Ensemble Learning
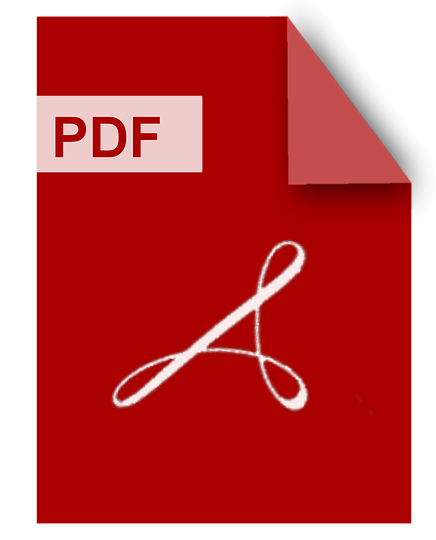
DOWNLOAD
FREE 30 Days
Download Ensemble Learning PDF/ePub or read online books in Mobi eBooks. Click Download or Read Online button to get Ensemble Learning book now. This website allows unlimited access to, at the time of writing, more than 1.5 million titles, including hundreds of thousands of titles in various foreign languages. If the content not found or just blank you must refresh this page
Ensemble Learning Algorithms With Python
DOWNLOAD
FREE 30 Days
Author : Jason Brownlee
language : en
Publisher: Machine Learning Mastery
Release Date : 2021-04-26
Ensemble Learning Algorithms With Python written by Jason Brownlee and has been published by Machine Learning Mastery this book supported file pdf, txt, epub, kindle and other format this book has been release on 2021-04-26 with Computers categories.
Predictive performance is the most important concern on many classification and regression problems. Ensemble learning algorithms combine the predictions from multiple models and are designed to perform better than any contributing ensemble member. Using clear explanations, standard Python libraries, and step-by-step tutorial lessons, you will discover how to confidently and effectively improve predictive modeling performance using ensemble algorithms.
Ensemble Machine Learning
DOWNLOAD
FREE 30 Days
Author : Cha Zhang
language : en
Publisher: Springer Science & Business Media
Release Date : 2012-02-17
Ensemble Machine Learning written by Cha Zhang and has been published by Springer Science & Business Media this book supported file pdf, txt, epub, kindle and other format this book has been release on 2012-02-17 with Computers categories.
It is common wisdom that gathering a variety of views and inputs improves the process of decision making, and, indeed, underpins a democratic society. Dubbed “ensemble learning” by researchers in computational intelligence and machine learning, it is known to improve a decision system’s robustness and accuracy. Now, fresh developments are allowing researchers to unleash the power of ensemble learning in an increasing range of real-world applications. Ensemble learning algorithms such as “boosting” and “random forest” facilitate solutions to key computational issues such as face recognition and are now being applied in areas as diverse as object tracking and bioinformatics. Responding to a shortage of literature dedicated to the topic, this volume offers comprehensive coverage of state-of-the-art ensemble learning techniques, including the random forest skeleton tracking algorithm in the Xbox Kinect sensor, which bypasses the need for game controllers. At once a solid theoretical study and a practical guide, the volume is a windfall for researchers and practitioners alike.
Ensemble Methods
DOWNLOAD
FREE 30 Days
Author : Zhi-Hua Zhou
language : en
Publisher: CRC Press
Release Date : 2012-06-06
Ensemble Methods written by Zhi-Hua Zhou and has been published by CRC Press this book supported file pdf, txt, epub, kindle and other format this book has been release on 2012-06-06 with Business & Economics categories.
An up-to-date, self-contained introduction to a state-of-the-art machine learning approach, Ensemble Methods: Foundations and Algorithms shows how these accurate methods are used in real-world tasks. It gives you the necessary groundwork to carry out further research in this evolving field. After presenting background and terminology, the book covers the main algorithms and theories, including Boosting, Bagging, Random Forest, averaging and voting schemes, the Stacking method, mixture of experts, and diversity measures. It also discusses multiclass extension, noise tolerance, error-ambiguity and bias-variance decompositions, and recent progress in information theoretic diversity. Moving on to more advanced topics, the author explains how to achieve better performance through ensemble pruning and how to generate better clustering results by combining multiple clusterings. In addition, he describes developments of ensemble methods in semi-supervised learning, active learning, cost-sensitive learning, class-imbalance learning, and comprehensibility enhancement.
