Temporal Data Processing For 3d Object Detection In Autonomous Driving
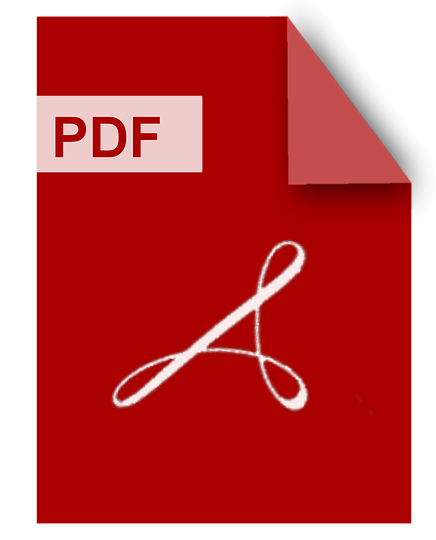
DOWNLOAD
Download Temporal Data Processing For 3d Object Detection In Autonomous Driving PDF/ePub or read online books in Mobi eBooks. Click Download or Read Online button to get Temporal Data Processing For 3d Object Detection In Autonomous Driving book now. This website allows unlimited access to, at the time of writing, more than 1.5 million titles, including hundreds of thousands of titles in various foreign languages. If the content not found or just blank you must refresh this page
Temporal Data Processing For 3d Object Detection In Autonomous Driving
DOWNLOAD
Author : Emeç Erçelik
language : en
Publisher:
Release Date : 2022
Temporal Data Processing For 3d Object Detection In Autonomous Driving written by Emeç Erçelik and has been published by this book supported file pdf, txt, epub, kindle and other format this book has been release on 2022 with categories.
Real Time 3d Object Detection For Autonomous Driving
DOWNLOAD
Author : Melissa Mozifian
language : en
Publisher:
Release Date : 2018
Real Time 3d Object Detection For Autonomous Driving written by Melissa Mozifian and has been published by this book supported file pdf, txt, epub, kindle and other format this book has been release on 2018 with Artificial intelligence categories.
This thesis focuses on advancing the state-of-the-art 3D object detection and localization in autonomous driving. An autonomous vehicle requires operating within a very unpredictable and dynamic environment. Hence a robust perception system is essential. This work proposes a novel architecture, AVOD, an \textbf{A}ggregate \textbf{V}iew \textbf{O}bject \textbf{D}etection architecture for autonomous driving capable of generating accurate 3D bounding boxes on road scenes. AVOD uses LIDAR point clouds and RGB images to generate features that are shared by two subnetworks: a region proposal network (RPN) and a second stage detector network. The proposed RPN uses a novel architecture capable of performing multimodal feature fusion on high resolution feature maps to generate reliable 3D object proposals for multiple object classes in road scenes. Using these proposals, the second stage detection network performs accurate oriented 3D bounding box regression and category classification to predict the extents, orientation, and classification of objects in 3D space. AVOD is differentiated from the state-of-the-art by using a high resolution feature extractor coupled with a multimodal fusion RPN architecture, and is therefore able to produce accurate region proposals for small classes in road scenes. AVOD also employs explicit orientation vector regression to resolve the ambiguous orientation estimate inferred from a bounding box. Experiments on the challenging KITTI dataset show the superiority of AVOD over the state-of-the-art detectors on the 3D localization, orientation estimation, and category classification tasks. Finally, AVOD is shown to run in real time and with a low memory overhead. The robustness of AVOD is also visually demonstrated when deployed on our autonomous vehicle operating under low lighting conditions such as night time as well as in snowy scenes. Furthermore, AVOD-SSD is proposed as a 3D Single Stage Detector. This work demonstrates how a single stage detector can achieve similar accuracy as that of a two-stage detector. An analysis of speed and accuracy trade-offs between AVOD and AVOD-SSD are presented.
Point Cloud Processing For Environmental Analysis In Autonomous Driving Using Deep Learning
DOWNLOAD
Author : Martin Simon
language : en
Publisher: BoD – Books on Demand
Release Date : 2023-01-01
Point Cloud Processing For Environmental Analysis In Autonomous Driving Using Deep Learning written by Martin Simon and has been published by BoD – Books on Demand this book supported file pdf, txt, epub, kindle and other format this book has been release on 2023-01-01 with Computers categories.
