentences in different languages and propose two cross-lingual representation learning approaches, one is based on deep neural networks with auxiliary bilingual word pairs and the other is based on annotation projection with auxiliary parallel sentences. All four specific learning scenarios are extensively evaluated with different sequence labeling tasks. The empirical results demonstrate the effectiveness of those generalized domain adaptation techniques for sequence labeling in natural language processing.
Transformers For Natural Language Processing
DOWNLOAD
Author : Denis Rothman
language : en
Publisher: Packt Publishing Ltd
Release Date : 2021-01-29
Transformers For Natural Language Processing written by Denis Rothman and has been published by Packt Publishing Ltd this book supported file pdf, txt, epub, kindle and other format this book has been release on 2021-01-29 with Computers categories.
Publisher's Note: A new edition of this book is out now that includes working with GPT-3 and comparing the results with other models. It includes even more use cases, such as casual language analysis and computer vision tasks, as well as an introduction to OpenAI's Codex. Key FeaturesBuild and implement state-of-the-art language models, such as the original Transformer, BERT, T5, and GPT-2, using concepts that outperform classical deep learning modelsGo through hands-on applications in Python using Google Colaboratory Notebooks with nothing to install on a local machineTest transformer models on advanced use casesBook Description The transformer architecture has proved to be revolutionary in outperforming the classical RNN and CNN models in use today. With an apply-as-you-learn approach, Transformers for Natural Language Processing investigates in vast detail the deep learning for machine translations, speech-to-text, text-to-speech, language modeling, question answering, and many more NLP domains with transformers. The book takes you through NLP with Python and examines various eminent models and datasets within the transformer architecture created by pioneers such as Google, Facebook, Microsoft, OpenAI, and Hugging Face. The book trains you in three stages. The first stage introduces you to transformer architectures, starting with the original transformer, before moving on to RoBERTa, BERT, and DistilBERT models. You will discover training methods for smaller transformers that can outperform GPT-3 in some cases. In the second stage, you will apply transformers for Natural Language Understanding (NLU) and Natural Language Generation (NLG). Finally, the third stage will help you grasp advanced language understanding techniques such as optimizing social network datasets and fake news identification. By the end of this NLP book, you will understand transformers from a cognitive science perspective and be proficient in applying pretrained transformer models by tech giants to various datasets. What you will learnUse the latest pretrained transformer modelsGrasp the workings of the original Transformer, GPT-2, BERT, T5, and other transformer modelsCreate language understanding Python programs using concepts that outperform classical deep learning modelsUse a variety of NLP platforms, including Hugging Face, Trax, and AllenNLPApply Python, TensorFlow, and Keras programs to sentiment analysis, text summarization, speech recognition, machine translations, and moreMeasure the productivity of key transformers to define their scope, potential, and limits in productionWho this book is for Since the book does not teach basic programming, you must be familiar with neural networks, Python, PyTorch, and TensorFlow in order to learn their implementation with Transformers. Readers who can benefit the most from this book include experienced deep learning & NLP practitioners and data analysts & data scientists who want to process the increasing amounts of language-driven data.
Neural Network Methods For Natural Language Processing
DOWNLOAD
Author : Yoav Goldberg
language : en
Publisher: Springer Nature
Release Date : 2022-06-01
Neural Network Methods For Natural Language Processing written by Yoav Goldberg and has been published by Springer Nature this book supported file pdf, txt, epub, kindle and other format this book has been release on 2022-06-01 with Computers categories.
Neural networks are a family of powerful machine learning models. This book focuses on the application of neural network models to natural language data. The first half of the book (Parts I and II) covers the basics of supervised machine learning and feed-forward neural networks, the basics of working with machine learning over language data, and the use of vector-based rather than symbolic representations for words. It also covers the computation-graph abstraction, which allows to easily define and train arbitrary neural networks, and is the basis behind the design of contemporary neural network software libraries. The second part of the book (Parts III and IV) introduces more specialized neural network architectures, including 1D convolutional neural networks, recurrent neural networks, conditioned-generation models, and attention-based models. These architectures and techniques are the driving force behind state-of-the-art algorithms for machine translation, syntactic parsing, and many other applications. Finally, we also discuss tree-shaped networks, structured prediction, and the prospects of multi-task learning.
Neural Network Methods In Natural Language Processing
DOWNLOAD
Author : Yoav Goldberg
language : en
Publisher: Morgan & Claypool Publishers
Release Date : 2017-04-17
Neural Network Methods In Natural Language Processing written by Yoav Goldberg and has been published by Morgan & Claypool Publishers this book supported file pdf, txt, epub, kindle and other format this book has been release on 2017-04-17 with Computers categories.
