Novel Random Forest And Variable Importance Methods For Clustered Data
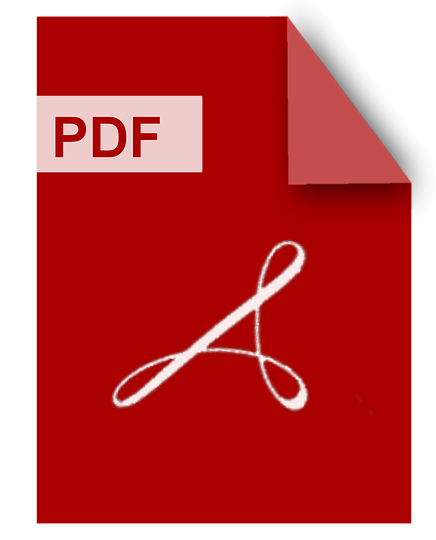
DOWNLOAD
Download Novel Random Forest And Variable Importance Methods For Clustered Data PDF/ePub or read online books in Mobi eBooks. Click Download or Read Online button to get Novel Random Forest And Variable Importance Methods For Clustered Data book now. This website allows unlimited access to, at the time of writing, more than 1.5 million titles, including hundreds of thousands of titles in various foreign languages. If the content not found or just blank you must refresh this page
Novel Random Forest And Variable Importance Methods For Clustered Data
DOWNLOAD
Author :
language : en
Publisher:
Release Date : 2017
Novel Random Forest And Variable Importance Methods For Clustered Data written by and has been published by this book supported file pdf, txt, epub, kindle and other format this book has been release on 2017 with Electronic books categories.
Tree-based methods are becoming increasingly popular due to their few statistical assumptions and accurate predictions. Classification and Regression Trees (CART) can handle a variety of data structures and give easy to interpret prediction rules. However, there are several limitations with CART including requiring independent outcomes, having high variance, giving poor predictive performance, and inducing a variable selection bias. In this dissertation, we discuss these limitations and propose algorithms that resolve these issues. n Chapter 1, we introduce CART and discuss the advantages with tree-based methods. We show CART handles interactions and nonlinear relationships and provides easy to interpret prediction rules. We conclude with an example and discuss some of the limitations with the standard CART implementation. In Chapter 2, we discuss the MST R package which extends the CART implementation to handle multivariate survival data. We introduce multivariate survival trees and illustrate how they can be constructed in R. We discuss some of the features of the MST R package. We analyze a dental study to predict tooth loss and estimate survival of molars and non-molars. We conclude with future directions of the MST R package. In Chapter 3, we introduce random forests. Random forests reduce the variance from CART and are one of the most accurate machine learning methods to make predictions and analyze studies. However, the variable selection bias found in CART still occurs with random forests. We propose a variant of the random forest called completely randomized with acceptance-rejection trees (CRAR). We compare our proposed method with three other methods of constructing random forests: standard random forest (RF), smooth sigmoid surrogate trees (SSS), and extremely randomized trees (ER). We find CRAR and ER have the best overall accuracy and performance for classification problems. They have the lowest misclassification rates, reduce or eliminate the variable selection bias, and are the fastest algorithms. The best algorithm for regression problems may be selected based on the overall objective — whether it be high accuracy, variable selection, or speed. We recommend considering all four algorithms based on the study and objective. In Chapter 4, we propose the repeated measures random forest (RMRF) algorithm that extends the standard random forest implementation to handle longitudinal designs. The RMRF algorithm uses subsamples, the robust Wald statistic, and an accept-reject quality control step to grow an ensemble of trees. We adopt an area under the curve (AUC) based permuted importance method to assess variable importance. We show the RMRF algorithm outperforms other algorithms that naively assume independence under a variety of data simulations. An algorithm that ignores the dependence will favor patient-level variables for strongly correlated responses. We also show the RMRF algorithm outperforms RF and ER at identifying the informative variable. The final chapter uses the RMRF algorithm to identify factors associated with nocturnal hypoglycemia. We adopt a permuted importance method to test significance of factors with random forests. We find hemoglobin A1c (P=0.01), bedtime blood glucose (P=0.01), insulin on board (P=0.03), time system activated (P=0.02), exercise (P=0.01), and daytime hypoglycemia (P=0.01) are associated with nocturnal hypoglycemia. We show interaction effects affect hypoglycemia and explore the significance of time system activated. Finally, we assign risk profiles to each night and show the RMRF algorithm accurately predicts nocturnal hypoglycemia. We conclude the proposed RMRF algorithm can identify influential variables while handling dependent outcomes.
Novel Random Forest And Variable Importance Methods For Correlated Survival Data With Applications To Tooth Prognosis
DOWNLOAD
Author :
language : en
Publisher:
Release Date : 2015
Novel Random Forest And Variable Importance Methods For Correlated Survival Data With Applications To Tooth Prognosis written by and has been published by this book supported file pdf, txt, epub, kindle and other format this book has been release on 2015 with Electronic books categories.
