Variable Selection With Incomplete Covariate Data
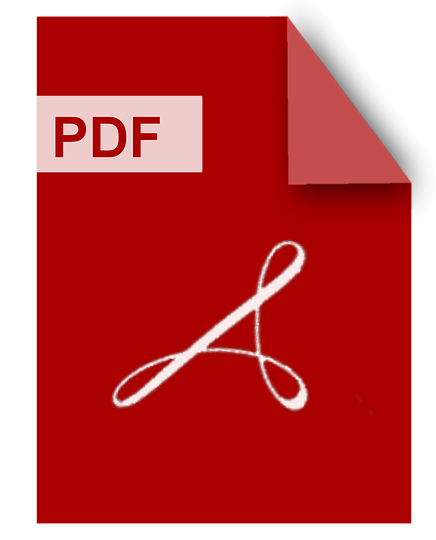
DOWNLOAD
Download Variable Selection With Incomplete Covariate Data PDF/ePub or read online books in Mobi eBooks. Click Download or Read Online button to get Variable Selection With Incomplete Covariate Data book now. This website allows unlimited access to, at the time of writing, more than 1.5 million titles, including hundreds of thousands of titles in various foreign languages. If the content not found or just blank you must refresh this page
Variable Selection With Incomplete Covariate Data
DOWNLOAD
Author :
language : en
Publisher:
Release Date : 2007
Variable Selection With Incomplete Covariate Data written by and has been published by this book supported file pdf, txt, epub, kindle and other format this book has been release on 2007 with categories.
Topics On Bayesian Analysis Of Missing Data
DOWNLOAD
Author : Yun Kai Jiang
language : en
Publisher:
Release Date : 2011
Topics On Bayesian Analysis Of Missing Data written by Yun Kai Jiang and has been published by this book supported file pdf, txt, epub, kindle and other format this book has been release on 2011 with categories.
This dissertation focuses on model selection in logistic regression with incompletely observed data. In particular, methods are presented for using Markov Chain Monte Carlo imputation and Bayesian variable selection to model a binary outcome. We consider multivariate missing covariates, with different types of predictors, such as continuous, counts, and categorical variables. Such type of data is considered in the analysis of Project Talent recorded from a longitudinal study. Roughly 400,000 were selected for the study from United States high school students in grades 9 through 12 during the year 1960; follow-up surveys were conducted 1, 5, and 11 years after graduation. We extend a methodology developed by Yang, Belin, and Boscardin (2005), to this Project Talent for a logistic regression model with incomplete covariates. The idea is to use data information as much as possible to fill in the missing values and study associations between a binary response variable and covariates. According to Yang, Belin, and Boscardin, one approach under a multivariate normal assumption for data, is to conduct Bayesian variable selection and missing data imputation simultaneously within one Gibbs Sampling process, called "Simultaneously Impute And Select" (SIAS). A modified strategy of SIAS is extended to a mixed data structure that allows for categorical, counts, and continuous variables. The first chapter consists of an introduction to some approaches to variable selection for missing data. The fact that missing data arise commonly in statistical analyses, leads to a variety of methods to handle missing data. The missing data mechanism needs to be considered in imputations. The multiple imputation methods and Markov Chain Mote Carlo (MCMC) algorithms are presented as general statistical approaches to missing data analysis. In the MCMC computational toolbox, various implementation methods for imputation are discussed: Metropolis-Hasting, Gibbs Sampler, and Data Augmentation. Compared to model selection methods in frequentist and likelihood inference, Bayesian inference takes an entirely different approach. The frequentist approach only looks at the current data to make inference. The Bayesian approach requires the specification of the prior distribution, which can come from historical data or expert opinion. Stochastic Search Variable Selection (SSVS) and Gibbs Variable Selection (GVS) are reviewed for model selection. Two alternative strategies, Impute Then Select (ITS) and Simultaneously Impute And Select (SIAS), are studied. In the second chapter, imputation and Bayesian variable selection methods for linear regression are extended to a binary response variable that is completely observed, but some covariates have missing values. We focus on extending SIAS strategy to logistic regression models via two alternative imputations, decomposition and Fully Conditional Specification (FCS). The decomposition method breaks a multivariate distribution into a series of univariate ones by decomposing the joint density function p(Y, X1, ..., X[p]) into the product of conditional distributions, using the factorization p(A, B) = p(A[vertical line]B)p(B). The FCS aims to involve iteratively sampling from the conditional distributions for one random variable, given all the others. These two methods are implemented in the imputation step of the SIAS procedure then applied to the Project Talent data. Simulations are also performed to validate these results and demonstrate the superiority of FCS over the decomposition method under certain circumstances. The third chapter presents a new approach for incorporating the sampling weight into imputation and Bayesian variable selection in logistic regression models. We develop the approach that extends SIAS by a Bayesian version of iterative weighted least squares algorithm to include a sampling step based on Gibbs sampler. This approach is illustrated using both simulation studies and Project Talent data.
