Robust Decision Making Applied To Model Selection
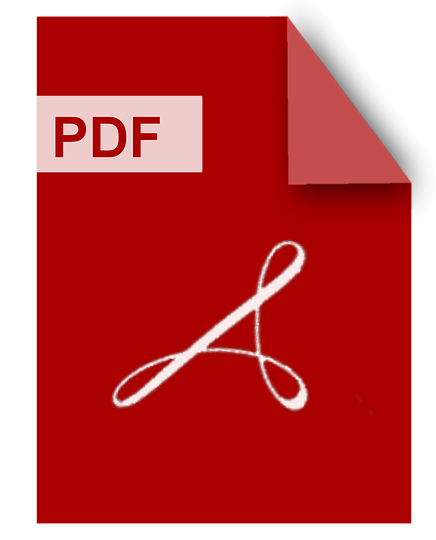
DOWNLOAD
Download Robust Decision Making Applied To Model Selection PDF/ePub or read online books in Mobi eBooks. Click Download or Read Online button to get Robust Decision Making Applied To Model Selection book now. This website allows unlimited access to, at the time of writing, more than 1.5 million titles, including hundreds of thousands of titles in various foreign languages. If the content not found or just blank you must refresh this page
Robust Decision Making Applied To Model Selection
DOWNLOAD
Author :
language : en
Publisher:
Release Date : 2012
Robust Decision Making Applied To Model Selection written by and has been published by this book supported file pdf, txt, epub, kindle and other format this book has been release on 2012 with categories.
The scientific and engineering communities are relying more and more on numerical models to simulate ever-increasingly complex phenomena. Selecting a model, from among a family of models that meets the simulation requirements, presents a challenge to modern-day analysts. To address this concern, a framework is adopted anchored in info-gap decision theory. The framework proposes to select models by examining the trade-offs between prediction accuracy and sensitivity to epistemic uncertainty. The framework is demonstrated on two structural engineering applications by asking the following question: Which model, of several numerical models, approximates the behavior of a structure when parameters that define each of those models are unknown? One observation is that models that are nominally more accurate are not necessarily more robust, and their accuracy can deteriorate greatly depending upon the assumptions made. It is posited that, as reliance on numerical models increases, establishing robustness will become as important as demonstrating accuracy.
Making Robust Decisions
DOWNLOAD
Author : David G. Ullman
language : en
Publisher: Trafford on Demand Pub
Release Date : 2006
Making Robust Decisions written by David G. Ullman and has been published by Trafford on Demand Pub this book supported file pdf, txt, epub, kindle and other format this book has been release on 2006 with Business & Economics categories.
How do you approach difficult decisions? Decision making is an integral part of business and technology, as well as almost every other facet of life. Now there is a uniquely practical book that can help you tackle your next decision with confidence. In Making Robust Decisions: Decision Management for Business, Service, and Technical Teams, you will learn: why decision making can be so difficult; how to address the challenges that uncertain, conflicting, incomplete, or evolving information present; and how to achieve robust decisions despite the varied personalities and perspectives on your team. Combining more than ten years of study of decision support, cognitive psychology, product development, and business management with modern Artificial Intelligence concepts, Making Robust Decisions gives you the tools you need to produce optimal decisions—those that make good use of available information, achieve buy-in from all parties, and yield the best possible results. Packed with practical examples and case studies, Making Robust Decisions strikes a middle ground between self-help books that, while interesting in theory, may not help with real-world problems and highly technical analysis texts. It provides some methods you can implement right away and others that you and your organization can grow into. It is readable, useful, and readily applicable to a wide variety of decision-making problems. The methods introduced in Making Robust Decisions can help with such varied issues as selecting a concept, managing a portfolio, choosing a vendor, evaluating a proposal, selecting from architecture options, choosing a design, and determining whether to make or buy an item. They support military selection of the best course of action (COA), Analysis of Alternatives (AoA), and homeland security strategies. Making Robust Decisions includes chapters on making estimates, working with decision teams, framing problems, the influence of belief, and using AccordÔ decision-making software to support robust decisions. It includes decision-making templates and demonstrates how the methods described support Design for Six Sigma practitioners and provide help in un-sticking the OODA Loop. If you’re in the business of making difficult decisions while managing uncertainty, risk, and team conflict, then discover the new, effective techniques presented in Making Robust Decisions.
Robust Decision Making With The Same Decision Probability
DOWNLOAD
Author : Suming Jeremiah Chen
language : en
Publisher:
Release Date : 2015
Robust Decision Making With The Same Decision Probability written by Suming Jeremiah Chen and has been published by this book supported file pdf, txt, epub, kindle and other format this book has been release on 2015 with categories.
