Hidden Markov Models And Probabilistic Learning With Applications To Bioinformatics
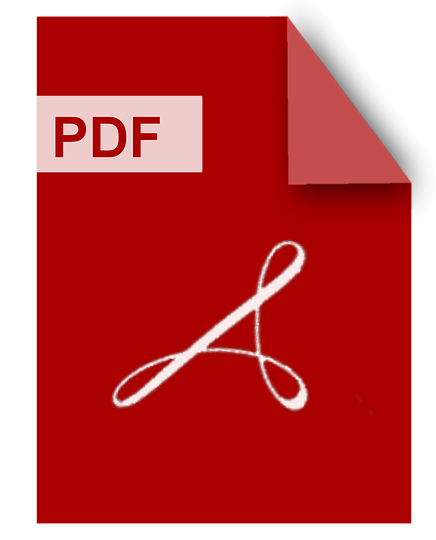
DOWNLOAD
Download Hidden Markov Models And Probabilistic Learning With Applications To Bioinformatics PDF/ePub or read online books in Mobi eBooks. Click Download or Read Online button to get Hidden Markov Models And Probabilistic Learning With Applications To Bioinformatics book now. This website allows unlimited access to, at the time of writing, more than 1.5 million titles, including hundreds of thousands of titles in various foreign languages. If the content not found or just blank you must refresh this page
Hidden Markov Models And Probabilistic Learning With Applications To Bioinformatics
DOWNLOAD
Author : Timo Koski
language : en
Publisher:
Release Date : 1999
Hidden Markov Models And Probabilistic Learning With Applications To Bioinformatics written by Timo Koski and has been published by this book supported file pdf, txt, epub, kindle and other format this book has been release on 1999 with categories.
Hidden Markov Models For Bioinformatics
DOWNLOAD
Author : T. Koski
language : en
Publisher: Springer Science & Business Media
Release Date : 2001-11-30
Hidden Markov Models For Bioinformatics written by T. Koski and has been published by Springer Science & Business Media this book supported file pdf, txt, epub, kindle and other format this book has been release on 2001-11-30 with Computers categories.
The purpose of this book is to give a thorough and systematic introduction to probabilistic modeling in bioinformatics. The book contains a mathematically strict and extensive presentation of the kind of probabilistic models that have turned out to be useful in genome analysis. Questions of parametric inference, selection between model families, and various architectures are treated. Several examples are given of known architectures (e.g., profile HMM) used in genome analysis.
Hidden Markov Models And Some Applications In Bioinformatics
DOWNLOAD
Author : Maryam Alyasin Arvizu
language : en
Publisher:
Release Date : 2003
Hidden Markov Models And Some Applications In Bioinformatics written by Maryam Alyasin Arvizu and has been published by this book supported file pdf, txt, epub, kindle and other format this book has been release on 2003 with Algorithms categories.
Probabilistic Modeling In Bioinformatics And Medical Informatics
DOWNLOAD
Author : Dirk Husmeier
language : en
Publisher: Springer Science & Business Media
Release Date : 2006-05-06
Probabilistic Modeling In Bioinformatics And Medical Informatics written by Dirk Husmeier and has been published by Springer Science & Business Media this book supported file pdf, txt, epub, kindle and other format this book has been release on 2006-05-06 with Computers categories.
Probabilistic Modelling in Bioinformatics and Medical Informatics has been written for researchers and students in statistics, machine learning, and the biological sciences. The first part of this book provides a self-contained introduction to the methodology of Bayesian networks. The following parts demonstrate how these methods are applied in bioinformatics and medical informatics. All three fields - the methodology of probabilistic modeling, bioinformatics, and medical informatics - are evolving very quickly. The text should therefore be seen as an introduction, offering both elementary tutorials as well as more advanced applications and case studies.
Bioinformatics Second Edition
DOWNLOAD
Author : Pierre Baldi
language : en
Publisher: MIT Press
Release Date : 2001-07-20
Bioinformatics Second Edition written by Pierre Baldi and has been published by MIT Press this book supported file pdf, txt, epub, kindle and other format this book has been release on 2001-07-20 with Computers categories.
A guide to machine learning approaches and their application to the analysis of biological data. An unprecedented wealth of data is being generated by genome sequencing projects and other experimental efforts to determine the structure and function of biological molecules. The demands and opportunities for interpreting these data are expanding rapidly. Bioinformatics is the development and application of computer methods for management, analysis, interpretation, and prediction, as well as for the design of experiments. Machine learning approaches (e.g., neural networks, hidden Markov models, and belief networks) are ideally suited for areas where there is a lot of data but little theory, which is the situation in molecular biology. The goal in machine learning is to extract useful information from a body of data by building good probabilistic models—and to automate the process as much as possible. In this book Pierre Baldi and Søren Brunak present the key machine learning approaches and apply them to the computational problems encountered in the analysis of biological data. The book is aimed both at biologists and biochemists who need to understand new data-driven algorithms and at those with a primary background in physics, mathematics, statistics, or computer science who need to know more about applications in molecular biology. This new second edition contains expanded coverage of probabilistic graphical models and of the applications of neural networks, as well as a new chapter on microarrays and gene expression. The entire text has been extensively revised.
Handbook Of Hidden Markov Models In Bioinformatics
DOWNLOAD
Author : Martin Gollery
language : en
Publisher: CRC Press
Release Date : 2008-06-12
Handbook Of Hidden Markov Models In Bioinformatics written by Martin Gollery and has been published by CRC Press this book supported file pdf, txt, epub, kindle and other format this book has been release on 2008-06-12 with Computers categories.
