Time Series Econometrics Conditional Variance Models
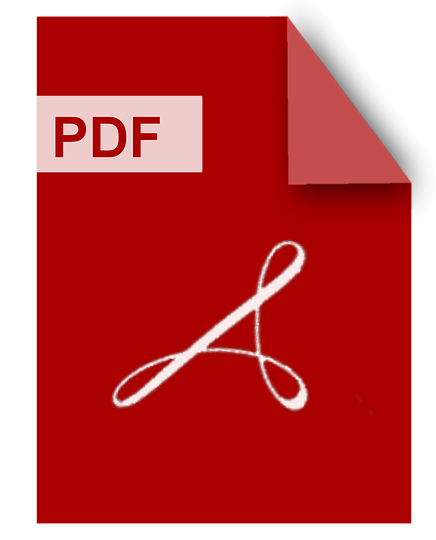
DOWNLOAD
Download Time Series Econometrics Conditional Variance Models PDF/ePub or read online books in Mobi eBooks. Click Download or Read Online button to get Time Series Econometrics Conditional Variance Models book now. This website allows unlimited access to, at the time of writing, more than 1.5 million titles, including hundreds of thousands of titles in various foreign languages. If the content not found or just blank you must refresh this page
Time Series Econometrics Conditional Variance Models
DOWNLOAD
Author : R. Prost
language : en
Publisher: Lulu.com
Release Date : 2020-09-20
Time Series Econometrics Conditional Variance Models written by R. Prost and has been published by Lulu.com this book supported file pdf, txt, epub, kindle and other format this book has been release on 2020-09-20 with Computers categories.
Conditional variance models are appropriate for time series that do not exhibit significant autocorrelation, but are serially dependent. For modeling time series that are both autocorrelated and serially dependent, you can consider using a composite conditional mean and variance model. Two characteristics of financial time series that conditional variance models address are: Volatility clustering and Leverage effects. Volatility is the conditional standard deviation of a time series. Autocorrelation in the conditional variance process results in volatility clustering. The GARCH model and its variants model autoregression in the variance series. Leverage effects. The volatility of some time series responds more to large decreases than to large increases. This asymmetric clustering behavior is known as the leverage effect. The EGARCH and GJR models have leverage terms to model this asymmetry. In this book a variety of examples are presented, all of them treated with MATLAB.
Econometric Modeling With Matlab Conditional Variance Time Series Models
DOWNLOAD
Author : B. Noriega
language : en
Publisher: Independently Published
Release Date : 2019-03-03
Econometric Modeling With Matlab Conditional Variance Time Series Models written by B. Noriega and has been published by Independently Published this book supported file pdf, txt, epub, kindle and other format this book has been release on 2019-03-03 with Mathematics categories.
Conditional variance models are appropriate for time series that do not exhibit significant autocorrelation, but are serially dependent. For modeling time series that are both autocorrelated and serially dependent, you can consider using a composite conditional mean and variance model.Two characteristics of financial time series that conditional variance models address are: -Volatility clustering. Volatility is the conditional standard deviation of a time series. Autocorrelation in the conditional variance process results in volatility clustering. The GARCH model and its variants model autoregression in the variance series.-Leverage effects. The volatility of some time series responds more to large decreases than to large increases. This asymmetric clustering behavior is known as the leverage effect. The EGARCH and GJR models have leverage terms to model this asymmetry.The generalized autoregressive conditional heteroscedastic (GARCH) model is an extension of Engle's ARCH model for variance heteroscedasticity. If a series exhibits volatility clustering, this suggests that past variances might be predictive of the current variance. The GARCH(P, Q) model is an autoregressive moving average model for conditional variances, with P GARCH coefficients associated with lagged variances, and Q ARCH coefficients associated with lagged squared innovations.The exponential GARCH (EGARCH) model is a GARCH variant that models the logarithm of the conditional variance process. In addition to modeling the logarithm, the EGARCH model has additional leverage terms to capture asymmetry in volatility clustering. The EGARCH(P, Q) model has P GARCH coefficients associated with lagged log variance terms, Q ARCH coefficients associated with the magnitude of lagged standardized innovations, and Q leverage coefficients associated with signed, lagged standardized innovations.
