Exploring Textual Data
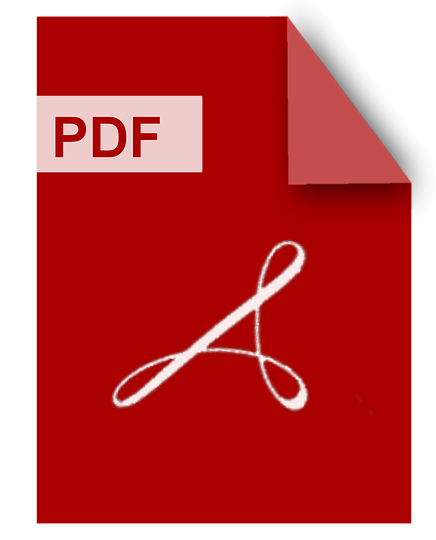
DOWNLOAD
Download Exploring Textual Data PDF/ePub or read online books in Mobi eBooks. Click Download or Read Online button to get Exploring Textual Data book now. This website allows unlimited access to, at the time of writing, more than 1.5 million titles, including hundreds of thousands of titles in various foreign languages. If the content not found or just blank you must refresh this page
Exploring Textual Data
DOWNLOAD
Author : Ludovic Lebart
language : en
Publisher: Springer Science & Business Media
Release Date : 2013-04-17
Exploring Textual Data written by Ludovic Lebart and has been published by Springer Science & Business Media this book supported file pdf, txt, epub, kindle and other format this book has been release on 2013-04-17 with Mathematics categories.
Researchers in a number of disciplines deal with large text sets requiring both text management and text analysis. Faced with a large amount of textual data collected in marketing surveys, literary investigations, historical archives and documentary data bases, these researchers require assistance with organizing, describing and comparing texts. Exploring Textual Data demonstrates how exploratory multivariate statistical methods such as correspondence analysis and cluster analysis can be used to help investigate, assimilate and evaluate textual data. The main text does not contain any strictly mathematical demonstrations, making it accessible to a large audience. This book is very user-friendly with proofs abstracted in the appendices. Full definitions of concepts, implementations of procedures and rules for reading and interpreting results are fully explored. A succession of examples is intended to allow the reader to appreciate the variety of actual and potential applications and the complementary processing methods. A glossary of terms is provided.
Introduction To Text Mining
DOWNLOAD
Author : Gabe Ignatow
language : en
Publisher:
Release Date : 2017
Introduction To Text Mining written by Gabe Ignatow and has been published by this book supported file pdf, txt, epub, kindle and other format this book has been release on 2017 with categories.
Gain a foundational understanding of the analysis of textual data sets from social media sites, digital archives, and digital surveys and interviews through the study of language and social interactions in digital environments. This course is perfect for social scientists who want to gain a conceptual overview of the text mining landscape to take first steps towards working on a text mining project or collaborating with computational colleagues. By taking this course you will: Learn the foundations of Natural Language Processing (NLP) Learn how text mining tools have been used successfully by social scientists Understand basic text processing techniques Understand how to approach narrative analysis, thematic analysis, and metaphor analysis Learn about key computer science methods for text mining, such as text classification and opinion mining.
Data Mining Of Unstructured Textual Information In Transportation Safety Domain
DOWNLOAD
Author : Keneth Morgan Kwayu
language : en
Publisher:
Release Date : 2021
Data Mining Of Unstructured Textual Information In Transportation Safety Domain written by Keneth Morgan Kwayu and has been published by this book supported file pdf, txt, epub, kindle and other format this book has been release on 2021 with Data mining categories.
