Unsupervised Learning For Residential Energy Consumption Analytics
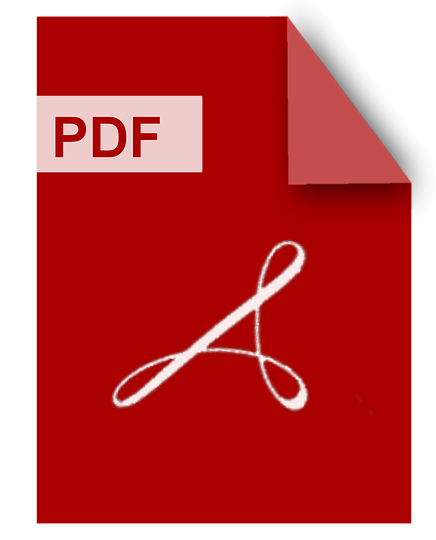
DOWNLOAD
Download Unsupervised Learning For Residential Energy Consumption Analytics PDF/ePub or read online books in Mobi eBooks. Click Download or Read Online button to get Unsupervised Learning For Residential Energy Consumption Analytics book now. This website allows unlimited access to, at the time of writing, more than 1.5 million titles, including hundreds of thousands of titles in various foreign languages. If the content not found or just blank you must refresh this page
Unsupervised Learning For Residential Energy Consumption Analytics
DOWNLOAD
Author : Thanchanok Teeraratkul
language : en
Publisher:
Release Date : 2018
Unsupervised Learning For Residential Energy Consumption Analytics written by Thanchanok Teeraratkul and has been published by this book supported file pdf, txt, epub, kindle and other format this book has been release on 2018 with categories.
Developing strategies to encourage reduction in households energy consumption requires utilities to categorize, predict and modify consumers electricity usage. Unfortunately, a typical consumer exhibits wide variation in daily 24-hour electricity usage patterns. Traditional clustering methods have resulted in many hundreds of clusters, with a given consumer often associated with several clusters, making it difficult to classify consumers into stable representative groups and to predict individual electricity usage patterns. This dissertation presents two methods that better cluster consumer electricity usage pattern. The first method uses Dynamic time warping (DTW), which seeks an optimal alignment between electricity usage patterns. The second method assumes that electricity usage composes of a sequence of blocks generated from electrical devices. The clustering uses a novel block factorization model that embeds consumer usage pattern into a low dimensional space that flexibly captures time shifts of usage. Compared to commonly used clustering algorithm, both methods result in a more distinct set of clusters, and on average, a given consumer associates with a fewer clusters. The ideas and results from clustering is then used for individual electricity usage prediction. Prediction is done at two levels. The first level is day-to-day prediction where the shape of next day electricity usage pattern is predicted by cluster representatives from DTW. The second level is finer grain prediction based on block idea where load curve is predicted through block sequences. Predictions at both levels result in lower prediction error compared to some popular load forecasting techniques.
Predictive Analytics For Energy Efficiency And Energy Retailing
DOWNLOAD
Author : Konstantin Hopf
language : en
Publisher: University of Bamberg Press
Release Date : 2019-07-15
Predictive Analytics For Energy Efficiency And Energy Retailing written by Konstantin Hopf and has been published by University of Bamberg Press this book supported file pdf, txt, epub, kindle and other format this book has been release on 2019-07-15 with Computers categories.
Data Analytics For Renewable Energy Integration Technologies Systems And Society
DOWNLOAD
Author : Wei Lee Woon
language : en
Publisher: Springer
Release Date : 2018-11-16
Data Analytics For Renewable Energy Integration Technologies Systems And Society written by Wei Lee Woon and has been published by Springer this book supported file pdf, txt, epub, kindle and other format this book has been release on 2018-11-16 with Computers categories.