Time Series Analysis With Matlab
DOWNLOAD
Author : Perez M.
language : en
Publisher: Createspace Independent Publishing Platform
Release Date : 2016-06-23
Time Series Analysis With Matlab written by Perez M. and has been published by Createspace Independent Publishing Platform this book supported file pdf, txt, epub, kindle and other format this book has been release on 2016-06-23 with categories.
Econometrics Toolbox(TM) provides functions for modeling economic data. You can select and calibrate economic models for simulation and forecasting. For time series modeling and analysis, the toolbox includes univariate ARMAX/GARCH composite models with several GARCH variants, multivariate VARMAX models, and cointegration analysis. This book focuses on conditional variance models. Conditional variance models attempt to address volatility clustering in univariate time series models to improve parameter estimates and forecast accuracy. To model volatility, Econometrics Toolbox(TM) supports the standard generalized autoregressive conditional heteroscedastic (ARCH/GARCH) model, the exponential GARCH (EGARCH) model, and the Glosten, Jagannathan, and Runkle (GJR) model.
Introduction To Modern Time Series Analysis
DOWNLOAD
Author : Gebhard Kirchgässner
language : en
Publisher: Springer Science & Business Media
Release Date : 2012-10-09
Introduction To Modern Time Series Analysis written by Gebhard Kirchgässner and has been published by Springer Science & Business Media this book supported file pdf, txt, epub, kindle and other format this book has been release on 2012-10-09 with Business & Economics categories.
This book presents modern developments in time series econometrics that are applied to macroeconomic and financial time series, bridging the gap between methods and realistic applications. It presents the most important approaches to the analysis of time series, which may be stationary or nonstationary. Modelling and forecasting univariate time series is the starting point. For multiple stationary time series, Granger causality tests and vector autogressive models are presented. As the modelling of nonstationary uni- or multivariate time series is most important for real applied work, unit root and cointegration analysis as well as vector error correction models are a central topic. Tools for analysing nonstationary data are then transferred to the panel framework. Modelling the (multivariate) volatility of financial time series with autogressive conditional heteroskedastic models is also treated.
Econometric With Matlab
DOWNLOAD
Author : A. Smith
language : en
Publisher:
Release Date : 2017-11-10
Econometric With Matlab written by A. Smith and has been published by this book supported file pdf, txt, epub, kindle and other format this book has been release on 2017-11-10 with categories.
Econometrics Toolbox provides functions for modeling economic data. You can select and estimate economic models for simulation and forecasting. For time series modeling and analysis, the toolbox includes univariate Bayesian linear regression, univariate ARIMAX/GARCH composite models with several GARCH variants, multivariate VARX models, and cointegration analysis. It also provides methods for modeling economic systems using state-space models and for estimating using the Kalman filter. You can use a variety of diagnostics for model selection, including hypothesis tests, unit root,stationarity, and structural change.Two characteristics of financial time series that conditional variance models address are:* Volatility clustering. Volatility is the conditional standard deviation of a time series. Autocorrelation in the conditional variance process results in volatility clustering. The GARCH model and its variants model autoregression in the variance series.* Leverage effects. The volatility of some time series responds more to large decreases than to large increases. This asymmetric clustering behavior is known as the leverage effect. The EGARCH and GJR models have leverage terms to model this asymmetry.The generalized autoregressive conditional heteroscedastic (GARCH) model is an extension of Engle's ARCH model for variance heteroscedasticity. If a series exhibits volatility clustering, this suggests that past variances might be predictive of the current variance. The GARCH(P,Q) model is an autoregressive moving average model for conditional variances, with P GARCH coefficients associated with lagged variances, and Q ARCH coefficients associated with lagged squared innovations.The exponential GARCH (EGARCH) model is a GARCH variant that models the logarithm of the conditional variance process. In addition to modeling the logarithm, the EGARCH model has additional leverage terms to capture asymmetry in volatility clustering. The EGARCH(P,Q) model has P GARCH coefficients associated with lagged log variance terms, Q ARCH coefficients associated with the magnitude of lagged standardized innovations, and Q leverage coefficients associated with signed, lagged standardized innovations.The GJR model is a GARCH variant that includes leverage terms for modeling asymmetric volatility clustering. In the GJR formulation, large negative changes are more likely to be clustered than positive changes. The GJR model is named for Glosten, Jagannathan, and Runkle. Close similarities exist between the GJR model and the threshold GARCH (TGARCH) model-a GJR model is a recursive equation for the variance process, and a TGARCH is the same recursion applied to the standard deviation process. The GJR(P,Q) model has P GARCH coefficients associated with lagged variances, Q ARCH coefficients associated with lagged squared innovations, and Q leverage coefficients associated with the square of negative lagged innovations.This book develops ARCH, GARCH, EGARCH, TGARCH and GJR time series models.