The unprecedented increase in volume and influx of structured and unstructured data has overwhelmed conventional data management system capabilities in organizing, analyzing, and procuring useful information in a timely fashion. Structured data sources have a pre-defined pattern that makes data preprocessing and information retrieval tasks relatively easy for the current technologies that have been designed to handle structured and repeatable data. Unlike structured data, unstructured data usually exists in an unorganized format that offers no or little insight unless indexed and stored in an organized fashion. The inherent format of unstructured data exacerbates difficulties in data preprocessing and information extraction. As a result, despite the vastness of unstructured data, most of the decisions are mainly based on information extracted from structured data. The objective of this research is to explore different text and data mining methods that can be leveraged in the transportation safety domain to improve the integration of unstructured textual information in the decision-making process. Different case studies in the field of transportation are explored utilizing the police officer crash narratives in Michigan and self-reported collision and near-miss reports from the crowdsourcing platform. Each case study covers distinctive data and text mining approaches. In transportation safety, millions of police crash report narratives are generated each year in the US that describes crash scenarios. Apart from these official police reports, road users have been provided with different crowdsourcing platforms whereby they can describe any incident such as near-miss and collision while sharing the road space. The information that is contained in these unstructured textual sources can offer salient knowledge that can help to improve the existing infrastructural safety and services. The advantages and challenges of incorporating extracted textual information with traditional structured crash data are thoroughly discussed. The first case study evaluates a way of integrating structured crash metadata with unstructured crash narratives. The data for testing the proposed procedure is the pedestrian crossing-related crashes at undesignated midblock locations. Both structured crash data and report narratives are used to discern human, environmental, and roadway factors associated with pedestrian crossing-related crashes at undesignated midblock areas. The main emphasis is the contribution of crash narratives in understanding the pattern and causes of pedestrian crashes. The extracted textual feature from crash narratives indicated the most important predictor of pedestrian fatalities were cases when a pedestrian was wearing dark clothing while crossing the road. The type of cloth information was only available in the crash narratives. Further, the Random Forest capability of predicting the fatality instances when pedestrians were crossing at undesignated midblock locations was improved when the extracted textual features from the crash narratives were incorporated in model calibration. The case study highlights the importance of incorporating information from an unstructured textual source in transportation safety studies. The second case study evaluates and proposes efficient ways of automating the process of information extraction using text analytics and a data mining approach. Reports of crashes at signal-controlled intersections in Michigan involving at-fault drivers who were issued a “fail to yield” or “disregard traffic control” hazardous action citation were used in the analysis. The semantic n-gram feature analysis is used to discern the most likely crash scenario at signal-controlled intersections for each of the hazardous actions. Support vector machines and boosted classification trees are developed using unigram and bigram features with different n-gram feature deployment scenarios to predict hazardous action citations. Further, the developed textual-based algorithm proved to be promising in detecting possible errors that were made by the police officers while coding hazardous actions in the crash reports. These findings and the proposed methodology in this case study can be used by the agencies in each state to improve their future editions of crash reporting manuals by providing detailed descriptions of the crash contributing factors. The third case study covers another interesting aspect of the text mining analytics approach namely topic modeling. Topic models are unsupervised probabilistic models that enable users to search and explore the documents based on the underlying themes that form a document. This case study explores the prevalence and co-occurrence of themes in traffic fatal crashes using structural topic modeling and network topology. The study uses Michigan traffic fatal crash narratives to generate topics that are mainly categorized into pre-crash events, crash locations, and involved parties in a crash. Various topics are discovered and variations of topics prevalence across crash types are observed. Also, the centrality and association between topics are observed to vary across crash types. Further, results indicate that automation of crash typing and consistency check can be accomplished with a decent level of accuracy by using extracted latent themes from the crash narratives. Therefore, the proposed textual-based framework in this case study can be part of the advanced and rigorous quality control of police crash reports and other safety-related reports. The fourth case study is an extension of the topic modeling incorporating an advanced machine learning technique namely Artificial Neural Networks. Artificial Neural Networks (ANN) or sometimes known as the connectionist systems is the framework that allows different machine learning algorithms to work together in solving complex tasks. The exploratory text mining, topic modeling approach, and ANN are used to study the self-reported cyclist near-miss and collision reports. The benefit of using text mining and machine learning in this case study is the ability to automatically provide a broad snapshot of near-miss and collision events from the textual data. This study not only exposes topics that led to near misses but also sorts out topics based on how likely the topic’s scenario can result in a collision using the proposed text-based ANN framework. The methodology helps sort out the most critical topics related to cyclist’s safety which require in-depth analysis and discussions to produce actionable insights. Lastly, an online-based tool is created amassing various text and data mining features that were explored in all the case studies. The tool provides a simple to use graphical user interface whereby users with limited statistical and programming skills can still use the tool to extract information from textual data. Users are required to upload textual data and associated metadata. The tool automatically preprocesses the textual data and produces ready-to-use results based on the user’s preferences. The interactive tool can help planners, engineers, and other stakeholders at large in the transportation safety domain to harness the power of text and data mining.
Data Exploration Using Example Based Methods
DOWNLOAD
Author : Matteo Lissandrini
language : en
Publisher: Springer
Release Date : 2018-11-27
Data Exploration Using Example Based Methods written by Matteo Lissandrini and has been published by Springer this book supported file pdf, txt, epub, kindle and other format this book has been release on 2018-11-27 with Computers categories.
