Neural Architecture
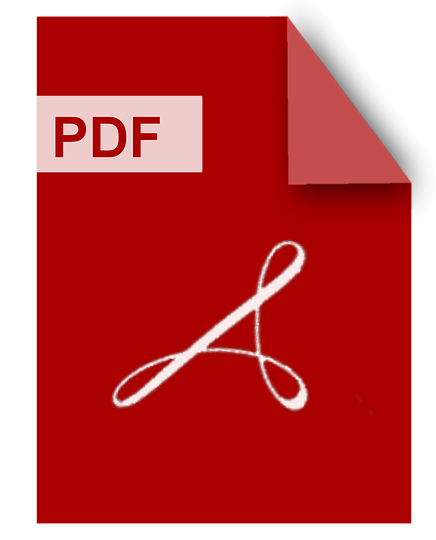
DOWNLOAD
Download Neural Architecture PDF/ePub or read online books in Mobi eBooks. Click Download or Read Online button to get Neural Architecture book now. This website allows unlimited access to, at the time of writing, more than 1.5 million titles, including hundreds of thousands of titles in various foreign languages. If the content not found or just blank you must refresh this page
How To Build A Brain
DOWNLOAD
Author : Chris Eliasmith
language : en
Publisher: Oxford University Press
Release Date : 2013-04-16
How To Build A Brain written by Chris Eliasmith and has been published by Oxford University Press this book supported file pdf, txt, epub, kindle and other format this book has been release on 2013-04-16 with Psychology categories.
How to Build a Brain provides a detailed exploration of a new cognitive architecture - the Semantic Pointer Architecture - that takes biological detail seriously, while addressing cognitive phenomena. Topics ranging from semantics and syntax, to neural coding and spike-timing-dependent plasticity are integrated to develop the world's largest functional brain model.
Evolutionary Deep Neural Architecture Search Fundamentals Methods And Recent Advances
DOWNLOAD
Author : Yanan Sun
language : en
Publisher: Springer Nature
Release Date : 2022-11-08
Evolutionary Deep Neural Architecture Search Fundamentals Methods And Recent Advances written by Yanan Sun and has been published by Springer Nature this book supported file pdf, txt, epub, kindle and other format this book has been release on 2022-11-08 with Technology & Engineering categories.
This book systematically narrates the fundamentals, methods, and recent advances of evolutionary deep neural architecture search chapter by chapter. This will provide the target readers with sufficient details learning from scratch. In particular, the method parts are devoted to the architecture search of unsupervised and supervised deep neural networks. The people, who would like to use deep neural networks but have no/limited expertise in manually designing the optimal deep architectures, will be the main audience. This may include the researchers who focus on developing novel evolutionary deep architecture search methods for general tasks, the students who would like to study the knowledge related to evolutionary deep neural architecture search and perform related research in the future, and the practitioners from the fields of computer vision, natural language processing, and others where the deep neural networks have been successfully and largely used in their respective fields.
New Perspectives In Neural Architecture Search
DOWNLOAD
Author : Shen Yan
language : en
Publisher:
Release Date : 2022
New Perspectives In Neural Architecture Search written by Shen Yan and has been published by this book supported file pdf, txt, epub, kindle and other format this book has been release on 2022 with Electronic dissertations categories.
Neural architecture search (NAS) has made significant strides in recent years, but challenges remain in terms of the stability of search performance and the high computational requirements of sampling-based NAS. Studying architecture representations offers a promising solution to these challenges, as it encourages neural architectures with similar structures or computations to cluster together. This helps to map neural architectures with similar performance to the same regions in the latent space and leads to smoother transitions in the latent space, benefiting downstream search. Additionally, learning curve extrapolation can accelerate the search process by estimating the final validation accuracy of a neural network from the learning curve of a partially trained network. Overall, understanding the neural architecture representations and their associated learning curves through theoretical analysis and empirical evaluations is crucial for achieving stable and scalable NAS.This dissertation presents our contributions to the field of neural architecture search (NAS), which push the limits of NAS and achieve state-of-the-art performance. Our contributions include efficient one-shot NAS via hierarchical masking, addressing the joint optimization problem of architecture representations and search through unsupervised pre-training, improving the generalization ability of architecture representations with computation-aware self-supervised training, and developing a method for facilitating multi-fidelity NAS research.
