Privacy Preserving Data Publishing Using Deep Learning Techniques
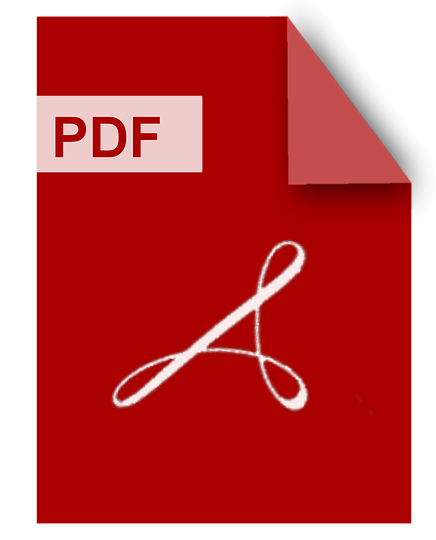
DOWNLOAD
Download Privacy Preserving Data Publishing Using Deep Learning Techniques PDF/ePub or read online books in Mobi eBooks. Click Download or Read Online button to get Privacy Preserving Data Publishing Using Deep Learning Techniques book now. This website allows unlimited access to, at the time of writing, more than 1.5 million titles, including hundreds of thousands of titles in various foreign languages. If the content not found or just blank you must refresh this page
Privacy Preserving Data Publishing Using Deep Learning Techniques
DOWNLOAD
Author : Tanbir Ahmed
language : en
Publisher:
Release Date : 2021
Privacy Preserving Data Publishing Using Deep Learning Techniques written by Tanbir Ahmed and has been published by this book supported file pdf, txt, epub, kindle and other format this book has been release on 2021 with categories.
According to a recent study, around 99 percent of hospitals across the United States now use electronic health record systems. One of the most common types of EHR data is unstructured textual data and unlocking hidden details from this data is critical for improving current medical practices and research endeavors. However, these textual data contain sensitive information, which could compromise our privacy. Therefore, medical textual data cannot be released publicly without any privacy protection. De-identification is a process of detecting and removing all sensitive information present in EHRs, and it is a necessary step towards privacy-preserving EHR data sharing. Since 2016, we have seen several deep learning-based approaches for de-identification, which achieved over 98% accuracy. However, these models are trained with sensitive information and can unwittingly memorize some of their training data, and a careful analysis of these models can reveal patients' data. This thesis presents two contributions. First, We introduce new methods to de-identify textual based on self-attention mechanism and stacked Recurrent Neural Network. Experimental results on three different datasets show that our model performs better than all state-of-the-art mechanisms irrespective of the dataset. Additionally, our proposed method is significantly faster than existing techniques. We also introduced three utility metrics to judge the quality of the de-identified data. Second, we propose a differentially private ensemble framework for de-identification, allowing medical researchers to collaborate through publicly publishing the de-identification models. Experiments in three different datasets showed competitive results compared to the state-of-the-art methods with guaranteed differential privacy.
Privacy Preserving Deep Learning
DOWNLOAD
Author : Kwangjo Kim
language : en
Publisher: Springer Nature
Release Date : 2021-07-22
Privacy Preserving Deep Learning written by Kwangjo Kim and has been published by Springer Nature this book supported file pdf, txt, epub, kindle and other format this book has been release on 2021-07-22 with Computers categories.
This book discusses the state-of-the-art in privacy-preserving deep learning (PPDL), especially as a tool for machine learning as a service (MLaaS), which serves as an enabling technology by combining classical privacy-preserving and cryptographic protocols with deep learning. Google and Microsoft announced a major investment in PPDL in early 2019. This was followed by Google’s infamous announcement of “Private Join and Compute,” an open source PPDL tools based on secure multi-party computation (secure MPC) and homomorphic encryption (HE) in June of that year. One of the challenging issues concerning PPDL is selecting its practical applicability despite the gap between the theory and practice. In order to solve this problem, it has recently been proposed that in addition to classical privacy-preserving methods (HE, secure MPC, differential privacy, secure enclaves), new federated or split learning for PPDL should also be applied. This concept involves building a cloud framework that enables collaborative learning while keeping training data on client devices. This successfully preserves privacy and while allowing the framework to be implemented in the real world. This book provides fundamental insights into privacy-preserving and deep learning, offering a comprehensive overview of the state-of-the-art in PPDL methods. It discusses practical issues, and leveraging federated or split-learning-based PPDL. Covering the fundamental theory of PPDL, the pros and cons of current PPDL methods, and addressing the gap between theory and practice in the most recent approaches, it is a valuable reference resource for a general audience, undergraduate and graduate students, as well as practitioners interested learning about PPDL from the scratch, and researchers wanting to explore PPDL for their applications.