Marginal Causal Sub Group Analysis With Incomplete Covariate Data
DOWNLOAD
Author : Meaghan S. Cuerden
language : en
Publisher:
Release Date : 2018
Marginal Causal Sub Group Analysis With Incomplete Covariate Data written by Meaghan S. Cuerden and has been published by this book supported file pdf, txt, epub, kindle and other format this book has been release on 2018 with Causation categories.
Incomplete data arises frequently in health research studies designed to investigate the causal relationship between a treatment or exposure, and a response of interest. Statistical methods for conditional causal effect parameters in the setting of incomplete data have been developed, and we expand upon these methods for estimating marginal causal effect parameters. This thesis focuses on the estimation of marginal causal odds ratios, which are distinct from conditional causal odds ratios in logistic regression models; marginal causal odds ratios are frequently of interest in population studies. We introduce three methods for estimating the marginal causal odds ratio of a binary response for different levels of a subgroup variable, where the subgroup variable is incomplete. In each chapter, the subgroup variable, exposure variable and the response variable are binary and the subgroup variable is missing at random. In Chapter 2, we begin with an overview of inverse probability weighted methods for confounding in an observational setting where data are complete. We also briefly review methods to deal with incomplete data in a randomized setting. We then introduce a doubly inverse probability weighted estimating equation approach to estimate marginal causal odds ratios in an observational setting, where an important subgroup variable is incomplete. One inverse probability weight accounts for the incomplete data, and the other weight accounts for treatment selection. Only complete cases are included in the response model. Consistency results are derived, and a method to obtain estimates of the asymptotic standard error is introduced; the extra variability introduced by estimating two weights is incorporated in the estimation of the asymptotic standard error. We give a method for hypothesis testing and calculation of confidence intervals. Simulation studies show that the doubly weighted estimating equation approach is effective in a non-ignorable missingness setting with confounding, and it is straightforward to implement. It also performs well when the missing data process is ignorable, and/or when confounding is not present. In Chapter 3, we begin with an overview of an EM algorithm approach for estimating conditional causal effect parameters in the setting of incomplete covariate data, in both randomized and observational settings. We then propose the use of a doubly weighted EM-type algorithm approach to estimate the marginal causal odds ratio in the setting of missing subgroup data. In this method, instead of using complete case analysis in the response model, all available data is used and the incomplete subgroup variable is “filled in” using a maximum likelihood approach. Two inverse probability weights are used here as well, to account for confounding and incomplete data. The weight which accounts for the incomplete data is needed, even though an EM approach is being used, because the marginal causal odds ratio is of interest. A method to obtain asymptotic standard error estimates is given where the extra variability introduced by estimating the two inverse probability weights, as well as the variability introduced by estimating the conditional expectation of the incomplete subgroup variable, is incorporated. Simulation studies show that this method is effective in terms of obtaining consistent estimates of the parameters of interest; however it is difficult to implement, and in certain settings there is a loss of efficiency in comparison to the methods introduced in Chapter 2. In Chapter 4, we begin by reviewing multiple imputation methods in randomized and observational settings, where estimation of the conditional causal odds ratio is of interest. We then propose the use of multiple imputation with one inverse probability weight to account for confounding in an observational setting where the subgroup variable is incomplete. We discuss methods to correctly specify the imputation model in the setting where the conditional causal odds ratio is of interest, as well as in the setting where the marginal causal odds ratio is of interest. We use standard methods for combining the estimates of the marginal log odds ratios from each imputed dataset. We propose a method for estimating the asymptotic standard error of the estimates, which incorporates both the estimation of the parameters in the weight for confounding, and the multiply imputed datasets. We give a method for hypothesis testing and calculation of confidence intervals. Simulation studies show that this method is efficient and straightforward to implement, but correct specification of the imputation model is necessary. In Chapter 5, the three methods that have been introduced are used in an application to an observational cohort study of 418 colorectal cancer patients. We compare patients who received an experimental chemotherapy with patients who received standard chemotherapy; of interest is estimation of the marginal causal odds ratio of a thrombotic event during the course of treatment or 30 days after treatment is discontinued. The important subgroups are (i) patients receiving first line of treatment, and (ii) patients receiving second line of treatment. In Chapter 6, we compare and contrast the three methods proposed. We also discuss extensions to different response models, models for missing response data, and weighted models in the longitudinal data setting.
