Inference For A Class Of Stochastic Volatility Models In Presence Of Jumps
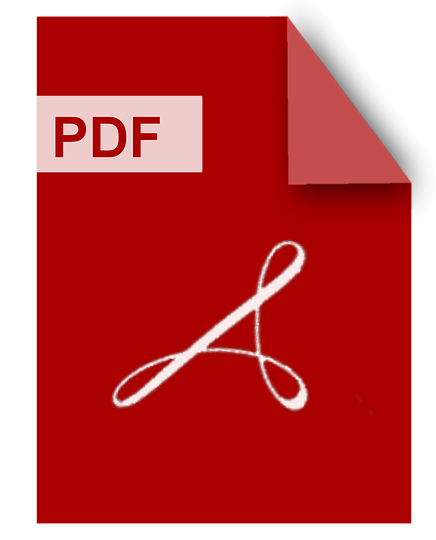
DOWNLOAD
Download Inference For A Class Of Stochastic Volatility Models In Presence Of Jumps PDF/ePub or read online books in Mobi eBooks. Click Download or Read Online button to get Inference For A Class Of Stochastic Volatility Models In Presence Of Jumps book now. This website allows unlimited access to, at the time of writing, more than 1.5 million titles, including hundreds of thousands of titles in various foreign languages. If the content not found or just blank you must refresh this page
Inference For A Class Of Stochastic Volatility Models In Presence Of Jumps
DOWNLOAD
Author : Petra Posedel
language : en
Publisher:
Release Date : 2007
Inference For A Class Of Stochastic Volatility Models In Presence Of Jumps written by Petra Posedel and has been published by this book supported file pdf, txt, epub, kindle and other format this book has been release on 2007 with categories.
A New Class Of Stochastic Volatility Models With Jumps Theory And Estimation
DOWNLOAD
Author : CIRANO.
language : en
Publisher: Montréal : CIRANO
Release Date : 1999
A New Class Of Stochastic Volatility Models With Jumps Theory And Estimation written by CIRANO. and has been published by Montréal : CIRANO this book supported file pdf, txt, epub, kindle and other format this book has been release on 1999 with categories.
A New Class Of Stochastic Volatility Models With Jumps
DOWNLOAD
Author : Mikhail Chernov
language : en
Publisher:
Release Date : 2012
A New Class Of Stochastic Volatility Models With Jumps written by Mikhail Chernov and has been published by this book supported file pdf, txt, epub, kindle and other format this book has been release on 2012 with categories.
The purpose of this paper is to propose a new class of jump diffusions which feature both stochastic volatility and random intensity jumps. Previous studies have focused primarily on pure jump processes with constant intensity and log-normal jumps or constant jump intensity combined with a one factor stochastic volatility model. We introduce several generalizations which can better accommodate several empirical features of returns data. In their most general form we introduce a class of processes which nests jump-diffusions previously considered in empirical work and includes the affine class of random intensity models studied by Bates (1998) and Duffie, Pan and Singleton (1998) but also allows for non-affine random intensity jump components. We attain the generality of our specification through a generic Levy process characterization of the jump component. The processes we introduce share the desirable feature with the affine class that they yield analytically tractable and explicit option pricing formula. The non-affine class of processes we study include specifications where the random intensity jump component depends on the size of the previous jump which represent an alternative to affine random intensity jump processes which feature correlation between the stochastic volatility and jump component. We also allow for and experiment with different empirical specifications of the jump size distributions. We use two types of data sets. One involves the Samp;P500 and the other comprises of 100 years of daily Dow Jones index. The former is a return series often used in the literature and allows us to compare our results with previous studies. The latter has the advantage to provide a long time series and enhances the possibility of estimating the jump component more precisely. The non-affine random intensity jump processes are more parsimonious than the affine class and appear to fit the data much better.
A Stochastic Volatility Model And Inference For The Term Structure Of Interest
DOWNLOAD
Author :
language : en
Publisher:
Release Date : 2004
A Stochastic Volatility Model And Inference For The Term Structure Of Interest written by and has been published by this book supported file pdf, txt, epub, kindle and other format this book has been release on 2004 with categories.
