Multi Classifiers And Decision Fusion For Robust Statistical Pattern Recognition With Applications To Hyperspectral Classification
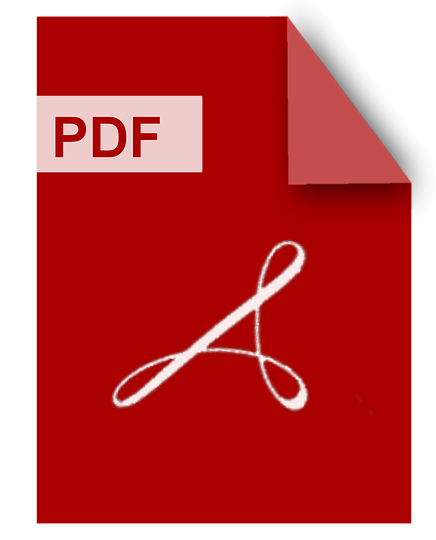
DOWNLOAD
Download Multi Classifiers And Decision Fusion For Robust Statistical Pattern Recognition With Applications To Hyperspectral Classification PDF/ePub or read online books in Mobi eBooks. Click Download or Read Online button to get Multi Classifiers And Decision Fusion For Robust Statistical Pattern Recognition With Applications To Hyperspectral Classification book now. This website allows unlimited access to, at the time of writing, more than 1.5 million titles, including hundreds of thousands of titles in various foreign languages. If the content not found or just blank you must refresh this page
Multi Classifiers And Decision Fusion For Robust Statistical Pattern Recognition With Applications To Hyperspectral Classification
DOWNLOAD
Author : Saurabh Prasad
language : en
Publisher:
Release Date : 2008
Multi Classifiers And Decision Fusion For Robust Statistical Pattern Recognition With Applications To Hyperspectral Classification written by Saurabh Prasad and has been published by this book supported file pdf, txt, epub, kindle and other format this book has been release on 2008 with Computer algorithms categories.
In this dissertation, a multi-classifier, decision fusion framework is proposed for robust classification of high dimensional data in small-sample-size conditions. Such datasets present two key challenges. (1) The high dimensional feature spaces compromise the classifiers' generalization ability in that the classifier tends to over-fit decision boundaries to the training data. This phenomenon is commonly known as the Hughes phenomenon in the pattern classification community. (2) The small-sample-size of the training data results in ill-conditioned estimates of its statistics. Most classifiers rely on accurate estimation of these statistics for modeling training data and labeling test data, and hence ill-conditioned statistical estimates result in poorer classification performance. This dissertation tests the efficacy of the proposed algorithms to classify primarily remotely sensed hyperspectral data and secondarily diagnostic digital mammograms, since these applications naturally result in very high dimensional feature spaces and often do not have sufficiently large training datasets to support the dimensionality of the feature space. Conventional approaches, such as Stepwise LDA (S-LDA) are sub-optimal, in that they utilize a small subset of the rich spectral information provided by hyperspectral data for classification. In contrast, the approach proposed in this dissertation utilizes the entire high dimensional feature space for classification by identifying a suitable partition of this space, employing a bank-of-classifiers to perform "local" classification over this partition, and then merging these local decisions using an appropriate decision fusion mechanism. Adaptive classifier weight assignment and nonlinear pre-processing (in kernel induced spaces) are also proposed within this framework to improve its robustness over a wide range of fidelity conditions. Experimental results demonstrate that the proposed framework results in significant improvements in classification accuracies (as high as a 12% increase) over conventional approaches.
Multi Classifiers And Decision Fusion For Robust Statistical Pattern Recognition With Applications To Hyperspectral Classification
DOWNLOAD
Author :
language : en
Publisher:
Release Date : 2008
Multi Classifiers And Decision Fusion For Robust Statistical Pattern Recognition With Applications To Hyperspectral Classification written by and has been published by this book supported file pdf, txt, epub, kindle and other format this book has been release on 2008 with categories.
In this dissertation, a multi-classifier, decision fusion framework is proposed for robust classification of high dimensional data in small-sample-size conditions. Such datasets present two key challenges. (1) The high dimensional feature spaces compromise the classifiers generalization ability in that the classifier tends to over-fit decision boundaries to the training data. This phenomenon is commonly known as the Hughes phenomenon in the pattern classification community. (2) The small-sample-size of the training data results in ill-conditioned estimates of its statistics. Most classifiers rely on accurate estimation of these statistics for modeling training data and labeling test data, and hence ill-conditioned statistical estimates result in poorer classification performance. This dissertation tests the efficacy of the proposed algorithms to classify primarily remotely sensed hyperspectral data and secondarily diagnostic digital mammograms, since these applications naturally result in very high dimensional feature spaces and often do not have sufficiently large training datasets to support the dimensionality of the feature space. Conventional approaches, such as Stepwise LDA (S-LDA) are sub-optimal, in that they utilize a small subset of the rich spectral information provided by hyperspectral data for classification. In contrast, the approach proposed in this dissertation utilizes the entire high dimensional feature space for classification by identifying a suitable partition of this space, employing a bank-of-classifiers to perform local classification over this partition, and then merging these local decisions using an appropriate decision fusion mechanism. Adaptive classifier weight assignment and nonlinear pre-processing (in kernel induced spaces) are also proposed within this framework to improve its robustness over a wide range of fidelity conditions. Experimental results demonstrate that the proposed framework results in significant improvements in classi.