Neural networks are a family of powerful machine learning models and this book focuses on their application to natural language data. The first half of the book (Parts I and II) covers the basics of supervised machine learning and feed-forward neural networks, the basics of working with machine learning over language data, and the use of vector-based rather than symbolic representations for words. It also covers the computation-graph abstraction, which allows to easily define and train arbitrary neural networks, and is the basis behind the design of contemporary neural network software libraries. The second part of the book (Parts III and IV) introduces more specialized neural network architectures, including 1D convolutional neural networks, recurrent neural networks, conditioned-generation models, and attention-based models. These architectures and techniques are the driving force behind state-of-the-art algorithms for machine translation, syntactic parsing, and many other applications. Finally, we also discuss tree-shaped networks, structured prediction, and the prospects of multi-task learning.
Domain Adaptation In Computer Vision Applications
DOWNLOAD
Author : Gabriela Csurka
language : en
Publisher: Springer
Release Date : 2018-05-17
Domain Adaptation In Computer Vision Applications written by Gabriela Csurka and has been published by Springer this book supported file pdf, txt, epub, kindle and other format this book has been release on 2018-05-17 with Computers categories.
This comprehensive text/reference presents a broad review of diverse domain adaptation (DA) methods for machine learning, with a focus on solutions for visual applications. The book collects together solutions and perspectives proposed by an international selection of pre-eminent experts in the field, addressing not only classical image categorization, but also other computer vision tasks such as detection, segmentation and visual attributes. Topics and features: surveys the complete field of visual DA, including shallow methods designed for homogeneous and heterogeneous data as well as deep architectures; presents a positioning of the dataset bias in the CNN-based feature arena; proposes detailed analyses of popular shallow methods that addresses landmark data selection, kernel embedding, feature alignment, joint feature transformation and classifier adaptation, or the case of limited access to the source data; discusses more recent deep DA methods, including discrepancy-based adaptation networks and adversarial discriminative DA models; addresses domain adaptation problems beyond image categorization, such as a Fisher encoding adaptation for vehicle re-identification, semantic segmentation and detection trained on synthetic images, and domain generalization for semantic part detection; describes a multi-source domain generalization technique for visual attributes and a unifying framework for multi-domain and multi-task learning. This authoritative volume will be of great interest to a broad audience ranging from researchers and practitioners, to students involved in computer vision, pattern recognition and machine learning.
Learning Based Algorithms For Speech Applications Under Domain Mismatch Conditions
DOWNLOAD
Author : Shivesh Ranjan
language : en
Publisher:
Release Date : 2019
Learning Based Algorithms For Speech Applications Under Domain Mismatch Conditions written by Shivesh Ranjan and has been published by this book supported file pdf, txt, epub, kindle and other format this book has been release on 2019 with Authentication categories.
Recent years have experienced a tremendous growth in the use of voice based virtual assistants. Communicating with the digital world via voice is becoming a very easy and pleasant experience, since this mode of interaction is much more naturalistic than text based keyboard entry. To this end, many virtual assistants can now accomplish much more than just providing answers to specific questions. For instance, today’s virtual assistants can now order a taxi just based on her/his voice command, and a person can order food for home delivery through only voice commands using a virtual assistant. A core component in all of these assistants is an effective Automatic Speech Recognition (ASR) engine that can recognize words spoken by humans, and act on it. Another key component of virtual assistants is a Speaker Identification (SID) system based voice authentication phase that only allows the intended user to have access to the device. With the widespread use of commercial speech products across the world, it has become critical to also identify the language of the speaker as a front-end task, since almost all the ASR models currently in use are language specific. To deliver customized content to any particular user, it has also become increasingly important to identify various speaker-specific traits such as gender, age, stress and emotional state among others. Far-field ASR has also received considerable attention recently, since removing the constraint that the subject be in close proximity to the audio capturing microphone allows more natural interaction with the virtual assistant. In this dissertation, we propose novel learning based algorithms to address several critical problems that impact various speech applications. Specifically, we address the issue of robust speaker and gender identification for severely degraded audio. We also propose a deep neural network (DNN) based approach to language identification. Furthermore, we also propose a novel approach to improve the performance of far-field ASR systems. The specific contributions of this dissertation are described next. First, we present an unsupervised domain adaptation based strategy for noise robust gender identification on utterances from the DARPA RATS corpora, where a relative reduction in Equal Error Rate (EER) of +14.75% is achieved using a state-of-the-art i-Vector Probabilistic Linear Discriminant Analysis (PLDA) back-end. Next, we propose a novel 2-step DNN based solution to language identification (LID), where an initial DNN trained for in-set languages is used to create an augmented set with out-of-set examples to train a second DNN that can explicitly detect out-of-set languages. Our proposed approach is shown to offer significant improvements over a baseline system for the NIST 2015 Language Recognition Evaluation i-Vector Machine Learning Challenge, and reduces a NIST defined cost function by up to 32.91% (relative). We also further noise robust speaker identification in this dissertation. To this end, we propose a Curriculum Learning (CL) based approach to noise robust SID that is shown to reduce the EER by up to +20.07% (relative) on severely degraded utterances of the DARPA RATS SID task. Here, we propose to use CL based PLDA estimation, and a CL based i-Vector extractor matrix training. Finally, we improve performance of end-to-end far-field ASR systems by proposing a novel CL based approach using Bidirectional Long Short Term (BLSTM) networks that is shown to reduce the Word Error Rate (WER) by 10.1% (relative) on the AMI corpus. Taken collectively, this investigation has made effective steps towards improving the robustness of voice based interactive systems.
