Fine Feature Reconstruction In Point Set Surfaces Using Deep Learning
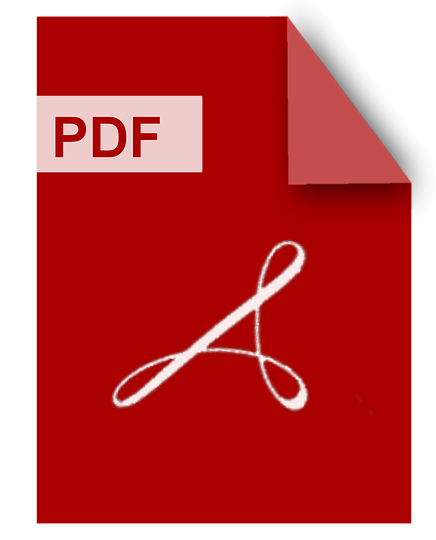
DOWNLOAD
Download Fine Feature Reconstruction In Point Set Surfaces Using Deep Learning PDF/ePub or read online books in Mobi eBooks. Click Download or Read Online button to get Fine Feature Reconstruction In Point Set Surfaces Using Deep Learning book now. This website allows unlimited access to, at the time of writing, more than 1.5 million titles, including hundreds of thousands of titles in various foreign languages. If the content not found or just blank you must refresh this page
Fine Feature Reconstruction In Point Set Surfaces Using Deep Learning
DOWNLOAD
Author : Prashant Raina
language : en
Publisher:
Release Date : 2020
Fine Feature Reconstruction In Point Set Surfaces Using Deep Learning written by Prashant Raina and has been published by this book supported file pdf, txt, epub, kindle and other format this book has been release on 2020 with categories.
Point clouds are typically captured from surfaces of real-world objects using scanning devices. This scanning process invariably results in the loss of sharp edges as well as other geometric features of the original surface, which we collectively refer to as "fine features". This thesis explores leveraging recent advances in deep learning in order to recover fine features of surfaces which were lost when acquiring the point cloud. We first focus on reconstructing sharp edges of the original surface. We define a new concept - a sharpness field - over the underlying surface of a point cloud, whose ridges give the locations of sharp edges even at points not originally sampled from the surface. We then demonstrate that with appropriate training data, deep neural networks can be trained to compute the sharpness field for a point cloud. We evaluate several different local neighborhood representations and deep learning models to improve the accuracy of sharpness field computation across different neighborhood scales. Some applications of the sharpness field are then described. The most important such application is feature-aware smoothing: using the computed sharpness field to preserve sharp edges while removing noise from point clouds. Our novel smoothing algorithm shows superior performance in reconstructing sharp edges and corners compared to the state-of-the-art RIMLS algorithm, while also yielding points lying on sharp edges. Other applications of the sharpness field are also presented: generating a graphical representation of sharp edges and segementing a point cloud into smooth surface patches. We then expand the scope of the problem from sharp edges to undersampled fine features in general. We tackle this by developing a unique deep learning approach to point cloud super-resolution using an innovative application of generative adversarial networks (GANs). The novelty of our super-resolution method lies in framing point cloud super-resolution as a domain translation task between heightmaps obtained from point clouds and heightmaps obtained from triangular meshes. By using recent developments in domain translation using GANs, we obtain results qualitatively and quantitatively superior to state-of-the-art point cloud super-resolution methods, all while using a radically different deep learning approach which is also more computationally efficient. The main contributions of this thesis are: 1. Establishing sharpness field computation as a novel method for localizing sharp edges in 3D point clouds. 2. Several new data-driven methods to compute the sharpness field for a point cloud using different local neighborhood representations and machine learning models. 3. A novel feature-aware smoothing algorithm for denoising point clouds while preserving sharp edges, using the aforementioned sharpness field. This method produces denoising results superior to the state-of-the-art RIMLS smoothing method. 4. An innovative application of GAN-based domain translation, in order to transform sparse heightmaps obtained from point cloud neighborhoods into dense heightmaps obtained from triangular meshes. 5. A unique method for reconstrucing fine features in point clouds, by using heightmap domain translation to perform point cloud super-resolution. The reconstructed surfaces are qualitatively and quantitatively superior to those produced by state-of-the-art point cloud super-resolution methods. Other contributions include: 1. An algorithm for extracting an explicit graphical representation of the sharp edges of a point cloud, using the sharpness field. 2. An algorithm for segmenting a point cloud into smooth patches, using the sharpness field. 3. Feature-aware smoothing algorithms which incorporate the aforementioned edge graph and patch segmentation.
