Forecasting Long Term Electricity Demand Time Series Using Artificial Neural Networks
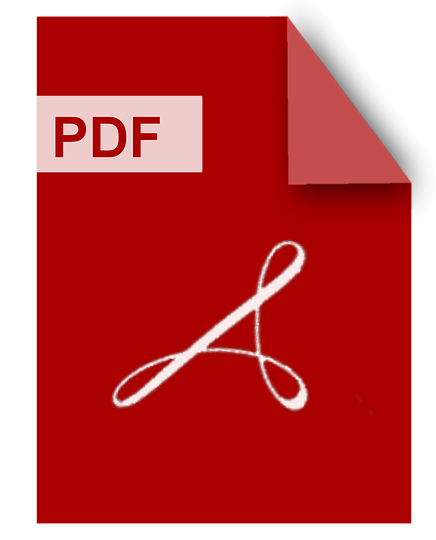
DOWNLOAD
Download Forecasting Long Term Electricity Demand Time Series Using Artificial Neural Networks PDF/ePub or read online books in Mobi eBooks. Click Download or Read Online button to get Forecasting Long Term Electricity Demand Time Series Using Artificial Neural Networks book now. This website allows unlimited access to, at the time of writing, more than 1.5 million titles, including hundreds of thousands of titles in various foreign languages. If the content not found or just blank you must refresh this page
Forecasting Long Term Electricity Demand Time Series Using Artificial Neural Networks
DOWNLOAD
Author : Christian Behm
language : en
Publisher:
Release Date : 2020
Forecasting Long Term Electricity Demand Time Series Using Artificial Neural Networks written by Christian Behm and has been published by this book supported file pdf, txt, epub, kindle and other format this book has been release on 2020 with categories.
Accurate forecast of electricity load are increasingly important. We present a method to forecast long-term weather-dependent hourly electricity load using artificial neural networks. The fully connected dense artificial neural networks with 5 hidden layers and 1,024 hidden nodes per layer are trained using historic data from 2006 to 2015. Input parameters comprise calendrical information, annual peak loads and weather data. The results are benchmarked against the method to forecast electric loads used in the current mid-term adequacy forecasts published by the European Network of Transmission System Operators (entso-e). For validation year 2016, our approach shows a mean absolute percentage error of 2.8%, whereas the common approach as used by entso-e shows an average error of 4.8% using peak load scaling. Further, we conduct forecasts for Germany, Sweden, Spain, and France for scenario year 2025 and assess parameter variations. Our approach can serve to increase prediction accuracy of future electricity loads.
Forecasting And Assessing Risk Of Individual Electricity Peaks
DOWNLOAD
Author : Maria Jacob
language : en
Publisher: Springer
Release Date : 2019-10-07
Forecasting And Assessing Risk Of Individual Electricity Peaks written by Maria Jacob and has been published by Springer this book supported file pdf, txt, epub, kindle and other format this book has been release on 2019-10-07 with Mathematics categories.
The overarching aim of this open access book is to present self-contained theory and algorithms for investigation and prediction of electric demand peaks. A cross-section of popular demand forecasting algorithms from statistics, machine learning and mathematics is presented, followed by extreme value theory techniques with examples. In order to achieve carbon targets, good forecasts of peaks are essential. For instance, shifting demand or charging battery depends on correct demand predictions in time. Majority of forecasting algorithms historically were focused on average load prediction. In order to model the peaks, methods from extreme value theory are applied. This allows us to study extremes without making any assumption on the central parts of demand distribution and to predict beyond the range of available data. While applied on individual loads, the techniques described in this book can be extended naturally to substations, or to commercial settings. Extreme value theory techniques presented can be also used across other disciplines, for example for predicting heavy rainfalls, wind speed, solar radiation and extreme weather events. The book is intended for students, academics, engineers and professionals that are interested in short term load prediction, energy data analytics, battery control, demand side response and data science in general.
