Nonlinear Forecasting Using Large Datasets
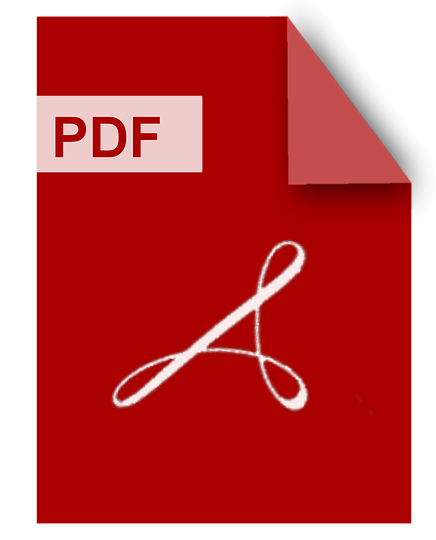
DOWNLOAD
Download Nonlinear Forecasting Using Large Datasets PDF/ePub or read online books in Mobi eBooks. Click Download or Read Online button to get Nonlinear Forecasting Using Large Datasets book now. This website allows unlimited access to, at the time of writing, more than 1.5 million titles, including hundreds of thousands of titles in various foreign languages. If the content not found or just blank you must refresh this page
Nonlinear Forecasting Using Large Datasets
DOWNLOAD
Author : Alessandro Giovannelli
language : en
Publisher:
Release Date : 2014
Nonlinear Forecasting Using Large Datasets written by Alessandro Giovannelli and has been published by this book supported file pdf, txt, epub, kindle and other format this book has been release on 2014 with categories.
The primary objective of this paper is to propose two nonlinear extensions for macroeconomic forecasting using large datasets. First, we propose an alternative technique for factor estimation, i.e., kernel principal component analysis, which allows the factors to have a nonlinear relationship to the input variables. Second, we propose arti ficial neural networks as an alternative to the factor augmented linear forecasting equation. These two extensions allow us to determine whether, in general, there is empirical evidence in favor of nonlinear methods and, in particular, to verify whether the nonlinearity occurs in the estimation of the factors or in the functional form that links the target variable to the factors. In an e ffort to verify the empirical performances of the methods proposed, we conducted several pseudo forecasting exercises on the industrial production index and consumer price index for the Euro area and US economies. These methods were employed to construct the forecasts at 1-, 3-, 6-, and 12-month horizons using a large dataset containing 259 predictors for the Euro area and 131 predictors for the US economy. The results obtained from the empirical study suggest that the estimation of nonlinear factors, using kernel principal components, signifi cantly improves the quality of forecasts compared to the linear method, while the results for artifi cial neural networks have the same forecasting ability as the factor augmented linear forecasting equation.
Nonlinear Modeling And Forecasting
DOWNLOAD
Author : Martin Casdagli
language : en
Publisher: Westview Press
Release Date : 1992-06-20
Nonlinear Modeling And Forecasting written by Martin Casdagli and has been published by Westview Press this book supported file pdf, txt, epub, kindle and other format this book has been release on 1992-06-20 with Science categories.
Nonlinear Forecasting Using A Large Number Of Predictors
DOWNLOAD
Author : Alessandro Giovannelli
language : en
Publisher:
Release Date : 2012
Nonlinear Forecasting Using A Large Number Of Predictors written by Alessandro Giovannelli and has been published by this book supported file pdf, txt, epub, kindle and other format this book has been release on 2012 with categories.
This paper aims to introduce a nonlinear model to forecast macroeconomic time series using a large number of predictors. The technique used to summarize the predictors in a small number of variables is Principal Component Analysis (PC), while the method used to capture nonlinearity is artificial neural network, specifically Feedforward Neural Network (FNN). Commonly in principal component regression forecasts are made using linear models. However linear techniques are often misspecified providing only a poor approximation to the best possible forecast. In an effort to address this issue, the FNN-PC technique is proposed. To determine the practical usefulness of the model, several pseudo forecasting exercises on 8 series of the United States economy, grouped in real and nominal categories, are conducted. This method was used to construct the forecasts at 1-, 3-, 6-, and 12-month horizons for monthly US economic variables using 131 predictors. The empirical study shows that FNN-PC has good ability to predict the variables under study in the period before the start of the "Great Moderation", namely 1984. After 1984, FNN-PC has the same accuracy in forecasting with respect to the benchmark.
