Learning Sparse Data Models Via Geometric Optimization With Applications To Image Processing
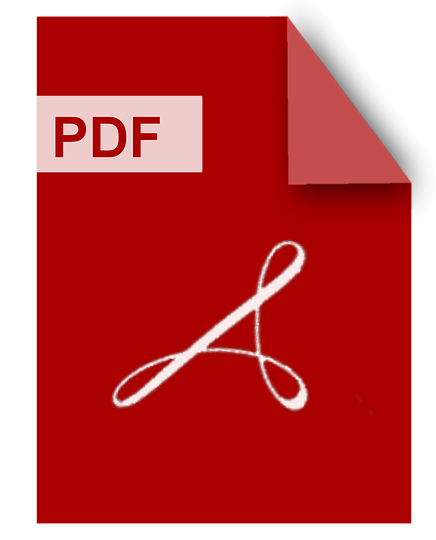
DOWNLOAD
Download Learning Sparse Data Models Via Geometric Optimization With Applications To Image Processing PDF/ePub or read online books in Mobi eBooks. Click Download or Read Online button to get Learning Sparse Data Models Via Geometric Optimization With Applications To Image Processing book now. This website allows unlimited access to, at the time of writing, more than 1.5 million titles, including hundreds of thousands of titles in various foreign languages. If the content not found or just blank you must refresh this page
Learning Sparse Data Models Via Geometric Optimization With Applications To Image Processing
DOWNLOAD
Author : Simon Alois Hawe
language : en
Publisher:
Release Date : 2013
Learning Sparse Data Models Via Geometric Optimization With Applications To Image Processing written by Simon Alois Hawe and has been published by this book supported file pdf, txt, epub, kindle and other format this book has been release on 2013 with categories.
Practical Applications Of Sparse Modeling
DOWNLOAD
Author : Irina Rish
language : en
Publisher: MIT Press
Release Date : 2014-09-19
Practical Applications Of Sparse Modeling written by Irina Rish and has been published by MIT Press this book supported file pdf, txt, epub, kindle and other format this book has been release on 2014-09-19 with Computers categories.
Key approaches in the rapidly developing area of sparse modeling, focusing on its application in fields including neuroscience, computational biology, and computer vision. Sparse modeling is a rapidly developing area at the intersection of statistical learning and signal processing, motivated by the age-old statistical problem of selecting a small number of predictive variables in high-dimensional datasets. This collection describes key approaches in sparse modeling, focusing on its applications in fields including neuroscience, computational biology, and computer vision. Sparse modeling methods can improve the interpretability of predictive models and aid efficient recovery of high-dimensional unobserved signals from a limited number of measurements. Yet despite significant advances in the field, a number of open issues remain when sparse modeling meets real-life applications. The book discusses a range of practical applications and state-of-the-art approaches for tackling the challenges presented by these applications. Topics considered include the choice of method in genomics applications; analysis of protein mass-spectrometry data; the stability of sparse models in brain imaging applications; sequential testing approaches; algorithmic aspects of sparse recovery; and learning sparse latent models. Contributors A. Vania Apkarian, Marwan Baliki, Melissa K. Carroll, Guillermo A. Cecchi, Volkan Cevher, Xi Chen, Nathan W. Churchill, Rémi Emonet, Rahul Garg, Zoubin Ghahramani, Lars Kai Hansen, Matthias Hein, Katherine Heller, Sina Jafarpour, Seyoung Kim, Mladen Kolar, Anastasios Kyrillidis, Seunghak Lee, Aurelie Lozano, Matthew L. Malloy, Pablo Meyer, Shakir Mohamed, Alexandru Niculescu-Mizil, Robert D. Nowak, Jean-Marc Odobez, Peter M. Rasmussen, Irina Rish, Saharon Rosset, Martin Slawski, Stephen C. Strother, Jagannadan Varadarajan, Eric P. Xing
Perspectives In Shape Analysis
DOWNLOAD
Author : Michael Breuß
language : en
Publisher: Springer
Release Date : 2016-09-30
Perspectives In Shape Analysis written by Michael Breuß and has been published by Springer this book supported file pdf, txt, epub, kindle and other format this book has been release on 2016-09-30 with Mathematics categories.
