Variable And Model Selection For Propensity Score Estimators In Causal Inference
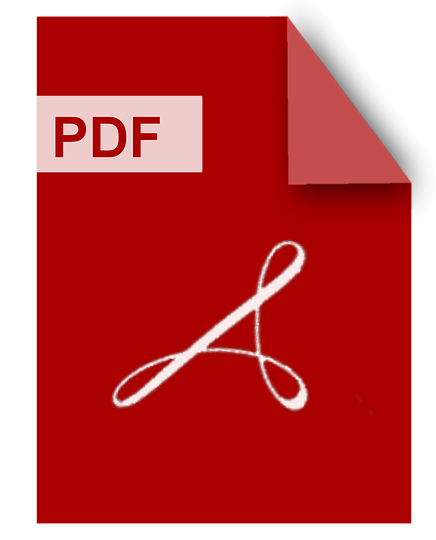
DOWNLOAD
Download Variable And Model Selection For Propensity Score Estimators In Causal Inference PDF/ePub or read online books in Mobi eBooks. Click Download or Read Online button to get Variable And Model Selection For Propensity Score Estimators In Causal Inference book now. This website allows unlimited access to, at the time of writing, more than 1.5 million titles, including hundreds of thousands of titles in various foreign languages. If the content not found or just blank you must refresh this page
Variable And Model Selection For Propensity Score Estimators In Causal Inference
DOWNLOAD
Author : Cheng Ju
language : en
Publisher:
Release Date : 2018
Variable And Model Selection For Propensity Score Estimators In Causal Inference written by Cheng Ju and has been published by this book supported file pdf, txt, epub, kindle and other format this book has been release on 2018 with categories.
Robust inference of a low-dimensional parameter in a large semi-parametric model relies on external estimators of infinite-dimensional features of the distribution of the data. Typically, only one of the latter is optimized for the sake of constructing a well behaved estimator of the low-dimensional parameter of interest. Optimizing more than one of them for the sake of achieving a better bias-variance trade-off in the estimation of the parameter of interest is the core idea driving the general template of the collaborative targeted minimum loss-based estimation (C-TMLE) procedure. In this dissertation, we first resolves the computational issue in the widely-used greedy variable selection C-TMLE. Then we further investigate how to extend the discrete, variable selection C-TMLE for a more general model selection purpose. Chapter 1 begins by introducing the framework of causal inference in observational studies. We introduce the non-parametric structural equation model for modeling the data generating distribution. We briefly review the targeted minimum loss-based estimation (TMLE). We also introduce the general template of C-TMLE and its greedy-search variable selection version. In chapter 2, we propose the template for scalable variable selection C-TMLEs to overcome the computational burden in the greedy variable selection C-TMLE. The original instantiation of the C-TMLE template can be presented as a greedy forward stepwise C-TMLE algorithm. It does not scale well when the number $p$ of covariates increases drastically. This motivates the introduction of a novel instantiation of the C-TMLE template where the covariates are pre-ordered. Its time complexity is $\mathcal{O}(p)$ as opposed to the original $\mathcal{O}(p^2)$, a remarkable gain. We propose two pre-ordering strategies and suggest a rule of thumb to develop other meaningful strategies. Because it is usually unclear a priori which pre-ordering strategy to choose, we also introduce another instantiation called SL-C-TMLE algorithm that enables the data-driven choice of the better pre-ordering strategy given the problem at hand. Its time complexity is $\mathcal{O}(p)$ as well. The computational burden and relative performance of these algorithms were compared in simulation studies involving fully synthetic data or partially synthetic data based on a real world large electronic health database; and in analyses of three real, large electronic health databases. In all analyses involving electronic health databases, the greedy C-TMLE algorithm is unacceptably slow. Simulation studies seem to indicate that our scalable C-TMLE and SL-C-TMLE algorithms work well. In chapter 3, we extend C-TMLE to a more general model selection problem: we apply C-TMLE to select from a set of continuously-indexed nuisance parameter (the propensity score, PS) estimators. The propensity score models have traditionally been selected based on the goodness-of-fit for the treatment mechanism itself, without consideration of the causal parameter of interest. In contrast, the C-TMLE takes into account information on the causal parameter of interest when selecting a PS model. This ``collaborative learning'' considers variable associations with both treatment and outcome when selecting a PS model in order to minimize a bias-variance trade off in the estimated treatment effect. In this study, we introduce a novel approach for collaborative model selection when using the LASSO estimator for PS estimation in high-dimensional covariate settings. To demonstrate the importance of selecting the PS model collaboratively, we designed quasi-experiments based on a real electronic healthcare database, where only the potential outcomes were manually generated, and the treatment and baseline covariates remained unchanged. Results showed that the C-TMLE algorithm outperformed other competing estimators for both point estimation and confidence interval coverage. In addition, the PS model selected by C-TMLE could be applied to other PS-based estimators, which also resulted in substantive improvement for both point estimation and confidence interval coverage. We illustrate the discussed concepts through an empirical example comparing the effects of non-selective Nonsteroidal anti-inflammatory drugs with selective COX-2 inhibitors on gastrointestinal complications in a population of Medicare beneficiaries. In chapter 4, we propose using C-TMLE to