Big Data Analytics With Matlab
DOWNLOAD
Author : L. Marvin
language : en
Publisher: Createspace Independent Publishing Platform
Release Date : 2017-09-10
Big Data Analytics With Matlab written by L. Marvin and has been published by Createspace Independent Publishing Platform this book supported file pdf, txt, epub, kindle and other format this book has been release on 2017-09-10 with categories.
MATLAB has the tools to work with large datasets and apply the necessary data analysis techniques. This book develops the work with nonlinear regression analysis techniques. Big data analytics examines large amounts of data to uncover hidden patterns, correlations and other insights. With today's technology, it's possible to analyze your data and get answers from it almost immediately - an effort that's slower and less efficient with more traditional business intelligence solutions. Nonlinear Regression models describe the nonlinear relationship between a response (output) variable, and one or more predictor (input) variables. Statistics and Machine Learning Toolbox allows you to fit linear, generalized linear, and nonlinear regression models, including stepwise models and mixed-effects models. Once you fit a model, you can use it to predict or simulate responses, assess the model fit using hypothesis tests, or use plots to visualize diagnostics, residuals, and interaction effects. Statistics and Machine Learning Toolbox also provides nonparametric regression methods to accommodate more complex regression curves without specifying the relationship between the response and the predictors with a predetermined regression function. You can predict responses for new data using the trained model. Gaussian process regression models also enable you to compute prediction intervals.
Modelling And Forecasting Financial Data
DOWNLOAD
Author : Abdol S. Soofi
language : en
Publisher: Springer Science & Business Media
Release Date : 2002-03-31
Modelling And Forecasting Financial Data written by Abdol S. Soofi and has been published by Springer Science & Business Media this book supported file pdf, txt, epub, kindle and other format this book has been release on 2002-03-31 with Business & Economics categories.
Over the last decade, dynamical systems theory and related nonlinear methods have had a major impact on the analysis of time series data from complex systems. Recent developments in mathematical methods of state-space reconstruction, time-delay embedding, and surrogate data analysis, coupled with readily accessible and powerful computational facilities used in gathering and processing massive quantities of high-frequency data, have provided theorists and practitioners unparalleled opportunities for exploratory data analysis, modelling, forecasting, and control. Until now, research exploring the application of nonlinear dynamics and associated algorithms to the study of economies and markets as complex systems is sparse and fragmentary at best. Modelling and Forecasting Financial Data brings together a coherent and accessible set of chapters on recent research results on this topic. To make such methods readily useful in practice, the contributors to this volume have agreed to make available to readers upon request all computer programs used to implement the methods discussed in their respective chapters. Modelling and Forecasting Financial Data is a valuable resource for researchers and graduate students studying complex systems in finance, biology, and physics, as well as those applying such methods to nonlinear time series analysis and signal processing.
Nonlinear Time Series Analysis
DOWNLOAD
Author : Ruey S. Tsay
language : en
Publisher: John Wiley & Sons
Release Date : 2018-10-23
Nonlinear Time Series Analysis written by Ruey S. Tsay and has been published by John Wiley & Sons this book supported file pdf, txt, epub, kindle and other format this book has been release on 2018-10-23 with Mathematics categories.
A comprehensive resource that draws a balance between theory and applications of nonlinear time series analysis Nonlinear Time Series Analysis offers an important guide to both parametric and nonparametric methods, nonlinear state-space models, and Bayesian as well as classical approaches to nonlinear time series analysis. The authors—noted experts in the field—explore the advantages and limitations of the nonlinear models and methods and review the improvements upon linear time series models. The need for this book is based on the recent developments in nonlinear time series analysis, statistical learning, dynamic systems and advanced computational methods. Parametric and nonparametric methods and nonlinear and non-Gaussian state space models provide a much wider range of tools for time series analysis. In addition, advances in computing and data collection have made available large data sets and high-frequency data. These new data make it not only feasible, but also necessary to take into consideration the nonlinearity embedded in most real-world time series. This vital guide: • Offers research developed by leading scholars of time series analysis • Presents R commands making it possible to reproduce all the analyses included in the text • Contains real-world examples throughout the book • Recommends exercises to test understanding of material presented • Includes an instructor solutions manual and companion website Written for students, researchers, and practitioners who are interested in exploring nonlinearity in time series, Nonlinear Time Series Analysis offers a comprehensive text that explores the advantages and limitations of the nonlinear models and methods and demonstrates the improvements upon linear time series models.
