Real Time Estimation Of Multivariate Stochastic Volatility Models
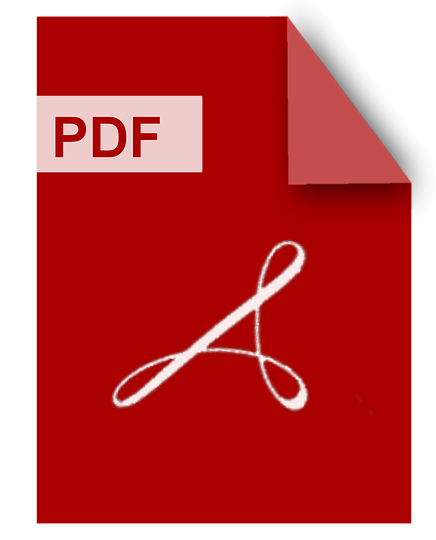
DOWNLOAD
Download Real Time Estimation Of Multivariate Stochastic Volatility Models PDF/ePub or read online books in Mobi eBooks. Click Download or Read Online button to get Real Time Estimation Of Multivariate Stochastic Volatility Models book now. This website allows unlimited access to, at the time of writing, more than 1.5 million titles, including hundreds of thousands of titles in various foreign languages. If the content not found or just blank you must refresh this page
Real Time Estimation Of Multivariate Stochastic Volatility Models
DOWNLOAD
Author : Jian Wang
language : en
Publisher:
Release Date : 2017
Real Time Estimation Of Multivariate Stochastic Volatility Models written by Jian Wang and has been published by this book supported file pdf, txt, epub, kindle and other format this book has been release on 2017 with categories.
Handbook Of Volatility Models And Their Applications
DOWNLOAD
Author : Luc Bauwens
language : en
Publisher: John Wiley & Sons
Release Date : 2012-03-22
Handbook Of Volatility Models And Their Applications written by Luc Bauwens and has been published by John Wiley & Sons this book supported file pdf, txt, epub, kindle and other format this book has been release on 2012-03-22 with Business & Economics categories.
A complete guide to the theory and practice of volatility models in financial engineering Volatility has become a hot topic in this era of instant communications, spawning a great deal of research in empirical finance and time series econometrics. Providing an overview of the most recent advances, Handbook of Volatility Models and Their Applications explores key concepts and topics essential for modeling the volatility of financial time series, both univariate and multivariate, parametric and non-parametric, high-frequency and low-frequency. Featuring contributions from international experts in the field, the book features numerous examples and applications from real-world projects and cutting-edge research, showing step by step how to use various methods accurately and efficiently when assessing volatility rates. Following a comprehensive introduction to the topic, readers are provided with three distinct sections that unify the statistical and practical aspects of volatility: Autoregressive Conditional Heteroskedasticity and Stochastic Volatility presents ARCH and stochastic volatility models, with a focus on recent research topics including mean, volatility, and skewness spillovers in equity markets Other Models and Methods presents alternative approaches, such as multiplicative error models, nonparametric and semi-parametric models, and copula-based models of (co)volatilities Realized Volatility explores issues of the measurement of volatility by realized variances and covariances, guiding readers on how to successfully model and forecast these measures Handbook of Volatility Models and Their Applications is an essential reference for academics and practitioners in finance, business, and econometrics who work with volatility models in their everyday work. The book also serves as a supplement for courses on risk management and volatility at the upper-undergraduate and graduate levels.
Estimating High Dimensional Multivariate Stochastic Volatility Models
DOWNLOAD
Author : Matteo Pelagatti
language : en
Publisher:
Release Date : 2020
Estimating High Dimensional Multivariate Stochastic Volatility Models written by Matteo Pelagatti and has been published by this book supported file pdf, txt, epub, kindle and other format this book has been release on 2020 with categories.
Multivariate Stochastic Volatility Models
DOWNLOAD
Author : Jun Yu
language : en
Publisher:
Release Date : 2004
Multivariate Stochastic Volatility Models written by Jun Yu and has been published by this book supported file pdf, txt, epub, kindle and other format this book has been release on 2004 with Bayesian statistical decision theory categories.