Introduction To Privacy Preserving Data Publishing
DOWNLOAD
Author : Benjamin C.M. Fung
language : en
Publisher: CRC Press
Release Date : 2010-08-02
Introduction To Privacy Preserving Data Publishing written by Benjamin C.M. Fung and has been published by CRC Press this book supported file pdf, txt, epub, kindle and other format this book has been release on 2010-08-02 with Computers categories.
Gaining access to high-quality data is a vital necessity in knowledge-based decision making. But data in its raw form often contains sensitive information about individuals. Providing solutions to this problem, the methods and tools of privacy-preserving data publishing enable the publication of useful information while protecting data privacy. Int
Privacy Preserving Machine Learning
DOWNLOAD
Author : J. Morris Chang
language : en
Publisher: Simon and Schuster
Release Date : 2023-05-02
Privacy Preserving Machine Learning written by J. Morris Chang and has been published by Simon and Schuster this book supported file pdf, txt, epub, kindle and other format this book has been release on 2023-05-02 with Computers categories.
Keep sensitive user data safe and secure without sacrificing the performance and accuracy of your machine learning models. In Privacy Preserving Machine Learning, you will learn: Privacy considerations in machine learning Differential privacy techniques for machine learning Privacy-preserving synthetic data generation Privacy-enhancing technologies for data mining and database applications Compressive privacy for machine learning Privacy-Preserving Machine Learning is a comprehensive guide to avoiding data breaches in your machine learning projects. You’ll get to grips with modern privacy-enhancing techniques such as differential privacy, compressive privacy, and synthetic data generation. Based on years of DARPA-funded cybersecurity research, ML engineers of all skill levels will benefit from incorporating these privacy-preserving practices into their model development. By the time you’re done reading, you’ll be able to create machine learning systems that preserve user privacy without sacrificing data quality and model performance. Purchase of the print book includes a free eBook in PDF, Kindle, and ePub formats from Manning Publications. About the Technology Machine learning applications need massive amounts of data. It’s up to you to keep the sensitive information in those data sets private and secure. Privacy preservation happens at every point in the ML process, from data collection and ingestion to model development and deployment. This practical book teaches you the skills you’ll need to secure your data pipelines end to end. About the Book Privacy-Preserving Machine Learning explores privacy preservation techniques through real-world use cases in facial recognition, cloud data storage, and more. You’ll learn about practical implementations you can deploy now, future privacy challenges, and how to adapt existing technologies to your needs. Your new skills build towards a complete security data platform project you’ll develop in the final chapter. What’s Inside Differential and compressive privacy techniques Privacy for frequency or mean estimation, naive Bayes classifier, and deep learning Privacy-preserving synthetic data generation Enhanced privacy for data mining and database applications About the Reader For machine learning engineers and developers. Examples in Python and Java. About the Author J. Morris Chang is a professor at the University of South Florida. His research projects have been funded by DARPA and the DoD. Di Zhuang is a security engineer at Snap Inc. Dumindu Samaraweera is an assistant research professor at the University of South Florida. The technical editor for this book, Wilko Henecka, is a senior software engineer at Ambiata where he builds privacy-preserving software. Table of Contents PART 1 - BASICS OF PRIVACY-PRESERVING MACHINE LEARNING WITH DIFFERENTIAL PRIVACY 1 Privacy considerations in machine learning 2 Differential privacy for machine learning 3 Advanced concepts of differential privacy for machine learning PART 2 - LOCAL DIFFERENTIAL PRIVACY AND SYNTHETIC DATA GENERATION 4 Local differential privacy for machine learning 5 Advanced LDP mechanisms for machine learning 6 Privacy-preserving synthetic data generation PART 3 - BUILDING PRIVACY-ASSURED MACHINE LEARNING APPLICATIONS 7 Privacy-preserving data mining techniques 8 Privacy-preserving data management and operations 9 Compressive privacy for machine learning 10 Putting it all together: Designing a privacy-enhanced platform (DataHub)
Privacy Preserving Data Publishing
DOWNLOAD
Author : Bee-Chung Chen
language : en
Publisher: Now Publishers Inc
Release Date : 2009-10-14
Privacy Preserving Data Publishing written by Bee-Chung Chen and has been published by Now Publishers Inc this book supported file pdf, txt, epub, kindle and other format this book has been release on 2009-10-14 with Data mining categories.