adaptively truncated the propensity score when there exist practical positivity violations. The positivity assumption, or the experimental treatment assignment (ETA) assumption, is important for identifiability in causal inference. Even if the positivity assumption holds, practical violations of this assumption may jeopardize the finite sample performance of the causal estimator. One of the consequences of practical violations of the positivity assumption is extreme values in the estimated propensity score. A common practice to address this issue is truncating the PS estimate when constructing PS-based estimators. In this study, we propose a novel adaptive truncation method, Positivity-C-TMLE, based on the C-TMLE methodology. We further show how to construct a robust confidence interval by a targeted variance estimator. We demonstrate the outstanding performance of our novel approach in a variety of simulations by comparing it with other commonly studied estimators, for both point estimation and confidence interval coverage. Results show that by adaptively truncating the estimated PS with a more targeted objective function, the Positivity-C-TMLE estimator achieves the best performance for both point estimation and confidence interval coverage among all estimators considered. The code for all the variations of C-TMLE in this dissertation are publicly available in the \emph{ctmle} R package.
Causal Inference With Selection And Confounding Variables
DOWNLOAD
Author : Linbo Wang
language : en
Publisher:
Release Date : 2016
Causal Inference With Selection And Confounding Variables written by Linbo Wang and has been published by this book supported file pdf, txt, epub, kindle and other format this book has been release on 2016 with categories.
Most complex observational and randomized studies are motivated by the potential of drawing causal statements. However, a usual statistical analysis may yield estimates that do not have causal interpretations. In fact, unlike most other parameters, identification of causal parameters usually relies on untestable assumptions. Moreover, even under these identification assumptions, estimation of causal parameters often relies on nuisance models. The parameter estimation in the nuisance models is crucial to obtain robust causal effect estimates. My research attempts to address these methodological hallenges. In Chapter 2 we study robust estimation of propensity score weights. The propensity score plays a central role in inferring causal effects from observational studies. In particular, weighting and subclassification are two principal approaches to estimate the average causal effect based on estimated propensity scores. Unlike the conventional version of the propensity score subclassification estimator, if the propensity score model is correctly specified, the weighting methods offer consistent and possibly efficient estimation of the average causal effect. However, this theoretical appeal may be diminished in practice by sensitivity to misspecification of the propensity score model. In contrast, subclassification methods are usually more robust to model misspecification. We hence propose to use subclassification for robust estimation of propensity score weights. Our approach is based on the intuition that the inverse probability weighting estimator can be seen as the limit of subclassification estimators as the number of subclasses goes to infinity. By formalizing this intuition, we propose novel propensity score weighting estimators that are both consistent and robust to model misspecification. Empirical studies show that the proposed estimators perform favorably compared to existing methods. In Chapter 3 we study identification and estimation of causal effects with outcomes truncated by death. It is common that in medical studies, the outcome of interest is truncated by death, meaning that a subject had died before the outcome could be measured. In this case, restricted analysis among survivors may be subject to selection bias. It is hence of interest to estimate the survivor average causal effect (SACE), defined as the average causal effect among subjects who would survive under either exposure. In this chapter, we consider the identification and estimation problems of the SACE. We propose to identify a substitution variable for the latent membership of the always-survivor group. The identifiability conditions required for a substitution variable are similar in idea to conditions for an instrumental variable. We show that the SACE is identifiable with use of a substitution variable, and propose novel model parameterizations for estimation of the SACE under our identification assumptions. Our approaches are illustrated via simulation studies and two data analyses. In Chapter 4, we study causal analysis of ordinal treatments and binary outcomes under truncation by death. It is common that in multi-arm randomized trials, the outcome of interest is “truncated by death,” meaning that it is only observed or well-defined conditioning on an intermediate outcome. In this case, in addition to pairwise contrasts, the joint inference for all treatment arms is also of interest. Under a monotonicity assumption we present methods for both pairwise and joint causal analyses of ordinal treatments and binary outcomes in presence of truncation by death. We illustrate via examples the appropriateness of our assumptions in different scientific contexts.
