Methods For High Dimensional Uncertainty Quantification
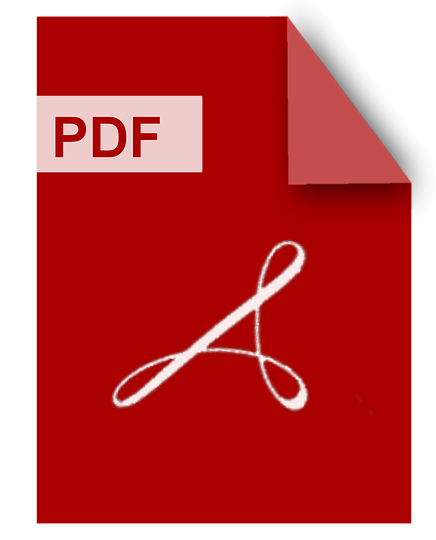
DOWNLOAD
Download Methods For High Dimensional Uncertainty Quantification PDF/ePub or read online books in Mobi eBooks. Click Download or Read Online button to get Methods For High Dimensional Uncertainty Quantification book now. This website allows unlimited access to, at the time of writing, more than 1.5 million titles, including hundreds of thousands of titles in various foreign languages. If the content not found or just blank you must refresh this page
Methods For High Dimensional Uncertainty Quantification
DOWNLOAD
Author : Gary Tang
language : en
Publisher:
Release Date : 2013
Methods For High Dimensional Uncertainty Quantification written by Gary Tang and has been published by this book supported file pdf, txt, epub, kindle and other format this book has been release on 2013 with categories.
Surrogates are used to mitigate the aggregate cost of simulation needed to perform a comprehensive uncertainty quantification (UQ) analysis. A realistic uncertainty analysis of any engineering system involves a large number of uncertainties, and as a result, the surrogates take inputs in a high dimensional space. We investigate surrogates that take the form of a truncated Legendre polynomial series, from which the coefficients associated to each polynomial basis function must be estimated. High dimensional estimation is a known instance of the curse of dimensionality, and for sufficiently "complex'" functions, an unsolved problem. In order to break the curse, we assume the function to be approximated is sparse in the Legendre polynomials and employ the machinery of l-1-regularized regression. We make three contributions under this theme. Firstly, we present a novel approach to choosing sample (design) points and show that it yields lower estimation error over a broad range of functions compared to existing sampling approaches. Secondly, we give a novel sparse estimator that effectively uses (partial) derivative information for estimation and show empirically that estimation using derivatives can be more efficient than function values if the derivatives are sparser than the function. Thirdly, we show that by exploiting the best k-term approximation} property of l-1-methods, we can quickly identify the most signfiicant uncertainties and reduce the dimensionality of the input space accordingly. We conclude by demonstrating the efficacy of these methods in a UQ analysis of a notional vertical axis wind turbine design.
Uncertainty Quantification Techniques In Statistics
DOWNLOAD
Author : Jong-Min Kim
language : en
Publisher: MDPI
Release Date : 2020-04-03
Uncertainty Quantification Techniques In Statistics written by Jong-Min Kim and has been published by MDPI this book supported file pdf, txt, epub, kindle and other format this book has been release on 2020-04-03 with Science categories.
Uncertainty quantification (UQ) is a mainstream research topic in applied mathematics and statistics. To identify UQ problems, diverse modern techniques for large and complex data analyses have been developed in applied mathematics, computer science, and statistics. This Special Issue of Mathematics (ISSN 2227-7390) includes diverse modern data analysis methods such as skew-reflected-Gompertz information quantifiers with application to sea surface temperature records, the performance of variable selection and classification via a rank-based classifier, two-stage classification with SIS using a new filter ranking method in high throughput data, an estimation of sensitive attribute applying geometric distribution under probability proportional to size sampling, combination of ensembles of regularized regression models with resampling-based lasso feature selection in high dimensional data, robust linear trend test for low-coverage next-generation sequence data controlling for covariates, and comparing groups of decision-making units in efficiency based on semiparametric regression.
Uncertainty Quantification In High Dimensional Model Selection And Inference For Regression
DOWNLOAD
Author : Juegang Hu
language : en
Publisher:
Release Date : 2012
Uncertainty Quantification In High Dimensional Model Selection And Inference For Regression written by Juegang Hu and has been published by this book supported file pdf, txt, epub, kindle and other format this book has been release on 2012 with categories.