Data usually comes in a plethora of formats and dimensions, rendering the exploration and information extraction processes challenging. Thus, being able to perform exploratory analyses in the data with the intent of having an immediate glimpse on some of the data properties is becoming crucial. Exploratory analyses should be simple enough to avoid complicate declarative languages (such as SQL) and mechanisms, and at the same time retain the flexibility and expressiveness of such languages. Recently, we have witnessed a rediscovery of the so-called example-based methods, in which the user, or the analyst, circumvents query languages by using examples as input. An example is a representative of the intended results, or in other words, an item from the result set. Example-based methods exploit inherent characteristics of the data to infer the results that the user has in mind, but may not able to (easily) express. They can be useful in cases where a user is looking for information in an unfamiliar dataset, when the task is particularly challenging like finding duplicate items, or simply when they are exploring the data. In this book, we present an excursus over the main methods for exploratory analysis, with a particular focus on example-based methods. We show how that different data types require different techniques, and present algorithms that are specifically designed for relational, textual, and graph data. The book presents also the challenges and the new frontiers of machine learning in online settings which recently attracted the attention of the database community. The lecture concludes with a vision for further research and applications in this area.
Text Mining With R
DOWNLOAD
Author : Julia Silge
language : en
Publisher: "O'Reilly Media, Inc."
Release Date : 2017-06-12
Text Mining With R written by Julia Silge and has been published by "O'Reilly Media, Inc." this book supported file pdf, txt, epub, kindle and other format this book has been release on 2017-06-12 with Computers categories.
Much of the data available today is unstructured and text-heavy, making it challenging for analysts to apply their usual data wrangling and visualization tools. With this practical book, you’ll explore text-mining techniques with tidytext, a package that authors Julia Silge and David Robinson developed using the tidy principles behind R packages like ggraph and dplyr. You’ll learn how tidytext and other tidy tools in R can make text analysis easier and more effective. The authors demonstrate how treating text as data frames enables you to manipulate, summarize, and visualize characteristics of text. You’ll also learn how to integrate natural language processing (NLP) into effective workflows. Practical code examples and data explorations will help you generate real insights from literature, news, and social media. Learn how to apply the tidy text format to NLP Use sentiment analysis to mine the emotional content of text Identify a document’s most important terms with frequency measurements Explore relationships and connections between words with the ggraph and widyr packages Convert back and forth between R’s tidy and non-tidy text formats Use topic modeling to classify document collections into natural groups Examine case studies that compare Twitter archives, dig into NASA metadata, and analyze thousands of Usenet messages
Humanities Data Analysis
DOWNLOAD
Author : Folgert Karsdorp
language : en
Publisher: Princeton University Press
Release Date : 2021-01-12
Humanities Data Analysis written by Folgert Karsdorp and has been published by Princeton University Press this book supported file pdf, txt, epub, kindle and other format this book has been release on 2021-01-12 with Computers categories.
A practical guide to data-intensive humanities research using the Python programming language The use of quantitative methods in the humanities and related social sciences has increased considerably in recent years, allowing researchers to discover patterns in a vast range of source materials. Despite this growth, there are few resources addressed to students and scholars who wish to take advantage of these powerful tools. Humanities Data Analysis offers the first intermediate-level guide to quantitative data analysis for humanities students and scholars using the Python programming language. This practical textbook, which assumes a basic knowledge of Python, teaches readers the necessary skills for conducting humanities research in the rapidly developing digital environment. The book begins with an overview of the place of data science in the humanities, and proceeds to cover data carpentry: the essential techniques for gathering, cleaning, representing, and transforming textual and tabular data. Then, drawing from real-world, publicly available data sets that cover a variety of scholarly domains, the book delves into detailed case studies. Focusing on textual data analysis, the authors explore such diverse topics as network analysis, genre theory, onomastics, literacy, author attribution, mapping, stylometry, topic modeling, and time series analysis. Exercises and resources for further reading are provided at the end of each chapter. An ideal resource for humanities students and scholars aiming to take their Python skills to the next level, Humanities Data Analysis illustrates the benefits that quantitative methods can bring to complex research questions. Appropriate for advanced undergraduates, graduate students, and scholars with a basic knowledge of Python Applicable to many humanities disciplines, including history, literature, and sociology Offers real-world case studies using publicly available data sets Provides exercises at the end of each chapter for students to test acquired skills Emphasizes visual storytelling via data visualizations
Text Mining With R
DOWNLOAD
Author : Julia Silge. David Robinson
language : en
Publisher:
Release Date : 2017
Text Mining With R written by Julia Silge. David Robinson and has been published by this book supported file pdf, txt, epub, kindle and other format this book has been release on 2017 with categories.