Ensemble Methods For Machine Learning
DOWNLOAD
FREE 30 Days
Author : Gautam Kunapuli
language : en
Publisher: Simon and Schuster
Release Date : 2023-05-30
Ensemble Methods For Machine Learning written by Gautam Kunapuli and has been published by Simon and Schuster this book supported file pdf, txt, epub, kindle and other format this book has been release on 2023-05-30 with Computers categories.
Ensemble machine learning combines the power of multiple machine learning approaches, working together to deliver models that are highly performant and highly accurate. Inside Ensemble Methods for Machine Learning you will find: Methods for classification, regression, and recommendations Sophisticated off-the-shelf ensemble implementations Random forests, boosting, and gradient boosting Feature engineering and ensemble diversity Interpretability and explainability for ensemble methods Ensemble machine learning trains a diverse group of machine learning models to work together, aggregating their output to deliver richer results than a single model. Now in Ensemble Methods for Machine Learning you’ll discover core ensemble methods that have proven records in both data science competitions and real-world applications. Hands-on case studies show you how each algorithm works in production. By the time you're done, you'll know the benefits, limitations, and practical methods of applying ensemble machine learning to real-world data, and be ready to build more explainable ML systems. About the Technology Automatically compare, contrast, and blend the output from multiple models to squeeze the best results from your data. Ensemble machine learning applies a “wisdom of crowds” method that dodges the inaccuracies and limitations of a single model. By basing responses on multiple perspectives, this innovative approach can deliver robust predictions even without massive datasets. About the Book Ensemble Methods for Machine Learning teaches you practical techniques for applying multiple ML approaches simultaneously. Each chapter contains a unique case study that demonstrates a fully functional ensemble method, with examples including medical diagnosis, sentiment analysis, handwriting classification, and more. There’s no complex math or theory—you’ll learn in a visuals-first manner, with ample code for easy experimentation! What’s Inside Bagging, boosting, and gradient boosting Methods for classification, regression, and retrieval Interpretability and explainability for ensemble methods Feature engineering and ensemble diversity About the Reader For Python programmers with machine learning experience. About the Author Gautam Kunapuli has over 15 years of experience in academia and the machine learning industry. Table of Contents PART 1 - THE BASICS OF ENSEMBLES 1 Ensemble methods: Hype or hallelujah? PART 2 - ESSENTIAL ENSEMBLE METHODS 2 Homogeneous parallel ensembles: Bagging and random forests 3 Heterogeneous parallel ensembles: Combining strong learners 4 Sequential ensembles: Adaptive boosting 5 Sequential ensembles: Gradient boosting 6 Sequential ensembles: Newton boosting PART 3 - ENSEMBLES IN THE WILD: ADAPTING ENSEMBLE METHODS TO YOUR DATA 7 Learning with continuous and count labels 8 Learning with categorical features 9 Explaining your ensembles
Hands On Ensemble Learning With Python
DOWNLOAD
FREE 30 Days
Author : George Kyriakides
language : en
Publisher: Packt Publishing Ltd
Release Date : 2019-07-19
Hands On Ensemble Learning With Python written by George Kyriakides and has been published by Packt Publishing Ltd this book supported file pdf, txt, epub, kindle and other format this book has been release on 2019-07-19 with Computers categories.
