Estimation And Inference For High Dimensional Time Series
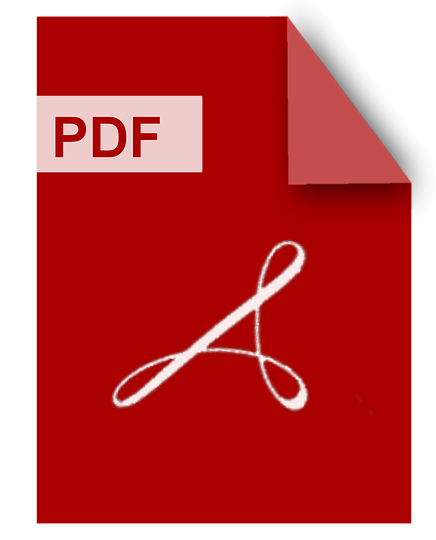
DOWNLOAD
Download Estimation And Inference For High Dimensional Time Series PDF/ePub or read online books in Mobi eBooks. Click Download or Read Online button to get Estimation And Inference For High Dimensional Time Series book now. This website allows unlimited access to, at the time of writing, more than 1.5 million titles, including hundreds of thousands of titles in various foreign languages. If the content not found or just blank you must refresh this page
Estimation And Inference For High Dimensional Time Series
DOWNLOAD
Author : Danna Zhang
language : en
Publisher:
Release Date : 2017
Estimation And Inference For High Dimensional Time Series written by Danna Zhang and has been published by this book supported file pdf, txt, epub, kindle and other format this book has been release on 2017 with categories.
There is a well-developed asymptotic theory for sample means and sample second-order statistics of low dimensional stationary processes. However, many important problems on their asymptotic behaviors are still unanswered for time series which can be high-dimensional, nonstationary and non-Gaussian.
High Dimensional Panel Data With Time Heterogeneity
DOWNLOAD
Author : Yinchu Zhu
language : en
Publisher:
Release Date : 2017
High Dimensional Panel Data With Time Heterogeneity written by Yinchu Zhu and has been published by this book supported file pdf, txt, epub, kindle and other format this book has been release on 2017 with categories.
We consider high-dimensional panel data models (large cross sections and long time horizons) with interactive fixed effects and allow the covariate/slope coefficients to vary over time without any restrictions. The parameter of interest is the vector that contains all the covariate effects across time. This vector has dimensionality tending to infinity, potentially much faster than the cross-sectional sample size. We develop methods for the estimation and inference of this high-dimensional vector, i.e., the entire trajectory of time variation in covariate effects. We show that both the consistency of our estimator and the asymptotic accuracy of the proposed inference procedure hold uniformly in time. Our methodology can be applied to several important issues in econometrics, such as constructing confidence bands for the entire path of covariate coefficients across time, testing the time-invariance of slope coefficients and estimation and inference of patterns of time variations, including structural breaks and regime switching. An important feature of our method is that it provides inference procedures for the time variation in prespecified components of slope coefficients while allowing for arbitrary time variation in other components. Computationally, our procedures do not require any numerical optimization and are very simple to implement. Monte Carlo simulations demonstrate favorable properties of our methods in finite samples. We illustrate our methods through empirical applications in finance and economics.
Essays On Estimation And Inference In High Dimensional Models With Applications To Finance And Economics
DOWNLOAD
Author : Yinchu Zhu
language : en
Publisher:
Release Date : 2017
Essays On Estimation And Inference In High Dimensional Models With Applications To Finance And Economics written by Yinchu Zhu and has been published by this book supported file pdf, txt, epub, kindle and other format this book has been release on 2017 with categories.
