Spatio Temporal Modeling For Action Recognition In Videos
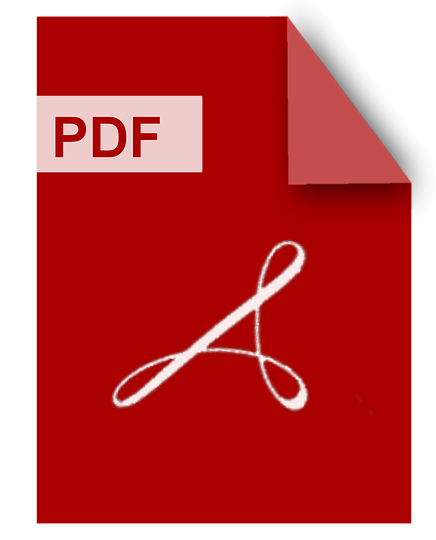
DOWNLOAD
Download Spatio Temporal Modeling For Action Recognition In Videos PDF/ePub or read online books in Mobi eBooks. Click Download or Read Online button to get Spatio Temporal Modeling For Action Recognition In Videos book now. This website allows unlimited access to, at the time of writing, more than 1.5 million titles, including hundreds of thousands of titles in various foreign languages. If the content not found or just blank you must refresh this page
Spatio Temporal Modeling For Action Recognition In Videos
DOWNLOAD
Author : Guoxi Huang
language : en
Publisher:
Release Date : 2022
Spatio Temporal Modeling For Action Recognition In Videos written by Guoxi Huang and has been published by this book supported file pdf, txt, epub, kindle and other format this book has been release on 2022 with categories.
Video Based Pattern Recognition By Spatio Temporal Modeling Via Multi Modality Co Learning
DOWNLOAD
Author : Haomian Zheng
language : en
Publisher:
Release Date : 2012
Video Based Pattern Recognition By Spatio Temporal Modeling Via Multi Modality Co Learning written by Haomian Zheng and has been published by this book supported file pdf, txt, epub, kindle and other format this book has been release on 2012 with Digital video categories.
Local Part Model For Action Recognition In Realistic Videos
DOWNLOAD
Author : Feng Shi
language : en
Publisher:
Release Date : 2014
Local Part Model For Action Recognition In Realistic Videos written by Feng Shi and has been published by this book supported file pdf, txt, epub, kindle and other format this book has been release on 2014 with University of Ottawa theses categories.
This thesis presents a framework for automatic recognition of human actions in uncontrolled, realistic video data such as movies, internet and surveillance videos. In this thesis, the human action recognition problem is solved from the perspective of local spatio-temporal feature and bag-of-features representation. The bag-of-features model only contains statistics of unordered low-level primitives, and any information concerning temporal ordering and spatial structure is lost. To address this issue, we proposed a novel multiscale local part model on the purpose of maintaining both structure information and ordering of local events for action recognition. The method includes both a coarse primitive level root feature covering event-content statistics and higher resolution overlapping part features incorporating local structure and temporal relationships. To extract the local spatio-temporal features, we investigated a random sampling strategy for efficient action recognition. We also introduced the idea of using very high sampling density for efficient and accurate classification. We further explored the potential of the method with the joint optimization of two constraints: the classification accuracy and its efficiency. On the performance side, we proposed a new local descriptor, called GBH, based on spatial and temporal gradients. It significantly improved the performance of the pure spatial gradient-based HOG descriptor on action recognition while preserving high computational efficiency. We have also shown that the performance of the state-of-the-art MBH descriptor can be improved with a discontinuity-preserving optical flow algorithm. In addition, a new method based on histogram intersection kernel was introduced to combine multiple channels of different descriptors. This method has the advantages of improving recognition accuracy with multiple descriptors and speeding up the classification process. On the efficiency side, we applied PCA to reduce the feature dimension which resulted in fast bag-of-features matching. We also evaluated the FLANN method on real-time action recognition. We conducted extensive experiments on real-world videos from challenging public action datasets. We showed that our methods achieved the state-of-the-art with real-time computational potential, thus highlighting the effectiveness and efficiency of the proposed methods.
Video Representation For Fine Grained Action Recognition
DOWNLOAD
Author : Yang Zhou
language : en
Publisher:
Release Date : 2016
Video Representation For Fine Grained Action Recognition written by Yang Zhou and has been published by this book supported file pdf, txt, epub, kindle and other format this book has been release on 2016 with High definition video recording categories.