When making decisions under uncertainty, the optimal choices are often difficult to discern, especially if not enough information has been gathered. Two key questions in this regard relate to whether one should stop the information gathering process and commit to a decision (stopping criterion), and if not, what information to gather next (selection criterion). The proposed thesis is concerned with addressing this problem in light of a new advance, known as the Same--Decision Probability (SDP), which is the probability that we would make the same decision had we known what we currently do not know. In this thesis, we show how the SDP can be used to be an effective stopping criterion, and compare it to traditional criteria to demonstrate how it provides a fresh perspective in decision making under uncertainty. Additionally, we develop the first exact algorithm to compute the SDP so that it may be used as a stopping criterion. We demonstrate the effectiveness of these algorithms on real and synthetic networks, and show that our proposed stopping criterion can lead to an early stopping of information gathering. Furthermore, we demonstrate that the SDP can be used as a selection criterion. In particular, since there are many criteria for measuring the value of information, each based on optimizing different objectives, we propose a new SDP-based criterion for measuring the value of information --- this criterion values information that leads to robust decisions (i.e., ones that are unlikely to change due to new information). We develop the first algorithm to optimize the value of information, given the SDP as the reward criterion, and show empirical results that prove the utility of this novel criterion. We further answer several questions regarding the computational complexity of the SDP, which is known to be PP^PP-complete. Finally, we present results of applying the SDP as an information gathering criterion in practical problems including tutoring systems (do we need to ask more questions?) and machine learning (do we have enough data?).
The Robustness Of Model Selection Rules
DOWNLOAD
Author : Jochen A. Jungeilges
language : en
Publisher: LIT Verlag Münster
Release Date : 1992
The Robustness Of Model Selection Rules written by Jochen A. Jungeilges and has been published by LIT Verlag Münster this book supported file pdf, txt, epub, kindle and other format this book has been release on 1992 with Business & Economics categories.
Robustness
DOWNLOAD
Author : Lars Peter Hansen
language : en
Publisher: Princeton University Press
Release Date : 2016-06-28
Robustness written by Lars Peter Hansen and has been published by Princeton University Press this book supported file pdf, txt, epub, kindle and other format this book has been release on 2016-06-28 with Business & Economics categories.
The standard theory of decision making under uncertainty advises the decision maker to form a statistical model linking outcomes to decisions and then to choose the optimal distribution of outcomes. This assumes that the decision maker trusts the model completely. But what should a decision maker do if the model cannot be trusted? Lars Hansen and Thomas Sargent, two leading macroeconomists, push the field forward as they set about answering this question. They adapt robust control techniques and apply them to economics. By using this theory to let decision makers acknowledge misspecification in economic modeling, the authors develop applications to a variety of problems in dynamic macroeconomics. Technical, rigorous, and self-contained, this book will be useful for macroeconomists who seek to improve the robustness of decision-making processes.
Decision Making Under Deep Uncertainty
DOWNLOAD
Author : Vincent A. W. J. Marchau
language : en
Publisher: Springer
Release Date : 2019-04-04
Decision Making Under Deep Uncertainty written by Vincent A. W. J. Marchau and has been published by Springer this book supported file pdf, txt, epub, kindle and other format this book has been release on 2019-04-04 with Business & Economics categories.
This open access book focuses on both the theory and practice associated with the tools and approaches for decisionmaking in the face of deep uncertainty. It explores approaches and tools supporting the design of strategic plans under deep uncertainty, and their testing in the real world, including barriers and enablers for their use in practice. The book broadens traditional approaches and tools to include the analysis of actors and networks related to the problem at hand. It also shows how lessons learned in the application process can be used to improve the approaches and tools used in the design process. The book offers guidance in identifying and applying appropriate approaches and tools to design plans, as well as advice on implementing these plans in the real world. For decisionmakers and practitioners, the book includes realistic examples and practical guidelines that should help them understand what decisionmaking under deep uncertainty is and how it may be of assistance to them. Decision Making under Deep Uncertainty: From Theory to Practice is divided into four parts. Part I presents five approaches for designing strategic plans under deep uncertainty: Robust Decision Making, Dynamic Adaptive Planning, Dynamic Adaptive Policy Pathways, Info-Gap Decision Theory, and Engineering Options Analysis. Each approach is worked out in terms of its theoretical foundations, methodological steps to follow when using the approach, latest methodological insights, and challenges for improvement. In Part II, applications of each of these approaches are presented. Based on recent case studies, the practical implications of applying each approach are discussed in depth. Part III focuses on using the approaches and tools in real-world contexts, based on insights from real-world cases. Part IV contains conclusions and a synthesis of the lessons that can be drawn for designing, applying, and implementing strategic plans under deep uncertainty, as well as recommendations for future work. The publication of this book has been funded by the Radboud University, the RAND Corporation, Delft University of Technology, and Deltares.