Economic modeling in a data-rich environment is often challenging. To allow for enough flexibility and to model heterogeneity, models might have parameters with dimensionality growing with (or even much larger than) the sample size of the data. Learning these high-dimensional parameters requires new methodologies and theories. We consider three important high-dimensional models and propose novel methods for estimation and inference. Empirical applications in economics and finance are also studied. In Chapter 1, we consider high-dimensional panel data models (large cross sections and long time horizons) with interactive fixed effects and allow the covariate/slope coefficients to vary over time without any restrictions. The parameter of interest is the vector that contains all the covariate effects across time. This vector has dimensionality tending to infinity, potentially much faster than the cross-sectional sample size. We develop methods for the estimation and inference of this high-dimensional vector, i.e., the entire trajectory of time variation in covariate effects. We show that both the consistency of our estimator and the asymptotic accuracy of the proposed inference procedure hold uniformly in time. Our methodology can be applied to several important issues in econometrics, such as constructing confidence bands for the entire path of covariate coefficients across time, testing the time-invariance of slope coefficients and estimation and inference of patterns of time variations, including structural breaks and regime switching. An important feature of our method is that it provides inference procedures for the time variation in pre-specified components of slope coefficients while allowing for arbitrary time variation in other components. Computationally, our procedures do not require any numerical optimization and are very simple to implement. Monte Carlo simulations demonstrate favorable properties of our methods in finite samples. We illustrate our methods through empirical applications in finance and economics. In Chapter 2, we consider large factor models with unobserved factors. We formalize the notion of common factors between different groups of variables and propose to use it as a general approach to study the structure of factors, i.e., which factors drive which variables. The spanning hypothesis, which states that factors driving one group are spanned by those driving another group, can be studied as a special case under our framework. We develop a statistical procedure for testing the number of common factors. Our inference procedure is built upon recent results on high-dimensional bootstrap and is shown to be valid under the asymptotic framework of large $n$ and large $T$. In Monte Carlo simulations, our procedure performs well in finite samples. As an empirical application, we construct confidence sets for the number of common factors between the macroeconomy and the financial markets. Chapter 3 is joint work with Jelena Bradic. We propose a methodology for testing linear hypothesis in high-dimensional linear models. The proposed test does not impose any restriction on the size of the model, i.e. model sparsity or the loading vector representing the hypothesis. Providing asymptotically valid methods for testing general linear functions of the regression parameters in high-dimensions is extremely challenging--especially without making restrictive or unverifiable assumptions on the number of non-zero elements. We propose to test the moment conditions related to the newly designed restructured regression, where the inputs are transformed and augmented features. These new features incorporate the structure of the null hypothesis directly. The test statistics are constructed in such a way that lack of sparsity in the original model parameter does not present a problem for the theoretical justification of our procedures. We establish asymptotically exact control on Type I error without imposing any sparsity assumptions on model parameter or the vector representing the linear hypothesis. Our method is also shown to achieve certain optimality in detecting deviations from the null hypothesis. We demonstrate the favorable finite-sample performance of the proposed methods, via a number of numerical and a real data example.
Large Dimensional Panel Data Econometrics Testing Estimation And Structural Changes
DOWNLOAD
Author : Feng Qu
language : en
Publisher: World Scientific
Release Date : 2020-08-24
Large Dimensional Panel Data Econometrics Testing Estimation And Structural Changes written by Feng Qu and has been published by World Scientific this book supported file pdf, txt, epub, kindle and other format this book has been release on 2020-08-24 with Business & Economics categories.
This book aims to fill the gap between panel data econometrics textbooks, and the latest development on 'big data', especially large-dimensional panel data econometrics. It introduces important research questions in large panels, including testing for cross-sectional dependence, estimation of factor-augmented panel data models, structural breaks in panels and group patterns in panels. To tackle these high dimensional issues, some techniques used in Machine Learning approaches are also illustrated. Moreover, the Monte Carlo experiments, and empirical examples are also utilised to show how to implement these new inference methods. Large-Dimensional Panel Data Econometrics: Testing, Estimation and Structural Changes also introduces new research questions and results in recent literature in this field.
The Estimation And Inference Of Complex Models
DOWNLOAD
Author : Min Zhou
language : en
Publisher:
Release Date : 2017
The Estimation And Inference Of Complex Models written by Min Zhou and has been published by this book supported file pdf, txt, epub, kindle and other format this book has been release on 2017 with Electronic books categories.
