New Models And Techniques On Privacy Preserving Information Sharing
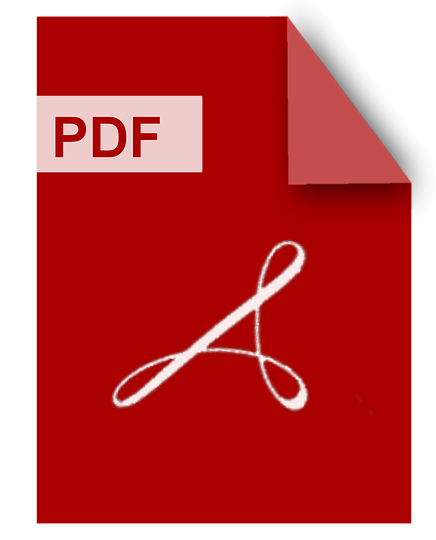
DOWNLOAD
Download New Models And Techniques On Privacy Preserving Information Sharing PDF/ePub or read online books in Mobi eBooks. Click Download or Read Online button to get New Models And Techniques On Privacy Preserving Information Sharing book now. This website allows unlimited access to, at the time of writing, more than 1.5 million titles, including hundreds of thousands of titles in various foreign languages. If the content not found or just blank you must refresh this page
New Models And Techniques On Privacy Preserving Information Sharing
DOWNLOAD
Author : Yabo Xu
language : en
Publisher:
Release Date : 2008
New Models And Techniques On Privacy Preserving Information Sharing written by Yabo Xu and has been published by this book supported file pdf, txt, epub, kindle and other format this book has been release on 2008 with Computer networks categories.
Due to the wide deployment of Internet and information technology, the ever growing privacy concern has been a major obstacle for information sharing. This thesis work thus centres on developing new models and techniques to deal with emerging privacy issues in various contexts of information sharing and exchange. Specifically, along with the main theme, this thesis work can be divided into three categories, summarized as follows. The first problem is privacy-preserving data mining spanning multiple private data sources. The goal of this research is to enable the computation as the data collected in a central place, but preserve the privacy of participating sites. This problem has been studied in the context of classification with multiple private data sources integrated with join semantics. The second problem privacy-preserving data publishing. This research aims to address the scenario where a data owner wishes to publish the data while preserving individual privacy. This topic has been extensively studied in the context of relational data, but much less is known for transaction data. We propose one way to address this issue in this thesis. The third problem is privacy-enhancing online personalized service. This research starts from an end user's point of view, and studies how to submit a piece of personal data to exchange for service without compromising individual privacy. Our contribution on this topic is a framework under which individual users can strike a balance between service quality and privacy protection.
Introduction To Privacy Preserving Data Publishing
DOWNLOAD
Author : Benjamin C.M. Fung
language : en
Publisher: CRC Press
Release Date : 2010-08-02
Introduction To Privacy Preserving Data Publishing written by Benjamin C.M. Fung and has been published by CRC Press this book supported file pdf, txt, epub, kindle and other format this book has been release on 2010-08-02 with Computers categories.
Gaining access to high-quality data is a vital necessity in knowledge-based decision making. But data in its raw form often contains sensitive information about individuals. Providing solutions to this problem, the methods and tools of privacy-preserving data publishing enable the publication of useful information while protecting data privacy. Int
Linking Sensitive Data
DOWNLOAD
Author : Peter Christen
language : en
Publisher:
Release Date : 2020
Linking Sensitive Data written by Peter Christen and has been published by this book supported file pdf, txt, epub, kindle and other format this book has been release on 2020 with Computer security categories.
This book provides modern technical answers to the legal requirements of pseudonymisation as recommended by privacy legislation. It covers topics such as modern regulatory frameworks for sharing and linking sensitive information, concepts and algorithms for privacy-preserving record linkage and their computational aspects, practical considerations such as dealing with dirty and missing data, as well as privacy, risk, and performance assessment measures. Existing techniques for privacy-preserving record linkage are evaluated empirically and real-world application examples that scale to population sizes are described. The book also includes pointers to freely available software tools, benchmark data sets, and tools to generate synthetic data that can be used to test and evaluate linkage techniques. This book consists of fourteen chapters grouped into four parts, and two appendices. The first part introduces the reader to the topic of linking sensitive data, the second part covers methods and techniques to link such data, the third part discusses aspects of practical importance, and the fourth part provides an outlook of future challenges and open research problems relevant to linking sensitive databases. The appendices provide pointers and describe freely available, open-source software systems that allow the linkage of sensitive data, and provide further details about the evaluations presented. A companion Web site at https://dmm.anu.edu.au/lsdbook2020 provides additional material and Python programs used in the book. This book is mainly written for applied scientists, researchers, and advanced practitioners in governments, industry, and universities who are concerned with developing, implementing, and deploying systems and tools to share sensitive information in administrative, commercial, or medical databases. The Book describes how linkage methods work and how to evaluate their performance. It covers all the major concepts and methods and also discusses practical matters such as computational efficiency, which are critical if the methods are to be used in practice - and it does all this in a highly accessible way! David J. Hand, Imperial College, London.
