Real Time Sentiment Based Anomaly Detection In Twitter Data Streams
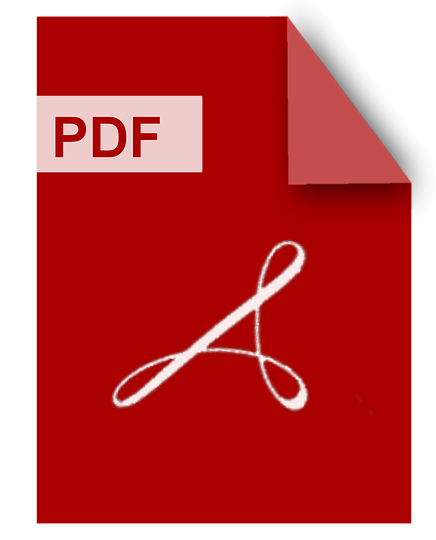
DOWNLOAD
Download Real Time Sentiment Based Anomaly Detection In Twitter Data Streams PDF/ePub or read online books in Mobi eBooks. Click Download or Read Online button to get Real Time Sentiment Based Anomaly Detection In Twitter Data Streams book now. This website allows unlimited access to, at the time of writing, more than 1.5 million titles, including hundreds of thousands of titles in various foreign languages. If the content not found or just blank you must refresh this page
Real Time Sentiment Based Anomaly Detection In Twitter Data Streams
DOWNLOAD
Author : Khantil Ragnesh Patel
language : en
Publisher:
Release Date : 2016
Real Time Sentiment Based Anomaly Detection In Twitter Data Streams written by Khantil Ragnesh Patel and has been published by this book supported file pdf, txt, epub, kindle and other format this book has been release on 2016 with categories.
Twitter has over 316 million active users and the engagement of these Twitter users results in the rapid production of data, notably in the context of popular topics (such as news stories, politics, and sports). This data is available in the form of data streams, which has led many researchers to develop analysis techniques especially for Twitter data streams. Although anomaly detection in time series is a well established research area, its application to detect sentiment-based anomalies in large volumes of streaming data began recently. A sentiment-based anomaly is de ned as a sudden increase in the time series of tweets individually associated with a positive, neutral, or negative sentiment. The goal of this research is to develop and evaluate a technique to automatically detect sentiment-based anomalies, while avoiding the repeated detection of anomalies of similar types. Detecting anomalies in data streams is challenging due the requirement that anomalies be detected in real-time. We propose an approach for real-time sentiment-based anomaly detection (RSAD) in Twitter data streams. Sentiment classi cation is used to split the input data stream into three independent streams (positive, neutral, and negative), which are then analyzed separately for anomalous spikes in the number of tweets. Rare anomalies and the rst occurrence of repeated anomalies are distinguished from the repeated occurrence of similar anomalies. Six approaches for anomaly detection in data streams, including two baseline approaches, are described. These approaches were tested on two user-generated datasets. The rst dataset concerned an international sports event and was collected from Twitter and the second concerned a political party and was collected from multiple social media platforms. Results from these evaluations show that a probabilistic exponentially weighted moving average (PEWMA), coupled with a sliding window that uses a median absolute deviation (MAD) calculation, is effective at identifying sentiment-based anomalies. The PEWMA-MAD approach is consistently among the top two methods for all cases tested. The simple linear regression approach is slightly better in the case of the second dataset. Overall, the results suggest that the PEWMA-MAD approach may be robust su ciently to be applied to a wide variety of datasets from di erent social media platforms. ii.
Advances In Artificial Intelligence
DOWNLOAD
Author : Denilson Barbosa
language : en
Publisher: Springer
Release Date : 2015-04-28
Advances In Artificial Intelligence written by Denilson Barbosa and has been published by Springer this book supported file pdf, txt, epub, kindle and other format this book has been release on 2015-04-28 with Computers categories.
This book constitutes the refereed proceedings of the 28th Canadian Conference on Artificial Intelligence, Canadian AI 2015, held in Halifax, Nova Scotia, Canada, in June 2015.The 15 regular papers and 12 short papers presented together with 8 papers from the Graduate Student Symposium were carefully reviewed and selected from 81 submissions. The papers are organized in topical sections such as agents, uncertainty and games; AI applications; NLP, text and social media mining; data mining and machine learning.