Multiple Classifier Systems
DOWNLOAD
Author : Jón Atli Benediktsson
language : en
Publisher: Springer
Release Date : 2009-06-10
Multiple Classifier Systems written by Jón Atli Benediktsson and has been published by Springer this book supported file pdf, txt, epub, kindle and other format this book has been release on 2009-06-10 with Computers categories.
These proceedings are a record of the Multiple Classi?er Systems Workshop, MCS 2009, held at the University of Iceland, Reykjavik, Iceland in June 2009. Being the eighth in a well-established series of meetings providing an inter- tional forum for the discussion of issues in multiple classi?er system design, the workshop achieved its objective of bringing together researchers from diverse communities (neural networks,pattern recognition,machine learning and stat- tics) concerned with this research topic. From more than 70 submissions, the Program Committee selected 54 papers to create an interesting scienti?c program. The special focus of MCS 2009 was on the application of multiple classi?er systems in remote sensing. This part- ular application uses multiple classi?ers for raw data fusion, feature level fusion and decision level fusion. In addition to the excellent regular submission in the technical program, outstanding contributions were made by invited speakers Melba Crawford from Purdue University and Zhi-Hua Zhou of Nanjing Univ- sity. Papers of these talks are included in these workshop proceedings. With the workshop’sapplicationfocusbeingonremotesensing,Prof.Crawford’sexpertise in the use of multiple classi?cation systems in this context made the discussions on this topic at MCS 2009 particularly fruitful.
Multiple Classifier Systems
DOWNLOAD
Author : Terry Windeatt
language : en
Publisher: Springer Science & Business Media
Release Date : 2003-05-27
Multiple Classifier Systems written by Terry Windeatt and has been published by Springer Science & Business Media this book supported file pdf, txt, epub, kindle and other format this book has been release on 2003-05-27 with Business & Economics categories.
This book constitutes the refereed proceedings of the 4th International Workshop on Multiple Classifier Systems, MCS 2003, held in Guildford, UK in June 2003. The 40 revised full papers presented with one invited paper were carefully reviewed and selected for presentation. The papers are organized in topical sections on boosting, combination rules, multi-class methods, fusion schemes and architectures, neural network ensembles, ensemble strategies, and applications
Optical Remote Sensing
DOWNLOAD
Author : Saurabh Prasad
language : en
Publisher: Springer Science & Business Media
Release Date : 2011-03-23
Optical Remote Sensing written by Saurabh Prasad and has been published by Springer Science & Business Media this book supported file pdf, txt, epub, kindle and other format this book has been release on 2011-03-23 with Technology & Engineering categories.
Optical remote sensing relies on exploiting multispectral and hyper spectral imagery possessing high spatial and spectral resolutions respectively. These modalities, although useful for most remote sensing tasks, often present challenges that must be addressed for their effective exploitation. This book presents current state-of-the-art algorithms that address the following key challenges encountered in representation and analysis of such optical remotely sensed data. Challenges in pre-processing images, storing and representing high dimensional data, fusing different sensor modalities, pattern classification and target recognition, visualization of high dimensional imagery.
Multiple Classifier Systems
DOWNLOAD
Author : Fabio Roli
language : en
Publisher: Springer
Release Date : 2004-09-21
Multiple Classifier Systems written by Fabio Roli and has been published by Springer this book supported file pdf, txt, epub, kindle and other format this book has been release on 2004-09-21 with Computers categories.
The fusion of di?erent information sourcesis a persistent and intriguing issue. It hasbeenaddressedforcenturiesinvariousdisciplines,includingpoliticalscience, probability and statistics, system reliability assessment, computer science, and distributed detection in communications. Early seminal work on fusion was c- ried out by pioneers such as Laplace and von Neumann. More recently, research activities in information fusion have focused on pattern recognition. During the 1990s,classi?erfusionschemes,especiallyattheso-calleddecision-level,emerged under a plethora of di?erent names in various scienti?c communities, including machine learning, neural networks, pattern recognition, and statistics. The d- ferent nomenclatures introduced by these communities re?ected their di?erent perspectives and cultural backgrounds as well as the absence of common forums and the poor dissemination of the most important results. In 1999, the ?rst workshop on multiple classi?er systems was organized with the main goal of creating a common international forum to promote the diss- ination of the results achieved in the diverse communities and the adoption of a common terminology, thus giving the di?erent perspectives and cultural ba- grounds some concrete added value. After ?ve meetings of this workshop, there is strong evidence that signi?cant steps have been made towards this goal. - searchers from these diverse communities successfully participated in the wo- shops, and world experts presented surveys of the state of the art from the perspectives of their communities to aid cross-fertilization.