Autonomous self-driving cars need a very precise perception system of their environment, working for every conceivable scenario. Therefore, different kinds of sensor types, such as lidar scanners, are in use. This thesis contributes highly efficient algorithms for 3D object recognition to the scientific community. It provides a Deep Neural Network with specific layers and a novel loss to safely localize and estimate the orientation of objects from point clouds originating from lidar sensors. First, a single-shot 3D object detector is developed that outputs dense predictions in only one forward pass. Next, this detector is refined by fusing complementary semantic features from cameras and joint probabilistic tracking to stabilize predictions and filter outliers. The last part presents an evaluation of data from automotive-grade lidar scanners. A Generative Adversarial Network is also being developed as an alternative for target-specific artificial data generation.
Autonomous Driving Algorithms And Its Ic Design
DOWNLOAD
Author : Jianfeng Ren
language : en
Publisher: Springer Nature
Release Date : 2023-08-09
Autonomous Driving Algorithms And Its Ic Design written by Jianfeng Ren and has been published by Springer Nature this book supported file pdf, txt, epub, kindle and other format this book has been release on 2023-08-09 with Technology & Engineering categories.
With the rapid development of artificial intelligence and the emergence of various new sensors, autonomous driving has grown in popularity in recent years. The implementation of autonomous driving requires new sources of sensory data, such as cameras, radars, and lidars, and the algorithm processing requires a high degree of parallel computing. In this regard, traditional CPUs have insufficient computing power, while DSPs are good at image processing but lack sufficient performance for deep learning. Although GPUs are good at training, they are too “power-hungry,” which can affect vehicle performance. Therefore, this book looks to the future, arguing that custom ASICs are bound to become mainstream. With the goal of ICs design for autonomous driving, this book discusses the theory and engineering practice of designing future-oriented autonomous driving SoC chips. The content is divided into thirteen chapters, the first chapter mainly introduces readers to the current challenges and research directions in autonomous driving. Chapters 2–6 focus on algorithm design for perception and planning control. Chapters 7–10 address the optimization of deep learning models and the design of deep learning chips, while Chapters 11-12 cover automatic driving software architecture design. Chapter 13 discusses the 5G application on autonomous drving. This book is suitable for all undergraduates, graduate students, and engineering technicians who are interested in autonomous driving.
Towards Real Time 3d Vehicle Detection From Monocular Images Using Deep Learning
DOWNLOAD
Author : Nils Gählert
language : de
Publisher:
Release Date : 2021*
Towards Real Time 3d Vehicle Detection From Monocular Images Using Deep Learning written by Nils Gählert and has been published by this book supported file pdf, txt, epub, kindle and other format this book has been release on 2021* with categories.
One key task of the environment perception pipeline for autonomous driving is object detection using monocular RGB images. This task is usually limited to 2D object detection. The question arises whether 3D object detection is also possible using only monocular RGB images. In this dissertation, we evaluate this question specifically for 3D vehicle detection in monocular RGB images in the scope of driver assistance systems and autonomous driving. We use modern deep learning techniques without utilizing temporal information and a so-called 2D-3D lifting. In particular, this includes the estimation of 3D location, orientation, and the size of the object. In addition to a reliable and high-quality detection performance, autonomous driving systems require a short runtime. Therefore, we opt for the best possible trade-off between detection performance and runtime. Since the basis of any deep learning approach is high-quality data, we introduce a new dataset, Cityscapes 3D. This dataset is characterized in particular by its annotations with 9 degrees of freedom, as well as novel and improved evaluation metrics. We published a publicly available benchmark that allows research groups to assess and compare their methods for 3D object detection to those of other researchers. We develop improvements for 2D object detection and prove their effectiveness. Firstly, we increase the 2D detection performance by more than 5% using an adapted error function during training. Secondly, we develop vg-NMS that particularly supports 2D amodal object detection. With MB-Net, BS3D, and 3D-GCK, we develop three different approaches based on the 2D-3D lifting scheme. All developed approaches stand out for their comparably good detection performances and their short runtime. In direct comparison to MB-Net and BS3D, 3D-GCK does not require any post-processing. It estimates all 9 degrees of freedom of a vehicle in 3D space and also requires no prior knowledge about possible vehicle extents.