Integrated Real Time Social Media Sentiment Analysis Service Using A Big Data Analytic Ecosystem
DOWNLOAD
Author : Danielle C. Aring
language : en
Publisher:
Release Date : 2017
Integrated Real Time Social Media Sentiment Analysis Service Using A Big Data Analytic Ecosystem written by Danielle C. Aring and has been published by this book supported file pdf, txt, epub, kindle and other format this book has been release on 2017 with categories.
Big data analytics are at the center of modern science and business. Our social media networks, mobile devices and enterprise systems generate enormous volumes of it on a daily basis. This wide range of availability provides many organizations in every field opportunities to discover valuable intelligence for critical decision-making. However, traditional analytic architectures are insufficient to handle unprecedentedly big volume of data and complexity of data processing.This thesis presents an analytic framework to combat unprecedented scale of big data that performs data stream sentiment analysis effectively in real time. The work presents a Social Media Big Data Sentiment Analytics Service System (SMBDSASS). The architecture leverages Apache Spark stream data processing framework, coupled with a NoSQL Hive big data ecosystem. Two sentiment analysis models were developed; the first, a topic based model, given user provided topic or person of interest sentiment (opinion) analysis was performed on related topic sentences in a tweet stream. The second, an aspect (feature) based model given user provided product of interest and related product features aspect (feature) analysis was performed on reviews containing important feature terms.The experimental results of the proposed framework using real time tweet stream and product reviews show comparable improvements from the results of the existing literature, with 73% accuracy for topic-based sentiment model, and 74% accuracy for aspect (feature) based sentiment model.The work demonstrated that our topic and aspect based sentiment analysis models on the real time stream data processing framework using Apache Spark and machine learning classifiers coupled with a NoSQL big data ecosystem offer an efficient, scalable, real-time stream data-processing alternative for the complex multiphase sentiment analysis over common batch data mining frameworks.
Strategic Risk Intelligence And Digital Transformation
DOWNLOAD
Author : Eduardo Rodriguez
language : en
Publisher: World Scientific
Release Date : 2024-03-19
Strategic Risk Intelligence And Digital Transformation written by Eduardo Rodriguez and has been published by World Scientific this book supported file pdf, txt, epub, kindle and other format this book has been release on 2024-03-19 with Business & Economics categories.
In this book, the study of strategic risk is not only for its control and mitigation using analytics and digital transformation in organizations, but also it is about the strategic risks that digital transformation can bring to organizations. Strategic risk control is one of the goals in creating intelligent organizations and at the same time it is part of the appetite for creating smarter organizations to support organizations' development. Knowledge that is created by data analytics and the capacity to operationalize that knowledge through digital transformation can produce potential sustainable competitive advantages.The core of the volume is connecting data analytics and artificial intelligence, risk management and digitalization to create strategic intelligence as the capacity of adaptation that organizations need to compete and to succeed. Strategic intelligence is a symbiotic work of artificial intelligence, business intelligence and competitive intelligence. Strategic risk is represented by the probability of having variations in the performance results of the organizations that can limit their capacity to maintain sustainable competitive advantages. There is an emphasis in the book about the conversion of models that support data analytics into actions to mitigate strategic risk based on digital transformation.This book reviews the steps that organizations have taken in using technology that connects the data analytics modeling process and digital operations, such as the shift from the use of statistical learning and machine learning for data analytics to the improvement and use of new technologies. The digitalization process is a potential opportunity for organizations however the results are not necessarily good for everyone. Hence, organizations implement strategic risk control in cloud computing, blockchain, artificial intelligence and create digital networks that are connected internally and externally to deal with internal and external customers, with suppliers and buyers, and with competitors and substitutes. The new risks appear once new knowledge emerges and is in use, but at the same time the new knowledge supports the initiatives to deal with risks arising from novel ways of competing and collaborating.