Bias Reduction In Variable Selection And The Analysis Of Competing Risks With Missing Covariate Values With Right Censored Data
DOWNLOAD
Author : Chang-Heok Soh
language : en
Publisher:
Release Date : 2006
Bias Reduction In Variable Selection And The Analysis Of Competing Risks With Missing Covariate Values With Right Censored Data written by Chang-Heok Soh and has been published by this book supported file pdf, txt, epub, kindle and other format this book has been release on 2006 with Analysis of covariance categories.
Flexible Imputation Of Missing Data Second Edition
DOWNLOAD
Author : Stef van Buuren
language : en
Publisher: CRC Press
Release Date : 2018-07-17
Flexible Imputation Of Missing Data Second Edition written by Stef van Buuren and has been published by CRC Press this book supported file pdf, txt, epub, kindle and other format this book has been release on 2018-07-17 with Mathematics categories.
Missing data pose challenges to real-life data analysis. Simple ad-hoc fixes, like deletion or mean imputation, only work under highly restrictive conditions, which are often not met in practice. Multiple imputation replaces each missing value by multiple plausible values. The variability between these replacements reflects our ignorance of the true (but missing) value. Each of the completed data set is then analyzed by standard methods, and the results are pooled to obtain unbiased estimates with correct confidence intervals. Multiple imputation is a general approach that also inspires novel solutions to old problems by reformulating the task at hand as a missing-data problem. This is the second edition of a popular book on multiple imputation, focused on explaining the application of methods through detailed worked examples using the MICE package as developed by the author. This new edition incorporates the recent developments in this fast-moving field. This class-tested book avoids mathematical and technical details as much as possible: formulas are accompanied by verbal statements that explain the formula in accessible terms. The book sharpens the reader’s intuition on how to think about missing data, and provides all the tools needed to execute a well-grounded quantitative analysis in the presence of missing data.
Statistical Methods For Analyzing Missing Covariate Data
DOWNLOAD
Author : Lan Huang
language : en
Publisher:
Release Date : 2004
Statistical Methods For Analyzing Missing Covariate Data written by Lan Huang and has been published by this book supported file pdf, txt, epub, kindle and other format this book has been release on 2004 with Electronic dissertations categories.
Missing covariate data often arise in various settings, including surveys, clinical trials, epidemiological studies, biological studies and environmental studies. Large scale studies often have large fractions of missing data, which can present serious problems to the data analyst. Motivated by real data applications, this dissertation addresses several aspects in modeling and analyzing data with missing covariates. First, we propose Bayesian methods for estimating parameters in generalized linear models (GLM's) with nonignorably missing covariate data. We specify a parametric distribution for the response variable given the covariates (GLM), a parametric distribution for the missing covariates, and a parametric multinomial selection model for the missing data mechanism. Then we characterize general conditions for the propriety of the joint posterior distribution of the parameters and extend two model selection criteria, weighted L measure and Deviance Information Criterion for model comparison in the presence of missing covariates. Second, we develop a novel modeling strategy for analyzing data with repeated binary responses over time as well as with time-dependent missing covariates. We use the generalized linear mixed logistic model for the repeated binary responses and then propose a joint model for time-dependent missing covariates using information from different sources. The Monte Carlo EM algorithm is developed for computing the maximum likelihood estimates. An extended version of the AIC criterion is proposed to identify factors of interest that may disrupt the cyclical pattern of flowering. Third, we develop an efficient Gibbs sampling algorithm to sample from the joint posterior distribution for the generalized linear mixed logistic model. Moreover, we propose a novel Monte Carlo method to compute a Bayesian model comparison criterion, DIC, for any variable subset model using a single Markov Chain Monte Carlo sample from the full model without sampling from the posterior distribution under each subset model. In the end, we provide a brief discussion of future research.
Developing A Protocol For Observational Comparative Effectiveness Research A User S Guide
DOWNLOAD
Author : Agency for Health Care Research and Quality (U.S.)
language : en
Publisher: Government Printing Office
Release Date : 2013-02-21
Developing A Protocol For Observational Comparative Effectiveness Research A User S Guide written by Agency for Health Care Research and Quality (U.S.) and has been published by Government Printing Office this book supported file pdf, txt, epub, kindle and other format this book has been release on 2013-02-21 with Medical categories.