Recent advances in $ell_1$-regularization methods have proved to be very useful for high dimensional model selection and inference. In the high dimensional regression context, the lasso and its extensions have been successfully employed to identify parsimonious sets of predictors It is well known that the lasso has the advantage of performing model selection and estimation simultaneously. It is less well understood how much uncertainty the lasso estimates may have due to small sample sizes. To model this uncertainty, we present a method, called the "contour Bayesian lasso" for the purposes of constructing joint credible regions for regression parameters. The contour Bayesian lasso is an extension of a recent approach called the "Bayesian lasso" which in turn is based on the Bayesian interpretation of the lasso. The Bayesian lasso uses a Gibbs sampler to generate from the Bayesian lasso posterior and is thus a convenient approach for quantifying uncertainty of lasso estimates. We give theoretical results regarding the optimality of the contour approach, study posterior consistency and the convergence of the Gibbs sampler. We also analyze the frequentist properties of the Bayesian lasso approach. A theoretical analysis of how the convergence of the Gibbs sampler depends on the dimensionality and sample size is undertaken. Our methodology is also illustrated on simulated and real data. We demonstrate that our posterior credible method has good coverage, and thus yields more accurate sparse solutions when the sample size is small. Real life examples are given for the South African prostate cancer data and the diabetes data set.
Uncertainty Quantification In High Dimensional Problems
DOWNLOAD
Author : Pamphile Roy
language : en
Publisher:
Release Date : 2019
Uncertainty Quantification In High Dimensional Problems written by Pamphile Roy and has been published by this book supported file pdf, txt, epub, kindle and other format this book has been release on 2019 with categories.
Uncertainties are predominant in the world that we know. Referring therefore to a nominal value is too restrictive, especially when it comes to complex systems. Understanding the nature and the impact of these uncertainties has become an important aspect of engineering work. On a societal point of view, uncertainties play a role in terms of decision-making. From the European Commission through the Better Regulation Guideline, impact assessments are now advised to take uncertainties into account. In order to understand the uncertainties, the mathematical field of uncertainty quantification has been formed. UQ encompasses a large palette of statistical tools and it seeks to link a set of input perturbations on a system (design of experiments) towards a quantity of interest. The purpose of this work is to propose improvements on various methodological aspects of uncertainty quantification applied to costly numerical simulations. This is achieved by using existing methods with a multi-strategy approach but also by creating new methods. In this context, novel sampling and resampling approaches have been developed to better capture the variability of the physical phenomenon when dealing with a high number of perturbed inputs. These allow to reduce the number of simulation required to describe the system. Moreover, novel methods are proposed to visualize uncertainties when dealing with either a high dimensional input parameter space or a high dimensional quantity of interest. The developed methods can be used in various fields like hydraulic modelling and aerodynamic modelling. Their capabilities are demonstrated in realistic systems using well established computational fluid dynamics tools. Lastly, they are not limited to the use of numerical experiments and can be used equally for real experiments.
Novel Uncertainty Quantification Methods For Stochastic Isogeometric Analysis
DOWNLOAD
Author : Ramin Jahanbin
language : en
Publisher:
Release Date : 2021
Novel Uncertainty Quantification Methods For Stochastic Isogeometric Analysis written by Ramin Jahanbin and has been published by this book supported file pdf, txt, epub, kindle and other format this book has been release on 2021 with Elasticity categories.
The main objective of this study is to develop novel computational methods for general high-dimensional uncertainty quantification (UQ) with a focus on stochastic isogeometric analysis. The objective is pursued by: (1) development of an isogeometric collocation method for random field discretization, (2) generalization of isogeometric methods for random field discretization on arbitrary multipatch domains, (3) establishment of a spline dimensional decomposition for high-dimensional UQ, (4) stochastic isogeometric analysis in linear elasticity, (5) stochastic isogeometric analysis on arbitrary multipatch domains, and (6) UQ in linear dynamical systems.
Uncertainty Quantification For Hyperbolic And Kinetic Equations
DOWNLOAD
Author : Shi Jin
language : en
Publisher: Springer
Release Date : 2018-03-20
Uncertainty Quantification For Hyperbolic And Kinetic Equations written by Shi Jin and has been published by Springer this book supported file pdf, txt, epub, kindle and other format this book has been release on 2018-03-20 with Mathematics categories.
This book explores recent advances in uncertainty quantification for hyperbolic, kinetic, and related problems. The contributions address a range of different aspects, including: polynomial chaos expansions, perturbation methods, multi-level Monte Carlo methods, importance sampling, and moment methods. The interest in these topics is rapidly growing, as their applications have now expanded to many areas in engineering, physics, biology and the social sciences. Accordingly, the book provides the scientific community with a topical overview of the latest research efforts.
Efficient Uncertainty Quantification Methodologies For High Dimensional Climate Land Models
DOWNLOAD
Author :
language : en
Publisher:
Release Date : 2011
Efficient Uncertainty Quantification Methodologies For High Dimensional Climate Land Models written by and has been published by this book supported file pdf, txt, epub, kindle and other format this book has been release on 2011 with categories.
Deep Learning Methods For High Dimensional Fluid Dynamics Problems
DOWNLOAD
Author : Pierre Jacquier
language : en
Publisher:
Release Date : 2020
Deep Learning Methods For High Dimensional Fluid Dynamics Problems written by Pierre Jacquier and has been published by this book supported file pdf, txt, epub, kindle and other format this book has been release on 2020 with categories.