Recurrent Neural Networks For Short Term Load Forecasting
DOWNLOAD
Author : Filippo Maria Bianchi
language : en
Publisher: Springer
Release Date : 2017-11-17
Recurrent Neural Networks For Short Term Load Forecasting written by Filippo Maria Bianchi and has been published by Springer this book supported file pdf, txt, epub, kindle and other format this book has been release on 2017-11-17 with Computers categories.
The key component in forecasting demand and consumption of resources in a supply network is an accurate prediction of real-valued time series. Indeed, both service interruptions and resource waste can be reduced with the implementation of an effective forecasting system. Significant research has thus been devoted to the design and development of methodologies for short term load forecasting over the past decades. A class of mathematical models, called Recurrent Neural Networks, are nowadays gaining renewed interest among researchers and they are replacing many practical implementations of the forecasting systems, previously based on static methods. Despite the undeniable expressive power of these architectures, their recurrent nature complicates their understanding and poses challenges in the training procedures. Recently, new important families of recurrent architectures have emerged and their applicability in the context of load forecasting has not been investigated completely yet. This work performs a comparative study on the problem of Short-Term Load Forecast, by using different classes of state-of-the-art Recurrent Neural Networks. The authors test the reviewed models first on controlled synthetic tasks and then on different real datasets, covering important practical cases of study. The text also provides a general overview of the most important architectures and defines guidelines for configuring the recurrent networks to predict real-valued time series.
Advanced Optimization Methods And Big Data Applications In Energy Demand Forecast
DOWNLOAD
Author : Federico Divina
language : en
Publisher: MDPI
Release Date : 2021-08-30
Advanced Optimization Methods And Big Data Applications In Energy Demand Forecast written by Federico Divina and has been published by MDPI this book supported file pdf, txt, epub, kindle and other format this book has been release on 2021-08-30 with Technology & Engineering categories.
The use of data collectors in energy systems is growing more and more. For example, smart sensors are now widely used in energy production and energy consumption systems. This implies that huge amounts of data are generated and need to be analyzed in order to extract useful insights from them. Such big data give rise to a number of opportunities and challenges for informed decision making. In recent years, researchers have been working very actively in order to come up with effective and powerful techniques in order to deal with the huge amount of data available. Such approaches can be used in the context of energy production and consumption considering the amount of data produced by all samples and measurements, as well as including many additional features. With them, automated machine learning methods for extracting relevant patterns, high-performance computing, or data visualization are being successfully applied to energy demand forecasting.
Short Term Load Forecasting By Artificial Intelligent Technologies
DOWNLOAD
Author : Wei-Chiang Hong
language : en
Publisher: MDPI
Release Date : 2019-01-29
Short Term Load Forecasting By Artificial Intelligent Technologies written by Wei-Chiang Hong and has been published by MDPI this book supported file pdf, txt, epub, kindle and other format this book has been release on 2019-01-29 with categories.
This book is a printed edition of the Special Issue "Short-Term Load Forecasting by Artificial Intelligent Technologies" that was published in Energies
Energy Planning Model Design For Forecasting The Final Energy Consumption Using Artificial Neural Networks
DOWNLOAD
Author : Haidy Eissa
language : en
Publisher:
Release Date : 2021
Energy Planning Model Design For Forecasting The Final Energy Consumption Using Artificial Neural Networks written by Haidy Eissa and has been published by this book supported file pdf, txt, epub, kindle and other format this book has been release on 2021 with Artificial intelligence categories.