Privacy Preserving Data Mining
DOWNLOAD
Author : Charu C. Aggarwal
language : en
Publisher: Springer Science & Business Media
Release Date : 2008-06-10
Privacy Preserving Data Mining written by Charu C. Aggarwal and has been published by Springer Science & Business Media this book supported file pdf, txt, epub, kindle and other format this book has been release on 2008-06-10 with Computers categories.
Advances in hardware technology have increased the capability to store and record personal data. This has caused concerns that personal data may be abused. This book proposes a number of techniques to perform the data mining tasks in a privacy-preserving way. This edited volume contains surveys by distinguished researchers in the privacy field. Each survey includes the key research content as well as future research directions of a particular topic in privacy. The book is designed for researchers, professors, and advanced-level students in computer science, but is also suitable for practitioners in industry.
The Ethics Of Cybersecurity
DOWNLOAD
Author : Markus Christen
language : en
Publisher: Springer Nature
Release Date : 2020-02-10
The Ethics Of Cybersecurity written by Markus Christen and has been published by Springer Nature this book supported file pdf, txt, epub, kindle and other format this book has been release on 2020-02-10 with Philosophy categories.
This open access book provides the first comprehensive collection of papers that provide an integrative view on cybersecurity. It discusses theories, problems and solutions on the relevant ethical issues involved. This work is sorely needed in a world where cybersecurity has become indispensable to protect trust and confidence in the digital infrastructure whilst respecting fundamental values like equality, fairness, freedom, or privacy. The book has a strong practical focus as it includes case studies outlining ethical issues in cybersecurity and presenting guidelines and other measures to tackle those issues. It is thus not only relevant for academics but also for practitioners in cybersecurity such as providers of security software, governmental CERTs or Chief Security Officers in companies.
Privacy Aware Knowledge Discovery
DOWNLOAD
Author : Francesco Bonchi
language : en
Publisher: CRC Press
Release Date : 2010-12-02
Privacy Aware Knowledge Discovery written by Francesco Bonchi and has been published by CRC Press this book supported file pdf, txt, epub, kindle and other format this book has been release on 2010-12-02 with Computers categories.
Covering research at the frontier of this field, Privacy-Aware Knowledge Discovery: Novel Applications and New Techniques presents state-of-the-art privacy-preserving data mining techniques for application domains, such as medicine and social networks, that face the increasing heterogeneity and complexity of new forms of data. Renowned authorities
Privacy Preserving Machine Learning
DOWNLOAD
Author : J. Morris Chang
language : en
Publisher: Simon and Schuster
Release Date : 2023-05-23
Privacy Preserving Machine Learning written by J. Morris Chang and has been published by Simon and Schuster this book supported file pdf, txt, epub, kindle and other format this book has been release on 2023-05-23 with Computers categories.
Keep sensitive user data safe and secure without sacrificing the performance and accuracy of your machine learning models. In Privacy Preserving Machine Learning, you will learn: Privacy considerations in machine learning Differential privacy techniques for machine learning Privacy-preserving synthetic data generation Privacy-enhancing technologies for data mining and database applications Compressive privacy for machine learning Privacy-Preserving Machine Learning is a comprehensive guide to avoiding data breaches in your machine learning projects. You’ll get to grips with modern privacy-enhancing techniques such as differential privacy, compressive privacy, and synthetic data generation. Based on years of DARPA-funded cybersecurity research, ML engineers of all skill levels will benefit from incorporating these privacy-preserving practices into their model development. By the time you’re done reading, you’ll be able to create machine learning systems that preserve user privacy without sacrificing data quality and model performance. About the Technology Machine learning applications need massive amounts of data. It’s up to you to keep the sensitive information in those data sets private and secure. Privacy preservation happens at every point in the ML process, from data collection and ingestion to model development and deployment. This practical book teaches you the skills you’ll need to secure your data pipelines end to end. About the Book Privacy-Preserving Machine Learning explores privacy preservation techniques through real-world use cases in facial recognition, cloud data storage, and more. You’ll learn about practical implementations you can deploy now, future privacy challenges, and how to adapt existing technologies to your needs. Your new skills build towards a complete security data platform project you’ll develop in the final chapter. What’s Inside Differential and compressive privacy techniques Privacy for frequency or mean estimation, naive Bayes classifier, and deep learning Privacy-preserving synthetic data generation Enhanced privacy for data mining and database applications About the Reader For machine learning engineers and developers. Examples in Python and Java. About the Author J. Morris Chang is a professor at the University of South Florida. His research projects have been funded by DARPA and the DoD. Di Zhuang is a security engineer at Snap Inc. Dumindu Samaraweera is an assistant research professor at the University of South Florida. The technical editor for this book, Wilko Henecka, is a senior software engineer at Ambiata where he builds privacy-preserving software. Table of Contents PART 1 - BASICS OF PRIVACY-PRESERVING MACHINE LEARNING WITH DIFFERENTIAL PRIVACY 1 Privacy considerations in machine learning 2 Differential privacy for machine learning 3 Advanced concepts of differential privacy for machine learning PART 2 - LOCAL DIFFERENTIAL PRIVACY AND SYNTHETIC DATA GENERATION 4 Local differential privacy for machine learning 5 Advanced LDP mechanisms for machine learning 6 Privacy-preserving synthetic data generation PART 3 - BUILDING PRIVACY-ASSURED MACHINE LEARNING APPLICATIONS 7 Privacy-preserving data mining techniques 8 Privacy-preserving data management and operations 9 Compressive privacy for machine learning 10 Putting it all together: Designing a privacy-enhanced platform (DataHub)