This book is dedicated to those who have something to hide. It is a book about "privacy preserving data publishing" -- the art of publishing sensitive personal data, collected from a group of individuals, in a form that does not violate their privacy. This problem has numerous and diverse areas of application, including releasing Census data, search logs, medical records, and interactions on a social network. The purpose of this book is to provide a detailed overview of the current state of the art as well as open challenges, focusing particular attention on four key themes: RIGOROUS PRIVACY POLICIES Repeated and highly-publicized attacks on published data have demonstrated that simplistic approaches to data publishing do not work. Significant recent advances have exposed the shortcomings of naive (and not-so-naive) techniques. They have also led to the development of mathematically rigorous definitions of privacy that publishing techniques must satisfy; METRICS FOR DATA UTILITY While it is necessary to enforce stringent privacy policies, it is equally important to ensure that the published version of the data is useful for its intended purpose. The authors provide an overview of diverse approaches to measuring data utility; ENFORCEMENT MECHANISMS This book describes in detail various key data publishing mechanisms that guarantee privacy and utility; EMERGING APPLICATIONS The problem of privacy-preserving data publishing arises in diverse application domains with unique privacy and utility requirements. The authors elaborate on the merits and limitations of existing solutions, based on which we expect to see many advances in years to come.
Privacy Preserving Machine Learning
DOWNLOAD
Author : J. Morris Chang
language : en
Publisher: Simon and Schuster
Release Date : 2023-05-23
Privacy Preserving Machine Learning written by J. Morris Chang and has been published by Simon and Schuster this book supported file pdf, txt, epub, kindle and other format this book has been release on 2023-05-23 with Computers categories.
Keep sensitive user data safe and secure without sacrificing the performance and accuracy of your machine learning models. In Privacy Preserving Machine Learning, you will learn: Privacy considerations in machine learning Differential privacy techniques for machine learning Privacy-preserving synthetic data generation Privacy-enhancing technologies for data mining and database applications Compressive privacy for machine learning Privacy-Preserving Machine Learning is a comprehensive guide to avoiding data breaches in your machine learning projects. You’ll get to grips with modern privacy-enhancing techniques such as differential privacy, compressive privacy, and synthetic data generation. Based on years of DARPA-funded cybersecurity research, ML engineers of all skill levels will benefit from incorporating these privacy-preserving practices into their model development. By the time you’re done reading, you’ll be able to create machine learning systems that preserve user privacy without sacrificing data quality and model performance. About the Technology Machine learning applications need massive amounts of data. It’s up to you to keep the sensitive information in those data sets private and secure. Privacy preservation happens at every point in the ML process, from data collection and ingestion to model development and deployment. This practical book teaches you the skills you’ll need to secure your data pipelines end to end. About the Book Privacy-Preserving Machine Learning explores privacy preservation techniques through real-world use cases in facial recognition, cloud data storage, and more. You’ll learn about practical implementations you can deploy now, future privacy challenges, and how to adapt existing technologies to your needs. Your new skills build towards a complete security data platform project you’ll develop in the final chapter. What’s Inside Differential and compressive privacy techniques Privacy for frequency or mean estimation, naive Bayes classifier, and deep learning Privacy-preserving synthetic data generation Enhanced privacy for data mining and database applications About the Reader For machine learning engineers and developers. Examples in Python and Java. About the Author J. Morris Chang is a professor at the University of South Florida. His research projects have been funded by DARPA and the DoD. Di Zhuang is a security engineer at Snap Inc. Dumindu Samaraweera is an assistant research professor at the University of South Florida. The technical editor for this book, Wilko Henecka, is a senior software engineer at Ambiata where he builds privacy-preserving software. Table of Contents PART 1 - BASICS OF PRIVACY-PRESERVING MACHINE LEARNING WITH DIFFERENTIAL PRIVACY 1 Privacy considerations in machine learning 2 Differential privacy for machine learning 3 Advanced concepts of differential privacy for machine learning PART 2 - LOCAL DIFFERENTIAL PRIVACY AND SYNTHETIC DATA GENERATION 4 Local differential privacy for machine learning 5 Advanced LDP mechanisms for machine learning 6 Privacy-preserving synthetic data generation PART 3 - BUILDING PRIVACY-ASSURED MACHINE LEARNING APPLICATIONS 7 Privacy-preserving data mining techniques 8 Privacy-preserving data management and operations 9 Compressive privacy for machine learning 10 Putting it all together: Designing a privacy-enhanced platform (DataHub)
Privacy Preserving Machine Learning
DOWNLOAD
Author : Srinivasa Rao Aravilli
language : en
Publisher: Packt Publishing Ltd
Release Date : 2024-05-24
Privacy Preserving Machine Learning written by Srinivasa Rao Aravilli and has been published by Packt Publishing Ltd this book supported file pdf, txt, epub, kindle and other format this book has been release on 2024-05-24 with Computers categories.