Low Rank Rnn Adaptation For Context Aware Language Modeling
DOWNLOAD
Author : Aaron Jaech
language : en
Publisher:
Release Date : 2018
Low Rank Rnn Adaptation For Context Aware Language Modeling written by Aaron Jaech and has been published by this book supported file pdf, txt, epub, kindle and other format this book has been release on 2018 with categories.
A long-standing weakness of statistical language models is that their performance drastically degrades if they are used on data that varies even slightly from the data on which they were trained. In practice, applications require the use of adaptation methods to adjust the predictions of the model to match the local context. For instance, in a speech recognition application, a single static language model would not be able to handle all the different ways that people speak to their voice assistants such as selecting music and sending a message to a friend. An adapted model would make its predictions conditioned on the knowledge of who is speaking and what task they are trying to do. The current standard approach to recurrent neural network language model adaptation is to apply a simple linear shift to the recurrent and/or output layer bias vector. Although this is helpful, it does not go far enough. This thesis introduces a new approach to adaptation, which we call the FactorCell, that generates a custom recurrent network for each context by applying a low-rank transformation. The FactorCell allows for a more substantial change to the recurrent layer weights. Different from previous approaches, the introduction of a rank hyperparameter gives control over how different or similar the adapted models should be. In our experiments on several different datasets and multiple types of context, the increased adaptation of the recurrent layer is always helpful, as measured by perplexity, the standard for evaluating language models. We also demonstrate impact on two applications: personalized query completion and context-specific text generation, finding that the enhanced adaptation benefits both. We also show that the FactorCell provides a more effective text classification model, but more importantly the classification results reveal that there are important differences between the models that are not captured by perplexity. The classification metric is particularly important for the text generation application.
Deep Learning For Nlp And Speech Recognition
DOWNLOAD
Author : Uday Kamath
language : en
Publisher: Springer
Release Date : 2019-06-24
Deep Learning For Nlp And Speech Recognition written by Uday Kamath and has been published by Springer this book supported file pdf, txt, epub, kindle and other format this book has been release on 2019-06-24 with Computers categories.
This textbook explains Deep Learning Architecture, with applications to various NLP Tasks, including Document Classification, Machine Translation, Language Modeling, and Speech Recognition. With the widespread adoption of deep learning, natural language processing (NLP),and speech applications in many areas (including Finance, Healthcare, and Government) there is a growing need for one comprehensive resource that maps deep learning techniques to NLP and speech and provides insights into using the tools and libraries for real-world applications. Deep Learning for NLP and Speech Recognition explains recent deep learning methods applicable to NLP and speech, provides state-of-the-art approaches, and offers real-world case studies with code to provide hands-on experience. Many books focus on deep learning theory or deep learning for NLP-specific tasks while others are cookbooks for tools and libraries, but the constant flux of new algorithms, tools, frameworks, and libraries in a rapidly evolving landscape means that there are few available texts that offer the material in this book. The book is organized into three parts, aligning to different groups of readers and their expertise. The three parts are: Machine Learning, NLP, and Speech Introduction The first part has three chapters that introduce readers to the fields of NLP, speech recognition, deep learning and machine learning with basic theory and hands-on case studies using Python-based tools and libraries. Deep Learning Basics The five chapters in the second part introduce deep learning and various topics that are crucial for speech and text processing, including word embeddings, convolutional neural networks, recurrent neural networks and speech recognition basics. Theory, practical tips, state-of-the-art methods, experimentations and analysis in using the methods discussed in theory on real-world tasks. Advanced Deep Learning Techniques for Text and Speech The third part has five chapters that discuss the latest and cutting-edge research in the areas of deep learning that intersect with NLP and speech. Topics including attention mechanisms, memory augmented networks, transfer learning, multi-task learning, domain adaptation, reinforcement learning, and end-to-end deep learning for speech recognition are covered using case studies.
Computational Linguistics And Intelligent Text Processing
DOWNLOAD
Author : Alexander Gelbukh
language : en
Publisher: Springer Nature
Release Date : 2023-02-25
Computational Linguistics And Intelligent Text Processing written by Alexander Gelbukh and has been published by Springer Nature this book supported file pdf, txt, epub, kindle and other format this book has been release on 2023-02-25 with Computers categories.
The two-volume set LNCS 13451 and 13452 constitutes revised selected papers from the CICLing 2019 conference which took place in La Rochelle, France, April 2019. The total of 95 papers presented in the two volumes was carefully reviewed and selected from 335 submissions. The book also contains 3 invited papers. The papers are organized in the following topical sections: General, Information extraction, Information retrieval, Language modeling, Lexical resources, Machine translation, Morphology, sintax, parsing, Name entity recognition, Semantics and text similarity, Sentiment analysis, Speech processing, Text categorization, Text generation, and Text mining.