Geometric Deep Learning
DOWNLOAD
Author : Chu Wang
language : en
Publisher:
Release Date : 2020
Geometric Deep Learning written by Chu Wang and has been published by this book supported file pdf, txt, epub, kindle and other format this book has been release on 2020 with categories.
"Advances in deep learning techniques have revolutionized computer vision research and have lead to unprecedented success in visual recognition tasks. As a result, many computer vision researchers are now engaged in developing neural architectures and loss functions to handle particular computer vision problems. However, most current neural architectures cannot easily handle 3D input data and this has lead to new interest in representation learning with deep architectures, but on 3D data formats.In an early development of this thesis, we worked on abstracting features from 2.5D point clouds, interpreted as a 2D colour image and depth map pair, thus enabling the use of well studied 2D neural networks. The community had overlooked the potential of deriving higher order representations from the depth map, which could grant invariance to rigid transformations. We proposed a principled method for transforming the 2.5D input data to higher order surface geometric feature maps, including surface normals and curvatures, and applied pretrained CNNs on these new modalities for geometric feature abstraction from the 2.5D input data. However, this approach did not directly allow for the handling of unorganized 3D input. It could only work on 2.5D point clouds where the points could be mapped to pixels in a 2D image. Therefore, we took a step in this direction by working on 3D mesh representation learning. We chose to model a 3D mesh using a graph comprised of rendered 2D views. In order to abstract a global representation of the 3D mesh from the constructed view graph, we proposed a novel recursive cluster-pooling aggregation algorithm. The proposed method demonstrated nontrivial improvements over related work at the time. The empirical results we reported on the ModelNet40 categorization task ranked in second place on the associated leaderboard.Despite their effectiveness, the above methods were not native 3D approaches in that they did not directly abstract features from unorganized 3D data. Thus we moved on to representation learning directly from unorganized point clouds, using spectral graph convolution on local point neighbourhoods. Here we first sampled local point neighbourhoods from the input cloud, and inside each fix sized neighbourhood, a local graph was constructed with each point as a node. Spectral graph convolution was carried out on each local graph, followed by the application of a cluster pooling algorithm to yield a single feature vector representing this neighbourhood. This method improved point set representation learning by incorporating structural features embedded via local graphs, and boosted performance in point set classification and segmentation benchmarks. However, the local graphs remained the same once constructed, and could not be adaptively learned during the training phase.In a complementary direction, research in attention mechanisms has demonstrated that it is beneficial to parametrize the graph structures in GCN-like models, where the adjacency matrix can be learned during training to further minimize the task loss. Despite the demonstrated boost over base models, these adaptive GCN variants rely solely on the task loss to carry out graph structure learning, and as a result, the learned graph structure is usually ad hoc. Motivated by the lack of interpretability in graph structure learning, in the fourth and final contribution of this thesis we propose to explicitly supervise the graphs in GCN-like neural networks, using a novel affinity mass loss. We aim to place emphasis on designated entries in the graph adjacency matrix, which are selected by a user-specified graph supervision target. We demonstrate the effectiveness of the proposed graph supervision method on visual attention networks and regular mini-batch training. In addition to the performance boost in visual recognition tasks, the graph structures learned with the affinity loss demonstrate a much higher degree of interpretability"--
Machine Learning In Medical Imaging
DOWNLOAD
Author : Heung-Il Suk
language : en
Publisher: Springer Nature
Release Date : 2019-10-09
Machine Learning In Medical Imaging written by Heung-Il Suk and has been published by Springer Nature this book supported file pdf, txt, epub, kindle and other format this book has been release on 2019-10-09 with Computers categories.