Time Series Analysis With Matlab
DOWNLOAD
Author : Mara Prez
language : en
Publisher: CreateSpace
Release Date : 2014-09-12
Time Series Analysis With Matlab written by Mara Prez and has been published by CreateSpace this book supported file pdf, txt, epub, kindle and other format this book has been release on 2014-09-12 with Mathematics categories.
MATLAB Econometrics Toolbox provides functions for modeling economic data You can select and calibrate economic models for simulation and forecasting Time series capabilities include univariate ARMAX/GARCH composite models with several GARCH variants, multivariate VARMAX models, and cointegration analysis The toolbox provides Monte Carlo methods for simulating systems of linear and nonlinear stochastic differential equations and a variety of diagnostics for model selection, including hypothesis, unit root, and stationarity tests.This book develops, among others, the following topics Conditional Variance Models GARCH Model Specify GARCH Models Using garch GARCH Model Specifications GARCH Model with a Mean Offset GARCH Model with Nonconsecutive Lags GARCH Model with Known Parameter Values GARCH Model with a t Innovation Distributio EGARCH Model Specify EGARCH Models Using egarch EGARCH Model Specifications EGARCH Model with a Mean Offset EGARCH Model with Nonconsecutive Lags EGARCH Model with Known Parameter Values EGARCH Model with a t Innovation Distribution GJR Model Specify GJR Models Using gjr GJR Model Specifications GJR Model with a Mean Offset GJR Model with Nonconsecutive Lags GJR Model with Known Parameter Values GJR Model with a t Innovation Distribution Modify Properties of Conditional Variance Model Objects Specify the Conditional Variance Model Innovation Distribution Specify a Conditional Variance Model Maximum Likelihood Estimation for Conditional Variance Models Innovation Distribution Loglikelihood Functions Conditional Variance Model Estimation with Equality Constraints Presample Data for Conditional Variance Model EstimationInitial Values for Conditional Variance Model Estimation Optimization Settings for Conditional Variance Model Estimation Conditional Variance Model Constraints Infer Conditional Variances and Residuals Likelihood Ratio Test for Conditional Variance Models Compare Conditional Variance Models Using Information Criteria Monte Carlo Simulation of Conditional Variance Models Presample Data for Conditional Variance Model Simulation Simulate GARCH Models Assess the EGARCH Forecast Bias Using Simulations Simulate Conditional Variance Model Monte Carlo Forecasting of Conditional Variance Models MMSE Forecasting of Conditional Variance Models EGARCH MMSE Forecasts Forecast GJR Models Forecast Conditional Variance Model Including an Exogenous Regression Component ARMAX Model Specifying ARMAX Models Using garchset Maximum Likelihood Estimation Initial Parameter Values for Optimization GARCHFIT Examples Estimation Presample Data GARCHSIM Examples Simulation Presample Data MMSE Forecasting GARCHPRED Examples
Non Linear Time Series Models In Empirical Finance
DOWNLOAD
Author : Philip Hans Franses
language : en
Publisher: Cambridge University Press
Release Date : 2000-07-27
Non Linear Time Series Models In Empirical Finance written by Philip Hans Franses and has been published by Cambridge University Press this book supported file pdf, txt, epub, kindle and other format this book has been release on 2000-07-27 with Business & Economics categories.
This 2000 volume reviews non-linear time series models, and their applications to financial markets.
