Inference For Heavy Tailed Data
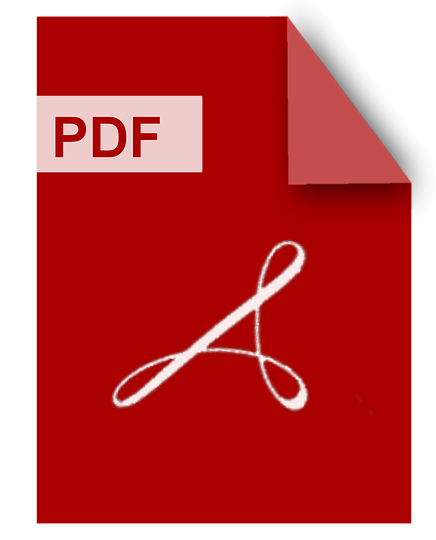
DOWNLOAD
Download Inference For Heavy Tailed Data PDF/ePub or read online books in Mobi eBooks. Click Download or Read Online button to get Inference For Heavy Tailed Data book now. This website allows unlimited access to, at the time of writing, more than 1.5 million titles, including hundreds of thousands of titles in various foreign languages. If the content not found or just blank you must refresh this page
Inference For Heavy Tailed Data
DOWNLOAD
Author : Liang Peng
language : en
Publisher: Academic Press
Release Date : 2017-08-11
Inference For Heavy Tailed Data written by Liang Peng and has been published by Academic Press this book supported file pdf, txt, epub, kindle and other format this book has been release on 2017-08-11 with Mathematics categories.
Heavy tailed data appears frequently in social science, internet traffic, insurance and finance. Statistical inference has been studied for many years, which includes recent bias-reduction estimation for tail index and high quantiles with applications in risk management, empirical likelihood based interval estimation for tail index and high quantiles, hypothesis tests for heavy tails, the choice of sample fraction in tail index and high quantile inference. These results for independent data, dependent data, linear time series and nonlinear time series are scattered in different statistics journals. Inference for Heavy-Tailed Data Analysis puts these methods into a single place with a clear picture on learning and using these techniques. Contains comprehensive coverage of new techniques of heavy tailed data analysis Provides examples of heavy tailed data and its uses Brings together, in a single place, a clear picture on learning and using these techniques
Inference On Heavy Tails From Dependent Data
DOWNLOAD
Author : S. Y. Novak
language : en
Publisher:
Release Date : 1999
Inference On Heavy Tails From Dependent Data written by S. Y. Novak and has been published by this book supported file pdf, txt, epub, kindle and other format this book has been release on 1999 with categories.
Inference For Heavy Tailed Distributions
DOWNLOAD
Author : R. B. Athreya
language : en
Publisher:
Release Date : 1993
Inference For Heavy Tailed Distributions written by R. B. Athreya and has been published by this book supported file pdf, txt, epub, kindle and other format this book has been release on 1993 with categories.
Inference For Extremal Regression With Dependent Heavy Tailed Data
DOWNLOAD
Author : Abdelaati Daouia
language : en
Publisher:
Release Date : 2022
Inference For Extremal Regression With Dependent Heavy Tailed Data written by Abdelaati Daouia and has been published by this book supported file pdf, txt, epub, kindle and other format this book has been release on 2022 with categories.
Inference For The Mean In The Heavy Tailed Case
DOWNLOAD
Author : Joseph P. Romano
language : en
Publisher:
Release Date : 1998
Inference For The Mean In The Heavy Tailed Case written by Joseph P. Romano and has been published by this book supported file pdf, txt, epub, kindle and other format this book has been release on 1998 with categories.
Subsampling Inference For The Mean Of Heavy Tailed Long Memory Time Series
DOWNLOAD
Author : Agnieszka Jach
language : en
Publisher:
Release Date : 2012
Subsampling Inference For The Mean Of Heavy Tailed Long Memory Time Series written by Agnieszka Jach and has been published by this book supported file pdf, txt, epub, kindle and other format this book has been release on 2012 with categories.
In this article, we revisit a time series model introduced by MCElroy and Politis (2007a) and generalize it in several ways to encompass a wider class of stationary, nonlinear, heavy-tailed time series with long memory. The joint asymptotic distribution for the sample mean and sample variance under the extended model is derived; the associated convergence rates are found to depend crucially on the tail thickness and long memory parameter. A self-normalized sample mean that concurrently captures the tail and memory behaviour, is defined. Its asymptotic distribution is approximated by subsampling without the knowledge of tail or/and memory parameters; a result of independent interest regarding subsampling consistency for certain long-range dependent processes is provided. The subsampling-based confidence intervals for the process mean are shown to have good empirical coverage rates in a simulation study. The influence of block size on the coverage and the performance of a data-driven rule for block size selection are assessed. The methodology is further applied to the series of packet-counts from ethernet traffic traces.
Robust Estimation And Inference For Heavy Tailed Garch
DOWNLOAD
Author : Jonathan B. Hill
language : en
Publisher:
Release Date : 2014
Robust Estimation And Inference For Heavy Tailed Garch written by Jonathan B. Hill and has been published by this book supported file pdf, txt, epub, kindle and other format this book has been release on 2014 with categories.