Data Transformation For Privacy Preserving Data Mining
DOWNLOAD
Author : Stanley Robson de Medeiros Oliveira
language : en
Publisher: Library and Archives Canada = Bibliothèque et Archives Canada
Release Date : 2005
Data Transformation For Privacy Preserving Data Mining written by Stanley Robson de Medeiros Oliveira and has been published by Library and Archives Canada = Bibliothèque et Archives Canada this book supported file pdf, txt, epub, kindle and other format this book has been release on 2005 with Data mining categories.
The sharing of data is often beneficial in data mining applications. It has been proven useful to support both decision-making processes and to promote social goals. However, the sharing of data has also raised a number of ethical issues. Some such issues include those of privacy, data security, and intellectual property rights. In this thesis, we focus primarily on privacy issues in data mining, notably when data are shared before mining. Specifically, we consider some scenarios in which applications of association rule mining and data clustering require privacy safeguards. Addressing privacy preservation in such scenarios is complex. One must not only meet privacy requirements but also guarantee valid data rnining results. This status indicates the pressing need for rethinking mechanisnis to enforce privacy safeguards without losing the benefit of mining. These mechanisms can lead to new privacy control methods to convert a database into a new one in such a waY as to preserve the main features of the original database for mining. In particular, we address the problem of transforming a database to be shared into a new one that conceals private information while preserving the general patterrns and trends from the original database. To address this challening problem, we propose a unified framework for privacy-preserving data mining that ensures that the mining process will not violate privacy up to a certain degree of security. The frarnework encompasses a family of privacy-preserving data transformation rnethods, a library of algoritImis, retrieval facilities to speed up the transformation process, and a set of metrics to evaluate the effectiveness of the proposed algorithms, in terms of information loss, and to quantify how much private information has been disclosed. Our investigation concludes that privacy-preserving data mining is to some extent possible. We demonstrate empirically and tlleoretically the practicality and feasibility of achieving privacy preservation in data mining. Our experiments reveal that our framework is efféctive, meets privacy requírements. and guarantees valid data mining results while protecting sensitive information (e.g., sensitive knowIedge and individuals' privacy).
Privacy Preserving Machine Learning
DOWNLOAD
Author : Jin Li
language : en
Publisher: Springer Nature
Release Date : 2022-03-14
Privacy Preserving Machine Learning written by Jin Li and has been published by Springer Nature this book supported file pdf, txt, epub, kindle and other format this book has been release on 2022-03-14 with Computers categories.
This book provides a thorough overview of the evolution of privacy-preserving machine learning schemes over the last ten years, after discussing the importance of privacy-preserving techniques. In response to the diversity of Internet services, data services based on machine learning are now available for various applications, including risk assessment and image recognition. In light of open access to datasets and not fully trusted environments, machine learning-based applications face enormous security and privacy risks. In turn, it presents studies conducted to address privacy issues and a series of proposed solutions for ensuring privacy protection in machine learning tasks involving multiple parties. In closing, the book reviews state-of-the-art privacy-preserving techniques and examines the security threats they face.
Privacy Preserving Data Mining
DOWNLOAD
Author : Jaideep Vaidya
language : en
Publisher: Springer Science & Business Media
Release Date : 2006-09-28
Privacy Preserving Data Mining written by Jaideep Vaidya and has been published by Springer Science & Business Media this book supported file pdf, txt, epub, kindle and other format this book has been release on 2006-09-28 with Computers categories.
Privacy preserving data mining implies the "mining" of knowledge from distributed data without violating the privacy of the individual/corporations involved in contributing the data. This volume provides a comprehensive overview of available approaches, techniques and open problems in privacy preserving data mining. Crystallizing much of the underlying foundation, the book aims to inspire further research in this new and growing area. Privacy Preserving Data Mining is intended to be accessible to industry practitioners and policy makers, to help inform future decision making and legislation, and to serve as a useful technical reference.