Protein Domain Linker Prediction A Direction For Detecting Protein Protein Interactions
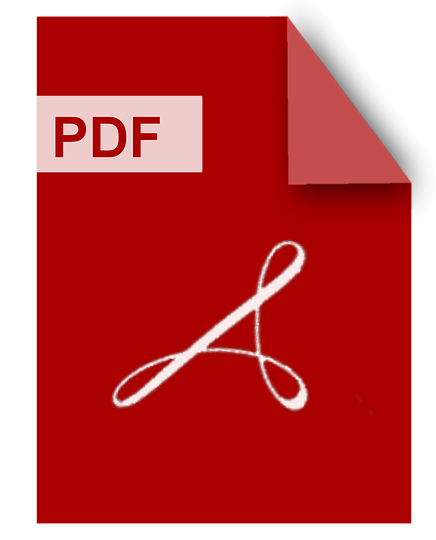
DOWNLOAD
Download Protein Domain Linker Prediction A Direction For Detecting Protein Protein Interactions PDF/ePub or read online books in Mobi eBooks. Click Download or Read Online button to get Protein Domain Linker Prediction A Direction For Detecting Protein Protein Interactions book now. This website allows unlimited access to, at the time of writing, more than 1.5 million titles, including hundreds of thousands of titles in various foreign languages. If the content not found or just blank you must refresh this page
Protein Domain Linker Prediction A Direction For Detecting Protein Protein Interactions
DOWNLOAD
Author :
language : en
Publisher:
Release Date :
Protein Domain Linker Prediction A Direction For Detecting Protein Protein Interactions written by and has been published by this book supported file pdf, txt, epub, kindle and other format this book has been release on with categories.
Protein chains are generally long and consist of multiple domains. Domains are the basic elements of protein structures that can exist, evolve, and function independently. The accurate and reliable identification of protein domains and their interactions has very important impacts in several protein research areas. The accurate prediction of protein domains is a fundamental stage in both experimental and computational proteomics. The knowledge of domains is an initial stage of protein tertiary structure prediction which can give insight into the way in which proteins work. The knowledge of domains is also useful in classifying proteins, understanding their structures, functions and evolution, and predicting proteinprotein interactions (PPI). However, predicting structural domains within proteins is a challenging task in computational biology. A promising direction of domain prediction is detecting inter-domain linkers and then predicting the reigns of the protein sequence in which the structural domains are located accordingly. Protein-protein interactions occur at almost every level of cell function. The identification of interaction among proteins and their associated domains provide a global picture of cellular functions and biological processes. It is also an essential step in the construction of PPI networks for human and other organisms. PPI prediction has been considered as a promising alternative to the traditional drug design techniques. The identification of possible viral-host protein interactions can lead to a better understanding of infection mechanisms and, in turn, to the development of several medication drugs and treatment optimization. In this work, a compact and accurate approach for inter-domain linker prediction is developed based solely on protein primary structure information. Then, inter-domain linker knowledge is used in predicting structural domains and detecting PPI. The research work in this dissertation can be summarized in three main contributions. The first contribution is predicting protein inter-domain linker regions by introducing the concept of amino acid compositional index and refining the prediction by using the Simulated Annealing optimization technique. The second contribution is identifying structural domains based on inter-domain linker knowledge. The inter-domain linker knowledge, represented by the compositional index, is enhanced by the incorporation of biological knowledge, represented by amino acid physiochemical properties, to develop a well-optimized Random Forest classifier for predicting novel domains and inter-domain linkers. In the third contribution, the domain information knowledge is utilized to predict protein-protein interactions. This is achieved by characterizing structural domains within protein sequences, analyzing their interactions, and predicting protein interactions based on their interacting domains. The experimental studies and the higher accuracy achieved is a valid argument in favor of the proposed framework.
Protein Protein Interactions And Networks
DOWNLOAD
Author : Anna Panchenko
language : en
Publisher: Springer Science & Business Media
Release Date : 2010-04-06
Protein Protein Interactions And Networks written by Anna Panchenko and has been published by Springer Science & Business Media this book supported file pdf, txt, epub, kindle and other format this book has been release on 2010-04-06 with Science categories.
The biological interactions of living organisms, and protein-protein interactions in particular, are astonishingly diverse. This comprehensive book provides a broad, thorough and multidisciplinary coverage of its field. It integrates different approaches from bioinformatics, biochemistry, computational analysis and systems biology to offer the reader a comprehensive global view of the diverse data on protein-protein interactions and protein interaction networks.
Predicting Protein Protein Interactions And Their Interacting Interfaces With Statistical Learning Techniques
DOWNLOAD
Author : Alvaro J. Gonzalez
language : en
Publisher:
Release Date : 2012
Predicting Protein Protein Interactions And Their Interacting Interfaces With Statistical Learning Techniques written by Alvaro J. Gonzalez and has been published by this book supported file pdf, txt, epub, kindle and other format this book has been release on 2012 with Protein-protein interactions categories.
