Electric Load Forecasting Using An Artificial Neural Networks
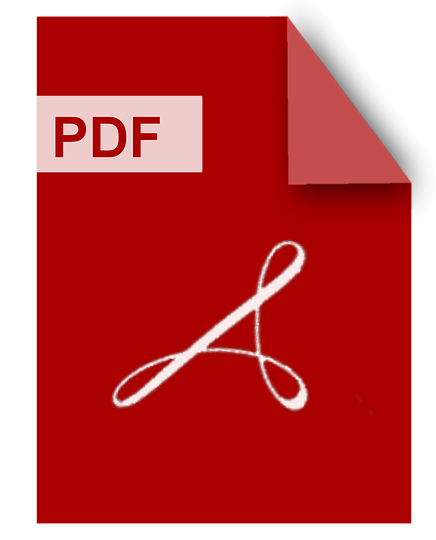
DOWNLOAD
Download Electric Load Forecasting Using An Artificial Neural Networks PDF/ePub or read online books in Mobi eBooks. Click Download or Read Online button to get Electric Load Forecasting Using An Artificial Neural Networks book now. This website allows unlimited access to, at the time of writing, more than 1.5 million titles, including hundreds of thousands of titles in various foreign languages. If the content not found or just blank you must refresh this page
Electric Load Forecasting Using An Artificial Neural Networks
DOWNLOAD
Author : Natalia Gotman
language : en
Publisher: LAP Lambert Academic Publishing
Release Date : 2014-03
Electric Load Forecasting Using An Artificial Neural Networks written by Natalia Gotman and has been published by LAP Lambert Academic Publishing this book supported file pdf, txt, epub, kindle and other format this book has been release on 2014-03 with categories.
Electric load forecasting is an important research field in electric power industry. It plays a crucial role in solving a wide range of tasks of short-term planning and operating control of electric power system operating modes. Load forecasting is carried out in different time spans. Load forecasting within a current day - operating forecasting; one-day-week-month-ahead load forecasting - short-term load forecasting; one-month-quarter-year-ahead load forecasting - long-term load forecasting. So far a great number of both conventional and non-conventional electric load forecasting methods and models have been developed. The work presents research results of electric load forecasting for electrical power systems using artificial neural networks and fuzzy logic as one of the most advanced and perspective directions of solving this task. A theoretical approach to the issues discussed is combined with the data of experimental studies implemented with application of load curves of regional electrical power systems. The book is addressed to specialists and researchers concerned with operational control modes of electric power systems.
Short Term Electric Load Forecasting Using Artificial Neural Networks
DOWNLOAD
Author : Eric Lee Daugherty
language : en
Publisher:
Release Date : 1994
Short Term Electric Load Forecasting Using Artificial Neural Networks written by Eric Lee Daugherty and has been published by this book supported file pdf, txt, epub, kindle and other format this book has been release on 1994 with categories.
Nebraska Electric Load Forecasting Using Artificial Neural Networks With C
DOWNLOAD
Author : Shujun Hou
language : en
Publisher:
Release Date : 1994
Nebraska Electric Load Forecasting Using Artificial Neural Networks With C written by Shujun Hou and has been published by this book supported file pdf, txt, epub, kindle and other format this book has been release on 1994 with Electric power-plants categories.
Electrical Load Forecasting
DOWNLOAD
Author : S.A. Soliman
language : en
Publisher: Elsevier
Release Date : 2010-05-26
Electrical Load Forecasting written by S.A. Soliman and has been published by Elsevier this book supported file pdf, txt, epub, kindle and other format this book has been release on 2010-05-26 with Business & Economics categories.