Automated Machine Learning
DOWNLOAD
Author : Adnan Masood
language : en
Publisher: Packt Publishing
Release Date : 2021-02-18
Automated Machine Learning written by Adnan Masood and has been published by Packt Publishing this book supported file pdf, txt, epub, kindle and other format this book has been release on 2021-02-18 with categories.
Get to grips with automated machine learning and adopt a hands-on approach to AutoML implementation and associated methodologies Key Features: Get up to speed with AutoML using OSS, Azure, AWS, GCP, or any platform of your choice Eliminate mundane tasks in data engineering and reduce human errors in machine learning models Find out how you can make machine learning accessible for all users to promote decentralized processes Book Description: Every machine learning engineer deals with systems that have hyperparameters, and the most basic task in automated machine learning (AutoML) is to automatically set these hyperparameters to optimize performance. The latest deep neural networks have a wide range of hyperparameters for their architecture, regularization, and optimization, which can be customized effectively to save time and effort. This book reviews the underlying techniques of automated feature engineering, model and hyperparameter tuning, gradient-based approaches, and much more. You'll discover different ways of implementing these techniques in open source tools and then learn to use enterprise tools for implementing AutoML in three major cloud service providers: Microsoft Azure, Amazon Web Services (AWS), and Google Cloud Platform. As you progress, you'll explore the features of cloud AutoML platforms by building machine learning models using AutoML. The book will also show you how to develop accurate models by automating time-consuming and repetitive tasks in the machine learning development lifecycle. By the end of this machine learning book, you'll be able to build and deploy AutoML models that are not only accurate, but also increase productivity, allow interoperability, and minimize feature engineering tasks. What You Will Learn: Explore AutoML fundamentals, underlying methods, and techniques Assess AutoML aspects such as algorithm selection, auto featurization, and hyperparameter tuning in an applied scenario Find out the difference between cloud and operations support systems (OSS) Implement AutoML in enterprise cloud to deploy ML models and pipelines Build explainable AutoML pipelines with transparency Understand automated feature engineering and time series forecasting Automate data science modeling tasks to implement ML solutions easily and focus on more complex problems Who this book is for: Citizen data scientists, machine learning developers, artificial intelligence enthusiasts, or anyone looking to automatically build machine learning models using the features offered by open source tools, Microsoft Azure Machine Learning, AWS, and Google Cloud Platform will find this book useful. Beginner-level knowledge of building ML models is required to get the best out of this book. Prior experience in using Enterprise cloud is beneficial.
The Neural Architecture Of Grammar
DOWNLOAD
Author : Stephen E. Nadeau
language : en
Publisher: MIT Press
Release Date : 2012
The Neural Architecture Of Grammar written by Stephen E. Nadeau and has been published by MIT Press this book supported file pdf, txt, epub, kindle and other format this book has been release on 2012 with Language Arts & Disciplines categories.
A comprehensive, neurally based theory of language function that draws on principles of neuroanatomy, cognitive psychology, cognitive neuropsychology, psycholinguistics, and parallel distributed processing. Linguists have mapped the topography of language behavior in many languages in intricate detail. To understand how the brain supports language function, however, we must take into account the principles and regularities of neural function. Mechanisms of neurolinguistic function cannot be inferred solely from observations of normal and impaired language. In The Neural Architecture of Grammar, Stephen Nadeau develops a neurologically plausible theory of grammatic function. He brings together principles of neuroanatomy, neurophysiology, and parallel distributed processing and draws on literature on language function from cognitive psychology, cognitive neuropsychology, psycholinguistics, and functional imaging to develop a comprehensive neurally based theory of language function. Nadeau reviews the aphasia literature, including cross-linguistic aphasia research, to test the model's ability to account for the findings of these empirical studies. Nadeau finds that the model readily accounts for a crucial finding in cross-linguistic studies--that the most powerful determinant of patterns of language breakdown in aphasia is the predisorder language spoken by the subject--and that it does so by conceptualizing grammatic function in terms of the statistical regularities of particular languages that are encoded in network connectivity. He shows that the model provides a surprisingly good account for many findings and offers solutions for a number of controversial problems. Moreover, aphasia studies provide the basis for elaborating the model in interesting and important ways.