Demonstrating that many useful resources, such as databases, can benefit most bioinformatics projects, the Handbook of Hidden Markov Models in Bioinformatics focuses on how to choose and use various methods and programs available for hidden Markov models (HMMs). The book begins with discussions on key HMM and related profile methods, incl
Inference In Hidden Markov Models
DOWNLOAD
Author : Olivier Cappé
language : en
Publisher: Springer Science & Business Media
Release Date : 2006-04-12
Inference In Hidden Markov Models written by Olivier Cappé and has been published by Springer Science & Business Media this book supported file pdf, txt, epub, kindle and other format this book has been release on 2006-04-12 with Mathematics categories.
This book is a comprehensive treatment of inference for hidden Markov models, including both algorithms and statistical theory. Topics range from filtering and smoothing of the hidden Markov chain to parameter estimation, Bayesian methods and estimation of the number of states. In a unified way the book covers both models with finite state spaces and models with continuous state spaces (also called state-space models) requiring approximate simulation-based algorithms that are also described in detail. Many examples illustrate the algorithms and theory. This book builds on recent developments to present a self-contained view.
Hidden Markov Processes
DOWNLOAD
Author : M. Vidyasagar
language : en
Publisher: Princeton University Press
Release Date : 2014-08-24
Hidden Markov Processes written by M. Vidyasagar and has been published by Princeton University Press this book supported file pdf, txt, epub, kindle and other format this book has been release on 2014-08-24 with Mathematics categories.
This book explores important aspects of Markov and hidden Markov processes and the applications of these ideas to various problems in computational biology. The book starts from first principles, so that no previous knowledge of probability is necessary. However, the work is rigorous and mathematical, making it useful to engineers and mathematicians, even those not interested in biological applications. A range of exercises is provided, including drills to familiarize the reader with concepts and more advanced problems that require deep thinking about the theory. Biological applications are taken from post-genomic biology, especially genomics and proteomics. The topics examined include standard material such as the Perron-Frobenius theorem, transient and recurrent states, hitting probabilities and hitting times, maximum likelihood estimation, the Viterbi algorithm, and the Baum-Welch algorithm. The book contains discussions of extremely useful topics not usually seen at the basic level, such as ergodicity of Markov processes, Markov Chain Monte Carlo (MCMC), information theory, and large deviation theory for both i.i.d and Markov processes. The book also presents state-of-the-art realization theory for hidden Markov models. Among biological applications, it offers an in-depth look at the BLAST (Basic Local Alignment Search Technique) algorithm, including a comprehensive explanation of the underlying theory. Other applications such as profile hidden Markov models are also explored.
Machine Learning In Bioinformatics
DOWNLOAD
Author : Yanqing Zhang
language : en
Publisher: John Wiley & Sons
Release Date : 2009-02-23
Machine Learning In Bioinformatics written by Yanqing Zhang and has been published by John Wiley & Sons this book supported file pdf, txt, epub, kindle and other format this book has been release on 2009-02-23 with Computers categories.
An introduction to machine learning methods and their applications to problems in bioinformatics Machine learning techniques are increasingly being used to address problems in computational biology and bioinformatics. Novel computational techniques to analyze high throughput data in the form of sequences, gene and protein expressions, pathways, and images are becoming vital for understanding diseases and future drug discovery. Machine learning techniques such as Markov models, support vector machines, neural networks, and graphical models have been successful in analyzing life science data because of their capabilities in handling randomness and uncertainty of data noise and in generalization. From an internationally recognized panel of prominent researchers in the field, Machine Learning in Bioinformatics compiles recent approaches in machine learning methods and their applications in addressing contemporary problems in bioinformatics. Coverage includes: feature selection for genomic and proteomic data mining; comparing variable selection methods in gene selection and classification of microarray data; fuzzy gene mining; sequence-based prediction of residue-level properties in proteins; probabilistic methods for long-range features in biosequences; and much more. Machine Learning in Bioinformatics is an indispensable resource for computer scientists, engineers, biologists, mathematicians, researchers, clinicians, physicians, and medical informaticists. It is also a valuable reference text for computer science, engineering, and biology courses at the upper undergraduate and graduate levels.
Machine Learning Approaches To Bioinformatics
DOWNLOAD
Author : Zheng Rong Yang
language : en
Publisher: World Scientific
Release Date : 2010
Machine Learning Approaches To Bioinformatics written by Zheng Rong Yang and has been published by World Scientific this book supported file pdf, txt, epub, kindle and other format this book has been release on 2010 with Computers categories.
This book covers a wide range of subjects in applying machine learning approaches for bioinformatics projects. The book succeeds on two key unique features. First, it introduces the most widely used machine learning approaches in bioinformatics and discusses, with evaluations from real case studies, how they are used in individual bioinformatics projects. Second, it introduces state-of-the-art bioinformatics research methods. The theoretical parts and the practical parts are well integrated for readers to follow the existing procedures in individual research. Unlike most of the bioinformatics books on the market, the content coverage is not limited to just one subject. A broad spectrum of relevant topics in bioinformatics including systematic data mining and computational systems biology researches are brought together in this book, thereby offering an efficient and convenient platform for teaching purposes. An essential reference for both final year undergraduates and graduate students in universities, as well as a comprehensive handbook for new researchers, this book will also serve as a practical guide for software development in relevant bioinformatics projects.