This book constitutes the revised selected papers from the 6th ECML PKDD Workshop on Data Analytics for Renewable Energy Integration, DARE 2018, held in Dublin, Ireland, in September 2018. The 9 papers presented in this volume were carefully reviewed and selected for inclusion in this book and handle topics such as time series forecasting, the detection of faults, cyber security, smart grid and smart cities, technology integration, demand response, and many others.
Towards Energy Smart Homes
DOWNLOAD
Author : Stephane Ploix
language : en
Publisher: Springer Nature
Release Date : 2021-11-11
Towards Energy Smart Homes written by Stephane Ploix and has been published by Springer Nature this book supported file pdf, txt, epub, kindle and other format this book has been release on 2021-11-11 with Technology & Engineering categories.
This book exemplifies how smart buildings have a crucial role to play for the future of energy. The book investigates what already exists in regards to technologies, approaches and solutions both with a scientific and technological point of view. The authors cover solutions for mirroring and tracing human activities, optimal strategies to configure home settings, and generating explanations and persuasive dashboards to get occupants better committed in their home energy managements. Solutions are adapted from the fields of Internet of Things, physical modeling, optimization, machine learning and applied artificial intelligence. Practical applications are given throughout.
Data Driven Analytics For Sustainable Buildings And Cities
DOWNLOAD
Author : Xingxing Zhang
language : en
Publisher: Springer Nature
Release Date : 2021-09-11
Data Driven Analytics For Sustainable Buildings And Cities written by Xingxing Zhang and has been published by Springer Nature this book supported file pdf, txt, epub, kindle and other format this book has been release on 2021-09-11 with Social Science categories.
This book explores the interdisciplinary and transdisciplinary fields of energy systems, occupant behavior, thermal comfort, air quality and economic modelling across levels of building, communities and cities, through various data analytical approaches. It highlights the complex interplay of heating/cooling, ventilation and power systems in different processes, such as design, renovation and operation, for buildings, communities and cities. Methods from classical statistics, machine learning and artificial intelligence are applied into analyses for different building/urban components and systems. Knowledge from this book assists to accelerate sustainability of the society, which would contribute to a prospective improvement through data analysis in the liveability of both built and urban environment. This book targets a broad readership with specific experience and knowledge in data analysis, energy system, built environment and urban planning. As such, it appeals to researchers, graduate students, data scientists, engineers, consultants, urban scientists, investors and policymakers, with interests in energy flexibility, building/city resilience and climate neutrality.
Investigating The Human Behavior Side Of Building Energy Efficiency
DOWNLOAD
Author : Chao Chen
language : en
Publisher:
Release Date : 2013
Investigating The Human Behavior Side Of Building Energy Efficiency written by Chao Chen and has been published by this book supported file pdf, txt, epub, kindle and other format this book has been release on 2013 with categories.
We describe and evaluate each of these contributions using electricity consumption data from actual smart homes as part of the CASAS smart home project. In each case we illustrate the efficacy of these algorithms to gaining insights on human behavior and its impact on energy consumption, and offer ideas for using these insights to promote sustainable behaviors.
Scalable Data Driven Modeling And Analytics For Smart Buildings
DOWNLOAD
Author : Srinivasan Iyengar
language : en
Publisher:
Release Date : 2019
Scalable Data Driven Modeling And Analytics For Smart Buildings written by Srinivasan Iyengar and has been published by this book supported file pdf, txt, epub, kindle and other format this book has been release on 2019 with categories.