Combine popular machine learning techniques to create ensemble models using Python Key FeaturesImplement ensemble models using algorithms such as random forests and AdaBoostApply boosting, bagging, and stacking ensemble methods to improve the prediction accuracy of your model Explore real-world data sets and practical examples coded in scikit-learn and KerasBook Description Ensembling is a technique of combining two or more similar or dissimilar machine learning algorithms to create a model that delivers superior predictive power. This book will demonstrate how you can use a variety of weak algorithms to make a strong predictive model. With its hands-on approach, you'll not only get up to speed on the basic theory but also the application of various ensemble learning techniques. Using examples and real-world datasets, you'll be able to produce better machine learning models to solve supervised learning problems such as classification and regression. Furthermore, you'll go on to leverage ensemble learning techniques such as clustering to produce unsupervised machine learning models. As you progress, the chapters will cover different machine learning algorithms that are widely used in the practical world to make predictions and classifications. You'll even get to grips with the use of Python libraries such as scikit-learn and Keras for implementing different ensemble models. By the end of this book, you will be well-versed in ensemble learning, and have the skills you need to understand which ensemble method is required for which problem, and successfully implement them in real-world scenarios. What you will learnImplement ensemble methods to generate models with high accuracyOvercome challenges such as bias and varianceExplore machine learning algorithms to evaluate model performanceUnderstand how to construct, evaluate, and apply ensemble modelsAnalyze tweets in real time using Twitter's streaming APIUse Keras to build an ensemble of neural networks for the MovieLens datasetWho this book is for This book is for data analysts, data scientists, machine learning engineers and other professionals who are looking to generate advanced models using ensemble techniques. An understanding of Python code and basic knowledge of statistics is required to make the most out of this book.
Hands On Ensemble Learning With R
DOWNLOAD
FREE 30 Days
Author : Prabhanjan Narayanachar Tattar
language : en
Publisher: Packt Publishing Ltd
Release Date : 2018-07-27
Hands On Ensemble Learning With R written by Prabhanjan Narayanachar Tattar and has been published by Packt Publishing Ltd this book supported file pdf, txt, epub, kindle and other format this book has been release on 2018-07-27 with Computers categories.
Explore powerful R packages to create predictive models using ensemble methods Key Features Implement machine learning algorithms to build ensemble-efficient models Explore powerful R packages to create predictive models using ensemble methods Learn to build ensemble models on large datasets using a practical approach Book Description Ensemble techniques are used for combining two or more similar or dissimilar machine learning algorithms to create a stronger model. Such a model delivers superior prediction power and can give your datasets a boost in accuracy. Hands-On Ensemble Learning with R begins with the important statistical resampling methods. You will then walk through the central trilogy of ensemble techniques – bagging, random forest, and boosting – then you'll learn how they can be used to provide greater accuracy on large datasets using popular R packages. You will learn how to combine model predictions using different machine learning algorithms to build ensemble models. In addition to this, you will explore how to improve the performance of your ensemble models. By the end of this book, you will have learned how machine learning algorithms can be combined to reduce common problems and build simple efficient ensemble models with the help of real-world examples. What you will learn Carry out an essential review of re-sampling methods, bootstrap, and jackknife Explore the key ensemble methods: bagging, random forests, and boosting Use multiple algorithms to make strong predictive models Enjoy a comprehensive treatment of boosting methods Supplement methods with statistical tests, such as ROC Walk through data structures in classification, regression, survival, and time series data Use the supplied R code to implement ensemble methods Learn stacking method to combine heterogeneous machine learning models Who this book is for This book is for you if you are a data scientist or machine learning developer who wants to implement machine learning techniques by building ensemble models with the power of R. You will learn how to combine different machine learning algorithms to perform efficient data processing. Basic knowledge of machine learning techniques and programming knowledge of R would be an added advantage.
Ensemble Machine Learning
DOWNLOAD
FREE 30 Days
Author : Cha Zhang
language : en
Publisher: Springer Science & Business Media
Release Date : 2012-02-17
Ensemble Machine Learning written by Cha Zhang and has been published by Springer Science & Business Media this book supported file pdf, txt, epub, kindle and other format this book has been release on 2012-02-17 with Technology & Engineering categories.
