System Identification By Bayesian Learning
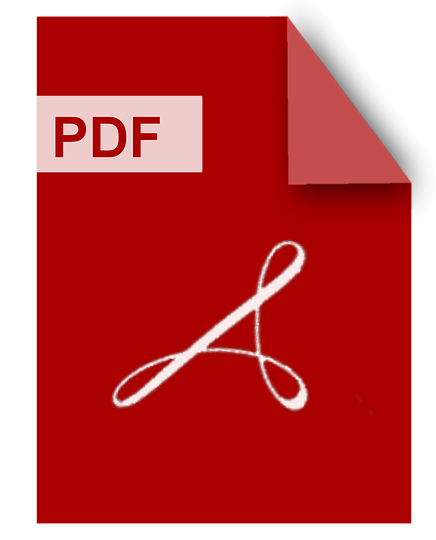
DOWNLOAD
Download System Identification By Bayesian Learning PDF/ePub or read online books in Mobi eBooks. Click Download or Read Online button to get System Identification By Bayesian Learning book now. This website allows unlimited access to, at the time of writing, more than 1.5 million titles, including hundreds of thousands of titles in various foreign languages. If the content not found or just blank you must refresh this page
System Identification By Bayesian Learning
DOWNLOAD
Author : Patrick John Donoghue
language : en
Publisher:
Release Date : 1968
System Identification By Bayesian Learning written by Patrick John Donoghue and has been published by this book supported file pdf, txt, epub, kindle and other format this book has been release on 1968 with Automatic control categories.
Bayesian Real Time System Identification
DOWNLOAD
Author : Ke Huang
language : en
Publisher: Springer Nature
Release Date : 2023-03-20
Bayesian Real Time System Identification written by Ke Huang and has been published by Springer Nature this book supported file pdf, txt, epub, kindle and other format this book has been release on 2023-03-20 with Technology & Engineering categories.
This book introduces some recent developments in Bayesian real-time system identification. It contains two different perspectives on data processing for system identification, namely centralized and distributed. A centralized Bayesian identification framework is presented to address challenging problems of real-time parameter estimation, which covers outlier detection, system, and noise parameters tracking. Besides, real-time Bayesian model class selection is introduced to tackle model misspecification problem. On the other hand, a distributed Bayesian identification framework is presented to handle asynchronous data and multiple outlier corrupted data. This book provides sufficient background to follow Bayesian methods for solving real-time system identification problems in civil and other engineering disciplines. The illustrative examples allow the readers to quickly understand the algorithms and associated applications. This book is intended for graduate students and researchers in civil and mechanical engineering. Practitioners can also find useful reference guide for solving engineering problems.
Bayesian Learning For Nonlinear System Identification
DOWNLOAD
Author : Wei Pan
language : en
Publisher:
Release Date : 2015
Bayesian Learning For Nonlinear System Identification written by Wei Pan and has been published by this book supported file pdf, txt, epub, kindle and other format this book has been release on 2015 with categories.
Regularized System Identification
DOWNLOAD
Author : Gianluigi Pillonetto
language : en
Publisher: Springer Nature
Release Date : 2022-05-13
Regularized System Identification written by Gianluigi Pillonetto and has been published by Springer Nature this book supported file pdf, txt, epub, kindle and other format this book has been release on 2022-05-13 with Computers categories.
This open access book provides a comprehensive treatment of recent developments in kernel-based identification that are of interest to anyone engaged in learning dynamic systems from data. The reader is led step by step into understanding of a novel paradigm that leverages the power of machine learning without losing sight of the system-theoretical principles of black-box identification. The authors’ reformulation of the identification problem in the light of regularization theory not only offers new insight on classical questions, but paves the way to new and powerful algorithms for a variety of linear and nonlinear problems. Regression methods such as regularization networks and support vector machines are the basis of techniques that extend the function-estimation problem to the estimation of dynamic models. Many examples, also from real-world applications, illustrate the comparative advantages of the new nonparametric approach with respect to classic parametric prediction error methods. The challenges it addresses lie at the intersection of several disciplines so Regularized System Identification will be of interest to a variety of researchers and practitioners in the areas of control systems, machine learning, statistics, and data science. This is an open access book.