Anywhere you look in the cell, you will see proteins at work. Proteins are molecular machines built in a multitude of shapes and sizes. They execute nearly all of the cell's functions. Typically proteins do not carry out their functions as isolated entities. They bind other proteins--other molecules in general--to create chemical factories with a definite spatial structure. With advances in genome sequencing scientists have made a good stride in defining what are all the proteins that may be found in an specific organism, i.e. a partial parts list of the organism's cellular components is now known. However, it is still far from clear how these proteins interact with one another to form the machineries that ultimately perform living functions in the cell. High-throughput experimental methods, such as yeast two hybrid (Y2H) and mass spectroscopy (MS), have been developed to screen a large number of proteins in a cell and assess their potential interactions. Yet, major drawbacks exist for these experimental methods, including the low interaction coverage, the experimental biases toward certain protein types and cellular localizations, and the high cost, both in money and time. These problems have motivated the development of computational methods as alternative and supplemental approaches to predicting protein-protein interaction (PPI). In this dissertation I propose new computational methods to address protein-protein interaction prediction. Specifically, this work tackles the following questions: given two proteins, (i) whether they interact, (ii) if they interact, where are the interacting residues, and (iii) how these interacting residues are paired up, namely the contact matrix. First, I developed a PPI predictor based on hidden Markov models (HMM) and support vector machines (SVM) to predict if two proteins interact. The method builds models of known interacting interfaces based on domain-domain interacting (DDI) families, i.e. groups of protein pairs that bind through the same pair of domains. Each interacting domain family is modeled with a HMM that differentiates interacting residues from non-interacting residues. The proposed algorithm is a two-stage pipeline that combines the flexibility of a generative learning model in the first stage--the domains' HMMs--with the differentiation power of a discriminator--a SVM--in the second stage, connected by a feature selection mechanism based on singular vector decomposition applied to the attributes extracted from domain HMMs as measured by the Fisher score. Once trained, the model can predict if two new proteins interact or not. The method significantly outperformed a previously proposed technique that uses the same input data. Second, I tackled the problem of predicting the binding/functional sites in protein-ligand and enzyme-substrate interactions. These functional residues actually correspond to a protein's binding surface that connects to a chemical, or the active (recognition) site in an enzyme. In the context of a family of related proteins, of which a quantitative measure of the functional relationships among member proteins is available, I developed statistical learning methods that predict the binding/functional residues by finding those positions in the family's multiple sequence alignment with highest correlation to the functional codification of the family. The methods utilize canonical correlation analysis (CCA), kernel CCA (kCCA) and multi-positional kCCA to incorporate non linear correlations between residues and also to analyze clusters of residues as a whole. When tested on benchmark datasets, the proposed methods significantly outperformed known algorithms that treat residues individually and independently. Third, I proposed a method to further predict how the residues are paired up across the interface, namely the contact matrix, whose rows and columns correspond to the residues in the two interacting domains respectively and whose values (1 or 0) indicate whether the corresponding residues (do or do not) interact. The method is based on the platform developed in the first part, the PPI predictor. Instead of using Fisher scores to represent the whole domains as modeled by HMMs, they are reformulated to represent individual residues. Each element of the contact matrix for a sequence-pair is now represented by a feature vector from concatenating the vectors of the two corresponding residues, and the task is to predict the element value (1 or 0) from the feature vector. The sequence-pairs in a DDI family are split into two sets, one for training and one for testing. A support vector machine is trained for a given DDI, using either a consensus contact matrix or contact matrices for individual sequence pairs. The method significantly outperformed a previous multiple sequence alignment based method. Our proposed algorithm is capable of extracting characteristic features and at the same time untying the residues from the rigid multiple sequence alignments that are used in the previous methods. This enables handling residues corresponding to delete and insert states, and allows for a supervised learning on individual contact points, eliminating the need of a consensus contact matrix for the domain families, which has been a main source for false predictions. While designed for predicting contact points between interacting protein domains, the method may be useful as a module in protein folding and docking.
Protein Protein Interaction Networks
DOWNLOAD
Author : Stefan Canzar
language : en
Publisher: Humana
Release Date : 2019-10-04
Protein Protein Interaction Networks written by Stefan Canzar and has been published by Humana this book supported file pdf, txt, epub, kindle and other format this book has been release on 2019-10-04 with Science categories.
