Rigorous Optimization Recipes For Sparse And Low Rank Inverse Problems With Applications In Data Sciences
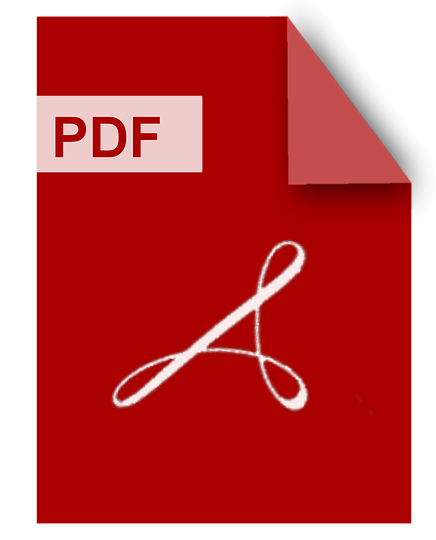
DOWNLOAD
Download Rigorous Optimization Recipes For Sparse And Low Rank Inverse Problems With Applications In Data Sciences PDF/ePub or read online books in Mobi eBooks. Click Download or Read Online button to get Rigorous Optimization Recipes For Sparse And Low Rank Inverse Problems With Applications In Data Sciences book now. This website allows unlimited access to, at the time of writing, more than 1.5 million titles, including hundreds of thousands of titles in various foreign languages. If the content not found or just blank you must refresh this page
Rigorous Optimization Recipes For Sparse And Low Rank Inverse Problems With Applications In Data Sciences
DOWNLOAD
Author : Anastasios Kyrillidis
language : en
Publisher:
Release Date : 2014
Rigorous Optimization Recipes For Sparse And Low Rank Inverse Problems With Applications In Data Sciences written by Anastasios Kyrillidis and has been published by this book supported file pdf, txt, epub, kindle and other format this book has been release on 2014 with categories.
High Dimensional Probability
DOWNLOAD
Author : Roman Vershynin
language : en
Publisher: Cambridge University Press
Release Date : 2018-09-27
High Dimensional Probability written by Roman Vershynin and has been published by Cambridge University Press this book supported file pdf, txt, epub, kindle and other format this book has been release on 2018-09-27 with Business & Economics categories.
An integrated package of powerful probabilistic tools and key applications in modern mathematical data science.
Handbook Of Variational Methods For Nonlinear Geometric Data
DOWNLOAD
Author : Philipp Grohs
language : en
Publisher: Springer Nature
Release Date : 2020-04-03
Handbook Of Variational Methods For Nonlinear Geometric Data written by Philipp Grohs and has been published by Springer Nature this book supported file pdf, txt, epub, kindle and other format this book has been release on 2020-04-03 with Mathematics categories.
This book covers different, current research directions in the context of variational methods for non-linear geometric data. Each chapter is authored by leading experts in the respective discipline and provides an introduction, an overview and a description of the current state of the art. Non-linear geometric data arises in various applications in science and engineering. Examples of nonlinear data spaces are diverse and include, for instance, nonlinear spaces of matrices, spaces of curves, shapes as well as manifolds of probability measures. Applications can be found in biology, medicine, product engineering, geography and computer vision for instance. Variational methods on the other hand have evolved to being amongst the most powerful tools for applied mathematics. They involve techniques from various branches of mathematics such as statistics, modeling, optimization, numerical mathematics and analysis. The vast majority of research on variational methods, however, is focused on data in linear spaces. Variational methods for non-linear data is currently an emerging research topic. As a result, and since such methods involve various branches of mathematics, there is a plethora of different, recent approaches dealing with different aspects of variational methods for nonlinear geometric data. Research results are rather scattered and appear in journals of different mathematical communities. The main purpose of the book is to account for that by providing, for the first time, a comprehensive collection of different research directions and existing approaches in this context. It is organized in a way that leading researchers from the different fields provide an introductory overview of recent research directions in their respective discipline. As such, the book is a unique reference work for both newcomers in the field of variational methods for non-linear geometric data, as well as for established experts that aim at to exploit new research directions or collaborations. Chapter 9 of this book is available open access under a CC BY 4.0 license at link.springer.com.
Optimization Models
DOWNLOAD
Author : Giuseppe C. Calafiore
language : en
Publisher: Cambridge University Press
Release Date : 2014-10-31
Optimization Models written by Giuseppe C. Calafiore and has been published by Cambridge University Press this book supported file pdf, txt, epub, kindle and other format this book has been release on 2014-10-31 with Business & Economics categories.