Bayesian And Quadratic Methods In System Identification And Iterative Learning Control
DOWNLOAD
Author : Michael Andrew Sherback
language : en
Publisher:
Release Date : 2009
Bayesian And Quadratic Methods In System Identification And Iterative Learning Control written by Michael Andrew Sherback and has been published by this book supported file pdf, txt, epub, kindle and other format this book has been release on 2009 with categories.
An Introduction To Identification
DOWNLOAD
Author : J. P. Norton
language : en
Publisher: Courier Corporation
Release Date : 2009-01-01
An Introduction To Identification written by J. P. Norton and has been published by Courier Corporation this book supported file pdf, txt, epub, kindle and other format this book has been release on 2009-01-01 with Mathematics categories.
Suitable for advanced undergraduates and graduate students, this text covers the theoretical basis for mathematical modeling as well as a variety of identification algorithms and their applications. 1986 edition.
Trends And Progress In System Identification
DOWNLOAD
Author : Pieter Eykhoff
language : en
Publisher: Elsevier
Release Date : 2014-05-20
Trends And Progress In System Identification written by Pieter Eykhoff and has been published by Elsevier this book supported file pdf, txt, epub, kindle and other format this book has been release on 2014-05-20 with Mathematics categories.
Trends and Progress in System Identification is a three-part book that focuses on model considerations, identification methods, and experimental conditions involved in system identification. Organized into 10 chapters, this book begins with a discussion of model method in system identification, citing four examples differing on the nature of the models involved, the nature of the fields, and their goals. Subsequent chapters describe the most important aspects of model theory; the ""classical"" methods and time series estimation; application of least squares and related techniques for the estimation of dynamic system parameters; the maximum likelihood and error prediction methods; and the modern development of statistical methods. Non-parametric approaches, identification of nonlinear systems by piecewise approximation, and the minimax identification are then explained. Other chapters explore the Bayesian approach to system identification; choice of input signals; and choice and effect of different feedback configurations in system identification. This book will be useful for control engineers, system scientists, biologists, and members of other disciplines dealing withdynamical relations.
Uncertainties In Neural Networks
DOWNLOAD
Author : Magnus Malmström
language : en
Publisher: Linköping University Electronic Press
Release Date : 2021-04-06
Uncertainties In Neural Networks written by Magnus Malmström and has been published by Linköping University Electronic Press this book supported file pdf, txt, epub, kindle and other format this book has been release on 2021-04-06 with categories.
In science, technology, and engineering, creating models of the environment to predict future events has always been a key component. The models could be everything from how the friction of a tire depends on the wheels slip to how a pathogen is spread throughout society. As more data becomes available, the use of data-driven black-box models becomes more attractive. In many areas they have shown promising results, but for them to be used widespread in safety-critical applications such as autonomous driving some notion of uncertainty in the prediction is required. An example of such a black-box model is neural networks (NNs). This thesis aims to increase the usefulness of NNs by presenting an method where uncertainty in the prediction is obtained by linearization of the model. In system identification and sensor fusion, under the condition that the model structure is identifiable, this is a commonly used approach to get uncertainty in the prediction from a nonlinear model. If the model structure is not identifiable, such as for NNs, the ambiguities that cause this have to be taken care of in order to make the approach applicable. This is handled in the first part of the thesis where NNs are analyzed from a system identification perspective, and sources of uncertainty are discussed. Another problem with data-driven black-box models is that it is difficult to know how flexible the model needs to be in order to correctly model the true system. One solution to this problem is to use a model that is more flexible than necessary to make sure that the model is flexible enough. But how would that extra flexibility affect the uncertainty in the prediction? This is handled in the later part of the thesis where it is shown that the uncertainty in the prediction is bounded from below by the uncertainty in the prediction of the model with lowest flexibility required for representing true system accurately. In the literature, many other approaches to handle the uncertainty in predictions by NNs have been suggested, of which some are summarized in this work. Furthermore, a simulation and an experimental studies inspired by autonomous driving are conducted. In the simulation study, different sources of uncertainty