Abstract: "Energy Trilemma" has recently received an increasing concern among policy makers. The trilemma conceptual framework is based on three main dimensions: environmental sustainability, energy equity, and energy security. Energy security reflects a nation's capability to meet current and future energy demand. Rational energy planning is thus a fundamental aspect to articulate energy policies. The energy system is huge and complex, accordingly in order to guarantee the availability of energy supply, it is necessary to implement strategies on the consumption side. Energy modeling is a tool that helps policy makers and researchers understand the fluctuations in the energy system. Over the years, there have been many attempts to develop energy system models, which have varying degrees of success. Artificial Neural Networks (ANN) is one of the modeling techniques that shows great performance in modeling the energy consumption side, which has the time-series characteristics of complexity and nonlinearity. Static feedforward neural networks are extensively used in literature due to their simplicity. In this thesis, we propose two artificial neural network topologies: feedforward and Nonlinear Autoregressive with Exogenous Inputs (NARX) neural networks, where four separate ANN models, are formulated to study and forecast the annual final energy consumption for four different sectors in the United Kingdom till 2035: transport, domestic, services, industrial. The outputs of all models are finally summed up to yield UK's total final energy consumption. Furthermore, in this thesis, we use the Bayesian optimization algorithm to search for the optimal network hyperparameters. Moreover, instead of arbitrarily selecting input parameters in a qualitative manner, a sequential backward selection technique is used to exclude any uninformative input variables that have no predictive power, and eventually select the optimal set of input parameters. The network performance is measured with regards to various accuracy metrics such as Root Mean Square Error (RMSE), and Mean Average Percentage Error (MAPE). The resulting forecasts are eventually compared to the final energy consumption outlook from UK's governmental Department for Business, Energy & Industrial Strategy (BEIS). The comparisons show that the NARX model offers superior results compared to the feedforward model in terms of the accuracy metrics as well as the long-term forecasts. Moreover, the developed NARX model succeeded in having a better performance than other models developed in the literature.
Modeling And Forecasting Electricity Loads And Prices
DOWNLOAD
Author : Rafal Weron
language : en
Publisher: John Wiley & Sons
Release Date : 2006-12-15
Modeling And Forecasting Electricity Loads And Prices written by Rafal Weron and has been published by John Wiley & Sons this book supported file pdf, txt, epub, kindle and other format this book has been release on 2006-12-15 with Business & Economics categories.
This book offers an in-depth and up-to-date review of different statistical tools that can be used to analyze and forecast the dynamics of two crucial for every energy company processes—electricity prices and loads. It provides coverage of seasonal decomposition, mean reversion, heavy-tailed distributions, exponential smoothing, spike preprocessing, autoregressive time series including models with exogenous variables and heteroskedastic (GARCH) components, regime-switching models, interval forecasts, jump-diffusion models, derivatives pricing and the market price of risk. Modeling and Forecasting Electricity Loads and Prices is packaged with a CD containing both the data and detailed examples of implementation of different techniques in Matlab, with additional examples in SAS. A reader can retrace all the intermediate steps of a practical implementation of a model and test his understanding of the method and correctness of the computer code using the same input data. The book will be of particular interest to the quants employed by the utilities, independent power generators and marketers, energy trading desks of the hedge funds and financial institutions, and the executives attending courses designed to help them to brush up on their technical skills. The text will be also of use to graduate students in electrical engineering, econometrics and finance wanting to get a grip on advanced statistical tools applied in this hot area. In fact, there are sixteen Case Studies in the book making it a self-contained tutorial to electricity load and price modeling and forecasting.
Deep Learning For Electricity Forecasting Using Time Series Data
DOWNLOAD
Author : Hanan Abdullah Alshehri
language : en
Publisher:
Release Date : 2021
Deep Learning For Electricity Forecasting Using Time Series Data written by Hanan Abdullah Alshehri and has been published by this book supported file pdf, txt, epub, kindle and other format this book has been release on 2021 with Electric power consumption categories.
The complexity and nonlinearities of the modern power grid render traditional physical modeling and mathematical computation unrealistic. AI and predictive machine learning techniques allow for accurate and efficient system modeling and analysis. Electricity consumption forecasting is highly valuable in energy management and sustainability research. Furthermore, accurate energy forecasting can be used to optimize energy allocation. This thesis introduces Deep Learning models including the Convolutional Neural Network (CNN), the Recurrent neural network (RNN), and Long Short-Term memory (LSTM). The Hourly Usage of Energy (HUE) dataset for buildings in British Columbia is used as an example for our investigation, as the dataset contains data from residential customers of BC Hydro, a provincial power utility company. Due to the temporal dependency in time-series observation data, data preprocessing is required before a model can be created. The LSTM model is utilized to create a predictive model for electricity consumption as output. Approximately 63% of the data is used for training, and the remaining 37% is used for testing. Various LSTM parameters are tested and tuned for best performance. Our LSTM predictive model can facilitate power companies’ resource management decisions.