This volume explores techniques that study interactions between proteins in different species, and combines them with context-specific data, analysis of omics datasets, and assembles individual interactions into higher-order semantic units, i.e., protein complexes and functional modules. The chapters in this book cover computational methods that solve diverse tasks such as the prediction of functional protein-protein interactions; the alignment-based comparison of interaction networks by SANA; using the RaptorX-ComplexContact webserver to predict inter-protein residue-residue contacts; the docking of alternative confirmations of proteins participating in binary interactions and the visually-guided selection of a docking model using COZOID; the detection of novel functional units by KeyPathwayMiner and how PathClass can use such de novo pathways to classify breast cancer subtypes. Written in the highly successful Methods in Molecular Biology series format, chapters include introductions to their respective topics, lists of the necessary hardware- and software, step-by-step, readily reproducible computational protocols, and tips on troubleshooting and avoiding known pitfalls. Cutting-edge and comprehensive, Protein-Protein Interaction Networks: Methods and Protocols is a valuable resource for both novice and expert researchers who are interested in learning more about this evolving field.
Protein Protein Interactions
DOWNLOAD
Author : Cheryl L. Meyerkord
language : en
Publisher: Humana
Release Date : 2016-10-29
Protein Protein Interactions written by Cheryl L. Meyerkord and has been published by Humana this book supported file pdf, txt, epub, kindle and other format this book has been release on 2016-10-29 with Science categories.
The second edition covers a wide range of protein-protein interaction detection topics. Protein-Protein Interactions: Methods and Applications focuses on core technological platforms used to study protein-protein interactions and cutting-edge technologies that reflect recent scientific advances and the emerging focus on therapeutic discovery. Written in the highly successful Methods in Molecular Biology series format, chapters include introductions to their respective topics, lists of necessary materials and reagents, step-by-step laboratory protocols, and tips on troubleshooting and avoiding known pitfalls. These well-detailed protocols describe methods for identifying protein-protein interaction partners, analyzing of protein-protein interactions quantitatively and qualitatively, monitoring protein-protein interactions in live cells, and predicting and determining interaction interfaces. Authoritative and cutting-edge, Protein-Protein Interactions: Methods and Applications, Second Edition is a valuable resource that will enable readers to elucidate the mechanisms of protein-protein interactions, determine the role of these interactions in diverse biological processes, and target protein-protein interactions for therapeutic discovery.
Protein Protein Interactions And Networks
DOWNLOAD
Author : Anna Panchenko
language : en
Publisher: Springer
Release Date : 2009-08-29
Protein Protein Interactions And Networks written by Anna Panchenko and has been published by Springer this book supported file pdf, txt, epub, kindle and other format this book has been release on 2009-08-29 with Science categories.
The biological interactions of living organisms, and protein-protein interactions in particular, are astonishingly diverse. This comprehensive book provides a broad, thorough and multidisciplinary coverage of its field. It integrates different approaches from bioinformatics, biochemistry, computational analysis and systems biology to offer the reader a comprehensive global view of the diverse data on protein-protein interactions and protein interaction networks.
A Structural Classification Of Protein Protein Interactions For Detection Of Convergently Evolved Motifs And For Prediction Of Protein Binding Sites On Sequence Level
DOWNLOAD
Author :
language : en
Publisher:
Release Date : 2002
A Structural Classification Of Protein Protein Interactions For Detection Of Convergently Evolved Motifs And For Prediction Of Protein Binding Sites On Sequence Level written by and has been published by this book supported file pdf, txt, epub, kindle and other format this book has been release on 2002 with categories.
BACKGROUND: A long-standing challenge in the post-genomic era of Bioinformatics is the prediction of protein-protein interactions, and ultimately the prediction of protein functions. The problem is intrinsically harder, when only amino acid sequences are available, but a solution is more universally applicable. So far, the problem of uncovering protein-protein interactions has been addressed in a variety of ways, both experimentally and computationally. MOTIVATION: The central problem is: How can protein complexes with solved threedimensional structure be utilized to identify and classify protein binding sites and how can knowledge be inferred from this classification such that protein interactions can be predicted for proteins without solved structure? The underlying hypothesis is that protein binding sites are often restricted to a small number of residues, which additionally often are well-conserved in order to maintain an interaction. Therefore, the signal-to-noise ratio in binding sites is expected to be higher than in other parts of the surface. This enables binding site detection in unknown proteins, when homology based annotation transfer fails. APPROACH: The problem is addressed by first investigating how geometrical aspects of domain-domain associations can lead to a rigorous structural classification of the multitude of protein interface types. The interface types are explored with respect to two aspects: First, how do interface types with one-sided homology reveal convergently evolved motifs? Second, how can sequential descriptors for local structural features be derived from the interface type classification? Then, the use of sequential representations for binding sites in order to predict protein interactions is investigated. The underlying algorithms are based on machine learning techniques, in particular Hidden Markov Models. RESULTS: This work includes a novel approach to a comprehensive geometrical classification of domain interfaces. Alternative s.