Gain hands-on experience in data privacy and privacy-preserving machine learning with open-source ML frameworks, while exploring techniques and algorithms to protect sensitive data from privacy breaches Key Features Understand machine learning privacy risks and employ machine learning algorithms to safeguard data against breaches Develop and deploy privacy-preserving ML pipelines using open-source frameworks Gain insights into confidential computing and its role in countering memory-based data attacks Purchase of the print or Kindle book includes a free PDF eBook Book Description– In an era of evolving privacy regulations, compliance is mandatory for every enterprise – Machine learning engineers face the dual challenge of analyzing vast amounts of data for insights while protecting sensitive information – This book addresses the complexities arising from large data volumes and the scarcity of in-depth privacy-preserving machine learning expertise, and covers a comprehensive range of topics from data privacy and machine learning privacy threats to real-world privacy-preserving cases – As you progress, you’ll be guided through developing anti-money laundering solutions using federated learning and differential privacy – Dedicated sections will explore data in-memory attacks and strategies for safeguarding data and ML models – You’ll also explore the imperative nature of confidential computation and privacy-preserving machine learning benchmarks, as well as frontier research in the field – Upon completion, you’ll possess a thorough understanding of privacy-preserving machine learning, equipping them to effectively shield data from real-world threats and attacks What you will learn Study data privacy, threats, and attacks across different machine learning phases Explore Uber and Apple cases for applying differential privacy and enhancing data security Discover IID and non-IID data sets as well as data categories Use open-source tools for federated learning (FL) and explore FL algorithms and benchmarks Understand secure multiparty computation with PSI for large data Get up to speed with confidential computation and find out how it helps data in memory attacks Who this book is for – This comprehensive guide is for data scientists, machine learning engineers, and privacy engineers – Prerequisites include a working knowledge of mathematics and basic familiarity with at least one ML framework (TensorFlow, PyTorch, or scikit-learn) – Practical examples will help you elevate your expertise in privacy-preserving machine learning techniques
Privacy Preserving Machine Learning
DOWNLOAD
Author : Jin Li
language : en
Publisher: Springer Nature
Release Date : 2022-03-14
Privacy Preserving Machine Learning written by Jin Li and has been published by Springer Nature this book supported file pdf, txt, epub, kindle and other format this book has been release on 2022-03-14 with Computers categories.
This book provides a thorough overview of the evolution of privacy-preserving machine learning schemes over the last ten years, after discussing the importance of privacy-preserving techniques. In response to the diversity of Internet services, data services based on machine learning are now available for various applications, including risk assessment and image recognition. In light of open access to datasets and not fully trusted environments, machine learning-based applications face enormous security and privacy risks. In turn, it presents studies conducted to address privacy issues and a series of proposed solutions for ensuring privacy protection in machine learning tasks involving multiple parties. In closing, the book reviews state-of-the-art privacy-preserving techniques and examines the security threats they face.
Privacy Preserving Data Publishing
DOWNLOAD
Author : Raymond Chi-Wing Wong
language : en
Publisher: Springer Nature
Release Date : 2022-05-31
Privacy Preserving Data Publishing written by Raymond Chi-Wing Wong and has been published by Springer Nature this book supported file pdf, txt, epub, kindle and other format this book has been release on 2022-05-31 with Computers categories.
Privacy preservation has become a major issue in many data analysis applications. When a data set is released to other parties for data analysis, privacy-preserving techniques are often required to reduce the possibility of identifying sensitive information about individuals. For example, in medical data, sensitive information can be the fact that a particular patient suffers from HIV. In spatial data, sensitive information can be a specific location of an individual. In web surfing data, the information that a user browses certain websites may be considered sensitive. Consider a dataset containing some sensitive information is to be released to the public. In order to protect sensitive information, the simplest solution is not to disclose the information. However, this would be an overkill since it will hinder the process of data analysis over the data from which we can find interesting patterns. Moreover, in some applications, the data must be disclosed under the government regulations. Alternatively, the data owner can first modify the data such that the modified data can guarantee privacy and, at the same time, the modified data retains sufficient utility and can be released to other parties safely. This process is usually called as privacy-preserving data publishing. In this monograph, we study how the data owner can modify the data and how the modified data can preserve privacy and protect sensitive information. Table of Contents: Introduction / Fundamental Concepts / One-Time Data Publishing / Multiple-Time Data Publishing / Graph Data / Other Data Types / Future Research Directions
Privacy Preserving Data Mining
DOWNLOAD
Author : Jaideep Vaidya
language : en
Publisher: Springer Science & Business Media
Release Date : 2006-09-28
Privacy Preserving Data Mining written by Jaideep Vaidya and has been published by Springer Science & Business Media this book supported file pdf, txt, epub, kindle and other format this book has been release on 2006-09-28 with Computers categories.
Privacy preserving data mining implies the "mining" of knowledge from distributed data without violating the privacy of the individual/corporations involved in contributing the data. This volume provides a comprehensive overview of available approaches, techniques and open problems in privacy preserving data mining. Crystallizing much of the underlying foundation, the book aims to inspire further research in this new and growing area. Privacy Preserving Data Mining is intended to be accessible to industry practitioners and policy makers, to help inform future decision making and legislation, and to serve as a useful technical reference.