Multivariate failure time data arise when individuals under study are naturally clustered. This type of data requires multivariate extensions of existing statistical methodolgy. Due to their nonparametric approach and interpretable results, tree-based methods have become some of the most flexible and popular analytic tools for modeling complex data structures. This dissertation is intended to present new methodology for random forests and variable importance measures. Brieman's [7] original random forest (RF) method is shown to be unreliable when the number of categories of potential predictor variables varies [38]. We introduce a new RF algorithm that reduces the bias in variable importance ranking for correlated survival data. The multivariate exponential tree algorithm of Fan and Su [15] is used to build trees, due to its superior prediction accuracy and computational efficiency. Simulation studies for assessing various variable importance methods are presented. We compare the proposed method to the traditional Cox proportional hazards frailty model in their prediction accuracy. We apply our method to the VA Dental Longitudinal Study to assess tooth loss. To generate even more randomization into the RF procedure, we introduce a second RF method for correlated survival data. It consists of randomizing completely both attribute and cut point choice while splitting a tree node. To ensure the quality of each split, two different split evaluation criteria are used, the likelihood ratio and the score test criteria based on the semi-parametric and exponential frailty models respectively. We show the proposed method is computationally inexpensive, yet accurate in uncovering the true variable importance rankings. In order to apply the proposed approach to RF, computational efficiency becomes particularly challenging. RF methods have been recognized in recent times to be highly effective and ideally suited for parallelization. We present a parallel formulation of the proposed RF method to address large datasets. We apply the new tree based method and its respective variable importance measures to correlated survival data from a dental school database, consisting of 373,202 observations and present the results of our analysis.
An Introduction To Clustering With R
DOWNLOAD
Author : Paolo Giordani
language : en
Publisher: Springer Nature
Release Date : 2020-08-27
An Introduction To Clustering With R written by Paolo Giordani and has been published by Springer Nature this book supported file pdf, txt, epub, kindle and other format this book has been release on 2020-08-27 with Mathematics categories.
The purpose of this book is to thoroughly prepare the reader for applied research in clustering. Cluster analysis comprises a class of statistical techniques for classifying multivariate data into groups or clusters based on their similar features. Clustering is nowadays widely used in several domains of research, such as social sciences, psychology, and marketing, highlighting its multidisciplinary nature. This book provides an accessible and comprehensive introduction to clustering and offers practical guidelines for applying clustering tools by carefully chosen real-life datasets and extensive data analyses. The procedures addressed in this book include traditional hard clustering methods and up-to-date developments in soft clustering. Attention is paid to practical examples and applications through the open source statistical software R. Commented R code and output for conducting, step by step, complete cluster analyses are available. The book is intended for researchers interested in applying clustering methods. Basic notions on theoretical issues and on R are provided so that professionals as well as novices with little or no background in the subject will benefit from the book.
Explanatory Model Analysis
DOWNLOAD
Author : Przemyslaw Biecek
language : en
Publisher: CRC Press
Release Date : 2021-02-15
Explanatory Model Analysis written by Przemyslaw Biecek and has been published by CRC Press this book supported file pdf, txt, epub, kindle and other format this book has been release on 2021-02-15 with Business & Economics categories.
Explanatory Model Analysis Explore, Explain and Examine Predictive Models is a set of methods and tools designed to build better predictive models and to monitor their behaviour in a changing environment. Today, the true bottleneck in predictive modelling is neither the lack of data, nor the lack of computational power, nor inadequate algorithms, nor the lack of flexible models. It is the lack of tools for model exploration (extraction of relationships learned by the model), model explanation (understanding the key factors influencing model decisions) and model examination (identification of model weaknesses and evaluation of model's performance). This book presents a collection of model agnostic methods that may be used for any black-box model together with real-world applications to classification and regression problems.
Interpretable Machine Learning
DOWNLOAD
Author : Christoph Molnar
language : en
Publisher: Lulu.com
Release Date : 2020
Interpretable Machine Learning written by Christoph Molnar and has been published by Lulu.com this book supported file pdf, txt, epub, kindle and other format this book has been release on 2020 with Artificial intelligence categories.
This book is about making machine learning models and their decisions interpretable. After exploring the concepts of interpretability, you will learn about simple, interpretable models such as decision trees, decision rules and linear regression. Later chapters focus on general model-agnostic methods for interpreting black box models like feature importance and accumulated local effects and explaining individual predictions with Shapley values and LIME. All interpretation methods are explained in depth and discussed critically. How do they work under the hood? What are their strengths and weaknesses? How can their outputs be interpreted? This book will enable you to select and correctly apply the interpretation method that is most suitable for your machine learning project.
Hands On Ensemble Learning With R
DOWNLOAD
Author : Prabhanjan Narayanachar Tattar
language : en
Publisher: Packt Publishing Ltd
Release Date : 2018-07-27
Hands On Ensemble Learning With R written by Prabhanjan Narayanachar Tattar and has been published by Packt Publishing Ltd this book supported file pdf, txt, epub, kindle and other format this book has been release on 2018-07-27 with Computers categories.