This book presents recent advances in the field of shape analysis. Written by experts in the fields of continuous-scale shape analysis, discrete shape analysis and sparsity, and numerical computing who hail from different communities, it provides a unique view of the topic from a broad range of perspectives. Over the last decade, it has become increasingly affordable to digitize shape information at high resolution. Yet analyzing and processing this data remains challenging because of the large amount of data involved, and because modern applications such as human-computer interaction require real-time processing. Meeting these challenges requires interdisciplinary approaches that combine concepts from a variety of research areas, including numerical computing, differential geometry, deformable shape modeling, sparse data representation, and machine learning. On the algorithmic side, many shape analysis tasks are modeled using partial differential equations, which can be solved using tools from the field of numerical computing. The fields of differential geometry and deformable shape modeling have recently begun to influence shape analysis methods. Furthermore, tools from the field of sparse representations, which aim to describe input data using a compressible representation with respect to a set of carefully selected basic elements, have the potential to significantly reduce the amount of data that needs to be processed in shape analysis tasks. The related field of machine learning offers similar potential. The goal of the Dagstuhl Seminar on New Perspectives in Shape Analysis held in February 2014 was to address these challenges with the help of the latest tools related to geometric, algorithmic and numerical concepts and to bring together researchers at the forefront of shape analysis who can work together to identify open problems and novel solutions. The book resulting from this seminar will appeal to researchers in the field of shape analysis, image and vision, from those who want to become more familiar with the field, to experts interested in learning about the latest advances.
Sparse Modeling For Image And Vision Processing
DOWNLOAD
Author : Julien Mairal
language : en
Publisher: Now Publishers
Release Date : 2014-12-19
Sparse Modeling For Image And Vision Processing written by Julien Mairal and has been published by Now Publishers this book supported file pdf, txt, epub, kindle and other format this book has been release on 2014-12-19 with Computers categories.
Sparse Modeling for Image and Vision Processing offers a self-contained view of sparse modeling for visual recognition and image processing. More specifically, it focuses on applications where the dictionary is learned and adapted to data, yielding a compact representation that has been successful in various contexts.
Advances In Data Science
DOWNLOAD
Author : Ilke Demir
language : en
Publisher: Springer Nature
Release Date : 2021-12-03
Advances In Data Science written by Ilke Demir and has been published by Springer Nature this book supported file pdf, txt, epub, kindle and other format this book has been release on 2021-12-03 with Mathematics categories.
This volume highlights recent advances in data science, including image processing and enhancement on large data, shape analysis and geometry processing in 2D/3D, exploration and understanding of neural networks, and extensions to atypical data types such as social and biological signals. The contributions are based on discussions from two workshops under Association for Women in Mathematics (AWM), namely the second Women in Data Science and Mathematics (WiSDM) Research Collaboration Workshop that took place between July 29 and August 2, 2019 at the Institute for Computational and Experimental Research in Mathematics (ICERM) in Providence, Rhode Island, and the third Women in Shape (WiSh) Research Collaboration Workshop that took place between July 16 and 20, 2018 at Trier University in Robert-Schuman-Haus, Trier, Germany. These submissions, seeded by working groups at the conference, form a valuable source for readers who are interested in ideas and methods developed in interdisciplinary research fields. The book features ideas, methods, and tools developed through a broad range of domains, ranging from theoretical analysis on graph neural networks to applications in health science. It also presents original results tackling real-world problems that often involve complex data analysis on large multi-modal data sources.
Learning The Nonlinear Geometric Structure Of High Dimensional Data
DOWNLOAD
Author : Tong Wu
language : en
Publisher:
Release Date : 2017
Learning The Nonlinear Geometric Structure Of High Dimensional Data written by Tong Wu and has been published by this book supported file pdf, txt, epub, kindle and other format this book has been release on 2017 with categories.