Succinct and understandable, this book is a step-by-step guide to the mathematics and construction of electrical load forecasting models. Written by one of the world’s foremost experts on the subject, Electrical Load Forecasting provides a brief discussion of algorithms, their advantages and disadvantages and when they are best utilized. The book begins with a good description of the basic theory and models needed to truly understand how the models are prepared so that they are not just blindly plugging and chugging numbers. This is followed by a clear and rigorous exposition of the statistical techniques and algorithms such as regression, neural networks, fuzzy logic, and expert systems. The book is also supported by an online computer program that allows readers to construct, validate, and run short and long term models. Step-by-step guide to model construction Construct, verify, and run short and long term models Accurately evaluate load shape and pricing Creat regional specific electrical load models
Short Term Electric Load Forecasting Using Neural Networks
DOWNLOAD
Author :
language : en
Publisher:
Release Date : 1993
Short Term Electric Load Forecasting Using Neural Networks written by and has been published by this book supported file pdf, txt, epub, kindle and other format this book has been release on 1993 with categories.
Short-term electric load forecasting (STELF) plays an important role in electric utilities, and several techniques are used to perform these predictions and system modelings. Recently, artificial neural networks (ANN's) have been implemented for STELF with some success. This paper will examine improved STELF by optimization of ANN techniques. The strategy for the research involves careful selection of input variables and utilization of effective generalization. Some results have been obtained which show that, with the selection of another input variable, the ANN's use for STELF can be improved.
Forecasting Electricity Load In New Jersey With Artificial Neural Networks
DOWNLOAD
Author : Erik W. Raab
language : en
Publisher:
Release Date : 2022
Forecasting Electricity Load In New Jersey With Artificial Neural Networks written by Erik W. Raab and has been published by this book supported file pdf, txt, epub, kindle and other format this book has been release on 2022 with Electric power-plants categories.
Load forecasting is an important tool for both the energy and environmental sectors. It has progressed hand-in-hand with machine learning innovation, where recurrent neural networks, a type of artificial neural network, is primarily used. This thesis compares progressively complex, feed-forward artificial neural networks using a mix of weather and temporal data. We demonstrate that electrical load in New Jersey can be reliably predicted using memory-less algorithms with minimal predictors drawn from preexisting public data sources. The methods used in this thesis could be used to build competitive load forecasting models in other states, and if included in diverse model ensembles, may generate significant improvements.
Short Term Electrical Load Forecasting For An Institutional Industrial Power System Using An Artificial Neural Network
DOWNLOAD
Author : Eric Lynn Taylor
language : en
Publisher:
Release Date : 2013
Short Term Electrical Load Forecasting For An Institutional Industrial Power System Using An Artificial Neural Network written by Eric Lynn Taylor and has been published by this book supported file pdf, txt, epub, kindle and other format this book has been release on 2013 with categories.
For optimal power system operation, electrical generation must follow electrical load demand. The generation, transmission, and distribution utilities require some means to forecast the electrical load so they can utilize their electrical infrastructure efficiently, securely, and economically. The short-term load forecast (STLF) represents the electric load forecast for a time interval of a few hours to a few days. This thesis will define STLF as a 24-hour-ahead load forecast whose results will provide an hourly electric load forecast in kilowatts (kW) for the future 24 hours (a 24-hour load profile). This thesis will use the method of Artificial Neural Networks (ANN) to create a STLF algorithm for the U.S. Department of Energy’s Oak Ridge National Laboratory (ORNL). ORNL’s power system can be described as an institutional/industrial-type electrical load. The ANN is a mathematical tool that mimics the thought processes of the human brain. The ANN can be created and trained to receive historical load and future weather forecasts as input and produce a load forecast as its output. Most ANNs in the literature are used to forecast the next day 24-hour load profile for a transmission-level system with resulting load forecast errors ranging from approximately 1 % to 3 %. This research will show that an ANN can be used to forecast the smaller, more chaotic load profile of an institutional/industrial-type power system and results in a similar forecast error range. In addition, the operating bounds of the ORNL electric load will be analyzed along with the weather profiles for the site. Correlations between load and weather and load and calendar descriptors, such as day of week and month, will be used as predictor inputs to the ANN to optimize is size and accuracy.