Protein Structure Prediction
DOWNLOAD
Author : David Webster
language : en
Publisher: Humana Press
Release Date : 2000-08-15
Protein Structure Prediction written by David Webster and has been published by Humana Press this book supported file pdf, txt, epub, kindle and other format this book has been release on 2000-08-15 with Science categories.
The number of protein sequences grows each year, yet the number of structures deposited in the Protein Data Bank remains relatively small. The importance of protein structure prediction cannot be overemphasized, and this volume is a timely addition to the literature in this field. Protein Structure Prediction: Methods and Protocols is a departure from the normal Methods in Molecular Biology series format. By its very nature, protein structure prediction demands that there be a greater mix of theoretical and practical aspects than is normally seen in this series. This book is aimed at both the novice and the experienced researcher who wish for detailed inf- mation in the field of protein structure prediction; a major intention here is to include important information that is needed in the day-to-day work of a research scientist, important information that is not always decipherable in scientific literature. Protein Structure Prediction: Methods and Protocols covers the topic of protein structure prediction in an eclectic fashion, detailing aspects of pred- tion that range from sequence analysis (a starting point for many algorithms) to secondary and tertiary methods, on into the prediction of docked complexes (an essential point in order to fully understand biological function). As this volume progresses, the authors contribute their expert knowledge of protein structure prediction to many disciplines, such as the identification of motifs and domains, the comparative modeling of proteins, and ab initio approaches to protein loop, side chain, and protein prediction.
Computational Prediction Of Protein Protein Interaction
DOWNLOAD
Author : Ranjan Kumar Barman
language : en
Publisher: LAP Lambert Academic Publishing
Release Date : 2011-12
Computational Prediction Of Protein Protein Interaction written by Ranjan Kumar Barman and has been published by LAP Lambert Academic Publishing this book supported file pdf, txt, epub, kindle and other format this book has been release on 2011-12 with categories.
Protein-protein interactions are extremely valuable towards protein functions and cellular processes or we can say that protein-protein interactions play an important role in living cells. Therefore, if we can control the interactions between proteins, as a result, we can control some functionality of cells. Main functionality of a protein is carried out by its domains. So, domain is a structural or/and functional unit of protein. Behind protein-protein interactions there exist some domain-domain interactions. Therefore, under standing protein-protein interaction at domain level gives a global view of protein-protein interaction network.In this book, we have made an attempt to infer domain-domain interactions from interacting and non-interacting protein pairs then we have predicted protein-protein interactions based on inferred domain-domain interactions.
Mapping Specificity Profiles And Protein Interaction Networks For Peptide Recognition Modules
DOWNLOAD
Author : Raffi Tonikian
language : en
Publisher:
Release Date : 2009
Mapping Specificity Profiles And Protein Interaction Networks For Peptide Recognition Modules written by Raffi Tonikian and has been published by this book supported file pdf, txt, epub, kindle and other format this book has been release on 2009 with categories.
Protein-protein interactions are of vital importance to the cell as they mediate the assembly of protein complexes that carry out diverse biological functions. Many proteins involved in cellular signaling are built by the combinatorial use of peptide recognition modules (PRMs), which are small protein domains that bind to their cognate ligands by recognizing short linear peptide motifs. Thousands of PRMs are found in nature, requiring improved methods to better elucidate their molecular determinants of binding and to allow accurate mapping of their interaction networks. In this thesis, I describe the development and application of phage-displayed peptide libraries to map the binding specificities of two common PRMs. First, I generated specificity profiles for 82 C. elegans and human PDZ domains that could be organized into a specificity map. The map revealed that PDZ domains have far greater substrate sequence specificity than previously believed, providing significant insights into the relationships between PDZ structure and specificity, and allowing specificity prediction for uncharacterized domains. My results were used to predict both endogenous and pathogenic PDZ interactions. This analysis revealed that viruses have evolved ligands that specifically mimic PDZ domains to subvert host cell immunity. The application of the techniques described here to other PRM families will significantly improve protein interaction maps for signaling pathways, which will illuminate our understanding of the cell circuitry, allow the use of PRMs as general affinity reagent and detection tools, and guide the development of small molecule inhibitors that mimic their peptide ligands for therapeutic intervention. Second, I analyzed the binding specificity for the SH3 domain family in S. cerevisiae. I found that, like PDZ domains, SH3 domains have binding specificities that are more detailed than the conventional classification system. The phage-derived specificity profiles were combined with data from oriented peptide and yeast two-hybrid screening to generate a highly accurate SH3 domain interaction network. Given the prominent role of SH3 domains in endocytosis, the SH3 domain interaction data was used to predict the dynamic localization of several uncharacterized endocytosis proteins, which was subsequently confirmed by cell-based assays.