Modern information processing relies on the axiom that high-dimensional data lie near low-dimensional geometric structures. The work presented in this thesis aims to develop new models and algorithms for learning the geometric structures underlying data and to exploit the application of geometry learning in image and video analytics. The first part of the thesis revisits the problem of data-driven learning of these geometric structures and puts forth two new nonlinear geometric models for data describing "related" objects/phenomena. The first one of these models straddles the two extremes of the subspace model and the union-of-subspaces model, and is termed the emph{metric-constrained union-of-subspaces} (MC-UoS) model. The second one of these models--suited for data drawn from a mixture of nonlinear manifolds--generalizes the kernel subspace model, and is termed the emph{metric-constrained kernel union-of-subspaces} (MC-KUoS) model. The main contributions in this regard are threefold. First, we motivate and formalize the problems of MC-UoS and MC-KUoS learning. Second, we present algorithms that efficiently learn an MC-UoS or an MC-KUoS underlying data of interest. Third, we extend these algorithms to the case when parts of the data are missing. The second part of the thesis considers the problem of learning meaningful human action attributes from video data. Representation of human actions as a sequence of human body movements or action attributes enables the development of models for human activity recognition and summarization. We first propose a hierarchical union-of-subspaces model and an approach called hierarchical sparse subspace clustering (HSSC) is developed to learn this model from the data in an unsupervised manner by capturing the variations or movements of each action in different subspaces. We then present an extension of the low-rank representation (LRR) model, termed the emph{clustering-aware structure-constrained low-rank representation} (CS-LRR) model, for unsupervised learning of human action attributes from video data. The CS-LRR model is based on the union-of-subspaces framework, and integrates spectral clustering into the LRR optimization problem for better subspace clustering results. We also introduce a hierarchical subspace clustering approach, termed hierarchical CS-LRR, to learn the attributes without the need for a priori specification of their number. By visualizing and labeling these action attributes, the hierarchical model can be used to semantically summarize long video sequences of human actions at multiple resolutions. A human action or activity can also be uniquely represented as a sequence of transitions from one action attribute to another, which can then be used for human action recognition.
Study On Efficient Sparse And Low Rank Optimization And Its Applications
DOWNLOAD
Author : Jian Lou
language : en
Publisher:
Release Date : 2018
Study On Efficient Sparse And Low Rank Optimization And Its Applications written by Jian Lou and has been published by this book supported file pdf, txt, epub, kindle and other format this book has been release on 2018 with Algorithms categories.
Sparse and low-rank models have been becoming fundamental machine learning tools and have wide applications in areas including computer vision, data mining, bioinformatics and so on. It is of vital importance, yet of great difficulty, to develop efficient optimization algorithms for solving these models, especially under practical design considerations of computational, communicational and privacy restrictions for ever-growing larger scale problems. This thesis proposes a set of new algorithms to improve the efficiency of the sparse and low-rank models optimization. First, facing a large number of data samples during training of empirical risk minimization (ERM) with structured sparse regularization, the gradient computation part of the optimization can be computationally expensive and becomes the bottleneck. Therefore, I propose two gradient efficient optimization algorithms to reduce the total or per-iteration computational cost of the gradient evaluation step, which are new variants of the widely used generalized conditional gradient (GCG) method and incremental proximal gradient (PG) method, correspondingly. In detail, I propose a novel algorithm under GCG framework that requires optimal count of gradient evaluations as proximal gradient. I also propose a refined variant for a type of gauge regularized problem, where approximation techniques are allowed to further accelerate linear subproblem computation. Moreover, under the incremental proximal gradient framework, I propose to approximate the composite penalty by its proximal average under incremental gradient framework, so that a trade-off is made between precision and efficiency. Theoretical analysis and empirical studies show the efficiency of the proposed methods. Furthermore, the large data dimension (e.g. the large frame size of high-resolution image and video data) can lead to high per-iteration computational complexity, thus results into poor-scalability of the optimization algorithm from practical perspective. In particular, in spectral k-support norm regularized robust low-rank matrix and tensor optimization, traditional proximal map based alternating direction method of multipliers (ADMM) requires to evaluate a super-linear complexity subproblem in each iteration. I propose a set of per-iteration computational efficient alternatives to reduce the cost to linear and nearly linear with respect to the input data dimension for matrix and tensor case, correspondingly. The proposed algorithms consider the dual objective of the original problem that can take advantage of the more computational efficient linear oracle of the spectral k-support norm to be evaluated. Further, by studying the sub-gradient of the loss of the dual objective, a line-search strategy is adopted in the algorithm to enable it to adapt to the Holder smoothness. The overall convergence rate is also provided. Experiments on various computer vision and image processing applications demonstrate the superior prediction performance and computation efficiency of the proposed algorithm. In addition, since machine learning datasets often contain sensitive individual information, privacy-preserving becomes more and more important during sparse optimization. I provide two differentially private optimization algorithms under two common large-scale machine learning computing contexts, i.e., distributed and streaming optimization, correspondingly. For the distributed setting, I develop a new algorithm with 1) guaranteed strict differential privacy requirement, 2) nearly optimal utility and 3) reduced uplink communication complexity, for a nearly unexplored context with features partitioned among different parties under privacy restriction. For the streaming setting, I propose to improve the utility of the private algorithm by trading the privacy of distant input instances, under the differential privacy restriction. I show that the proposed method can either solve the private approximation function by a projected gradient update for projection-friendly constraints, or by a conditional gradient step for linear oracle-friendly constraint, both of which improve the regret bound to match the nonprivate optimal counterpart.