Applying Language Technology In Humanities Research
DOWNLOAD
Author : Barbara McGillivray
language : en
Publisher: Springer Nature
Release Date : 2020-07-13
Applying Language Technology In Humanities Research written by Barbara McGillivray and has been published by Springer Nature this book supported file pdf, txt, epub, kindle and other format this book has been release on 2020-07-13 with Language Arts & Disciplines categories.
This book presents established and state-of-the-art methods in Language Technology (including text mining, corpus linguistics, computational linguistics, and natural language processing), and demonstrates how they can be applied by humanities scholars working with textual data. The landscape of humanities research has recently changed thanks to the proliferation of big data and large textual collections such as Google Books, Early English Books Online, and Project Gutenberg. These resources have yet to be fully explored by new generations of scholars, and the authors argue that Language Technology has a key role to play in the exploration of large-scale textual data. The authors use a series of illustrative examples from various humanistic disciplines (mainly but not exclusively from History, Classics, and Literary Studies) to demonstrate basic and more complex use-case scenarios. This book will be useful to graduate students and researchers in humanistic disciplines working with textual data, including History, Modern Languages, Literary studies, Classics, and Linguistics. This is also a very useful book for anyone teaching or learning Digital Humanities and interested in the basic concepts from computational linguistics, corpus linguistics, and natural language processing.
Text Data Management And Analysis
DOWNLOAD
Author : ChengXiang Zhai
language : en
Publisher: Morgan & Claypool
Release Date : 2016-06-30
Text Data Management And Analysis written by ChengXiang Zhai and has been published by Morgan & Claypool this book supported file pdf, txt, epub, kindle and other format this book has been release on 2016-06-30 with Computers categories.
Recent years have seen a dramatic growth of natural language text data, including web pages, news articles, scientific literature, emails, enterprise documents, and social media such as blog articles, forum posts, product reviews, and tweets. This has led to an increasing demand for powerful software tools to help people analyze and manage vast amounts of text data effectively and efficiently. Unlike data generated by a computer system or sensors, text data are usually generated directly by humans, and are accompanied by semantically rich content. As such, text data are especially valuable for discovering knowledge about human opinions and preferences, in addition to many other kinds of knowledge that we encode in text. In contrast to structured data, which conform to well-defined schemas (thus are relatively easy for computers to handle), text has less explicit structure, requiring computer processing toward understanding of the content encoded in text. The current technology of natural language processing has not yet reached a point to enable a computer to precisely understand natural language text, but a wide range of statistical and heuristic approaches to analysis and management of text data have been developed over the past few decades. They are usually very robust and can be applied to analyze and manage text data in any natural language, and about any topic. This book provides a systematic introduction to all these approaches, with an emphasis on covering the most useful knowledge and skills required to build a variety of practically useful text information systems. The focus is on text mining applications that can help users analyze patterns in text data to extract and reveal useful knowledge. Information retrieval systems, including search engines and recommender systems, are also covered as supporting technology for text mining applications. The book covers the major concepts, techniques, and ideas in text data mining and information retrieval from a practical viewpoint, and includes many hands-on exercises designed with a companion software toolkit (i.e., MeTA) to help readers learn how to apply techniques of text mining and information retrieval to real-world text data and how to experiment with and improve some of the algorithms for interesting application tasks. The book can be used as a textbook for a computer science undergraduate course or a reference book for practitioners working on relevant problems in analyzing and managing text data.
Text Mining And Analysis
DOWNLOAD
Author : Dr. Goutam Chakraborty
language : en
Publisher: SAS Institute
Release Date : 2014-11-22
Text Mining And Analysis written by Dr. Goutam Chakraborty and has been published by SAS Institute this book supported file pdf, txt, epub, kindle and other format this book has been release on 2014-11-22 with Computers categories.
Big data: It's unstructured, it's coming at you fast, and there's lots of it. In fact, the majority of big data is text-oriented, thanks to the proliferation of online sources such as blogs, emails, and social media. However, having big data means little if you can't leverage it with analytics. Now you can explore the large volumes of unstructured text data that your organization has collected with Text Mining and Analysis: Practical Methods, Examples, and Case Studies Using SAS. This hands-on guide to text analytics using SAS provides detailed, step-by-step instructions and explanations on how to mine your text data for valuable insight. Through its comprehensive approach, you'll learn not just how to analyze your data, but how to collect, cleanse, organize, categorize, explore, and interpret it as well. Text Mining and Analysis also features an extensive set of case studies, so you can see examples of how the applications work with real-world data from a variety of industries. Text analytics enables you to gain insights about your customers' behaviors and sentiments. Leverage your organization's text data, and use those insights for making better business decisions with Text Mining and Analysis. This book is part of the SAS Press program.