Applied Economic Forecasting Using Time Series Methods
DOWNLOAD
Author : Eric Ghysels
language : en
Publisher: Oxford University Press
Release Date : 2018-03-23
Applied Economic Forecasting Using Time Series Methods written by Eric Ghysels and has been published by Oxford University Press this book supported file pdf, txt, epub, kindle and other format this book has been release on 2018-03-23 with Business & Economics categories.
Economic forecasting is a key ingredient of decision making both in the public and in the private sector. Because economic outcomes are the result of a vast, complex, dynamic and stochastic system, forecasting is very difficult and forecast errors are unavoidable. Because forecast precision and reliability can be enhanced by the use of proper econometric models and methods, this innovative book provides an overview of both theory and applications. Undergraduate and graduate students learning basic and advanced forecasting techniques will be able to build from strong foundations, and researchers in public and private institutions will have access to the most recent tools and insights. Readers will gain from the frequent examples that enhance understanding of how to apply techniques, first by using stylized settings and then by real data applications--focusing on macroeconomic and financial topics. This is first and foremost a book aimed at applying time series methods to solve real-world forecasting problems. Applied Economic Forecasting using Time Series Methods starts with a brief review of basic regression analysis with a focus on specific regression topics relevant for forecasting, such as model specification errors, dynamic models and their predictive properties as well as forecast evaluation and combination. Several chapters cover univariate time series models, vector autoregressive models, cointegration and error correction models, and Bayesian methods for estimating vector autoregressive models. A collection of special topics chapters study Threshold and Smooth Transition Autoregressive (TAR and STAR) models, Markov switching regime models, state space models and the Kalman filter, mixed frequency data models, nowcasting, forecasting using large datasets and, finally, volatility models. There are plenty of practical applications in the book and both EViews and R code are available online at authors' website.
Big Data Analytics With Matlab
DOWNLOAD
Author : L. Marvin
language : en
Publisher: Createspace Independent Publishing Platform
Release Date : 2017-09-09
Big Data Analytics With Matlab written by L. Marvin and has been published by Createspace Independent Publishing Platform this book supported file pdf, txt, epub, kindle and other format this book has been release on 2017-09-09 with categories.
MATLAB has the tools to work with large datasets and apply the necessary data analysis techniques. This book develops the work with predictive linear and nonlinear models. Big data analytics examines large amounts of data to uncover hidden patterns, correlations and other insights. With today's technology, it's possible to analyze your data and get answers from it almost immediately - an effort that's slower and less efficient with more traditional business intelligence solutions. Predictive models describe the relationship between a response (output) variable, and one or more predictor (input) variables. Statistics and Machine Learning Toolbox allows you to fit linear, generalized linear, and nonlinear regression models, including stepwise models and mixed-effects models. Once you fit a model, you can use it to predict or simulate responses, assess the model fit using hypothesis tests, or use plots to visualize diagnostics, residuals, and interaction effects. Statistics and Machine Learning Toolbox also provides nonparametric regression methods to accommodate more complex regression curves without specifying the relationship between the response and the predictors with a predetermined regression function. You can predict responses for new data using the trained model. Gaussian process regression models also enable you to compute prediction intervals.
Large Scale Automated Forecasting Using Fractals
DOWNLOAD
Author : Deepayan Chakrabarti
language : en
Publisher:
Release Date : 2002
Large Scale Automated Forecasting Using Fractals written by Deepayan Chakrabarti and has been published by this book supported file pdf, txt, epub, kindle and other format this book has been release on 2002 with Database design categories.
Abstract: "Forecasting has attracted a lot of research interest, with very successful methods for periodic time sequences. Here, we propose a fast, automated method to do non-linear forecasting, for both periodic as well as chaotic time sequences. We use the technique of delay coordinate embedding, which needs several parameters; our contribution is the automated way of setting these parameters, using the concept of 'intrinsic dimensionality'. Our operational system has fast and scalable algorithms for preprocessing and, using R-trees, also has fast methods for forecasting. The result of this work is a black-box which, given a time series as input, finds the best parameter settings, and generates a prediction system. Tests on real and synthetic data show that our system achieves low error, while it can handle arbitrarily large datasets."
Of Needles And Haystacks
DOWNLOAD
Author : Peter Exterkate
language : en
Publisher:
Release Date : 2011
Of Needles And Haystacks written by Peter Exterkate and has been published by this book supported file pdf, txt, epub, kindle and other format this book has been release on 2011 with categories.