Load Forecasting Using Artificial Neural Network
DOWNLOAD
Author : Pradeepta Kumar Sarangi
language : en
Publisher:
Release Date : 2010
Load Forecasting Using Artificial Neural Network written by Pradeepta Kumar Sarangi and has been published by this book supported file pdf, txt, epub, kindle and other format this book has been release on 2010 with categories.
Short Term Load Forecasting (STLF) is an essential and integral component for energy management and distribution corporations. Accurate load forecasting not only increases the efficiency of a distribution system, but also saves money. STLF is essential in taking quick decisions for a short period such as, for the next few hours or next day or a week. It helps to prevent load shedding and evaluations of various sophisticated financial products on energy pricing. It is important to consider that the forecasting is neither too optimistic nor too conservative because optimistic forecasting creates generation of surplus energy, causing loss of money and resources. Similarly, conservative forecasting will lead to shortage of energy requirement and hence will be a cause for power blackout. This paper examines and analyzes the use of Artificial Neural Network (ANN) as forecasting tools for predicting the future electric load demand and the impact of different numbers of neurons in the hidden layer in a three layered ANN architecture.
Short Term Forecasting Of Electric Loads Using Nonlinear Autoregressive Artificial Neural Networks With Exogenous Vector Inputs
DOWNLOAD
Author :
language : en
Publisher:
Release Date : 2017
Short Term Forecasting Of Electric Loads Using Nonlinear Autoregressive Artificial Neural Networks With Exogenous Vector Inputs written by and has been published by this book supported file pdf, txt, epub, kindle and other format this book has been release on 2017 with categories.
Short-term load forecasting is crucial for the operations planning of an electrical grid. Forecasting the next 24 h of electrical load in a grid allows operators to plan and optimize their resources. The purpose of this study is to develop a more accurate short-term load forecasting method utilizing non-linear autoregressive artificial neural networks (ANN) with exogenous multi-variable input (NARX). The proposed implementation of the network is new: the neural network is trained in open-loop using actual load and weather data, and then, the network is placed in closed-loop to generate a forecast using the predicted load as the feedback input. Unlike the existing short-term load forecasting methods using ANNs, the proposed method uses its own output as the input in order to improve the accuracy, thus effectively implementing a feedback loop for the load, making it less dependent on external data. Using the proposed framework, mean absolute percent errors in the forecast in the order of 1% have been achieved, which is a 30% improvement on the average error using feedforward ANNs, ARMAX and state space methods, which can result in large savings by avoiding commissioning of unnecessary power plants. Finally, the New England electrical load data are used to train and validate the forecast prediction.
Recurrent Neural Networks For Short Term Load Forecasting
DOWNLOAD
Author : Filippo Maria Bianchi
language : en
Publisher: Springer
Release Date : 2017-11-09
Recurrent Neural Networks For Short Term Load Forecasting written by Filippo Maria Bianchi and has been published by Springer this book supported file pdf, txt, epub, kindle and other format this book has been release on 2017-11-09 with Computers categories.
The key component in forecasting demand and consumption of resources in a supply network is an accurate prediction of real-valued time series. Indeed, both service interruptions and resource waste can be reduced with the implementation of an effective forecasting system. Significant research has thus been devoted to the design and development of methodologies for short term load forecasting over the past decades. A class of mathematical models, called Recurrent Neural Networks, are nowadays gaining renewed interest among researchers and they are replacing many practical implementations of the forecasting systems, previously based on static methods. Despite the undeniable expressive power of these architectures, their recurrent nature complicates their understanding and poses challenges in the training procedures. Recently, new important families of recurrent architectures have emerged and their applicability in the context of load forecasting has not been investigated completely yet. This work performs a comparative study on the problem of Short-Term Load Forecast, by using different classes of state-of-the-art Recurrent Neural Networks. The authors test the reviewed models first on controlled synthetic tasks and then on different real datasets, covering important practical cases of study. The text also provides a general overview of the most important architectures and defines guidelines for configuring the recurrent networks to predict real-valued time series.