Python Based Deep Learning Methods For Energy Consumption Forecasting
DOWNLOAD
Author : Josep Roman Cardell
language : en
Publisher:
Release Date : 2020
Python Based Deep Learning Methods For Energy Consumption Forecasting written by Josep Roman Cardell and has been published by this book supported file pdf, txt, epub, kindle and other format this book has been release on 2020 with categories.
In a society where we do nothing but increase the use of electricity in our daily life, en-ergy consumption and the corresponding management is a major issue. The predictionof electric energy demand is a key component, for the power system operators, in themanagement of the electrical grid. The importance of forecasting a particular house-hold daily energy consumption does concern the end-user too, by reason of the designand sizing of a suitable renewable energy system and energy storage.The aim of this thesis is to develop and train a computing system capable of predict-ing, with best accuracy as possible, electricity consumption at household-level. Thispaper presents a Short Term Load Forecasting (STLF) with Artificial Neural Networks(ANN), which lead to accurate results in spite of the dwelling consumption unpre-dictability. The recorded data, containing the daily track of electricity consumption overa particular household from 2015 to 2018, was analysed. Subsequently, a study over theANN architecture and training algorithms was carried out in order to define a robustmodel. Furthermore, several experiments were conducted with different models, con-taining distinct inputs, aiming to compare the relevance of a diversity of parametersfor the network's training. Finally, the forecasting of the optimal models, created withthe insights collected over the whole research, was performed and compared in severalspecially selected time periods.The results showed how with the appropriate inputs and selection of hyperparame-ters, a shallow ANN can provide certain accuracy on the forecasting of electric energydemand. As well as a methodology to develop and train an artificial neural network.
Dynamic Activity Predictions Using Graph Based Neural Networks For Time Series Forecasting
DOWNLOAD
Author : Bhuvan Kumar Chennoju
language : en
Publisher:
Release Date : 2023
Dynamic Activity Predictions Using Graph Based Neural Networks For Time Series Forecasting written by Bhuvan Kumar Chennoju and has been published by this book supported file pdf, txt, epub, kindle and other format this book has been release on 2023 with Computer algorithms categories.
Time series forecasting is a vital task in numerous fields, and traditional methods, machine learning models, and neural graph networks have been employed to improve prediction accuracy. However, these techniques need to be revised to understand interdependencies and establishing long-term dependencies when dealing with a network of time series, such as predicting energy demand on interconnected grids. To tackle these challenges, this thesis introduces a framework implementing Attention-based Temporal Graph Convolutional Networks (ATGCNs) that enables holistic treatment of a group of time series while learning inter-dependencies and facilitating message passing for enhanced model efficiency. The major contribution of this thesis lies in developing graph embedding algorithms that convert Microbusiness density data into graph data, considering the spatial distance and time series for the proposed ATGCNs model, enabling dynamic activity predictions. The proposed framework is evaluated through experiments using a U.S. Microbusiness density dataset from the GoDaddy Open Survey. The results reveal that ATGCNs outperform traditional time series statistics and machine learning methods in various evaluation metrics, demonstrating comparable forecasting performance to conventional time series forecasting while addressing network scalability and dynamic nature. Additionally, real-time prediction visualizations based on Tableau were developed to showcase the dynamic nature of predictions in the U.S. Microbusiness density domain. In conclusion, this study’s findings highlight the potential advantages of employing graph-based neural networks for time series forecasting, suggesting that incorporating additional data sources could improve prediction accuracy. As future work, transfer learning with ATGCNs will be applied to new domains such as climate prediction or energy demand on interconnected grids. Furthermore, the graph-embedding algorithm and visualization techniques developed in this project will be applied to new domains and datasets across different domains.