This thesis builds a stochastic volatility model for the term structure of interest rates, which is also known as the dynamics of the yield curve. The main purpose of the model is to propose a parsimonious and plausible approach to capture some characteristics that conform to some empirical evidences and conventions. Eventually, the development reaches a class of multivariate stochastic volatility models, which is flexible, extensible, providing the existence of an inexpensive inference approach. The thesis points out some inconsistency among conventions and practice. First, yield curves and its related curves are conventionally smooth. But in the literature that these curves are modeled as random functions, the co-movement of points on the curve are usually assumed to be governed by some covariance structures that do not generate smooth random curves. Second, it is commonly agreed that the constant volatility is not a sound assumption, but stochastic volatilities have not been commonly considered in related studies. Regarding the above problems, we propose a multiplicative factor stochastic volatility model, which has a relatively simple structure. Though it is apparently simple, the inference is not, because of the presence of stochastic volatilities. We first study the sequential-Monte-Carlo-based maximum likelihood approach, which extends the perspectives of Gaussian linear state-space modeling. We propose a systematic procedure that guides the inference based on this approach. In addition, we also propose a saddlepoint approximation approach, which integrates out states. Then the state propagates by an exact Gaussian approximation. The approximation works reasonably well for univariate models. Moreover, it works even better for the multivariate model that we propose. Because we can enjoy the asymptotic property of the saddlepoint approximation.
Modelling Stochastic Volatility With Leverage And Jumps
DOWNLOAD
Author : Sheheryar Malik
language : en
Publisher:
Release Date : 2010
Modelling Stochastic Volatility With Leverage And Jumps written by Sheheryar Malik and has been published by this book supported file pdf, txt, epub, kindle and other format this book has been release on 2010 with categories.
In this paper we provide a unified methodology for conducting likelihood-based inference on the unknown parameters of a general class of discrete-time stochastic volatility (SV) models, characterized by both a leverage effect and jumps in returns. Given the nonlinear/non-Gaussian state-space form, approximating the likelihood for the parameters is conducted with output generated by the particle filter. Methods are employed to ensure that the approximating likelihood is continuous as a function of the unknown parameters thus enabling the use of standard Newton-Raphson type maximization algorithms. Our approach is robust and efficient relative to alternative Markov Chain Monte Carlo schemes employed in such contexts. In addition it provides a feasible basis for undertaking the nontrivial task of model comparison. Furthermore, we introduce new volatility model, namely SV-GARCH which attempts to bridge the gap between GARCH and stochastic volatility specifications. In nesting the standard GARCH model as a special case, it has the attractive feature of inheriting the same unconditional properties of the standard GARCH model but being conditionally heavier-tailed; thus more robust to outliers. It is demonstrated how this model can be estimated using the described methodology. The technique is applied to daily returns data for S&P 500 stock price index for various spans. In assessing the relative performance of SV with leverage and jumps and nested specifications, we find strong evidence in favour of a including leverage effect and jumps when modelling stochastic volatility. Additionally, we find very encouraging results for SV-GARCH in terms of predictive ability which is comparable to the other models considered.
Abc Of Sv
DOWNLOAD
Author : Michael Creel
language : en
Publisher:
Release Date : 2014
Abc Of Sv written by Michael Creel and has been published by this book supported file pdf, txt, epub, kindle and other format this book has been release on 2014 with categories.
Stochastic Volatility And Jumps
DOWNLOAD
Author : Katja Ignatieva
language : en
Publisher:
Release Date : 2009
Stochastic Volatility And Jumps written by Katja Ignatieva and has been published by this book supported file pdf, txt, epub, kindle and other format this book has been release on 2009 with categories.
This paper analyzes exponentially affine and non-affine stochastic volatility models with jumps in returns and volatility. Markov Chain Monte Carlo (MCMC) technique is applied within a Bayesian inference to estimate model parameters and latent variables using daily returns from the Samp;P 500 stock index. There are two approaches to overcome the problem of misspecification of the square root stochastic volatility model. The first approach proposed by Christo ersen, Jacobs and Mimouni (2008) suggests to investigate some non-affine alternatives of the volatility process. The second approach consists in examining more heavily parametrized models by adding jumps to the return and possibly to the volatility process. The aim of this paper is to combine both model frameworks and to test whether the class of affine models is outperformed by the class of non-affine models if we include jumps into the stochastic processes. We conclude that the non-affine model structure have promising statistical properties and are worth further investigations. Further, we find affine models with jump components that perform similar to the non affine models without jump components. Since non affine models yield economically unrealistic parameter estimates, and research is rather developed for the affine model structures we have a tendency to prefer the affine jump diffusion models.