Uncertainty Quantification Techniques In Statistics
DOWNLOAD
Author : Jong-Min Kim
language : en
Publisher:
Release Date : 2020
Uncertainty Quantification Techniques In Statistics written by Jong-Min Kim and has been published by this book supported file pdf, txt, epub, kindle and other format this book has been release on 2020 with Social sciences (General) categories.
Uncertainty quantification (UQ) is a mainstream research topic in applied mathematics and statistics. To identify UQ problems, diverse modern techniques for large and complex data analyses have been developed in applied mathematics, computer science, and statistics. This Special Issue of Mathematics (ISSN 2227-7390) includes diverse modern data analysis methods such as skew-reflected-Gompertz information quantifiers with application to sea surface temperature records, the performance of variable selection and classification via a rank-based classifier, two-stage classification with SIS using a new filter ranking method in high throughput data, an estimation of sensitive attribute applying geometric distribution under probability proportional to size sampling, combination of ensembles of regularized regression models with resampling-based lasso feature selection in high dimensional data, robust linear trend test for low-coverage next-generation sequence data controlling for covariates, and comparing groups of decision-making units in efficiency based on semiparametric regression.
Uncertainty Quantification In Stochastic Models For Extreme Loads
DOWNLOAD
Author : Phong The Truong Nguyen
language : en
Publisher:
Release Date : 2019
Uncertainty Quantification In Stochastic Models For Extreme Loads written by Phong The Truong Nguyen and has been published by this book supported file pdf, txt, epub, kindle and other format this book has been release on 2019 with categories.
Many response parameters for offshore structures such as wave energy converters (WECs), wind turbines, oil and gas platforms, etc. can be modeled as stochastic processes. The extreme of such a response process over any selected interval of time is a random variable. Having accurate estimates of such extremes during a structure's life is crucial in structural design, but there are challenges in their estimation due to various sources of uncertainty. These include uncertainty from environmental conditions or the climate/weather as well as from short-term simulations of these stochastic processes at appropriate time and space resolution. Together, these uncertainty sources make up a high-dimension vector of random variables (that can be on the order of thousands). Many offshore structures must withstand many years of exposure and use return periods for design that are on order of 50 to 100 years. The focus of this study is on rare events or response levels that are associated with very low probabilities of exceedance (e.g., on the order of $10−6 over a typical 1-hour duration). Time-domain simulations of dynamic offshore structures can be computationally expensive even for a single simulation. Various approaches can be adopted in practice to account for uncertainties in extreme response prediction. Monte Carlo Simulation (MCS) is the most common for exhaustive prediction of the response for all conditions. Since MCS can be computationally very demanding, the development of efficient surrogate models is presented to more efficiently deal with these uncertainties. A proposed method, in this study, is based on the use of an ensemble of multiple polynomial chaos expansion (M-PCE) surrogate models to propagate the uncertainty from the environment through the stochastic input simulation to eventual design load prediction. In particular, each PCE model in the ensemble provides an approximate relationship between the structural response and the underlying environmental variables, while variability in the short-term simulations is accounted for by the multiple surrogates. M-PCE helps overcome the curse of dimensionality since, instead of dealing with development of a high-dimensional surrogate model, the M-PCE ensemble includes multiple low-dimensional PCE models, each defined in terms of only the long-term environmental variables, which are of low dimension. It is found that the M-PCE ensemble can efficiently predict long-term extreme loads associated at exceedance probability levels (in 1 hour) of $10−5 or higher. Next, by considering MCS and M-PCE as high-fidelity and low-fidelity models, respectively, this study proposes a bi-fidelity approach that combines M-PCE and MCS outputs so as to control, or even eliminate bias introduced by the use of the M-PCE ensemble alone. The approach takes advantage of the robustness of MCS on the one hand and the efficiency of M-PCE model on the other. The key idea is that many of the model simulations are carried out using the inexpensive M-PCE ensemble while a very small number of simulations use the costly high-fidelity model. In this way, the new method significantly enhances the efficiency of MCS and improves the accuracy of the M-PCE ensemble. Finally, this dissertation explores the use of a combination of sliced inverse regression (SIR) and polynomial chaos expansion in uncertainty quantification of response extremes. The SIR procedure is adopted to reduce the original high-dimensional problem to a low-dimensional one; then, the PCE model is employed as a surrogate in the reduced-dimension space in this SIR-PCE scheme. All the proposed approaches including the M-PCE ensemble, the bi-fidelity MPCE-MCS and the SIR-PCE scheme can help mitigate the curse of dimensionality issue; thus, they are all viable approaches for probabilistic assessment of high-dimensional stochastic models, especially when predicting very rare long-term extreme response levels for offshore structures. The proposed methods are validated using examples ranging from benchmarking analytical functions to offshore structures that include studies on a maximum wave elevation, a linear single-degree-of-freedom system response, and a nonlinear wave energy converter. All the proposed methods are found to be efficient and need significantly less effort to achieve unbiased estimations of extreme response levels compared with MCS