Recently, fine-grained action analysis has raised a lot of research interests due to its potential applications in smart home, medical surveillance, daily living assist and child/elderly care, where action videos are captured indoor with fixed camera. Although background motion (i.e. one of main challenges for general action recognition) is more controlled compared to general action recognition, it is widely acknowledged that fine-grained action recognition is very challenging due to large intra-class variability, small inter-class variability, large variety of action categories, complex motions and complicated interactions. Fine-Grained actions, especially the manipulation sequences involve a large amount of interactions between hands and objects, therefore how to model the interactions between human hands and objects (i.e., context) plays an important role in action representation and recognition. We propose to discover the manipulated objects by human by modeling which objects are being manipulated and how they are being operated. Firstly, we propose a representation and classification pipeline which seamlessly incorporates localized semantic information into every processing step for fine-grained action recognition. In the feature extraction stage, we explore the geometric information between local motion features and the surrounding objects. In the feature encoding stage, we develop a semantic-grouped locality-constrained linear coding (SG-LLC) method that captures the joint distributions between motion and object-in-use information. Finally, we propose a semantic-aware multiple kernel learning framework (SA-MKL) by utilizing the empirical joint distribution between action and object type for more discriminative action classification. This approach can discover and model the inter- actions between human and objects. However, discovering the detailed knowledge of pre-detected objects (e.g. drawer and refrigerator). Thus, the performance of action recognition is constrained by object recognition, not to mention detection of objects requires tedious human labor for object annotation. Secondly, we propose a mid-level video representation to be suitable for fine-grained action classification. Given an input video sequence, we densely sample a large amount of spatio-temporal motion parts by temporal segmentation with spatial segmentation, and represent them with local motion features. The dense mid-level candidate parts are rich in localized motion information, which is crucial to fine-grained action recognition. From the candidate spatio-temporal parts, we perform an unsupervised approach to discover and learn the representative part detectors for final video representation. By utilizing the dense spatio-temporal motion parts, we highlight the human-object interactions and localized delicate motion in the local spatio-temporal sub-volume of the video. Thirdly, we propose a novel fine-grained action recognition pipeline by interaction part proposal and discriminative mid-level part mining. Firstly, we generate a large number of candidate object regions using off-the-shelf object proposal tool, e.g., BING. Secondly, these object regions are matched and tracked across frames to form a large spatio-temporal graph based on the appearance matching and the dense motion trajectories through them. We then propose an efficient approximate graph segmentation algorithm to partition and filter the graph into consistent local dense sub-graphs. These sub-graphs, which are spatio-temporal sub-volumes, represent our candidate interaction parts. Finally, we mine discriminative mid-level part detectors from the features computed over the candidate interaction parts. Bag-of-detection scores based on a novel Max-N pooling scheme are computed as the action representation for a video sample. Finally, we also focus on the first-view (egocentric) action recognition problem, which contains lots of hand-object interactions. On one hand, we propose a novel end-to-end trainable semantic parsing network for hand segmentation. On the other hand, we propose a second end-to-end deep convolutional network to maximally utilize the contextual information among hand, foreground object, and motion for interactional foreground object detection.
Scalable Action Recognition In Continuous Video Streams
DOWNLOAD
Author : Hamed Pirsiavash
language : en
Publisher:
Release Date : 2012
Scalable Action Recognition In Continuous Video Streams written by Hamed Pirsiavash and has been published by this book supported file pdf, txt, epub, kindle and other format this book has been release on 2012 with categories.
Activity recognition in video has a variety of applications, including rehabilitation, surveillance, and video retrieval. It is relatively easy for a human to recognize actions in a video once he/she watches it. However, in many applications the videos are very long, eg. in life-logging, and/or we need the real-time detection, eg. in human computer interaction. This motivates us to build computer vision and artificial intelligence algorithms to recognize activities in video sequences automatically. We are addressing several challenges in activity recognition, including (1) computational scalability, (2) spatio-temporal feature extraction, (3) spatio-temporal models, and finally, (4) dataset development. (1) Computational Scalability: We develop ``steerable'' models that parsimoniously represent a large collection of templates with a small number of parameters. This results in local detectors scalable enough for a large number of frames and object/action categories. (2) Spatio-temporal feature extraction: Spatio-temporal feature extraction is difficult for scenes with many moving objects that interact and occlude each other. We tackle this problem using the framework of multi-object tracking and developing linear-time, scalable graph-theoretic algorithms for inference. (3) Spatio-temporal models: Actions exhibit complex temporal structure, such as sub-actions of variable durations and compositional orderings. Much research on action recognition ignores such structure and instead focuses on K-way classification of temporally pre-segmented video clips \cite{poppe2010survey, DBLP:journals/csur/AggarwalR11}. We describe lightweight and efficient grammars that segment a continuous video stream into a hierarchical parse of multiple actions and sub-actions. (4) Dataset development: Finally, in terms of evaluation, video benchmarks are relatively scarce compared to the abundance of image benchmarks. It appears difficult to collect (and annotate) large-scale, unscripted footage of people doing interesting things. We discuss one solution, introducing a new, large-scale benchmark for the problem of detecting activities of daily living (ADL) in first-person camera views.
Computer Vision Accv 2010
DOWNLOAD
Author : Ron Kimmel
language : en
Publisher: Springer
Release Date : 2011-02-28
Computer Vision Accv 2010 written by Ron Kimmel and has been published by Springer this book supported file pdf, txt, epub, kindle and other format this book has been release on 2011-02-28 with Computers categories.