This accessible textbook demonstrates how to recognize, simplify, model and solve optimization problems - and apply these principles to new projects.
Iterative Methods For Sparse Linear Systems
DOWNLOAD
Author : Yousef Saad
language : en
Publisher: SIAM
Release Date : 2003-04-01
Iterative Methods For Sparse Linear Systems written by Yousef Saad and has been published by SIAM this book supported file pdf, txt, epub, kindle and other format this book has been release on 2003-04-01 with Mathematics categories.
Mathematics of Computing -- General.
Bayesian Approach To Inverse Problems
DOWNLOAD
Author : Jérôme Idier
language : en
Publisher: John Wiley & Sons
Release Date : 2013-03-01
Bayesian Approach To Inverse Problems written by Jérôme Idier and has been published by John Wiley & Sons this book supported file pdf, txt, epub, kindle and other format this book has been release on 2013-03-01 with Mathematics categories.
Many scientific, medical or engineering problems raise the issue of recovering some physical quantities from indirect measurements; for instance, detecting or quantifying flaws or cracks within a material from acoustic or electromagnetic measurements at its surface is an essential problem of non-destructive evaluation. The concept of inverse problems precisely originates from the idea of inverting the laws of physics to recover a quantity of interest from measurable data. Unfortunately, most inverse problems are ill-posed, which means that precise and stable solutions are not easy to devise. Regularization is the key concept to solve inverse problems. The goal of this book is to deal with inverse problems and regularized solutions using the Bayesian statistical tools, with a particular view to signal and image estimation. The first three chapters bring the theoretical notions that make it possible to cast inverse problems within a mathematical framework. The next three chapters address the fundamental inverse problem of deconvolution in a comprehensive manner. Chapters 7 and 8 deal with advanced statistical questions linked to image estimation. In the last five chapters, the main tools introduced in the previous chapters are put into a practical context in important applicative areas, such as astronomy or medical imaging.
The Data Science Design Manual
DOWNLOAD
Author : Steven S. Skiena
language : en
Publisher: Springer
Release Date : 2017-07-01
The Data Science Design Manual written by Steven S. Skiena and has been published by Springer this book supported file pdf, txt, epub, kindle and other format this book has been release on 2017-07-01 with Computers categories.
This engaging and clearly written textbook/reference provides a must-have introduction to the rapidly emerging interdisciplinary field of data science. It focuses on the principles fundamental to becoming a good data scientist and the key skills needed to build systems for collecting, analyzing, and interpreting data. The Data Science Design Manual is a source of practical insights that highlights what really matters in analyzing data, and provides an intuitive understanding of how these core concepts can be used. The book does not emphasize any particular programming language or suite of data-analysis tools, focusing instead on high-level discussion of important design principles. This easy-to-read text ideally serves the needs of undergraduate and early graduate students embarking on an “Introduction to Data Science” course. It reveals how this discipline sits at the intersection of statistics, computer science, and machine learning, with a distinct heft and character of its own. Practitioners in these and related fields will find this book perfect for self-study as well. Additional learning tools: Contains “War Stories,” offering perspectives on how data science applies in the real world Includes “Homework Problems,” providing a wide range of exercises and projects for self-study Provides a complete set of lecture slides and online video lectures at www.data-manual.com Provides “Take-Home Lessons,” emphasizing the big-picture concepts to learn from each chapter Recommends exciting “Kaggle Challenges” from the online platform Kaggle Highlights “False Starts,” revealing the subtle reasons why certain approaches fail Offers examples taken from the data science television show “The Quant Shop” (www.quant-shop.com)
Practical Statistics For Data Scientists
DOWNLOAD
Author : Peter Bruce
language : en
Publisher: "O'Reilly Media, Inc."
Release Date : 2017-05-10
Practical Statistics For Data Scientists written by Peter Bruce and has been published by "O'Reilly Media, Inc." this book supported file pdf, txt, epub, kindle and other format this book has been release on 2017-05-10 with Computers categories.