Buildings account for over 40% of the energy and 75% of the electricity usage. Thus, by reducing our energy footprint in buildings, we can improve our overall energysustainability. Further, the proliferation of networked sensors and IoT devices in recent years have enabled monitoring of buildings to provide data at various granularity. For example, smart plugs monitor appliance level usage inside the house, while solar meters monitor residential rooftop solar installations. Furthermore, smart meters record energy usage at a grid-scale. In this thesis, I argue that data-driven modeling applied to the IoT data from a smart building, at varying granularity, in association with third party data can help to understand and reduce human energy consumption. I present four data-driven modeling approaches - that use sophisticated techniques from Machine Learning, Optimization, and Time Series Analysis - applied at different granularities. First, I study IoT devices inside the house and discuss an approach called NIMD that automatically models individual electrical loads found in a household. The analytical model resulting from this approach can be used in several applications. For example, these models can improve the performance of NILM algorithms to disaggregate loads in a given household. Further, faulty or energy-inefficient appliances can be identified by observing deviations in model parameters over its lifetime. Second, I examine data from solar meters and present a machine learning framework called SolarCast to forecast energy generation from residential rooftop installations. The predictions enable exploiting the benefits of locally-generated solar energy. Third, I employ a sensorless approach utilizing a graphical model representation to report city-scale photovoltaic panel health and identify anomalies in solar energy production. Immediate identification of faults maximizes the solar investment by aiding in optimal operational performance. Finally, I focus on grid-level smart meter data and use correlations between energy usage and external weather to derive probabilistic estimates of energy, which is leveraged to identify the least efficient buildings from a large population along with the underlying cause of energy inefficiency. The identified homes can be targeted for custom energy efficiency programs.
Advanced Optimization Methods And Big Data Applications In Energy Demand Forecast
DOWNLOAD
Author : Federico Divina
language : en
Publisher: MDPI
Release Date : 2021-08-30
Advanced Optimization Methods And Big Data Applications In Energy Demand Forecast written by Federico Divina and has been published by MDPI this book supported file pdf, txt, epub, kindle and other format this book has been release on 2021-08-30 with Technology & Engineering categories.
The use of data collectors in energy systems is growing more and more. For example, smart sensors are now widely used in energy production and energy consumption systems. This implies that huge amounts of data are generated and need to be analyzed in order to extract useful insights from them. Such big data give rise to a number of opportunities and challenges for informed decision making. In recent years, researchers have been working very actively in order to come up with effective and powerful techniques in order to deal with the huge amount of data available. Such approaches can be used in the context of energy production and consumption considering the amount of data produced by all samples and measurements, as well as including many additional features. With them, automated machine learning methods for extracting relevant patterns, high-performance computing, or data visualization are being successfully applied to energy demand forecasting.
Internet Of Energy For Smart Cities
DOWNLOAD
Author : Anish Jindal
language : en
Publisher: CRC Press
Release Date : 2021-07-19
Internet Of Energy For Smart Cities written by Anish Jindal and has been published by CRC Press this book supported file pdf, txt, epub, kindle and other format this book has been release on 2021-07-19 with Computers categories.
Covers all aspects of Internet of Energy (IoE) and smart cities including research problems and solutions. Points to the solutions provided by machine learning to optimize the grids within a smart city set-up. Discusses relevant IoE design principles and architecture. Helps to automate various services in smart cities for energy management. Includes case studies to show the effectiveness of the discussed schemes.
Data Mining And Machine Learning In Building Energy Analysis
DOWNLOAD
Author : Frédéric Magoules
language : en
Publisher: John Wiley & Sons
Release Date : 2016-01-05
Data Mining And Machine Learning In Building Energy Analysis written by Frédéric Magoules and has been published by John Wiley & Sons this book supported file pdf, txt, epub, kindle and other format this book has been release on 2016-01-05 with Computers categories.
The energy consumption of a building has, in recent years, become a determining factor during its design and construction. With carbon footprints being a growing issue, it is important that buildings be optimized for energy conservation and CO2 reduction. This book therefore presents AI models and optimization techniques related to this application. The authors start with a review of recent models for the prediction of building energy consumption: engineering methods, statistical methods, artificial intelligence methods, ANNs and SVMs in particular. The book then focuses on SVMs, by first applying them to building energy consumption, then presenting the principles and various extensions, and SVR. The authors then move on to RDP, which they use to determine building energy faults through simulation experiments before presenting SVR model reduction methods and the benefits of parallel computing. The book then closes by presenting some of the current research and advancements in the field.