Robustness In Sample Selection Models
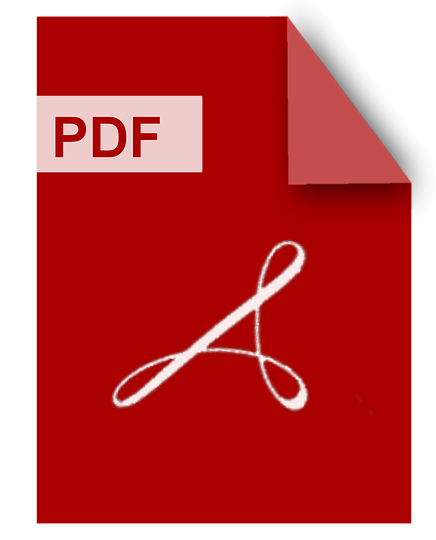
DOWNLOAD
Download Robustness In Sample Selection Models PDF/ePub or read online books in Mobi eBooks. Click Download or Read Online button to get Robustness In Sample Selection Models book now. This website allows unlimited access to, at the time of writing, more than 1.5 million titles, including hundreds of thousands of titles in various foreign languages. If the content not found or just blank you must refresh this page
Robustness In Sample Selection Models
DOWNLOAD
Author : Mikhail Zhelonkin
language : en
Publisher:
Release Date : 2013
Robustness In Sample Selection Models written by Mikhail Zhelonkin and has been published by this book supported file pdf, txt, epub, kindle and other format this book has been release on 2013 with categories.
The Robustness Of Model Selection Rules
DOWNLOAD
Author : Jochen A. Jungeilges
language : en
Publisher: LIT Verlag Münster
Release Date : 1992
The Robustness Of Model Selection Rules written by Jochen A. Jungeilges and has been published by LIT Verlag Münster this book supported file pdf, txt, epub, kindle and other format this book has been release on 1992 with Business & Economics categories.
Robustness In Econometrics
DOWNLOAD
Author : Vladik Kreinovich
language : en
Publisher: Springer
Release Date : 2017-02-11
Robustness In Econometrics written by Vladik Kreinovich and has been published by Springer this book supported file pdf, txt, epub, kindle and other format this book has been release on 2017-02-11 with Technology & Engineering categories.
This book presents recent research on robustness in econometrics. Robust data processing techniques – i.e., techniques that yield results minimally affected by outliers – and their applications to real-life economic and financial situations are the main focus of this book. The book also discusses applications of more traditional statistical techniques to econometric problems. Econometrics is a branch of economics that uses mathematical (especially statistical) methods to analyze economic systems, to forecast economic and financial dynamics, and to develop strategies for achieving desirable economic performance. In day-by-day data, we often encounter outliers that do not reflect the long-term economic trends, e.g., unexpected and abrupt fluctuations. As such, it is important to develop robust data processing techniques that can accommodate these fluctuations.
Robustness Of Statistical Methods And Nonparametric Statistics
DOWNLOAD
Author : Dieter Rasch
language : en
Publisher: Springer Science & Business Media
Release Date : 2012-12-06
Robustness Of Statistical Methods And Nonparametric Statistics written by Dieter Rasch and has been published by Springer Science & Business Media this book supported file pdf, txt, epub, kindle and other format this book has been release on 2012-12-06 with Mathematics categories.
This volume contains most of the invited and contributed papers presented at the Conference on Robustness of Statistical Methods and Nonparametric Statistics held in the castle oj'Schwerin, Mai 29 - June 4 1983. This conference was organized by the Mathematical Society of the GDR in cooperation with the Society of Physical and Mathematical Biology of the GDR, the GDR-Region of the International Biometric Society and the Academy of Agricultural Sciences of the GDR. All papers included were thoroughly reviewed by scientist listed under the heading "Editorial Collabora tories·'. Some contributions, we are sorry to report, were not recommended for publi cation by the rf'vif'wers and do not appear in these proceedings. The editors thank the reviewers for their valuable comments and suggestions. The conference was organizf'd bv a Programme Committee, its chairman was Prof. Dr. Dieter Rasch (Research Centre of Animal Production, Dummerstorf-Rostock). The members of the Programme Committee were Prof. Dr., Johannes Adam (Martin-Luther-University Halle) Prof. Dr. Heinz Ahrens (Academy of Sciences of the GDR, Berlin) Doz. Dr. Jana Jureckova (Charles University Praha) Prof. Dr. Moti Lal Tiku (McMaster University, Hamilton, Ontario) The aim of the conference was to discuss several aspects of robustness but mainly to present new results regarding the robustness of classical statistical methods especially tests, confidence estimations, and selection procedures, and to compare their perfor mance with nonparametric procedures. Robustness in this sens~ is understood as intensivity against. violation of the normal assumption.