Shows that fully likelihood-based estimation and comparison of multivariate stochastic volatility (SV) models can be easily performed via a freely available Bayesian software called WinBUGS.
Multivariate Stochastic Volatility Models
DOWNLOAD
Author : Jón Daníelsson
language : en
Publisher:
Release Date : 1996
Multivariate Stochastic Volatility Models written by Jón Daníelsson and has been published by this book supported file pdf, txt, epub, kindle and other format this book has been release on 1996 with categories.
Analysis Of High Dimensional Multivariate Stochastic Volatility Models
DOWNLOAD
Author : Siddhartha Chib
language : en
Publisher:
Release Date : 2005
Analysis Of High Dimensional Multivariate Stochastic Volatility Models written by Siddhartha Chib and has been published by this book supported file pdf, txt, epub, kindle and other format this book has been release on 2005 with categories.
This paper is concerned with the fitting and comparison of high dimensional multivariate time series models with time varying correlations. The models considered here combine features of the classical factor model with those of the univariate stochastic volatility model. Specifically, a set of unobserved time-dependent factors, along with an associated loading matrix, are used to model the contemporaneous correlation while, conditioned on the factors, the noise in each factor and each series is assumed to follow independent three-parameter univariate stochastic volatility processes. A complete analysis of these models, and its special cases, is developed that encompasses estimation, filtering and model choice. The centerpieces of our estimation algorithm (which relies on MCMC methods) is (1) a reduced blocking scheme for sampling the free elements of the loading matrix and the factors and (2) a special method for sampling the parameters of the univariate SV process. The sampling of the loading matrix (containing typically many hundreds of parameters) is done via a highly tuned Metropolis-Hastings step. The resulting algorithm is completely scalable in terms of series and factors and very simulation-efficient. We also provide methods for estimating the log-likelihood function and the filtered values of the time-varying volatilities and correlations. We pay special attention to the problem of comparing one version of the model with another and for determining the number of factors. For this purpose we use MCMC methods to find the marginal likelihood and associated Bayes factors of each fitted model. In sum, these procedures lead to the first unified and practical likelihood based analysis of truly high dimensional models of stochastic volatility. We apply our methods in detail to two datasets. The first is the return vector on 20 exchange rates against the US Dollar. The second is the return vector on 40 common stocks quoted on the New York Stock Exchange.
Bayesian Stochastic Volatility Models
DOWNLOAD
Author : Stefanos Giakoumatos
language : en
Publisher: LAP Lambert Academic Publishing
Release Date : 2010-08
Bayesian Stochastic Volatility Models written by Stefanos Giakoumatos and has been published by LAP Lambert Academic Publishing this book supported file pdf, txt, epub, kindle and other format this book has been release on 2010-08 with categories.
The phenomenon of changing variance and covariance is often encountered in financial time series. As a result, during the last years researchers focused on the time-varying volatility models. These models are able to describe the main characteristics of the financial data such as the volatility clustering. In addition, the development of the Markov Chain Monte Carlo Techniques (MCMC) provides a powerful tool for the estimation of the parameters of the time-varying volatility models, in the context of Bayesian analysis. In this thesis, we adopt the Bayesian inference and we propose easy-to-apply MCMC algorithms for a variety of time-varying volatility models. We use a recent development in the context of the MCMC techniques, the Auxiliary variable sampler. This technique enables us to construct MCMC algorithms, which only consist of Gibbs steps. We propose new MCMC algorithms for many univariate and multivariate models. Furthermore, we apply the proposed MCMC algorithms to real data and compare the above models based on their predictive distribution
Multivariate Stochastic Volatility Models With Correlated Errors
DOWNLOAD
Author : David X. Chan
language : en
Publisher:
Release Date : 2008
Multivariate Stochastic Volatility Models With Correlated Errors written by David X. Chan and has been published by this book supported file pdf, txt, epub, kindle and other format this book has been release on 2008 with categories.