Classification Methods For Remotely Sensed Data
DOWNLOAD
Author : Taskin Kavzoglu
language : en
Publisher: CRC Press
Release Date : 2024-09-04
Classification Methods For Remotely Sensed Data written by Taskin Kavzoglu and has been published by CRC Press this book supported file pdf, txt, epub, kindle and other format this book has been release on 2024-09-04 with Technology & Engineering categories.
The third edition of the bestselling Classification Methods for Remotely Sensed Data covers current state-of-the-art machine learning algorithms and developments in the analysis of remotely sensed data. This book is thoroughly updated to meet the needs of readers today and provides six new chapters on deep learning, feature extraction and selection, multisource image fusion, hyperparameter optimization, accuracy assessment with model explainability, and object-based image analysis, which is relatively a new paradigm in image processing and classification. It presents new AI-based analysis tools and metrics together with ongoing debates on accuracy assessment strategies and XAI methods. New in this edition: Provides comprehensive background on the theory of deep learning and its application to remote sensing data. Includes a chapter on hyperparameter optimization techniques to guarantee the highest performance in classification applications. Outlines the latest strategies and accuracy measures in accuracy assessment and summarizes accuracy metrics and assessment strategies. Discusses the methods used for explaining inherent structures and weighing the features of ML and AI algorithms that are critical for explaining the robustness of the models. This book is intended for industry professionals, researchers, academics, and graduate students who want a thorough and up-to-date guide to the many and varied techniques of image classification applied in the fields of geography, geospatial and earth sciences, electronic and computer science, environmental engineering, etc.
Multi Class Classification With Machine Learning And Fusion
DOWNLOAD
Author : Cristina Garcia Cifuentes
language : en
Publisher:
Release Date : 2009
Multi Class Classification With Machine Learning And Fusion written by Cristina Garcia Cifuentes and has been published by this book supported file pdf, txt, epub, kindle and other format this book has been release on 2009 with categories.
Multi-class classification is the core issue of many pattern recognition tasks. Several applications require high-end machine learning solutions to provide satisfying results in operational contexts. However, most efficient ones, like SVM or Boosting, are generally mono-class, which introduces the problem of translating a global multi-class problem is several binary problems, while still being able to provide at the end an answer to the original multi-class issue. Present work aims at providing a solution to this multi-class problematic, by introducing a complete framework with a strong probabilistic and structured basis. It includes the study of error correcting output codes correlated with the definition of an optimal subdivision of the multi-class issue in several binary problems, in a complete automatic way. Machine learning algorithms are studied and benchmarked to facilitate and justify the final selection. Coupling of automatically calibrated classifiers output is obtained by applying iterative constrained regularisations, and a logical temporal fusion is applied on temporal-redundant data (like tracked vehicles) to enhance performances. Finally, ranking scores are computed to optimize precision and recall is ranking-based systems. Each step of the previously described system has been analysed from a theoretical an empirical point of view and new contributions are introduced, so as to obtain a complete mathematically coherent framework which is both generic and easy-to-use, as the learning procedure is almost completely automatic. On top of that, quantitative evaluations on two completely different datasets have assessed both the exactitude of previous assertions and the improvements that were achieved compared to previous methods.
Pattern Classification
DOWNLOAD
Author : Richard O. Duda
language : en
Publisher: John Wiley & Sons
Release Date : 2012-11-09
Pattern Classification written by Richard O. Duda and has been published by John Wiley & Sons this book supported file pdf, txt, epub, kindle and other format this book has been release on 2012-11-09 with Technology & Engineering categories.
The first edition, published in 1973, has become a classicreference in the field. Now with the second edition, readers willfind information on key new topics such as neural networks andstatistical pattern recognition, the theory of machine learning,and the theory of invariances. Also included are worked examples,comparisons between different methods, extensive graphics, expandedexercises and computer project topics. An Instructor's Manual presenting detailed solutions to all theproblems in the book is available from the Wiley editorialdepartment.
Hyperspectral Image Analysis
DOWNLOAD
Author : Saurabh Prasad
language : en
Publisher: Springer Nature
Release Date : 2020-04-27
Hyperspectral Image Analysis written by Saurabh Prasad and has been published by Springer Nature this book supported file pdf, txt, epub, kindle and other format this book has been release on 2020-04-27 with Computers categories.
This book reviews the state of the art in algorithmic approaches addressing the practical challenges that arise with hyperspectral image analysis tasks, with a focus on emerging trends in machine learning and image processing/understanding. It presents advances in deep learning, multiple instance learning, sparse representation based learning, low-dimensional manifold models, anomalous change detection, target recognition, sensor fusion and super-resolution for robust multispectral and hyperspectral image understanding. It presents research from leading international experts who have made foundational contributions in these areas. The book covers a diverse array of applications of multispectral/hyperspectral imagery in the context of these algorithms, including remote sensing, face recognition and biomedicine. This book would be particularly beneficial to graduate students and researchers who are taking advanced courses in (or are working in) the areas of image analysis, machine learning and remote sensing with multi-channel optical imagery. Researchers and professionals in academia and industry working in areas such as electrical engineering, civil and environmental engineering, geosciences and biomedical image processing, who work with multi-channel optical data will find this book useful.