Robustness In Confirmatory Factor Analysis
DOWNLOAD
Author : Youngkyoung Min
language : en
Publisher:
Release Date : 2008
Robustness In Confirmatory Factor Analysis written by Youngkyoung Min and has been published by this book supported file pdf, txt, epub, kindle and other format this book has been release on 2008 with categories.
When the scale was set by specifying factor loadings equal to one, there were no important effects of the factors on the factor loading, factor variance, or factor covariance estimates. Results for standard error estimates indicate that Robust ML estimates were superior to the non-robust estimates in the bias of the standard error estimates for the non-normal distributions, and the standard error estimates were underestimated for the distribution with positive kurtosis and overestimated for the distribution with negative kurtosis. From the results, it can be concluded that ML estimation method should be adopted for a normal distribution regardless of sample size, model, and scale-setting method to obtain less biased estimates of parameters and standard errors, and Robust ML should be used for nonnormal distributions to improve estimation of standard errors. However, Robust ML estimation works very well even for a normal distribution and some cases better than GLS. It was also found that robust estimation generally worked better than non-robust estimation for the nonnormal distributions regardless of the sample size and the model type. When the distribution is non-normal, Robust GLS generally performs well, although Robust ML has less bias than Robust GLS.
Robustness Theory And Application
DOWNLOAD
Author : Brenton R. Clarke
language : en
Publisher: John Wiley & Sons
Release Date : 2018-06-21
Robustness Theory And Application written by Brenton R. Clarke and has been published by John Wiley & Sons this book supported file pdf, txt, epub, kindle and other format this book has been release on 2018-06-21 with Mathematics categories.
A preeminent expert in the field explores new and exciting methodologies in the ever-growing field of robust statistics Used to develop data analytical methods, which are resistant to outlying observations in the data, while capable of detecting outliers, robust statistics is extremely useful for solving an array of common problems, such as estimating location, scale, and regression parameters. Written by an internationally recognized expert in the field of robust statistics, this book addresses a range of well-established techniques while exploring, in depth, new and exciting methodologies. Local robustness and global robustness are discussed, and problems of non-identifiability and adaptive estimation are considered. Rather than attempt an exhaustive investigation of robustness, the author provides readers with a timely review of many of the most important problems in statistical inference involving robust estimation, along with a brief look at confidence intervals for location. Throughout, the author meticulously links research in maximum likelihood estimation with the more general M-estimation methodology. Specific applications and R and some MATLAB subroutines with accompanying data sets—available both in the text and online—are employed wherever appropriate. Providing invaluable insights and guidance, Robustness Theory and Application: Offers a balanced presentation of theory and applications within each topic-specific discussion Features solved examples throughout which help clarify complex and/or difficult concepts Meticulously links research in maximum likelihood type estimation with the more general M-estimation methodology Delves into new methodologies which have been developed over the past decade without stinting on coverage of “tried-and-true” methodologies Includes R and some MATLAB subroutines with accompanying data sets, which help illustrate the power of the methods described Robustness Theory and Application is an important resource for all statisticians interested in the topic of robust statistics. This book encompasses both past and present research, making it a valuable supplemental text for graduate-level courses in robustness.
Robust Methods In Biostatistics
DOWNLOAD
Author : Stephane Heritier
language : en
Publisher: John Wiley & Sons
Release Date : 2009-05-11
Robust Methods In Biostatistics written by Stephane Heritier and has been published by John Wiley & Sons this book supported file pdf, txt, epub, kindle and other format this book has been release on 2009-05-11 with Medical categories.
Robust statistics is an extension of classical statistics that specifically takes into account the concept that the underlying models used to describe data are only approximate. Its basic philosophy is to produce statistical procedures which are stable when the data do not exactly match the postulated models as it is the case for example with outliers. Robust Methods in Biostatistics proposes robust alternatives to common methods used in statistics in general and in biostatistics in particular and illustrates their use on many biomedical datasets. The methods introduced include robust estimation, testing, model selection, model check and diagnostics. They are developed for the following general classes of models: Linear regression Generalized linear models Linear mixed models Marginal longitudinal data models Cox survival analysis model The methods are introduced both at a theoretical and applied level within the framework of each general class of models, with a particular emphasis put on practical data analysis. This book is of particular use for research students,applied statisticians and practitioners in the health field interested in more stable statistical techniques. An accompanying website provides R code for computing all of the methods described, as well as for analyzing all the datasets used in the book.