We develop two new estimators for a general class of stationary GARCH models with possibly heavy tailed asymmetrically distributed errors, covering processes with symmetric and asymmetric feedback like GARCH, Asymmetric GARCH, VGARCH and Quadratic GARCH. The first estimator arises from negligibly trimming QML criterion equations according to error extremes. The second imbeds negligibly transformed errors into QML score equations for a Method of Moments estimator. In this case we exploit a sub-class of redescending transforms that includes tail-trimming and functions popular in the robust estimation literature, and we re-center the transformed errors to minimize small sample bias. The negligible transforms allow both identification of the true parameter and asymptotic normality. We present a consistent estimator of the covariance matrix that permits classic inference without knowledge of the rate of convergence. A simulation study shows both of our estimators trump existing ones for sharpness and approximate normality including QML, Log-LAD, and two types of non-Gaussian QML (Laplace and Power-Law). Finally, we apply the tail-trimmed QML estimator to financial data.
Gel Estimation For Heavy Tailed Garch Models With Robust Empirical Likelihood Inference
DOWNLOAD
Author : Jonathan B. Hill
language : en
Publisher:
Release Date : 2013
Gel Estimation For Heavy Tailed Garch Models With Robust Empirical Likelihood Inference written by Jonathan B. Hill and has been published by this book supported file pdf, txt, epub, kindle and other format this book has been release on 2013 with categories.
We construct a Generalized Empirical Likelihood estimator for a GARCH(1,1) model with a possibly heavy tailed error. The estimator imbeds tail-trimmed estimating equations allowing for over-identifying conditions, asymptotic normality, efficiency and empirical likelihood based confidence regions for very heavy-tailed random volatility data. We show the implied probabilities from the tail-trimmed Continuously Updated Estimator elevate weight for usable large values, assign large but not maximum weight to extreme observations, and give the lowest weight to non-leverage points. Finally, we present robust versions of Generalized Empirical Likelihood Ratio, Wald, and Lagrange Multiplier tests, and an efficient and heavy tail robust moment estimator with an application to the estimation of a conditionally heteroscedastic asset's expected shortfall.
Kernel Based Specification Testing With Skewed And Heavy Tailed Data
DOWNLOAD
Author : Alice Sheehan
language : en
Publisher:
Release Date : 2019
Kernel Based Specification Testing With Skewed And Heavy Tailed Data written by Alice Sheehan and has been published by this book supported file pdf, txt, epub, kindle and other format this book has been release on 2019 with Electronic dissertations categories.
In this work, we revisit some of the most common nonparametric specification tests and assess their robustness to real world economic data. Most nonparametric econometric theory is based on a compact support assumption of the data, that is, that the data are well behaved and uniform. In economics, this assumption is regularly violated and the consequences of which are unknown. The intent of this research is twofold. First, we investigate what kind of inference practitioners are obtaining when they apply nonparametric specification tests to economic data. Then, having found that this leads to questionable inference, we modify test statistics and suggest other practices to further improve inference with such data. In the first chapter, we modify a nonparametric test for heteroskedasticity by removing the random denominator in the test statistic, an issue that can be exacerbated when applied to skewed and/or heavy tailed data. We find improvements using our modified test and suggest other methods to improve inference for practitioners applying kernel-based specification tests. In the second chapter, we propose a consistent local-linear test for variable significance that has an asymptotically standard normal distribution. Local-linear estimators are generally the dominant and preferred choice in theoretical and applied kernel regression; however, local-constant estimators are typically employed to construct test statistics. Through Monte Carlo simulations and empirical illustrations, we assess the finite sample performance of the proposed test as compared to a local-constant version. The simulations show that our local-linear test performs well, even with skewed and heavy-tailed regressors and errors, and generally outperforms the local-constant version using a wide range of data generating processes. In the third chapter, using two nonparametric kernel-based specification tests, we investigate the relative performance various auxiliary distributions used to implement the wild bootstrap in the presence of skewed and heavy-tailed regressors. Using a data driven method we identify the most appropriate auxiliary distribution for a given sample. Through Monte Carlo simulations, we show that contrary to popular practice, the Rademacher distribution provides better asymptotic refinements than that of the most commonly employed skew-corrected wild bootstrap for these tests.
Nonparametric Analysis Of Univariate Heavy Tailed Data
DOWNLOAD
Author : Natalia Markovich
language : en
Publisher: John Wiley & Sons
Release Date : 2008-03-11
Nonparametric Analysis Of Univariate Heavy Tailed Data written by Natalia Markovich and has been published by John Wiley & Sons this book supported file pdf, txt, epub, kindle and other format this book has been release on 2008-03-11 with Mathematics categories.
Heavy-tailed distributions are typical for phenomena in complex multi-component systems such as biometry, economics, ecological systems, sociology, web access statistics, internet traffic, biblio-metrics, finance and business. The analysis of such distributions requires special methods of estimation due to their specific features. These are not only the slow decay to zero of the tail, but also the violation of Cramer’s condition, possible non-existence of some moments, and sparse observations in the tail of the distribution. The book focuses on the methods of statistical analysis of heavy-tailed independent identically distributed random variables by empirical samples of moderate sizes. It provides a detailed survey of classical results and recent developments in the theory of nonparametric estimation of the probability density function, the tail index, the hazard rate and the renewal function. Both asymptotical results, for example convergence rates of the estimates, and results for the samples of moderate sizes supported by Monte-Carlo investigation, are considered. The text is illustrated by the application of the considered methodologies to real data of web traffic measurements.