Propensity Score Methods And Applications
DOWNLOAD
Author : Haiyan Bai
language : en
Publisher: SAGE Publications
Release Date : 2018-11-20
Propensity Score Methods And Applications written by Haiyan Bai and has been published by SAGE Publications this book supported file pdf, txt, epub, kindle and other format this book has been release on 2018-11-20 with Social Science categories.
A concise, introductory text, Propensity Score Methods and Applications describes propensity score methods (PSM) and how they are used to balance the distributions of observed covariates between treatment conditions as a means to reduce selection bias. This new QASS title specifically focuses on the procedures of implementing PSM for research in social sciences, instead of merely demonstrating the effectiveness of the method. Using succinct and approachable language to introduce the basic concepts of PSM, authors Haiyan Bai and M. H. Clark present basic concepts, assumptions, procedures, available software packages, and step-by-step examples for implementing PSM using real-world data, with exercises at the end of each chapter allowing readers to replicate examples on their own.
Statistical Causal Inferences And Their Applications In Public Health Research
DOWNLOAD
Author : Hua He
language : en
Publisher: Springer
Release Date : 2016-10-26
Statistical Causal Inferences And Their Applications In Public Health Research written by Hua He and has been published by Springer this book supported file pdf, txt, epub, kindle and other format this book has been release on 2016-10-26 with Medical categories.
This book compiles and presents new developments in statistical causal inference. The accompanying data and computer programs are publicly available so readers may replicate the model development and data analysis presented in each chapter. In this way, methodology is taught so that readers may implement it directly. The book brings together experts engaged in causal inference research to present and discuss recent issues in causal inference methodological development. This is also a timely look at causal inference applied to scenarios that range from clinical trials to mediation and public health research more broadly. In an academic setting, this book will serve as a reference and guide to a course in causal inference at the graduate level (Master's or Doctorate). It is particularly relevant for students pursuing degrees in statistics, biostatistics, and computational biology. Researchers and data analysts in public health and biomedical research will also find this book to be an important reference.
Treatment Effect Estimation With Censored Outcome And Covariate Selection
DOWNLOAD
Author : Li Li
language : en
Publisher:
Release Date : 2023
Treatment Effect Estimation With Censored Outcome And Covariate Selection written by Li Li and has been published by this book supported file pdf, txt, epub, kindle and other format this book has been release on 2023 with categories.
Covariates selection is essential when faced with many variables in modern causal inference in a data-rich environment. Particularly, the efficiency of the average causal effect (ACE) can be improved by including covariates only related to the outcome and reduced by including covariates related to the treatment but not the outcome in the propensity score (PS) model. In this paper, we estimate the causal effect in the presence of censored outcome and high-dimensional covariates. To improve the efficiency of the estimation of ACE, we propose the censored outcome adaptive Lasso (COAL) to select covariates, where the weighted least square method is applied to account for censoring. Based on the covariate selection, we propose a double inverse propensity weighted estimator for ACE. Furthermore, we establish the oracle properties of the variable selection and derive the asymptotic properties of the proposed estimator.
Secondary Analysis Of Electronic Health Records
DOWNLOAD
Author : MIT Critical Data
language : en
Publisher: Springer
Release Date : 2016-09-09
Secondary Analysis Of Electronic Health Records written by MIT Critical Data and has been published by Springer this book supported file pdf, txt, epub, kindle and other format this book has been release on 2016-09-09 with Medical categories.
This book trains the next generation of scientists representing different disciplines to leverage the data generated during routine patient care. It formulates a more complete lexicon of evidence-based recommendations and support shared, ethical decision making by doctors with their patients. Diagnostic and therapeutic technologies continue to evolve rapidly, and both individual practitioners and clinical teams face increasingly complex ethical decisions. Unfortunately, the current state of medical knowledge does not provide the guidance to make the majority of clinical decisions on the basis of evidence. The present research infrastructure is inefficient and frequently produces unreliable results that cannot be replicated. Even randomized controlled trials (RCTs), the traditional gold standards of the research reliability hierarchy, are not without limitations. They can be costly, labor intensive, and slow, and can return results that are seldom generalizable to every patient population. Furthermore, many pertinent but unresolved clinical and medical systems issues do not seem to have attracted the interest of the research enterprise, which has come to focus instead on cellular and molecular investigations and single-agent (e.g., a drug or device) effects. For clinicians, the end result is a bit of a “data desert” when it comes to making decisions. The new research infrastructure proposed in this book will help the medical profession to make ethically sound and well informed decisions for their patients.
The Sage Handbook Of Regression Analysis And Causal Inference
DOWNLOAD
Author : Henning Best
language : en
Publisher: SAGE
Release Date : 2013-12-20
The Sage Handbook Of Regression Analysis And Causal Inference written by Henning Best and has been published by SAGE this book supported file pdf, txt, epub, kindle and other format this book has been release on 2013-12-20 with Social Science categories.