Statistical methods are a key part of of data science, yet very few data scientists have any formal statistics training. Courses and books on basic statistics rarely cover the topic from a data science perspective. This practical guide explains how to apply various statistical methods to data science, tells you how to avoid their misuse, and gives you advice on what's important and what's not. Many data science resources incorporate statistical methods but lack a deeper statistical perspective. If you’re familiar with the R programming language, and have some exposure to statistics, this quick reference bridges the gap in an accessible, readable format. With this book, you’ll learn: Why exploratory data analysis is a key preliminary step in data science How random sampling can reduce bias and yield a higher quality dataset, even with big data How the principles of experimental design yield definitive answers to questions How to use regression to estimate outcomes and detect anomalies Key classification techniques for predicting which categories a record belongs to Statistical machine learning methods that “learn” from data Unsupervised learning methods for extracting meaning from unlabeled data
Optimization Algorithms On Matrix Manifolds
DOWNLOAD
Author : P.-A. Absil
language : en
Publisher: Princeton University Press
Release Date : 2009-04-11
Optimization Algorithms On Matrix Manifolds written by P.-A. Absil and has been published by Princeton University Press this book supported file pdf, txt, epub, kindle and other format this book has been release on 2009-04-11 with Mathematics categories.
Many problems in the sciences and engineering can be rephrased as optimization problems on matrix search spaces endowed with a so-called manifold structure. This book shows how to exploit the special structure of such problems to develop efficient numerical algorithms. It places careful emphasis on both the numerical formulation of the algorithm and its differential geometric abstraction--illustrating how good algorithms draw equally from the insights of differential geometry, optimization, and numerical analysis. Two more theoretical chapters provide readers with the background in differential geometry necessary to algorithmic development. In the other chapters, several well-known optimization methods such as steepest descent and conjugate gradients are generalized to abstract manifolds. The book provides a generic development of each of these methods, building upon the material of the geometric chapters. It then guides readers through the calculations that turn these geometrically formulated methods into concrete numerical algorithms. The state-of-the-art algorithms given as examples are competitive with the best existing algorithms for a selection of eigenspace problems in numerical linear algebra. Optimization Algorithms on Matrix Manifolds offers techniques with broad applications in linear algebra, signal processing, data mining, computer vision, and statistical analysis. It can serve as a graduate-level textbook and will be of interest to applied mathematicians, engineers, and computer scientists.
Large Scale Inverse Problems
DOWNLOAD
Author : Mike Cullen
language : en
Publisher: Walter de Gruyter
Release Date : 2013-08-29
Large Scale Inverse Problems written by Mike Cullen and has been published by Walter de Gruyter this book supported file pdf, txt, epub, kindle and other format this book has been release on 2013-08-29 with Mathematics categories.
This book is thesecond volume of a three volume series recording the "Radon Special Semester 2011 on Multiscale Simulation & Analysis in Energy and the Environment" that took placein Linz, Austria, October 3-7, 2011. This volume addresses the common ground in the mathematical and computational procedures required for large-scale inverse problems and data assimilation in forefront applications. The solution of inverse problems is fundamental to a wide variety of applications such as weather forecasting, medical tomography, and oil exploration. Regularisation techniques are needed to ensure solutions of sufficient quality to be useful, and soundly theoretically based. This book addresses the common techniques required for all the applications, and is thus truly interdisciplinary. Thiscollection of surveyarticlesfocusses onthe large inverse problems commonly arising in simulation and forecasting in the earth sciences. For example, operational weather forecasting models have between 107 and 108 degrees of freedom. Even so, these degrees of freedom represent grossly space-time averaged properties of the atmosphere. Accurate forecasts require accurate initial conditions. With recent developments in satellite data, there are between 106 and 107 observations each day. However, while these also represent space-time averaged properties, the averaging implicit in the measurements is quite different from that used in the models. In atmosphere and ocean applications, there is a physically-based model available which can be used to regularise the problem. We assume that there is a set of observations with known error characteristics available over a period of time. The basic deterministic technique is to fit a model trajectory to the observations over a period of time to within the observation error. Since the model is not perfect the model trajectory has to be corrected, which defines the data assimilation problem. The stochastic view can be expressed by using an ensemble of model trajectories, and calculating corrections to both the mean value and the spread which allow the observations to be fitted by each ensemble member. In other areas of earth science, only the structure of the model formulation itself is known and the aim is to use the past observation history to determine the unknown model parameters. The book records the achievements of Workshop2 "Large-Scale Inverse Problems and Applications in the Earth Sciences". Itinvolves experts in the theory of inverse problems together with experts working on both theoretical and practical aspects of the techniques by which large inverse problems arise in the earth sciences.