Biometrics Concepts Methodologies Tools And Applications
DOWNLOAD
Author : Management Association, Information Resources
language : en
Publisher: IGI Global
Release Date : 2016-08-30
Biometrics Concepts Methodologies Tools And Applications written by Management Association, Information Resources and has been published by IGI Global this book supported file pdf, txt, epub, kindle and other format this book has been release on 2016-08-30 with Social Science categories.
Security and authentication issues are surging to the forefront of the research realm in global society. As technology continues to evolve, individuals are finding it easier to infiltrate various forums and facilities where they can illegally obtain information and access. By implementing biometric authentications to these forums, users are able to prevent attacks on their privacy and security. Biometrics: Concepts, Methodologies, Tools, and Applications is a multi-volume publication highlighting critical topics related to access control, user identification, and surveillance technologies. Featuring emergent research on the issues and challenges in security and privacy, various forms of user authentication, biometric applications to image processing and computer vision, and security applications within the field, this publication is an ideal reference source for researchers, engineers, technology developers, students, and security specialists.
Mathematics In Image Processing
DOWNLOAD
Author : Hong-Kai Zhao
language : en
Publisher: American Mathematical Soc.
Release Date : 2013-06-12
Mathematics In Image Processing written by Hong-Kai Zhao and has been published by American Mathematical Soc. this book supported file pdf, txt, epub, kindle and other format this book has been release on 2013-06-12 with Mathematics categories.
The theme of the 2010 PCMI Summer School was Mathematics in Image Processing in a broad sense, including mathematical theory, analysis, computation algorithms and applications. In image processing, information needs to be processed, extracted and analyzed from visual content, such as photographs or videos. These demands include standard tasks such as compression and denoising, as well as high-level understanding and analysis, such as recognition and classification. Centered on the theme of mathematics in image processing, the summer school covered quite a wide spectrum of topics in this field. The summer school is particularly timely and exciting due to the very recent advances and developments in the mathematical theory and computational methods for sparse representation. This volume collects three self-contained lecture series. The topics are multi-resolution based wavelet frames and applications to image processing, sparse and redundant representation modeling of images and simulation of elasticity, biomechanics, and virtual surgery. Recent advances in image processing, compressed sensing and sparse representation are discussed.
Handbook Of Convex Optimization Methods In Imaging Science
DOWNLOAD
Author : Vishal Monga
language : en
Publisher: Springer
Release Date : 2017-10-27
Handbook Of Convex Optimization Methods In Imaging Science written by Vishal Monga and has been published by Springer this book supported file pdf, txt, epub, kindle and other format this book has been release on 2017-10-27 with Computers categories.
This book covers recent advances in image processing and imaging sciences from an optimization viewpoint, especially convex optimization with the goal of designing tractable algorithms. Throughout the handbook, the authors introduce topics on the most key aspects of image acquisition and processing that are based on the formulation and solution of novel optimization problems. The first part includes a review of the mathematical methods and foundations required, and covers topics in image quality optimization and assessment. The second part of the book discusses concepts in image formation and capture from color imaging to radar and multispectral imaging. The third part focuses on sparsity constrained optimization in image processing and vision and includes inverse problems such as image restoration and de-noising, image classification and recognition and learning-based problems pertinent to image understanding. Throughout, convex optimization techniques are shown to be a critically important mathematical tool for imaging science problems and applied extensively. Convex Optimization Methods in Imaging Science is the first book of its kind and will appeal to undergraduate and graduate students, industrial researchers and engineers and those generally interested in computational aspects of modern, real-world imaging and image processing problems.