Multimodal Spatio Temporal Deep Learning Framework For 3d Object Detection In Instrumented Vehicles
DOWNLOAD
Author : Venkatesh Gurram Munirathnam
language : en
Publisher:
Release Date : 2023
Multimodal Spatio Temporal Deep Learning Framework For 3d Object Detection In Instrumented Vehicles written by Venkatesh Gurram Munirathnam and has been published by this book supported file pdf, txt, epub, kindle and other format this book has been release on 2023 with categories.
This thesis presents the utilization of multiple modalities, such as image and lidar, to incorporate spatio-temporal information from sequence data into deep learning architectures for 3Dobject detection in instrumented vehicles. The race to autonomy in instrumented vehicles or self-driving cars has stimulated significant research in developing autonomous driver assistance systems (ADAS) technologies related explicitly to perception systems. Object detection plays a crucial role in perception systems by providing spatial information to its subsequent modules; hence, accurate detection is a significant task supporting autonomous driving. The advent of deep learning in computer vision applications and the availability of multiple sensing modalities such as 360° imaging, lidar, and radar have led to state-of-the-art 2D and 3Dobject detection architectures. Most current state-of-the-art 3D object detection frameworks consider single-frame reference. However, these methods do not utilize temporal information associated with the objects or scenes from the sequence data. Thus, the present research hypothesizes that multimodal temporal information can contribute to bridging the gap between 2D and 3D metric space by improving the accuracy of deep learning frameworks for 3D object estimations. The thesis presents understanding multimodal data representations and selecting hyper-parameters using public datasets such as KITTI and nuScenes with Frustum-ConvNet as a baseline architecture. Secondly, an attention mechanism was employed along with convolutional-LSTM to extract spatial-temporal information from sequence data to improve 3D estimations and to aid the architecture in focusing on salient lidar point cloud features. Finally, various fusion strategies are applied to fuse the modalities and temporal information into the architecture to assess its efficacy on performance and computational complexity. Overall, this thesis has established the importance and utility of multimodal systems for refined 3D object detection and proposed a complex pipeline incorporating spatial, temporal and attention mechanisms to improve specific, and general class accuracy demonstrated on key autonomous driving data sets.
Proceedings Of The 2021 Joint Workshop Of Fraunhofer Iosb And Institute For Anthropomatics Vision And Fusion Laboratory
DOWNLOAD
Author : Beyerer, Jürgen
language : en
Publisher: KIT Scientific Publishing
Release Date : 2022-07-05
Proceedings Of The 2021 Joint Workshop Of Fraunhofer Iosb And Institute For Anthropomatics Vision And Fusion Laboratory written by Beyerer, Jürgen and has been published by KIT Scientific Publishing this book supported file pdf, txt, epub, kindle and other format this book has been release on 2022-07-05 with Computers categories.
2021, the annual joint workshop of the Fraunhofer IOSB and KIT IES was hosted at the IOSB in Karlsruhe. For a week from the 2nd to the 6th July the doctoral students extensive reports on the status of their research. The results and ideas presented at the workshop are collected in this book in the form of detailed technical reports.
3d Object Detection And Tracking For Autonomous Vehicles
DOWNLOAD
Author : Su Pang
language : en
Publisher:
Release Date : 2022
3d Object Detection And Tracking For Autonomous Vehicles written by Su Pang and has been published by this book supported file pdf, txt, epub, kindle and other format this book has been release on 2022 with Electronic dissertations categories.