This User’s Guide is a resource for investigators and stakeholders who develop and review observational comparative effectiveness research protocols. It explains how to (1) identify key considerations and best practices for research design; (2) build a protocol based on these standards and best practices; and (3) judge the adequacy and completeness of a protocol. Eleven chapters cover all aspects of research design, including: developing study objectives, defining and refining study questions, addressing the heterogeneity of treatment effect, characterizing exposure, selecting a comparator, defining and measuring outcomes, and identifying optimal data sources. Checklists of guidance and key considerations for protocols are provided at the end of each chapter. The User’s Guide was created by researchers affiliated with AHRQ’s Effective Health Care Program, particularly those who participated in AHRQ’s DEcIDE (Developing Evidence to Inform Decisions About Effectiveness) program. Chapters were subject to multiple internal and external independent reviews. More more information, please consult the Agency website: www.effectivehealthcare.ahrq.gov)
Statistical Methods For Incomplete Covariates And Two Phase Designs
DOWNLOAD
Author : Michael McIsaac
language : en
Publisher:
Release Date : 2013
Statistical Methods For Incomplete Covariates And Two Phase Designs written by Michael McIsaac and has been published by this book supported file pdf, txt, epub, kindle and other format this book has been release on 2013 with categories.
Handbook Of Missing Data Methodology
DOWNLOAD
Author : Geert Molenberghs
language : en
Publisher: CRC Press
Release Date : 2014-11-06
Handbook Of Missing Data Methodology written by Geert Molenberghs and has been published by CRC Press this book supported file pdf, txt, epub, kindle and other format this book has been release on 2014-11-06 with Mathematics categories.
Missing data affect nearly every discipline by complicating the statistical analysis of collected data. But since the 1990s, there have been important developments in the statistical methodology for handling missing data. Written by renowned statisticians in this area, Handbook of Missing Data Methodology presents many methodological advances and the latest applications of missing data methods in empirical research. Divided into six parts, the handbook begins by establishing notation and terminology. It reviews the general taxonomy of missing data mechanisms and their implications for analysis and offers a historical perspective on early methods for handling missing data. The following three parts cover various inference paradigms when data are missing, including likelihood and Bayesian methods; semi-parametric methods, with particular emphasis on inverse probability weighting; and multiple imputation methods. The next part of the book focuses on a range of approaches that assess the sensitivity of inferences to alternative, routinely non-verifiable assumptions about the missing data process. The final part discusses special topics, such as missing data in clinical trials and sample surveys as well as approaches to model diagnostics in the missing data setting. In each part, an introduction provides useful background material and an overview to set the stage for subsequent chapters. Covering both established and emerging methodologies for missing data, this book sets the scene for future research. It provides the framework for readers to delve into research and practical applications of missing data methods.
Multiple Imputation Of Missing Data In Practice
DOWNLOAD
Author : Yulei He
language : en
Publisher: CRC Press
Release Date : 2021-11-20
Multiple Imputation Of Missing Data In Practice written by Yulei He and has been published by CRC Press this book supported file pdf, txt, epub, kindle and other format this book has been release on 2021-11-20 with Mathematics categories.
Multiple Imputation of Missing Data in Practice: Basic Theory and Analysis Strategies provides a comprehensive introduction to the multiple imputation approach to missing data problems that are often encountered in data analysis. Over the past 40 years or so, multiple imputation has gone through rapid development in both theories and applications. It is nowadays the most versatile, popular, and effective missing-data strategy that is used by researchers and practitioners across different fields. There is a strong need to better understand and learn about multiple imputation in the research and practical community. Accessible to a broad audience, this book explains statistical concepts of missing data problems and the associated terminology. It focuses on how to address missing data problems using multiple imputation. It describes the basic theory behind multiple imputation and many commonly-used models and methods. These ideas are illustrated by examples from a wide variety of missing data problems. Real data from studies with different designs and features (e.g., cross-sectional data, longitudinal data, complex surveys, survival data, studies subject to measurement error, etc.) are used to demonstrate the methods. In order for readers not only to know how to use the methods, but understand why multiple imputation works and how to choose appropriate methods, simulation studies are used to assess the performance of the multiple imputation methods. Example datasets and sample programming code are either included in the book or available at a github site (https://github.com/he-zhang-hsu/multiple_imputation_book). Key Features Provides an overview of statistical concepts that are useful for better understanding missing data problems and multiple imputation analysis Provides a detailed discussion on multiple imputation models and methods targeted to different types of missing data problems (e.g., univariate and multivariate missing data problems, missing data in survival analysis, longitudinal data, complex surveys, etc.) Explores measurement error problems with multiple imputation Discusses analysis strategies for multiple imputation diagnostics Discusses data production issues when the goal of multiple imputation is to release datasets for public use, as done by organizations that process and manage large-scale surveys with nonresponse problems For some examples, illustrative datasets and sample programming code from popular statistical packages (e.g., SAS, R, WinBUGS) are included in the book. For others, they are available at a github site (https://github.com/he-zhang-hsu/multiple_imputation_book)