This book constitutes the proceedings of the 10th International Workshop on Machine Learning in Medical Imaging, MLMI 2019, held in conjunction with MICCAI 2019, in Shenzhen, China, in October 2019. The 78 papers presented in this volume were carefully reviewed and selected from 158 submissions. They focus on major trends and challenges in the area, aiming to identify new-cutting-edge techniques and their uses in medical imaging. Topics dealt with are: deep learning, generative adversarial learning, ensemble learning, sparse learning, multi-task learning, multi-view learning, manifold learning, and reinforcement learning, with their applications to medical image analysis, computer-aided detection and diagnosis, multi-modality fusion, image reconstruction, image retrieval, cellular image analysis, molecular imaging, digital pathology, etc.
Computer Vision Eccv 2020
DOWNLOAD
Author : Andrea Vedaldi
language : en
Publisher: Springer Nature
Release Date : 2020-10-28
Computer Vision Eccv 2020 written by Andrea Vedaldi and has been published by Springer Nature this book supported file pdf, txt, epub, kindle and other format this book has been release on 2020-10-28 with Computers categories.
The 30-volume set, comprising the LNCS books 12346 until 12375, constitutes the refereed proceedings of the 16th European Conference on Computer Vision, ECCV 2020, which was planned to be held in Glasgow, UK, during August 23-28, 2020. The conference was held virtually due to the COVID-19 pandemic. The 1360 revised papers presented in these proceedings were carefully reviewed and selected from a total of 5025 submissions. The papers deal with topics such as computer vision; machine learning; deep neural networks; reinforcement learning; object recognition; image classification; image processing; object detection; semantic segmentation; human pose estimation; 3d reconstruction; stereo vision; computational photography; neural networks; image coding; image reconstruction; object recognition; motion estimation.
Computer Vision Eccv 2018
DOWNLOAD
Author : Vittorio Ferrari
language : en
Publisher: Springer
Release Date : 2018-10-05
Computer Vision Eccv 2018 written by Vittorio Ferrari and has been published by Springer this book supported file pdf, txt, epub, kindle and other format this book has been release on 2018-10-05 with Computers categories.
The sixteen-volume set comprising the LNCS volumes 11205-11220 constitutes the refereed proceedings of the 15th European Conference on Computer Vision, ECCV 2018, held in Munich, Germany, in September 2018.The 776 revised papers presented were carefully reviewed and selected from 2439 submissions. The papers are organized in topical sections on learning for vision; computational photography; human analysis; human sensing; stereo and reconstruction; optimization; matching and recognition; video attention; and poster sessions.
Ecai 2020
DOWNLOAD
Author : G. De Giacomo
language : en
Publisher: IOS Press
Release Date : 2020-09-11
Ecai 2020 written by G. De Giacomo and has been published by IOS Press this book supported file pdf, txt, epub, kindle and other format this book has been release on 2020-09-11 with Computers categories.
This book presents the proceedings of the 24th European Conference on Artificial Intelligence (ECAI 2020), held in Santiago de Compostela, Spain, from 29 August to 8 September 2020. The conference was postponed from June, and much of it conducted online due to the COVID-19 restrictions. The conference is one of the principal occasions for researchers and practitioners of AI to meet and discuss the latest trends and challenges in all fields of AI and to demonstrate innovative applications and uses of advanced AI technology. The book also includes the proceedings of the 10th Conference on Prestigious Applications of Artificial Intelligence (PAIS 2020) held at the same time. A record number of more than 1,700 submissions was received for ECAI 2020, of which 1,443 were reviewed. Of these, 361 full-papers and 36 highlight papers were accepted (an acceptance rate of 25% for full-papers and 45% for highlight papers). The book is divided into three sections: ECAI full papers; ECAI highlight papers; and PAIS papers. The topics of these papers cover all aspects of AI, including Agent-based and Multi-agent Systems; Computational Intelligence; Constraints and Satisfiability; Games and Virtual Environments; Heuristic Search; Human Aspects in AI; Information Retrieval and Filtering; Knowledge Representation and Reasoning; Machine Learning; Multidisciplinary Topics and Applications; Natural Language Processing; Planning and Scheduling; Robotics; Safe, Explainable, and Trustworthy AI; Semantic Technologies; Uncertainty in AI; and Vision. The book will be of interest to all those whose work involves the use of AI technology.
Computer Vision Accv 2022
DOWNLOAD
Author : Lei Wang
language : en
Publisher: Springer Nature
Release Date : 2023-03-03
Computer Vision Accv 2022 written by Lei Wang and has been published by Springer Nature this book supported file pdf, txt, epub, kindle and other format this book has been release on 2023-03-03 with Computers categories.