It is common wisdom that gathering a variety of views and inputs improves the process of decision making, and, indeed, underpins a democratic society. Dubbed “ensemble learning” by researchers in computational intelligence and machine learning, it is known to improve a decision system’s robustness and accuracy. Now, fresh developments are allowing researchers to unleash the power of ensemble learning in an increasing range of real-world applications. Ensemble learning algorithms such as “boosting” and “random forest” facilitate solutions to key computational issues such as face recognition and are now being applied in areas as diverse as object tracking and bioinformatics. Responding to a shortage of literature dedicated to the topic, this volume offers comprehensive coverage of state-of-the-art ensemble learning techniques, including the random forest skeleton tracking algorithm in the Xbox Kinect sensor, which bypasses the need for game controllers. At once a solid theoretical study and a practical guide, the volume is a windfall for researchers and practitioners alike.
Ensemble Learning Pattern Classification Using Ensemble Methods Second Edition
DOWNLOAD
FREE 30 Days
Author : Lior Rokach
language : en
Publisher: World Scientific
Release Date : 2019-02-27
Ensemble Learning Pattern Classification Using Ensemble Methods Second Edition written by Lior Rokach and has been published by World Scientific this book supported file pdf, txt, epub, kindle and other format this book has been release on 2019-02-27 with Computers categories.
This updated compendium provides a methodical introduction with a coherent and unified repository of ensemble methods, theories, trends, challenges, and applications. More than a third of this edition comprised of new materials, highlighting descriptions of the classic methods, and extensions and novel approaches that have recently been introduced.Along with algorithmic descriptions of each method, the settings in which each method is applicable and the consequences and tradeoffs incurred by using the method is succinctly featured. R code for implementation of the algorithm is also emphasized.The unique volume provides researchers, students and practitioners in industry with a comprehensive, concise and convenient resource on ensemble learning methods.
Ensemble Learning For Ai Developers
DOWNLOAD
FREE 30 Days
Author : Alok Kumar
language : en
Publisher: Apress
Release Date : 2020-06-18
Ensemble Learning For Ai Developers written by Alok Kumar and has been published by Apress this book supported file pdf, txt, epub, kindle and other format this book has been release on 2020-06-18 with Computers categories.
Use ensemble learning techniques and models to improve your machine learning results. Ensemble Learning for AI Developers starts you at the beginning with an historical overview and explains key ensemble techniques and why they are needed. You then will learn how to change training data using bagging, bootstrap aggregating, random forest models, and cross-validation methods. Authors Kumar and Jain provide best practices to guide you in combining models and using tools to boost performance of your machine learning projects. They teach you how to effectively implement ensemble concepts such as stacking and boosting and to utilize popular libraries such as Keras, Scikit Learn, TensorFlow, PyTorch, and Microsoft LightGBM. Tips are presented to apply ensemble learning in different data science problems, including time series data, imaging data, and NLP. Recent advances in ensemble learning are discussed. Sample code is provided in the form of scripts and the IPython notebook. What You Will Learn Understand the techniques and methods utilized in ensemble learningUse bagging, stacking, and boosting to improve performance of your machine learning projects by combining models to decrease variance, improve predictions, and reduce biasEnhance your machine learning architecture with ensemble learning Who This Book Is For Data scientists and machine learning engineers keen on exploring ensemble learning
Ensemble Methods
DOWNLOAD
FREE 30 Days
Author : Zhi-Hua Zhou
language : en
Publisher: CRC Press
Release Date : 2012-06-06
Ensemble Methods written by Zhi-Hua Zhou and has been published by CRC Press this book supported file pdf, txt, epub, kindle and other format this book has been release on 2012-06-06 with Business & Economics categories.
An up-to-date, self-contained introduction to a state-of-the-art machine learning approach, Ensemble Methods: Foundations and Algorithms shows how these accurate methods are used in real-world tasks. It gives you the necessary groundwork to carry out further research in this evolving field.After presenting background and terminology, the book cover