Neural Architecture
DOWNLOAD
Author : Matias del Campo
language : en
Publisher: Applied Research & Design
Release Date : 2022-03-14
Neural Architecture written by Matias del Campo and has been published by Applied Research & Design this book supported file pdf, txt, epub, kindle and other format this book has been release on 2022-03-14 with categories.
This book explores the interdisciplinary project that brings the long tradition of humanistic inquiry in architecture together with cutting-edge research in artificial intelligence. The main goal of Neural Architecture is to understand how to interrogate artificial intelligence - a technological tool - in the field of architectural design, traditionally a practice that combines humanities and visual arts. Matias del Campo, the author of Neural Architecture is currently exploring specific applications of artificial intelligence in contemporary architecture, focusing on their relationship to material and symbolic culture. AI has experienced an explosive growth in recent years in a range of fields including architecture but its implications for the humanistic values that distinguish architecture from technology have yet to be measured. The book illustrates in a series of projects a set of crucial questions for the development of architecture in the future. An opportunity to survey the emerging field of Architecture and Artificial Intelligence, and to reflect on the implications of a world increasingly entangled in questions of the agency, culture and ethics of AI.
Efficient Neural Architecture Search With Multiobjective Evolutionary Optimization
DOWNLOAD
Author : Maria Gabriela Baldeón Calisto
language : en
Publisher:
Release Date : 2020
Efficient Neural Architecture Search With Multiobjective Evolutionary Optimization written by Maria Gabriela Baldeón Calisto and has been published by this book supported file pdf, txt, epub, kindle and other format this book has been release on 2020 with Diagnostic imaging categories.
Deep neural networks have become very successful at solving many complex tasks such as image classification, image segmentation, and speech recognition. These models are composed of multiple layers that have the capacity to learn increasingly higher-level features, without prior handcrafted specifications. However, the success of a deep neural network relies on finding the proper configuration for the task in hand. Given the vast number of hyperparameters and the massive search space, manually designing or fine-tuning deep learning architectures requires extensive knowledge, time, and computational resources. There is a growing interest in developing methods that automatically design a neural network ́s architecture, known as neural architecture search (NAS). NAS is usually modeled as a single-objective optimization problem where the aim is to find an architecture that maximizes the prediction ́s accuracy. However, most deep learning applications require accurate as well as efficient architectures to reduce memory consumption and enable their use in computationally-limited environments. This has led to the need to model NAS as a multiple objective problem that optimizes both the predictive performance and efficiency of the network. Furthermore, most NAS framework have focused on either optimizing the micro-structure (structure of the basic cell), or macro-structure (optimal number of cells and their connection) of the architecture. Consequently, manual engineering is required to find the topology of the non-optimized structure. Although NAS has demonstrated great potential in automatically designing an architecture, it remains a computationally expensive and time-consuming process because it requires training and evaluating many potential configurations. Recent work has focused on improving the search time of NAS algorithms, but most techniques have been developed and applied only for single-objective optimization problems. Given that optimizing multiple objectives has a higher complexity and requires more iterations to approximate the Pareto Front, it is critical to investigate algorithms that decrease the search time of multiobjective NAS. One critical application of deep learning is medical image segmentation. Segmentation of medical images provides valuable information for various critical tasks such as analyzing anatomical structures, monitoring disease progression, and predicting patient outcomes. Nonetheless, achieving accurate segmentation is challenging due to the inherent variability in appearance, shape, and location of the region of interest (ROI) between patients and the differences in imagining equipment and acquisition protocols. Therefore, neural networks are usually tailored to a specific