are investigated, as well as how large the uncertainty in the predictions by NNs are in areas without training data. In the experimental study, the uncertainty in predictions done by different models are investigated. The results show that, compared to existing methods, the linearization method produces similar results for the uncertainty in predictions by NNs. An introduction video is available at https://youtu.be/O4ZcUTGXFN0 Inom forskning och utveckling har det har alltid varit centralt att skapa modeller av verkligheten. Dessa modeller har bland annat använts till att förutspå framtida händelser eller för att styra ett system till att bete sig som man önskar. Modellerna kan beskriva allt från hur friktionen hos ett bildäck påverkas av hur mycket hjulen glider till hur ett virus kan sprida sig i ett samhälle. I takt med att mer och mer data blir tillgänglig ökar potentialen för datadrivna black-box modeller. Dessa modeller är universella approximationer vilka ska kunna representera vilken godtycklig funktion som helst. Användningen av dessa modeller har haft stor framgång inom många områden men för att verkligen kunna etablera sig inom säkerhetskritiska områden såsom självkörande farkoster behövs en förståelse för osäkerhet i prediktionen från modellen. Neuronnät är ett exempel på en sådan black-box modell. I denna avhandling kommer olika sätt att tillförskaffa sig kunskap om osäkerhet i prediktionen av neuronnät undersökas. En metod som bygger på linjärisering av modellen för att tillförskaffa sig osäkerhet i prediktionen av neuronnätet kommer att presenteras. Denna metod är välbeprövad inom systemidentifiering och sensorfusion under antagandet att modellen är identifierbar. För modeller såsom neuronnät, vilka inte är identifierbara behövs det att det tas hänsyn till tvetydigheterna i modellen. En annan utmaning med datadrivna black-box modeller, är att veta om den valda modellmängden är tillräckligt generell för att kunna modellera det sanna systemet. En lösning på detta problem är att använda modeller som har mer flexibilitet än vad som behövs, det vill säga en överparameteriserad modell. Men hur påverkas osäkerheten i prediktionen av detta? Detta är något som undersöks i denna avhandling, vilken visar att osäkerheten i den överparameteriserad modellen kommer att vara begränsad underifrån av modellen med minst flexibilitet som ändå är tillräckligt generell för att modellera det sanna systemet. Som avslutning kommer dessa resultat att demonstreras i både en simuleringsstudie och en experimentstudie inspirerad av självkörande farkoster. Fokuset i simuleringsstudien är hur osäkerheten hos modellen är i områden med och utan tillgång till träningsdata medan experimentstudien fokuserar på jämförelsen mellan osäkerheten i olika typer av modeller.Resultaten från dessa studier visar att metoden som bygger på linjärisering ger liknande resultat för skattningen av osäkerheten i prediktionen av neuronnät, jämfört med existerande metoder.
System Identification Sysid 03
DOWNLOAD
Author : Paul Van Den Hof
language : en
Publisher: Elsevier
Release Date : 2004-06-29
System Identification Sysid 03 written by Paul Van Den Hof and has been published by Elsevier this book supported file pdf, txt, epub, kindle and other format this book has been release on 2004-06-29 with Science categories.
The scope of the symposium covers all major aspects of system identification, experimental modelling, signal processing and adaptive control, ranging from theoretical, methodological and scientific developments to a large variety of (engineering) application areas. It is the intention of the organizers to promote SYSID 2003 as a meeting place where scientists and engineers from several research communities can meet to discuss issues related to these areas. Relevant topics for the symposium program include: Identification of linear and multivariable systems, identification of nonlinear systems, including neural networks, identification of hybrid and distributed systems, Identification for control, experimental modelling in process control, vibration and modal analysis, model validation, monitoring and fault detection, signal processing and communication, parameter estimation and inverse modelling, statistical analysis and uncertainty bounding, adaptive control and data-based controller tuning, learning, data mining and Bayesian approaches, sequential Monte Carlo methods, including particle filtering, applications in process control systems, motion control systems, robotics, aerospace systems, bioengineering and medical systems, physical measurement systems, automotive systems, econometrics, transportation and communication systems *Provides the latest research on System Identification *Contains contributions written by experts in the field *Part of the IFAC Proceedings Series which provides a comprehensive overview of the major topics in control engineering.
Towards Bayesian System Identification
DOWNLOAD
Author : Timothy J. Rogers
language : en
Publisher:
Release Date : 2019
Towards Bayesian System Identification written by Timothy J. Rogers and has been published by this book supported file pdf, txt, epub, kindle and other format this book has been release on 2019 with categories.