Explore powerful R packages to create predictive models using ensemble methods Key Features Implement machine learning algorithms to build ensemble-efficient models Explore powerful R packages to create predictive models using ensemble methods Learn to build ensemble models on large datasets using a practical approach Book Description Ensemble techniques are used for combining two or more similar or dissimilar machine learning algorithms to create a stronger model. Such a model delivers superior prediction power and can give your datasets a boost in accuracy. Hands-On Ensemble Learning with R begins with the important statistical resampling methods. You will then walk through the central trilogy of ensemble techniques – bagging, random forest, and boosting – then you'll learn how they can be used to provide greater accuracy on large datasets using popular R packages. You will learn how to combine model predictions using different machine learning algorithms to build ensemble models. In addition to this, you will explore how to improve the performance of your ensemble models. By the end of this book, you will have learned how machine learning algorithms can be combined to reduce common problems and build simple efficient ensemble models with the help of real-world examples. What you will learn Carry out an essential review of re-sampling methods, bootstrap, and jackknife Explore the key ensemble methods: bagging, random forests, and boosting Use multiple algorithms to make strong predictive models Enjoy a comprehensive treatment of boosting methods Supplement methods with statistical tests, such as ROC Walk through data structures in classification, regression, survival, and time series data Use the supplied R code to implement ensemble methods Learn stacking method to combine heterogeneous machine learning models Who this book is for This book is for you if you are a data scientist or machine learning developer who wants to implement machine learning techniques by building ensemble models with the power of R. You will learn how to combine different machine learning algorithms to perform efficient data processing. Basic knowledge of machine learning techniques and programming knowledge of R would be an added advantage.
Properties Of Random Forest Variable Importance Measures
DOWNLOAD
Author : Eva Maria Deutschmann
language : en
Publisher:
Release Date : 2017
Properties Of Random Forest Variable Importance Measures written by Eva Maria Deutschmann and has been published by this book supported file pdf, txt, epub, kindle and other format this book has been release on 2017 with categories.
Hands On Machine Learning With R
DOWNLOAD
Author : Brad Boehmke
language : en
Publisher: CRC Press
Release Date : 2019-11-07
Hands On Machine Learning With R written by Brad Boehmke and has been published by CRC Press this book supported file pdf, txt, epub, kindle and other format this book has been release on 2019-11-07 with Business & Economics categories.
Hands-on Machine Learning with R provides a practical and applied approach to learning and developing intuition into today’s most popular machine learning methods. This book serves as a practitioner’s guide to the machine learning process and is meant to help the reader learn to apply the machine learning stack within R, which includes using various R packages such as glmnet, h2o, ranger, xgboost, keras, and others to effectively model and gain insight from their data. The book favors a hands-on approach, providing an intuitive understanding of machine learning concepts through concrete examples and just a little bit of theory. Throughout this book, the reader will be exposed to the entire machine learning process including feature engineering, resampling, hyperparameter tuning, model evaluation, and interpretation. The reader will be exposed to powerful algorithms such as regularized regression, random forests, gradient boosting machines, deep learning, generalized low rank models, and more! By favoring a hands-on approach and using real word data, the reader will gain an intuitive understanding of the architectures and engines that drive these algorithms and packages, understand when and how to tune the various hyperparameters, and be able to interpret model results. By the end of this book, the reader should have a firm grasp of R’s machine learning stack and be able to implement a systematic approach for producing high quality modeling results. Features: · Offers a practical and applied introduction to the most popular machine learning methods. · Topics covered include feature engineering, resampling, deep learning and more. · Uses a hands-on approach and real world data.
Mixed Effects Random Forest For Clustered Data
DOWNLOAD
Author : Ahlem Hajjem
language : en
Publisher:
Release Date : 2010
Mixed Effects Random Forest For Clustered Data written by Ahlem Hajjem and has been published by this book supported file pdf, txt, epub, kindle and other format this book has been release on 2010 with categories.
Alternative Methods Via Random Forest To Identify Interactions In A General Framework And Variable Importance In The Context Of Value Added Models
DOWNLOAD
Author : Arturo Valdivia
language : en
Publisher:
Release Date : 2013
Alternative Methods Via Random Forest To Identify Interactions In A General Framework And Variable Importance In The Context Of Value Added Models written by Arturo Valdivia and has been published by this book supported file pdf, txt, epub, kindle and other format this book has been release on 2013 with Data mining categories.
The second study develops two novel interaction measures. These measures could be used within but are not restricted to the VAM framework. The distribution-based measure is constructed to identify interactions in a general setting where a model specification is not assumed in advance. In turn, the mean-based measure is built to estimate interactions when the model specification is assumed to be linear. Both measures are unique in their construction; they take into account not only the outcome values, but also the internal structure of the trees in a random forest. In a separate simulation study, under a variety of conditions, the proposed measures are found to identify and estimate second-order interactions.