Autonomous driving systems require accurate 3D object detection and tracking to achieve reliable path planning and navigation. For object detection, there have been significant advances in neural networks for single-modality approaches. However, it has been surprisingly difficult to train networks to use multiple modalities in a way that demonstrates gain over single-modality networks. In this dissertation, we first propose three networks for Camera-LiDAR and Camera-Radar fusion. For Camera-LiDAR fusion, CLOCs (Camera-LiDAR Object Candidates fusion) and Fast-CLOCs are presented. CLOCs fusion provides a multi-modal fusion framework that significantly improves the performance of single-modality detectors. CLOCs operates on the combined output candidates before Non-Maximum Suppression (NMS) of any 2D and any 3D detector, and is trained to leverage their geometric and semantic consistencies to produce more accurate 3D detection results. Fast-CLOCs can run in near real-time with less computational requirements compared to CLOCs. Fast-CLOCs eliminates the separate heavy 2D detector, and instead uses a 3D detector-cued 2D image detector (3D-Q-2D) to reduce memory and computation. For Camera-Radar fusion, we propose TransCAR, a Transformer-based Camera-And-Radar fusion solution for 3D object detection. The cross-attention layer within the transformer decoder can adaptively learn the soft-association between the radar features and vision queries instead of hard-association based on sensor calibration only. Then, we propose to solve the 3D multiple object tracking (MOT) problem for autonomous driving applications using a random finite set-based (RFS) Multiple Measurement Models filter (RFS-M3). In particular, we propose multiple measurement models for a Poisson multi-Bernoulli mixture (PMBM) filter in support of different application scenarios. Our RFS-M3 filter can naturally model these uncertainties accurately and elegantly. We combine learning-based detections with our RFS-M3 tracker by incorporating the detection confidence score into the PMBM prediction and update step. We have evaluated our CLOCs, Fast-CLOCs and TransCAR fusion-based 3D detector and RFS-M3 3D tracker using challenging datasets including KITTI, nuScenes, Argoverse and Waymo that are released by academia and industry leaders. Superior experimental results demonstrated the effectiveness of the proposed approaches.
Computer Vision Eccv 2020 Workshops
DOWNLOAD
Author : Adrien Bartoli
language : en
Publisher: Springer Nature
Release Date : 2021-01-02
Computer Vision Eccv 2020 Workshops written by Adrien Bartoli and has been published by Springer Nature this book supported file pdf, txt, epub, kindle and other format this book has been release on 2021-01-02 with Computers categories.
The 6-volume set, comprising the LNCS books 12535 until 12540, constitutes the refereed proceedings of 28 out of the 45 workshops held at the 16th European Conference on Computer Vision, ECCV 2020. The conference was planned to take place in Glasgow, UK, during August 23-28, 2020, but changed to a virtual format due to the COVID-19 pandemic. The 249 full papers, 18 short papers, and 21 further contributions included in the workshop proceedings were carefully reviewed and selected from a total of 467 submissions. The papers deal with diverse computer vision topics. Part II focusses on commands for autonomous vehicles; computer vision for ART analysis; sign language recognition, translation and production; visual inductive priors for data-efficient deep learning; 3D poses in the wild challenge; map-based localization for autonomous driving; recovering 6D object pose; and shape recovery from partial textured 3D scans.
Rgb D Image Analysis And Processing
DOWNLOAD
Author : Paul L. Rosin
language : en
Publisher: Springer Nature
Release Date : 2019-10-26
Rgb D Image Analysis And Processing written by Paul L. Rosin and has been published by Springer Nature this book supported file pdf, txt, epub, kindle and other format this book has been release on 2019-10-26 with Computers categories.
This book focuses on the fundamentals and recent advances in RGB-D imaging as well as covering a range of RGB-D applications. The topics covered include: data acquisition, data quality assessment, filling holes, 3D reconstruction, SLAM, multiple depth camera systems, segmentation, object detection, salience detection, pose estimation, geometric modelling, fall detection, autonomous driving, motor rehabilitation therapy, people counting and cognitive service robots. The availability of cheap RGB-D sensors has led to an explosion over the last five years in the capture and application of colour plus depth data. The addition of depth data to regular RGB images vastly increases the range of applications, and has resulted in a demand for robust and real-time processing of RGB-D data. There remain many technical challenges, and RGB-D image processing is an ongoing research area. This book covers the full state of the art, and consists of a series of chapters by internationally renowned experts in the field. Each chapter is written so as to provide a detailed overview of that topic. RGB-D Image Analysis and Processing will enable both students and professional developers alike to quickly get up to speed with contemporary techniques, and apply RGB-D imaging in their own projects.