Breakthroughs In Decision Science And Risk Analysis
DOWNLOAD
Author : Louis Anthony Cox, Jr.
language : en
Publisher: John Wiley & Sons
Release Date : 2015-02-18
Breakthroughs In Decision Science And Risk Analysis written by Louis Anthony Cox, Jr. and has been published by John Wiley & Sons this book supported file pdf, txt, epub, kindle and other format this book has been release on 2015-02-18 with Business & Economics categories.
Discover recent powerful advances in the theory, methods, and applications of decision and risk analysis Focusing on modern advances and innovations in the field of decision analysis (DA), Breakthroughs in Decision Science and Risk Analysis presents theories and methods for making, improving, and learning from significant practical decisions. The book explains these new methods and important applications in an accessible and stimulating style for readers from multiple backgrounds, including psychology, economics, statistics, engineering, risk analysis, operations research, and management science. Highlighting topics not conventionally found in DA textbooks, the book illustrates genuine advances in practical decision science, including developments and trends that depart from, or break with, the standard axiomatic DA paradigm in fundamental and useful ways. The book features methods for coping with realistic decision-making challenges such as online adaptive learning algorithms, innovations in robust decision-making, and the use of a variety of models to explain available data and recommend actions. In addition, the book illustrates how these techniques can be applied to dramatically improve risk management decisions. Breakthroughs in Decision Science and Risk Analysis also includes: An emphasis on new approaches rather than only classical and traditional ideas Discussions of how decision and risk analysis can be applied to improve high-stakes policy and management decisions Coverage of the potential value and realism of decision science within applications in financial, health, safety, environmental, business, engineering, and security risk management Innovative methods for deciding what actions to take when decision problems are not completely known or described or when useful probabilities cannot be specified Recent breakthroughs in the psychology and brain science of risky decisions, mathematical foundations and techniques, and integration with learning and pattern recognition methods from computational intelligence Breakthroughs in Decision Science and Risk Analysis is an ideal reference for researchers, consultants, and practitioners in the fields of decision science, operations research, business, management science, engineering, statistics, and mathematics. The book is also an appropriate guide for managers, analysts, and decision and policy makers in the areas of finance, health and safety, environment, business, engineering, and security risk management.
Perspective Chapter
DOWNLOAD
Author : Marc Juanpera
language : en
Publisher:
Release Date : 2019
Perspective Chapter written by Marc Juanpera and has been published by this book supported file pdf, txt, epub, kindle and other format this book has been release on 2019 with Economics categories.
Multicriteria decision-making usually requires a set of experts to evaluate the importance of selected criteria and the adequacy of feasible alternatives according to the criteria. Uncertainty can arise in these evaluations, since experts can be hesitant about their responses due to the difficulty of quantifying human language or lack of required knowledge. The Methodology for Integrated Multicriteria Decision-making with Uncertainty (MIMDU) tackles both factors of uncertainty by using non-predefined fuzzy numbers that are continuously adapted taking into account the level of confidence of the experts,Äô opinions. The methodology also offers useful and complementary information to lead to a robust decision-making. This chapter proposes a novel methodology and provides a sample use case to demonstrate its capability to model uncertainty during decision-making process. In particular, a sensitivity analysis is included, which demonstrates (i) how uncertainty is incorporated into alternatives evaluation, and (ii) that the integrated multicriteria decision-making with uncertainty can be more reliable for decision-makers. The methodology is applied to the robust selection of the most sustainable technology to improve agriculture efficiency in rural areas by means of a case study of a low-cost biogas digester in a small-scale farm in Colombia.
Dynamics Of Decision Making From Evidence To Preference And Belief
DOWNLOAD
Author : Erica Yu
language : en
Publisher: Frontiers E-books
Release Date : 2014-10-24
Dynamics Of Decision Making From Evidence To Preference And Belief written by Erica Yu and has been published by Frontiers E-books this book supported file pdf, txt, epub, kindle and other format this book has been release on 2014-10-24 with Decision making categories.