Developing A Real Time Data Analytics Framework For Twitter Streaming Data
DOWNLOAD
Author : Babak Yadranjiaghdam
language : en
Publisher:
Release Date : 2016
Developing A Real Time Data Analytics Framework For Twitter Streaming Data written by Babak Yadranjiaghdam and has been published by this book supported file pdf, txt, epub, kindle and other format this book has been release on 2016 with categories.
Twitter is an online social networking service with more than 300 million users, generating a huge amount of information every day. Twitter's most important characteristic is its ability for users to tweet about events, situations, feelings, opinions, or even something totally new, in real time. Currently there are different workflows offering real-time data analysis for Twitter, presenting general processing over streaming data. This study will attempt to develop an analytical framework with the ability of in-memory processing to extract and analyze structured and unstructured Twitter data. The proposed framework includes data ingestion and stream processing and data visualization components with the Apache Kafka messaging system that is used to perform data ingestion task. Furthermore, Spark makes it possible to perform sophisticated data processing and machine learning algorithms in real time. We have conducted a case study on tweets about the earthquake in Japan and the reactions of people around the world with analysis on the time and origin of the tweets.
Routledge Handbook Of Sustainable And Resilient Infrastructure
DOWNLOAD
Author : Paolo Gardoni
language : en
Publisher: Routledge
Release Date : 2018-12-17
Routledge Handbook Of Sustainable And Resilient Infrastructure written by Paolo Gardoni and has been published by Routledge this book supported file pdf, txt, epub, kindle and other format this book has been release on 2018-12-17 with Business & Economics categories.
To best serve current and future generations, infrastructure needs to be resilient to the changing world while using limited resources in a sustainable manner. Research on and funding towards sustainability and resilience are growing rapidly, and significant research is being carried out at a number of institutions and centers worldwide. This handbook brings together current research on sustainable and resilient infrastructure and, in particular, stresses the fundamental nexus between sustainability and resilience. It aims to coalesce work from a large and diverse group of contributors across a wide range of disciplines including engineering, technology and informatics, urban planning, public policy, economics, and finance. Not only does it present a theoretical formulation of sustainability and resilience but it also demonstrates how these ideals can be realized in practice. This work will provide a reference text to students and scholars of a number of disciplines.
Visual Sentiment Analysis On Twitter Data Streams
DOWNLOAD
Author : Ming Hao
language : en
Publisher:
Release Date : 2012
Visual Sentiment Analysis On Twitter Data Streams written by Ming Hao and has been published by this book supported file pdf, txt, epub, kindle and other format this book has been release on 2012 with categories.
End To End Anomaly Detection In Stream Data
DOWNLOAD
Author : Zahra Zohrevand
language : en
Publisher:
Release Date : 2020
End To End Anomaly Detection In Stream Data written by Zahra Zohrevand and has been published by this book supported file pdf, txt, epub, kindle and other format this book has been release on 2020 with categories.
Nowadays, huge volumes of data are generated with increasing velocity through various systems, applications, and activities. This increases the demand for stream and time series analysis to react to changing conditions in real-time for enhanced efficiency and quality of service delivery as well as upgraded safety and security in private and public sectors. Despite its very rich history, time series anomaly detection is still one of the vital topics in machine learning research and is receiving increasing attention. Identifying hidden patterns and selecting an appropriate model that fits the observed data well and also carries over to unobserved data is not a trivial task. Due to the increasing diversity of data sources and associated stochastic processes, this pivotal data analysis topic is loaded with various challenges like complex latent patterns, concept drift, and overfitting that may mislead the model and cause a high false alarm rate. Handling these challenges leads the advanced anomaly detection methods to develop sophisticated decision logic, which turns them into mysterious and inexplicable black-boxes. Contrary to this trend, end-users expect transparency and verifiability to trust a model and the outcomes it produces. Also, pointing the users to the most anomalous/malicious areas of time series and causal features could save them time, energy, and money. For the mentioned reasons, this thesis is addressing the crucial challenges in an end-to-end pipeline of stream-based anomaly detection through the three essential phases of behavior prediction, inference, and interpretation. The first step is focused on devising a time series model that leads to high average accuracy as well as small error deviation. On this basis, we propose higher-quality anomaly detection and scoring techniques that utilize the related contexts to reclassify the observations and post-pruning the unjustified events. Last but not least, we make the predictive process transparent and verifiable by providing meaningful reasoning behind its generated results based on the understandable concepts by a human. The provided insight can pinpoint the anomalous regions of time series and explain why the current status of a system has been flagged as anomalous. Stream-based anomaly detection research is a principal area of innovation to support our economy, security, and even the safety and health of societies worldwide. We believe our proposed analysis techniques can contribute to building a situational awareness platform and open new perspectives in a variety of domains like cybersecurity, and health.