We develop a Bayesian approach for parsimoniously estimating the correlation structure of the errors in a multivariate stochastic volatility model. Since the number of parameters in the joint correlation matrix of the return and volatility errors is potentially very large, we impose a prior that allows the off-diagonal elements of the inverse of the correlation matrix to be identically zero. The model is estimated using a Markov chain simulation method that samples from the posterior distribution of the volatilities and parameters. We illustrate the approach using both simulated and real examples. In the real examples, the method is applied to equities at three levels of aggregation: returns for firms within the same industry, returns for different industries and returns aggregated at the index level. We find pronounced correlation effects only at the highest level of aggregation.
Bayesian Inference For Stochastic Volatility Models
DOWNLOAD
Author : Zhongxian Men
language : en
Publisher:
Release Date : 2012
Bayesian Inference For Stochastic Volatility Models written by Zhongxian Men and has been published by this book supported file pdf, txt, epub, kindle and other format this book has been release on 2012 with categories.
Stochastic volatility (SV) models provide a natural framework for a representation of time series for financial asset returns. As a result, they have become increasingly popular in the finance literature, although they have also been applied in other fields such as signal processing, telecommunications, engineering, biology, and other areas. In working with the SV models, an important issue arises as how to estimate their parameters efficiently and to assess how well they fit real data. In the literature, commonly used estimation methods for the SV models include general methods of moments, simulated maximum likelihood methods, quasi Maximum likelihood method, and Markov Chain Monte Carlo (MCMC) methods. Among these approaches, MCMC methods are most flexible in dealing with complicated structure of the models. However, due to the difficulty in the selection of the proposal distribution for Metropolis-Hastings methods, in general they are not easy to implement and in some cases we may also encounter convergence problems in the implementation stage. In the light of these concerns, we propose in this thesis new estimation methods for univariate and multivariate SV models.
Garch Models
DOWNLOAD
Author : Christian Francq
language : en
Publisher: John Wiley & Sons
Release Date : 2019-03-21
Garch Models written by Christian Francq and has been published by John Wiley & Sons this book supported file pdf, txt, epub, kindle and other format this book has been release on 2019-03-21 with Mathematics categories.
Provides a comprehensive and updated study of GARCH models and their applications in finance, covering new developments in the discipline This book provides a comprehensive and systematic approach to understanding GARCH time series models and their applications whilst presenting the most advanced results concerning the theory and practical aspects of GARCH. The probability structure of standard GARCH models is studied in detail as well as statistical inference such as identification, estimation, and tests. The book also provides new coverage of several extensions such as multivariate models, looks at financial applications, and explores the very validation of the models used. GARCH Models: Structure, Statistical Inference and Financial Applications, 2nd Edition features a new chapter on Parameter-Driven Volatility Models, which covers Stochastic Volatility Models and Markov Switching Volatility Models. A second new chapter titled Alternative Models for the Conditional Variance contains a section on Stochastic Recurrence Equations and additional material on EGARCH, Log-GARCH, GAS, MIDAS, and intraday volatility models, among others. The book is also updated with a more complete discussion of multivariate GARCH; a new section on Cholesky GARCH; a larger emphasis on the inference of multivariate GARCH models; a new set of corrected problems available online; and an up-to-date list of references. Features up-to-date coverage of the current research in the probability, statistics, and econometric theory of GARCH models Covers significant developments in the field, especially in multivariate models Contains completely renewed chapters with new topics and results Handles both theoretical and applied aspects Applies to researchers in different fields (time series, econometrics, finance) Includes numerous illustrations and applications to real financial series Presents a large collection of exercises with corrections Supplemented by a supporting website featuring R codes, Fortran programs, data sets and Problems with corrections GARCH Models, 2nd Edition is an authoritative, state-of-the-art reference that is ideal for graduate students, researchers, and practitioners in business and finance seeking to broaden their skills of understanding of econometric time series models.