Modeling Financial Time Series With S Plus
DOWNLOAD
Author : Eric Zivot
language : en
Publisher: Springer Science & Business Media
Release Date : 2013-11-11
Modeling Financial Time Series With S Plus written by Eric Zivot and has been published by Springer Science & Business Media this book supported file pdf, txt, epub, kindle and other format this book has been release on 2013-11-11 with Business & Economics categories.
The field of financial econometrics has exploded over the last decade This book represents an integration of theory, methods, and examples using the S-PLUS statistical modeling language and the S+FinMetrics module to facilitate the practice of financial econometrics. This is the first book to show the power of S-PLUS for the analysis of time series data. It is written for researchers and practitioners in the finance industry, academic researchers in economics and finance, and advanced MBA and graduate students in economics and finance. Readers are assumed to have a basic knowledge of S-PLUS and a solid grounding in basic statistics and time series concepts. This Second Edition is updated to cover S+FinMetrics 2.0 and includes new chapters on copulas, nonlinear regime switching models, continuous-time financial models, generalized method of moments, semi-nonparametric conditional density models, and the efficient method of moments. Eric Zivot is an associate professor and Gary Waterman Distinguished Scholar in the Economics Department, and adjunct associate professor of finance in the Business School at the University of Washington. He regularly teaches courses on econometric theory, financial econometrics and time series econometrics, and is the recipient of the Henry T. Buechel Award for Outstanding Teaching. He is an associate editor of Studies in Nonlinear Dynamics and Econometrics. He has published papers in the leading econometrics journals, including Econometrica, Econometric Theory, the Journal of Business and Economic Statistics, Journal of Econometrics, and the Review of Economics and Statistics. Jiahui Wang is an employee of Ronin Capital LLC. He received a Ph.D. in Economics from the University of Washington in 1997. He has published in leading econometrics journals such as Econometrica and Journal of Business and Economic Statistics, and is the Principal Investigator of National Science Foundation SBIR grants. In 2002 Dr. Wang was selected as one of the "2000 Outstanding Scholars of the 21st Century" by International Biographical Centre.
Modelling Financial Time Series
DOWNLOAD
Author : Stephen J. Taylor
language : en
Publisher: World Scientific
Release Date : 2008
Modelling Financial Time Series written by Stephen J. Taylor and has been published by World Scientific this book supported file pdf, txt, epub, kindle and other format this book has been release on 2008 with Business & Economics categories.
This book contains several innovative models for the prices of financial assets. First published in 1986, it is a classic text in the area of financial econometrics. It presents ARCH and stochastic volatility models that are often used and cited in academic research and are applied by quantitative analysts in many banks. Another often-cited contribution of the first edition is the documentation of statistical characteristics of financial returns, which are referred to as stylized facts.This second edition takes into account the remarkable progress made by empirical researchers during the past two decades from 1986 to 2006. In the new Preface, the author summarizes this progress in two key areas: firstly, measuring, modelling and forecasting volatility; and secondly, detecting and exploiting price trends.
Time Series Techniques For Economists
DOWNLOAD
Author : Terence C. Mills
language : en
Publisher: Cambridge University Press
Release Date : 1990
Time Series Techniques For Economists written by Terence C. Mills and has been published by Cambridge University Press this book supported file pdf, txt, epub, kindle and other format this book has been release on 1990 with Business & Economics categories.
The application of time series techniques in economics has become increasingly important, both for forecasting purposes and in the empirical analysis of time series in general. In this book, Terence Mills not only brings together recent research at the frontiers of the subject, but also analyses the areas of most importance to applied economics. It is an up-to-date text which extends the basic techniques of analysis to cover the development of methods that can be used to analyse a wide range of economic problems. The book analyses three basic areas of time series analysis: univariate models, multivariate models, and non-linear models. In each case the basic theory is outlined and then extended to cover recent developments. Particular emphasis is placed on applications of the theory to important areas of applied economics and on the computer software and programs needed to implement the techniques. This book clearly distinguishes itself from its competitors by emphasising the techniques of time series modelling rather than technical aspects such as estimation, and by the breadth of the models considered. It features many detailed real-world examples using a wide range of actual time series. It will be useful to econometricians and specialists in forecasting and finance and accessible to most practitioners in economics and the allied professions.