′The editors of the new SAGE Handbook of Regression Analysis and Causal Inference have assembled a wide-ranging, high-quality, and timely collection of articles on topics of central importance to quantitative social research, many written by leaders in the field. Everyone engaged in statistical analysis of social-science data will find something of interest in this book.′ - John Fox, Professor, Department of Sociology, McMaster University ′The authors do a great job in explaining the various statistical methods in a clear and simple way - focussing on fundamental understanding, interpretation of results, and practical application - yet being precise in their exposition.′ - Ben Jann, Executive Director, Institute of Sociology, University of Bern ′Best and Wolf have put together a powerful collection, especially valuable in its separate discussions of uses for both cross-sectional and panel data analysis.′ -Tom Smith, Senior Fellow, NORC, University of Chicago Edited and written by a team of leading international social scientists, this Handbook provides a comprehensive introduction to multivariate methods. The Handbook focuses on regression analysis of cross-sectional and longitudinal data with an emphasis on causal analysis, thereby covering a large number of different techniques including selection models, complex samples, and regression discontinuities. Each Part starts with a non-mathematical introduction to the method covered in that section, giving readers a basic knowledge of the method’s logic, scope and unique features. Next, the mathematical and statistical basis of each method is presented along with advanced aspects. Using real-world data from the European Social Survey (ESS) and the Socio-Economic Panel (GSOEP), the book provides a comprehensive discussion of each method’s application, making this an ideal text for PhD students and researchers embarking on their own data analysis.
Propensity Score Matching And Policy Impact Analysis
DOWNLOAD
Author : Boniface Essama-Nssah
language : en
Publisher: World Bank Publications
Release Date : 2006
Propensity Score Matching And Policy Impact Analysis written by Boniface Essama-Nssah and has been published by World Bank Publications this book supported file pdf, txt, epub, kindle and other format this book has been release on 2006 with Estimation theory categories.
Effective development policymaking creates a need for reliable methods of assessing effectiveness. There should be, therefore, an intimate relationship between effective policymaking and impact analysis. The goal of a development intervention defines the metric by which to assess its impact, while impact evaluation can produce reliable information on which policymakers may base decisions to modify or cancel ineffective programs and thus make the most of limited resources. This paper reviews the logic of propensity score matching (PSM) and, using data on the National Support Work Demonstration, compares that approach with other evaluation methods such as double difference, instrumental variable, and Heckman's method of selection bias correction. In addition, it demonstrates how to implement nearest-neighbor and kernel-based methods, and plot program incidence curves in E-Views. In the end, the plausibility of an evaluation method hinges critically on the correctness of the socioeconomic model underlying program design and implementation, and on the quality and quantity of available data. In any case, PSM can act as an effective adjuvant to other methods.
Propensity Score Analysis
DOWNLOAD
Author : Shenyang Guo
language : en
Publisher: SAGE
Release Date : 2015
Propensity Score Analysis written by Shenyang Guo and has been published by SAGE this book supported file pdf, txt, epub, kindle and other format this book has been release on 2015 with Business & Economics categories.
Provides readers with a systematic review of the origins, history, and statistical foundations of Propensity Score Analysis (PSA) and illustrates how it can be used for solving evaluation and causal-inference problems.
Developing A Protocol For Observational Comparative Effectiveness Research A User S Guide
DOWNLOAD
Author : Agency for Health Care Research and Quality (U.S.)
language : en
Publisher: Government Printing Office
Release Date : 2013-02-21
Developing A Protocol For Observational Comparative Effectiveness Research A User S Guide written by Agency for Health Care Research and Quality (U.S.) and has been published by Government Printing Office this book supported file pdf, txt, epub, kindle and other format this book has been release on 2013-02-21 with Medical categories.
This User’s Guide is a resource for investigators and stakeholders who develop and review observational comparative effectiveness research protocols. It explains how to (1) identify key considerations and best practices for research design; (2) build a protocol based on these standards and best practices; and (3) judge the adequacy and completeness of a protocol. Eleven chapters cover all aspects of research design, including: developing study objectives, defining and refining study questions, addressing the heterogeneity of treatment effect, characterizing exposure, selecting a comparator, defining and measuring outcomes, and identifying optimal data sources. Checklists of guidance and key considerations for protocols are provided at the end of each chapter. The User’s Guide was created by researchers affiliated with AHRQ’s Effective Health Care Program, particularly those who participated in AHRQ’s DEcIDE (Developing Evidence to Inform Decisions About Effectiveness) program. Chapters were subject to multiple internal and external independent reviews. More more information, please consult the Agency website: www.effectivehealthcare.ahrq.gov)