Collecting Mining And Analyzing University Related Tweets Using Sentiment Analysis Based On Machine Learning Algorithms
DOWNLOAD
Author :
language : en
Publisher:
Release Date : 2020
Collecting Mining And Analyzing University Related Tweets Using Sentiment Analysis Based On Machine Learning Algorithms written by and has been published by this book supported file pdf, txt, epub, kindle and other format this book has been release on 2020 with Data mining categories.
Due to the rapid growth of Social Media Platforms usage as a medium for providing views, feedbacks, and opinions, a tremendous amount of informal statements on various academic institutes are created. As a social platform, Twitter has high accessibility, reducing the stress of freely providing personal opinions performing a vital source for Opinion Mining and Sentiment Analysis. Thus, public discourses on Twitter based on opinions and views in the context of topics and events related to HEIs can generate an extensive amount of informal statements, providing valuable insights into the HEIs environments. However, this informal statement is still unexplored by HEIs policymakers. Hence, this research focuses on Mining and Analyzing the informal statements published as Tweets related to HEIs, in contrast with the surveys, interviews, or the Unit of Study Evaluation questionnaires as a traditional data collection method. This analysis is essential to understand individuals' views, sentiment, comments, mind-setup towards a university. This will provide vital insights related to the overall academic, institutitional, and social experience among Twitter users in the context of HEIs. This dissertation introduced a Twitter Data Collection and Extraction method incorporating a comprehensive guideline to automatically collect Twitter users' real-time streamed Tweets in the context of HEIs based on the regular and extended Tweets (up to 280 char), using the Twitter API. This established a novel University-related Twitter Dataset-Michigan (UTD-MI), by collecting tweets related to some selected HEIs in the State of Michigan. We further automatically analyzed the sentiment of the collected Tweets by employing Sentiment Analysis methods based on the Supervised Machine Learning (ML) algorithms to classify the Tweets into "Positive," "Negative," or "Neutral". Accordingly, the percentage of "Positive" Tweets was used to carry out a comparison between the selected universities. We implemented six ML classifiers including the Voting classifier on the extracted feature which is based on a feature selection technique using Part-of-Speech (PoS) Tagging with polarity scores from the SentiWordNet or the VADER lexicons. As a result, we achieved a high accuracy of 93% by the SVM classifier. Moreover, we semantically categorized the collected Tweets into academic and social contexts to provide vital insights on which context and topic the expressed opinions and feedback were when classified in terms of their sentiment. The results of this research can be utilized by HEIs policymakers for further modifications and adjusting plans to improve their overall educational environments. In addition, university comparison and evaluation results can be enhanced using such vital indicators perceived from social media, establishing a new measurement of the reputation indicator in HEIs ranking systems. As a conclusion, this will serve as a complementary source for evaluating and comparing universities.
Database And Expert Systems Applications
DOWNLOAD
Author : Christine Strauss
language : en
Publisher: Springer Nature
Release Date : 2021-08-31
Database And Expert Systems Applications written by Christine Strauss and has been published by Springer Nature this book supported file pdf, txt, epub, kindle and other format this book has been release on 2021-08-31 with Computers categories.
This two-volume set, LNCS 12923 and 12924, constitutes the thoroughly refereed proceedings of the 5th International Conference on Database and Expert Systems Applications, DEXA 2021. Due to COVID-19 pandemic, the conference was held virtually. The 37 full papers presented together with 31 short papers in these volumes were carefully reviewed and selected from a total of 149 submissions. The papers are organized around the following topics: big data; data analysis and data modeling; data mining; databases and data management; information retrieval; prediction and decision support.