Robustness Tests For Quantitative Research
DOWNLOAD
Author : Eric Neumayer
language : en
Publisher: Cambridge University Press
Release Date : 2017-08-17
Robustness Tests For Quantitative Research written by Eric Neumayer and has been published by Cambridge University Press this book supported file pdf, txt, epub, kindle and other format this book has been release on 2017-08-17 with Business & Economics categories.
This highly accessible book presents robustness testing as the methodology for conducting quantitative analyses in the presence of model uncertainty.
Essays On Robust Model Selection And Model Averaging For Linear Models
DOWNLOAD
Author : Le Chang
language : en
Publisher:
Release Date : 2017
Essays On Robust Model Selection And Model Averaging For Linear Models written by Le Chang and has been published by this book supported file pdf, txt, epub, kindle and other format this book has been release on 2017 with categories.
Model selection is central to all applied statistical work. Selecting the variables for use in a regression model is one important example of model selection. This thesis is a collection of essays on robust model selection procedures and model averaging for linear regression models. In the first essay, we propose robust Akaike information criteria (AIC) for MM-estimation and an adjusted robust scale based AIC for M and MM-estimation. Our proposed model selection criteria can maintain their robust properties in the presence of a high proportion of outliers and the outliers in the covariates. We compare our proposed criteria with other robust model selection criteria discussed in previous literature. Our simulation studies demonstrate a significant outperformance of robust AIC based on MM-estimation in the presence of outliers in the covariates. The real data example also shows a better performance of robust AIC based on MM-estimation. The second essay focuses on robust versions of the "Least Absolute Shrinkage and Selection Operator" (lasso). The adaptive lasso is a method for performing simultaneous parameter estimation and variable selection. The adaptive weights used in its penalty term mean that the adaptive lasso achieves the oracle property. In this essay, we propose an extension of the adaptive lasso named the Tukey-lasso. By using Tukey's biweight criterion, instead of squared loss, the Tukey-lasso is resistant to outliers in both the response and covariates. Importantly, we demonstrate that the Tukey-lasso also enjoys the oracle property. A fast accelerated proximal gradient (APG) algorithm is proposed and implemented for computing the Tukey-lasso. Our extensive simulations show that the Tukey-lasso, implemented with the APG algorithm, achieves very reliable results, including for high-dimensional data where p>n. In the presence of outliers, the Tukey-lasso is shown to offer substantial improvements in performance compared to the adaptive lasso and other robust implementations of the lasso. Real data examples further demonstrate the utility of the Tukey-lasso. In many statistical analyses, a single model is used for statistical inference, ignoring the process that leads to the model being selected. To account for this model uncertainty, many model averaging procedures have been proposed. In the last essay, we propose an extension of a bootstrap model averaging approach, called bootstrap lasso averaging (BLA). BLA utilizes the lasso for model selection. This is in contrast to other forms of bootstrap model averaging that use AIC or Bayesian information criteria (BIC). The use of the lasso improves the computation speed and allows BLA to be applied even when the number of variables p is larger than the sample size n. Extensive simulations confirm that BLA has outstanding finite sample performance, in terms of both variable and prediction accuracies, compared with traditional model selection and model averaging methods. Several real data examples further demonstrate an improved out-of-sample predictive performance of BLA.
Robustness In Statistical Pattern Recognition
DOWNLOAD
Author : Y. Kharin
language : en
Publisher: Springer Science & Business Media
Release Date : 1996-09-30
Robustness In Statistical Pattern Recognition written by Y. Kharin and has been published by Springer Science & Business Media this book supported file pdf, txt, epub, kindle and other format this book has been release on 1996-09-30 with Mathematics categories.
This book is concerned with important problems of robust (stable) statistical pat tern recognition when hypothetical model assumptions about experimental data are violated (disturbed). Pattern recognition theory is the field of applied mathematics in which prin ciples and methods are constructed for classification and identification of objects, phenomena, processes, situations, and signals, i. e. , of objects that can be specified by a finite set of features, or properties characterizing the objects (Mathematical Encyclopedia (1984)). Two stages in development of the mathematical theory of pattern recognition may be observed. At the first stage, until the middle of the 1970s, pattern recogni tion theory was replenished mainly from adjacent mathematical disciplines: mathe matical statistics, functional analysis, discrete mathematics, and information theory. This development stage is characterized by successful solution of pattern recognition problems of different physical nature, but of the simplest form in the sense of used mathematical models. One of the main approaches to solve pattern recognition problems is the statisti cal approach, which uses stochastic models of feature variables. Under the statistical approach, the first stage of pattern recognition theory development is characterized by the assumption that the probability data model is known exactly or it is esti mated from a representative sample of large size with negligible estimation errors (Das Gupta, 1973, 1977), (Rey, 1978), (Vasiljev, 1983)).