In this thesis, we investigate the estimation problem and inference problem for the complex models. Two major categories of complex models are emphasized by us, one is generalized linear models, the other is time series models. For the generalized linear models, we consider one fundamental problem about sure screening for interaction terms in ultra-high dimensional feature space; for time series models, an important model assumption about Markov property is considered by us. The first part of this thesis illustrates the significant interaction pursuit problem for ultra-high dimensional models with two-way interaction effects. We propose a simple sure screening procedure (SSI) to detect significant interactions between the explanatory variables and the response variable in the high or ultra-high dimensional generalized linear regression models. Sure screening method is a simple, but powerful tool for the first step of feature selection or variable selection for ultra-high dimensional data. We investigate the sure screening properties of the proposal method from theoretical insight. Furthermore, we indicate that our proposed method can control the false discovery rate at a reasonable size, so the regularized variable selection methods can be easily applied to get more accurate feature selection in the following model selection procedures. Moreover, from the viewpoint of computational efficiency, we suggest a much more efficient algorithm-discretized SSI (DSSI) to realize our proposed sure screening method in practice. And we also investigate the properties of these two algorithms SSI and DSSI in simulation studies and apply them to some real data analyses for illustration. For the second part, our concern is the testing of the Markov property in time series processes. Markovian assumption plays an extremely important role in time series analysis and is also a fundamental assumption in economic and financial models. However, few existing research mainly focused on how to test the Markov properties for the time series processes. Therefore, for the Markovian assumption, we propose a new test procedure to check if the time series with beta-mixing possesses the Markov property. Our test is based on the Conditional Distance Covariance (CDCov). We investigate the theoretical properties of the proposed method. The asymptotic distribution of the proposed test statistic under the null hypothesis is obtained, and the power of the test procedure under local alternative hypothesizes have been studied. Simulation studies are conducted to demonstrate the finite sample performance of our test.
Statistical Inference For Change Points In High Dimensional Offline And Online Data
DOWNLOAD
Author : Lingjun Li
language : en
Publisher:
Release Date : 2020
Statistical Inference For Change Points In High Dimensional Offline And Online Data written by Lingjun Li and has been published by this book supported file pdf, txt, epub, kindle and other format this book has been release on 2020 with Change-point problems categories.
High-dimensional offline and online time series data are characterized by a large number of measurements and complex dependence, and often involve change points. Change point detection in offline time series data improves the parameter testing and estimation by pooling homogeneous observations between two successive change points. Change point detection in online time series data provides timely snapshots of the monitored system and allows for real-time anomaly detection. Despite its importance, methods available for change point detection in high-dimensional offline and online time series data are scarce.In the first part of the thesis, we present some new statistics for change-point testing and estimation in high dimensional offline time series data. We establish their theoretical properties including asymptotic distributions and consistency under mild conditions. The developed new methods are non-parametric without imposing restrictive structural assumptions. They incorporate spatial and temporal dependence in data. Most importantly, they can detect the change point near the boundary of time series data.In the second part of the thesis, we extend these new statistics to high-dimensional online time series data and provide a new stopping rule to detect a change point as early as possible after an anomaly occurs. We study theoretical properties of the new stopping rule, and derive an explicit expression for the average run length (ARL) so that the level of threshold in the stopping rule can be easily obtained with no need to run time-consuming Monte Carlo simulations. We also establish an upper bound for the expected detection delay (EDD), which demonstrates the impact of data dependence and magnitude of structure change in data. Simulation and case studies are provided to demonstrate the empirical performance of the proposed offline and online change-point detection methods.
Factor Modelling For High Dimensional Time Series
DOWNLOAD
Author : Ngai Hang Chan
language : en
Publisher:
Release Date : 2017
Factor Modelling For High Dimensional Time Series written by Ngai Hang Chan and has been published by this book supported file pdf, txt, epub, kindle and other format this book has been release on 2017 with categories.
Analysis of high-dimensional time series data is of increasing interest among different fields. This article studies high-dimensional time series from a dimension reduction perspective using factor modelling. Statistical inference is conducted using eigen-analysis of a certain non-negative definite matrix related to autocovariance matrices of the time series, which is applicable to fixed or increasing dimension. When the dimension goes to infinity, the rate of convergence and limiting distributions of estimated factors are established. Using the limiting distributions of estimated factors, a high-dimensional final prediction error criterion is proposed to select the number of factors. Asymptotic properties of the criterion are illustrated by simulation studies and real applications.