application, anatomical region, and image modality. Moreover, medical image data is often volumetric requiring expensive 3D operations that result in large and complex architectures. Hence, training and deploying them requires considerable storage and memory bandwidth that makes them less suitable for clinical applications. To overcome these challenges, the main goal of this research is to automatically design accurate and efficient deep neural networks using multiobjective optimization algorithms for medical image segmentation. The proposed research consists of three major objectives: (1) to design a deep neural network that uses a multiobjective evolutionary based algorithm to automatically adapt to different medical image datasets while minimizing the model’s size; (2) to design a self-adaptive 2D-3D Fully Convolutional network (FCN) ensemble that incorporates volumetric information and optimizes both the performance and the size of the architecture; and (3) to design an efficient multiobjective neural architecture search framework that decreases the search time while simultaneously optimizing the micro- and macro-structure of the neural architecture. For the first objective, a multiobjective adaptive convolutional neural network named AdaResU-Net is presented for 2D medical image segmentation. The proposed AdaResU-Net is comprised of a fixed architecture and a learning framework that adjusts the hyperparameters to a particular training dataset using a multiobjective evolutionary based algorithm (MEA algorithm). The MEA algorithm evolves the AdaResU-Net network to optimize both the segmentation accuracy and model size. In the second objective, a self-adaptive ensemble of 2D-3D FCN named AdaEn-Net is proposed for 3D medical image segmentation. The AdaEn-Net is comprised of a 2D FCN that extracts intra-slice and long-range 2D context, and a 3D FCN architecture that exploits inter-slice and volumetric information. The 2D and 3D FCN architectures are automatically fitted for a specific medical image segmentation task by simultaneously optimizing the expected segmentation error and size of the network using the MEA algorithm. Finally, for the third objective, an efficient multiobjective neural architecture search framework named EMONAS is presented for 3D medical image segmentation. EMONAS has two main components, a novel search space that includes the hyperparameters that define the micro- and macro-structure of the architecture, and a Surrogate-assisted multiobjective evolutionary based algorithm (SaMEA algorithm) that efficiently searches for the best hyperparameter values using a Random Forest surrogate and guiding selection probabilities. The broader impact of the proposed research is as follows: (1) automating the design of deep neural networks’ architecture and hyperparameters to improve the performance and efficiency of the models; and (2) increase the accessibility of deep learning to a broader range of organizations and people by reducing the need of expert knowledge and GPU time when automatically designing deep neural networks. In the medical area, the proposed models aim to improve the automatic extraction of data from medical images to potentially enhance diagnosis, treatment planning and survival prediction of various diseases such as cardiac disease and prostate cancer. Although the proposed techniques are applied to medical image segmentation tasks, they can also be implemented in other applications where accurate and resource-efficient deep neural networks are needed such as autonomous navigation, augmented reality and internet-of-things.
Neural Computing Architectures
DOWNLOAD
Author : Igor Aleksander
language : en
Publisher:
Release Date : 1989
Neural Computing Architectures written by Igor Aleksander and has been published by this book supported file pdf, txt, epub, kindle and other format this book has been release on 1989 with Artificial intelligence categories.
Efficient Neural Architecture Generation With An Invertible Neural Network For Neural Architecture Search
DOWNLOAD
Author : 陳冠頴
language : en
Publisher:
Release Date : 2023
Efficient Neural Architecture Generation With An Invertible Neural Network For Neural Architecture Search written by 陳冠頴 and has been published by this book supported file pdf, txt, epub, kindle and other format this book has been release on 2023 with categories.
Target Aware Neural Architecture Search Coupled With Channel Pruning
DOWNLOAD
Author :
language : en
Publisher:
Release Date : 2019
Target Aware Neural Architecture Search Coupled With Channel Pruning written by and has been published by this book supported file pdf, txt, epub, kindle and other format this book has been release on 2019 with categories.