Statistical Inference For Stochastic Volatility Models
DOWNLOAD
Author : Md. Nazmul Ahsan
language : en
Publisher:
Release Date : 2021
Statistical Inference For Stochastic Volatility Models written by Md. Nazmul Ahsan and has been published by this book supported file pdf, txt, epub, kindle and other format this book has been release on 2021 with categories.
"Although stochastic volatility (SV) models have many appealing features, estimation and inference on SV models are challenging problems due to the inherent difficulty of evaluating the likelihood function. The existing methods are either computationally costly and/or inefficient. This thesis studies and contributes to the SV literature from the estimation, inference, and volatility forecasting viewpoints. It consists of three essays, which include both theoretical and empirical contributions. On the whole, the thesis develops easily applicable statistical methods for stochastic volatility models.The first essay proposes computationally simple moment-based estimators for the first-order SV model. In addition to confirming the enormous computational advantage of the proposed estimators, the results show that the proposed estimators match (or exceed) alternative estimators in terms of precision – including Bayesian estimators proposed in this context, which have the best performance among alternative estimators. Using this simple estimator, we study three crucial test problems (no persistence, no latent specification of volatility, and no stochastic volatility hypothesis), and evaluate these null hypotheses in three ways: asymptotic critical values, a parametric bootstrap procedure, and a maximized Monte Carlo procedure. The proposed methods are applied to daily observations on the returns for three major stock prices [Coca-Cola, Walmart, Ford], and the Standard and Poor’s Composite Price Index. The results show the presence of stochastic volatility with strong persistence.The second essay studies the problem of estimating higher-order stochastic volatility [SV(p)] models. The estimation of SV(p) models is even more challenging and rarely considered in the literature. We propose several estimators for higher-order stochastic volatility models. Among these, the simple winsorized ARMA-based estimator is uniformly superior in terms of bias and RMSE to other estimators, including the Bayesian MCMC estimator. The proposed estimators are applied to stock return data, and the usefulness of the proposed estimators is assessed in two ways. First, using daily returns on the S&P 500 index from 1928 to 2016, we find that higher-order SV models – in particular an SV(3) model – are preferable to an SV(1), from the viewpoints of model fit and both asymptotic and finite-sample tests. Second, using different volatility proxies (squared return and realized volatility), we find that higher-order SV models are preferable for out-of-sample volatility forecasting, whether a high volatility period (such as financial crisis) is included in the estimation sample or the forecasted sample. Our results highlight the usefulness of higher-order SV models for volatility forecasting.In the final essay, we introduce a novel class of generalized stochastic volatility (GSV) models which utilize high-frequency (HF) information (realized volatility (RV) measures). GSV models can accommodate nonstationary volatility process, various distributional assumptions, and exogenous regressors in the latent volatility equation. Instrumental variable methods are employed to provide a unified framework for the analysis (estimation and inference) of GSV models. We consider the parameter inference problem in GSV models with nonstationary volatility and develop identification-robust methods for joint hypotheses involving the volatility persistence parameter and the autocorrelation parameter of the composite error (or the noise ratio). For distributional theory, three different sets of assumptions are considered. In simulations, the proposed tests outperform the usual asymptotic test regarding size and exhibit excellent power. We apply our inference methods to IBM price and option data andidentify several empirical relationships"--
Jump Diffusion And Stochastic Volatility Models In Securities Pricing
DOWNLOAD
Author : Mthuli Ncube
language : en
Publisher:
Release Date : 2012
Jump Diffusion And Stochastic Volatility Models In Securities Pricing written by Mthuli Ncube and has been published by this book supported file pdf, txt, epub, kindle and other format this book has been release on 2012 with categories.
Inference In Stochastic Volatility Models For Gaussian And T Data
DOWNLOAD
Author : Nan Zheng
language : en
Publisher:
Release Date : 2013
Inference In Stochastic Volatility Models For Gaussian And T Data written by Nan Zheng and has been published by this book supported file pdf, txt, epub, kindle and other format this book has been release on 2013 with Estimation theory categories.