The 7-volume set of LNCS 13841-13847 constitutes the proceedings of the 16th Asian Conference on Computer Vision, ACCV 2022, held in Macao, China, December 2022. The total of 277 contributions included in the proceedings set was carefully reviewed and selected from 836 submissions during two rounds of reviewing and improvement. The papers focus on the following topics: Part I: 3D computer vision; optimization methods; Part II: applications of computer vision, vision for X; computational photography, sensing, and display; Part III: low-level vision, image processing; Part IV: face and gesture; pose and action; video analysis and event recognition; vision and language; biometrics; Part V: recognition: feature detection, indexing, matching, and shape representation; datasets and performance analysis; Part VI: biomedical image analysis; deep learning for computer vision; Part VII: generative models for computer vision; segmentation and grouping; motion and tracking; document image analysis; big data, large scale methods.
Geometric Modeling And Processing Gmp 2006
DOWNLOAD
Author : Myung-Soo Kim
language : en
Publisher: Springer Science & Business Media
Release Date : 2006-07-11
Geometric Modeling And Processing Gmp 2006 written by Myung-Soo Kim and has been published by Springer Science & Business Media this book supported file pdf, txt, epub, kindle and other format this book has been release on 2006-07-11 with Computers categories.
This book constitutes the refereed proceedings of the 4th International Conference on Geometric Modeling and Processing, GMP 2006, held in Pittsburgh, PA, USA in July 2006. The 36 revised full papers and 21 revised short papers presented were carefully reviewed and selected from a total of 84 submissions. All current issues in the area of geometric modeling and processing are addressed and the impact in such areas as computer graphics, computer vision, machining, robotics, and scientific visualization is shown. The papers are organized in topical sections on shape reconstruction, curves and surfaces, geometric processing, shape deformation, shape description, shape recognition, geometric modeling, subdivision surfaces, and engineering applications.
Mathematical Methods For Curves And Surfaces
DOWNLOAD
Author : Morten Dæhlen
language : en
Publisher: Springer
Release Date : 2010-02-12
Mathematical Methods For Curves And Surfaces written by Morten Dæhlen and has been published by Springer this book supported file pdf, txt, epub, kindle and other format this book has been release on 2010-02-12 with Computers categories.
This volume constitutes the thoroughly refereed post-conference proceedings of the 7th International Conference on Mathematical Methods for Curves and Surfaces, MMCS 2008, held in Tønsberg, Norway, in June/July 2008. The 28 revised full papers presented were carefully reviewed and selected from 129 talks presented at the conference. The topics addressed by the papers range from mathematical analysis of various methods to practical implementation on modern graphics processing units.
Applied Mathematics Modeling And Computer Simulation
DOWNLOAD
Author : C.-H. Chen
language : en
Publisher: IOS Press
Release Date : 2022-02-25
Applied Mathematics Modeling And Computer Simulation written by C.-H. Chen and has been published by IOS Press this book supported file pdf, txt, epub, kindle and other format this book has been release on 2022-02-25 with Computers categories.
The pervasiveness of computers in every field of science, industry and everyday life has meant that applied mathematics, particularly in relation to modeling and simulation, has become ever more important in recent years. This book presents the proceedings of the 2021 International Conference on Applied Mathematics, Modeling and Computer Simulation (AMMCS 2021), hosted in Wuhan, China, and held as a virtual event from 13 to 14 November 2021. The aim of the conference is to foster the knowledge and understanding of recent advances across the broad fields of applied mathematics, modeling and computer simulation, and it provides an annual platform for scholars and researchers to communicate important recent developments in their areas of specialization to colleagues and other scientists in related disciplines. This year more than 150 participants were able to exchange knowledge and discuss recent developments via the conference. The book contains 115 peer-reviewed papers, selected from more than 250 submissions and ranging from the theoretical and conceptual to the strongly pragmatic and all addressing industrial best practice. Topics covered include mathematical modeling and applications, engineering applications and scientific computations, and the simulation of intelligent systems. Providing an overview of recent development and with a mix of practical experiences and enlightening ideas, the book will be of interest to researchers and practitioners everywhere.