The four-volume set LNCS 6492-6495 constitutes the thoroughly refereed post-proceedings of the 10th Asian Conference on Computer Vision, ACCV 2009, held in Queenstown, New Zealand in November 2010. All together the four volumes present 206 revised papers selected from a total of 739 Submissions. All current issues in computer vision are addressed ranging from algorithms that attempt to automatically understand the content of images, optical methods coupled with computational techniques that enhance and improve images, and capturing and analyzing the world's geometry while preparing the higher level image and shape understanding. Novel geometry techniques, statistical learning methods, and modern algebraic procedures are dealt with as well.
Spatio Temporal Representation For Reasoning With Action Genome
DOWNLOAD
Author : Kesar Murthy
language : en
Publisher:
Release Date : 2021
Spatio Temporal Representation For Reasoning With Action Genome written by Kesar Murthy and has been published by this book supported file pdf, txt, epub, kindle and other format this book has been release on 2021 with categories.
Representing Spatio-temporal information in videos has proven to be a difficult task compared to action recognition in videos involving multiple actions. A single activity consists many smaller actions that can provide better understanding of the activity. This paper tries to represent the varying information in a scene-graph format in order to answer temporal questions to obtain improved insights for the video, resulting in a directed temporal information graph. This project will use the Action Genome dataset, which is a variation of the charades dataset, to capture pairwise relationships in a graph. The model performs significantly better than the benchmark results of the dataset providing state-of-the-art results in predicate classification. The paper presents a novel Spatio-temporal scene graph for videos, represented as a directed acyclic graph that maximises the information in the scene. The results obtained in the counting task suggest some interesting finds that are described in the paper. The graph can be used for reasoning with a much lower computational requirement explored in this work among other downstream tasks such as video captioning, action recognition and more, trying to bridge the gap between videos and textual analysis.
Learning Action Primitives For Multi Level Video Event Understanding
DOWNLOAD
Author : Erhunzi
language : en
Publisher:
Release Date : 2015
Learning Action Primitives For Multi Level Video Event Understanding written by Erhunzi and has been published by this book supported file pdf, txt, epub, kindle and other format this book has been release on 2015 with categories.
Human action categories exhibit significant intra-class variation. Changes in viewpoint, human appearance, and the temporal evolution of an action confound recognition algorithms. In order to address this, we present an approach to discover action primitives, sub-categoriesof action classes, that allow us to model this intra-class variation. We learn action primitives and their interrelations in a multi-level spatio-temporal model for action recognition. Action primitives are discovered via a data-driven clustering approach that focuses on repeatable,discriminative sub-categories. Higher-level interactions between action primitives and the actions of a set of people present in a scene are learned. Empirical results demonstrate that these action primitives can be effectively localized, and using them to model action classesimproves action recognition performance on challenging datasets.
Learning Action Primitives For Multi Level Video Event Understanding
DOWNLOAD
Author : Lei Chen
language : en
Publisher:
Release Date : 2015
Learning Action Primitives For Multi Level Video Event Understanding written by Lei Chen and has been published by this book supported file pdf, txt, epub, kindle and other format this book has been release on 2015 with categories.
Human action categories exhibit significant intra-class variation. Changes in viewpoint, human appearance, and the temporal evolution of an action confound recognition algorithms. In order to address this, we present an approach to discover action primitives, sub-categoriesof action classes, that allow us to model this intra-class variation. We learn action primitives and their interrelations in a multi-level spatio-temporal model for action recognition. Action primitives are discovered via a data-driven clustering approach that focuses on repeatable,discriminative sub-categories. Higher-level interactions between action primitives and the actions of a set of people present in a scene are learned. Empirical results demonstrate that these action primitives can be effectively localized, and using them to model action classesimproves action recognition performance on challenging datasets.
Objects For Spatio Temporal Activity Recognition In Videos
DOWNLOAD
Author : Pascal Simon Maria Mettes
language : en
Publisher:
Release Date : 2017
Objects For Spatio Temporal Activity Recognition In Videos written by Pascal Simon Maria Mettes and has been published by this book supported file pdf, txt, epub, kindle and other format this book has been release on 2017 with categories.
"This thesis investigates the role of objects for the spatio-temporal recognition of activities in videos. More specifically, we investigate what, when, and where specific activities occur in visual content by examining object representations, centered around the main question: what do objects tell about the extent of activities in visual space and time? The thesis presents six works on this topic. First, the spatial extent of activities is investigated using objects and their parts. Second, over two works, it is investigated whether activities exhibit different object preferences over time and which objects matter for representing activities. Third, the full spatio-temporal extent of activities is investigated, where over three works the extensive annotation burden of action localization is replaced respectively with point annotations, pseudo-annotations, and a zero-shot setting, where no video examples are given during training. The works lead to the conclusion that objects provide valuable information about the presence and spatio-temporal extent of activities in videos."--Samenvatting auteur.