Statistical Methods For Clustering And High Dimensional Time Series Analysis
DOWNLOAD
Author : Kunhui Zhang
language : en
Publisher:
Release Date : 2022
Statistical Methods For Clustering And High Dimensional Time Series Analysis written by Kunhui Zhang and has been published by this book supported file pdf, txt, epub, kindle and other format this book has been release on 2022 with categories.
This dissertation mainly explores two statistical tasks, namely clustering and analysis of high-dimensional time series. Clustering, a very important unsupervised learning problem, studies the structure of unlabeled datasets. The goal of clustering is to partition the data points into subsets such that data points in the same subset are similar and different from those in other subsets. Mode-clustering is a clustering analysis method that partitions the data into groups by the local modes of the underlying density function. Sometimes, finding clusters is not the ultimate goal. The connectivity among clusters may yield valuable information for scientists. This dissertation presents a new clustering method inspired by mode-clustering that not only finds clusters but also assigns each cluster with an attribute label. Clusters obtained from our method show connectivity of the underlying distribution. We also design a local two-sample test based on the clustering result that has more power than a conventional method. We apply our method to the Astronomy and GvHD data and show that our method finds meaningful clusters. In addition, we derive the statistical and computational theory of our method. Motivated by the challenges of modeling time series data sets that exhibit non-linear patterns, especially in high dimensions, this dissertation also considers the threshold Auto-Regressive (TAR) process. The TAR process provides a family of non-linear auto-regressive time series models in which the process dynamics are specific step functions of a thresholding variable. While estimation and inference for low-dimensional TAR models have been investigated, high-dimensional TAR models have received less attention. In this dissertation, we develop a new framework for estimating high-dimensional TAR models and propose two different sparsity-inducing penalties. The first penalty corresponds to a natural extension of the classical TAR model to high-dimensional settings, where the same threshold is enforced for all model parameters. Our second penalty develops a more flexible TAR model, where different thresholds are allowed for different auto-regressive coefficients. We show that both penalized estimation strategies can be utilized in a three-step procedure that consistently learns both the thresholds and the corresponding auto-regressive coefficients. However, our theoretical and empirical investigations show that the direct extension of the TAR model is not appropriate for high-dimensional settings and is better suited for moderate dimensions. In contrast, the more flexible extension of the TAR model leads to consistent estimation and superior empirical performance in high dimensions. In addition to the three-step procedure, the dynamic programming approach can successfully handle high dimensions with diverging number of thresholds as well. In particular, extensive numerical analysis and theoretical results demonstrate the advantages of the dynamic programming approach. Finally, we also discuss a method to select the optimal thresholding variable automatically.
Consistent Causal Inference For High Dimensional Time Series
DOWNLOAD
Author : Francesco Cordoni
language : en
Publisher:
Release Date : 2022
Consistent Causal Inference For High Dimensional Time Series written by Francesco Cordoni and has been published by this book supported file pdf, txt, epub, kindle and other format this book has been release on 2022 with categories.
A methodology for high dimensional causal inference in a time series context is introduced. It is assumed that there is a monotonic transformation of the data such that the dynamics of the transformed variables are described by a Gaussian vector autoregressive process. This is tantamount to assume that the dynamics are captured by a Gaussian copula. No knowledge or estimation of the marginal distribution of the data is required. The procedure consistently identifies the parameters that describe the dynamics of the process and the conditional causal relations among the possibly high dimensional variables under sparsity conditions. The methodology allows us to identify such causal relations in the form of a directed acyclic graph. As an application we estimate the directed acyclic graph for the order book on one-minute aggregated data on four stock constituents of the S&P500.
Inference For Regression Models With Time Series Errors Inverse Autocovariance Matrix Estimation And High Dimensional Model Selection
DOWNLOAD
Author : 邱海唐
language : en
Publisher:
Release Date : 2017
Inference For Regression Models With Time Series Errors Inverse Autocovariance Matrix Estimation And High Dimensional Model Selection written by 邱海唐 and has been published by this book supported file pdf, txt, epub, kindle and other format this book has been release on 2017 with categories.