At the core of the many debates throughout cognitive science concerning how decisions are made are the processes governing the time course of preference formation and decision. From perceptual choices, such as whether the signal on a radar screen indicates an enemy missile or a spot on a CT scan indicates a tumor, to cognitive value-based decisions, such as selecting an agreeable flatmate or deciding the guilt of a defendant, significant and everyday decisions are dynamic over time. Phenomena such as decoy effects, preference reversals and order effects are still puzzling researchers. For example, in a legal context, jurors receive discrete pieces of evidence in sequence, and must integrate these pieces together to reach a singular verdict. From a standard Bayesian viewpoint the order in which people receive the evidence should not influence their final decision, and yet order effects seem a robust empirical phenomena in many decision contexts. Current research on how decisions unfold, especially in a dynamic environment, is advancing our theoretical understanding of decision making. This Research Topic aims to review and further explore the time course of a decision - from how prior beliefs are formed to how those beliefs are used and updated over time, towards the formation of preferences and choices and post-decision processes and effects. Research literatures encompassing varied approaches to the time-scale of decisions will be brought into scope: a) Speeded decisions (and post-decision processes) that require the accumulation of noisy and possibly non-stationary perceptual evidence (e.g., randomly moving dots stimuli), within a few seconds, with or without temporal uncertainty. b) Temporally-extended, value-based decisions that integrate feedback values (e.g., gambling machines) and internally-generated decision criteria (e.g., when one switches attention, selectively, between the various aspects of several choice alternatives). c) Temporally extended, belief-based decisions that build on the integration of evidence, which interacts with the decision maker's belief system, towards the updating of the beliefs and the formation of judgments and preferences (as in the legal context). Research that emphasizes theoretical concerns (including optimality analysis) and mechanisms underlying the decision process, both neural and cognitive, is presented, as well as research that combines experimental and computational levels of analysis.
Essays On Robust Model Selection And Model Averaging For Linear Models
DOWNLOAD
Author : Le Chang
language : en
Publisher:
Release Date : 2017
Essays On Robust Model Selection And Model Averaging For Linear Models written by Le Chang and has been published by this book supported file pdf, txt, epub, kindle and other format this book has been release on 2017 with categories.
Model selection is central to all applied statistical work. Selecting the variables for use in a regression model is one important example of model selection. This thesis is a collection of essays on robust model selection procedures and model averaging for linear regression models. In the first essay, we propose robust Akaike information criteria (AIC) for MM-estimation and an adjusted robust scale based AIC for M and MM-estimation. Our proposed model selection criteria can maintain their robust properties in the presence of a high proportion of outliers and the outliers in the covariates. We compare our proposed criteria with other robust model selection criteria discussed in previous literature. Our simulation studies demonstrate a significant outperformance of robust AIC based on MM-estimation in the presence of outliers in the covariates. The real data example also shows a better performance of robust AIC based on MM-estimation. The second essay focuses on robust versions of the "Least Absolute Shrinkage and Selection Operator" (lasso). The adaptive lasso is a method for performing simultaneous parameter estimation and variable selection. The adaptive weights used in its penalty term mean that the adaptive lasso achieves the oracle property. In this essay, we propose an extension of the adaptive lasso named the Tukey-lasso. By using Tukey's biweight criterion, instead of squared loss, the Tukey-lasso is resistant to outliers in both the response and covariates. Importantly, we demonstrate that the Tukey-lasso also enjoys the oracle property. A fast accelerated proximal gradient (APG) algorithm is proposed and implemented for computing the Tukey-lasso. Our extensive simulations show that the Tukey-lasso, implemented with the APG algorithm, achieves very reliable results, including for high-dimensional data where p>n. In the presence of outliers, the Tukey-lasso is shown to offer substantial improvements in performance compared to the adaptive lasso and other robust implementations of the lasso. Real data examples further demonstrate the utility of the Tukey-lasso. In many statistical analyses, a single model is used for statistical inference, ignoring the process that leads to the model being selected. To account for this model uncertainty, many model averaging procedures have been proposed. In the last essay, we propose an extension of a bootstrap model averaging approach, called bootstrap lasso averaging (BLA). BLA utilizes the lasso for model selection. This is in contrast to other forms of bootstrap model averaging that use AIC or Bayesian information criteria (BIC). The use of the lasso improves the computation speed and allows BLA to be applied even when the number of variables p is larger than the sample size n. Extensive simulations confirm that BLA has outstanding finite sample performance, in terms of both variable and prediction accuracies, compared with traditional model selection and model averaging methods. Several real data examples further demonstrate an improved out-of-sample predictive performance of BLA.