A Data Scientist S Guide To Acquiring Cleaning And Managing Data In R
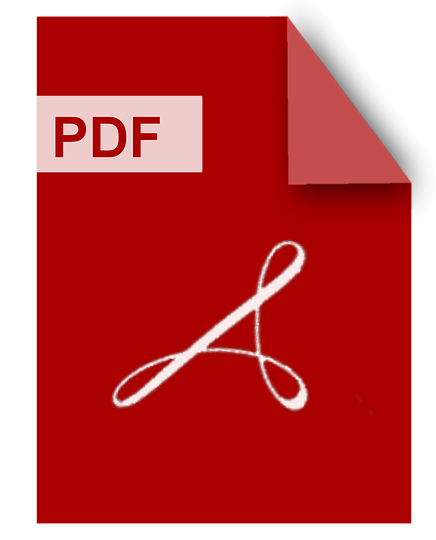
DOWNLOAD
Download A Data Scientist S Guide To Acquiring Cleaning And Managing Data In R PDF/ePub or read online books in Mobi eBooks. Click Download or Read Online button to get A Data Scientist S Guide To Acquiring Cleaning And Managing Data In R book now. This website allows unlimited access to, at the time of writing, more than 1.5 million titles, including hundreds of thousands of titles in various foreign languages. If the content not found or just blank you must refresh this page
A Data Scientist S Guide To Acquiring Cleaning And Managing Data In R
DOWNLOAD
Author : Samuel E. Buttrey
language : en
Publisher: John Wiley & Sons
Release Date : 2017-10-24
A Data Scientist S Guide To Acquiring Cleaning And Managing Data In R written by Samuel E. Buttrey and has been published by John Wiley & Sons this book supported file pdf, txt, epub, kindle and other format this book has been release on 2017-10-24 with Computers categories.
The only how-to guide offering a unified, systemic approach to acquiring, cleaning, and managing data in R Every experienced practitioner knows that preparing data for modeling is a painstaking, time-consuming process. Adding to the difficulty is that most modelers learn the steps involved in cleaning and managing data piecemeal, often on the fly, or they develop their own ad hoc methods. This book helps simplify their task by providing a unified, systematic approach to acquiring, modeling, manipulating, cleaning, and maintaining data in R. Starting with the very basics, data scientists Samuel E. Buttrey and Lyn R. Whitaker walk readers through the entire process. From what data looks like and what it should look like, they progress through all the steps involved in getting data ready for modeling. They describe best practices for acquiring data from numerous sources; explore key issues in data handling, including text/regular expressions, big data, parallel processing, merging, matching, and checking for duplicates; and outline highly efficient and reliable techniques for documenting data and recordkeeping, including audit trails, getting data back out of R, and more. The only single-source guide to R data and its preparation, it describes best practices for acquiring, manipulating, cleaning, and maintaining data Begins with the basics and walks readers through all the steps necessary to get data ready for the modeling process Provides expert guidance on how to document the processes described so that they are reproducible Written by seasoned professionals, it provides both introductory and advanced techniques Features case studies with supporting data and R code, hosted on a companion website A Data Scientist's Guide to Acquiring, Cleaning and Managing Data in R is a valuable working resource/bench manual for practitioners who collect and analyze data, lab scientists and research associates of all levels of experience, and graduate-level data mining students.
A Data Scientist S Guide To Acquiring Cleaning And Managing Data In R
DOWNLOAD
Author : Samuel E. Buttrey
language : en
Publisher: John Wiley & Sons
Release Date : 2017-10-24
A Data Scientist S Guide To Acquiring Cleaning And Managing Data In R written by Samuel E. Buttrey and has been published by John Wiley & Sons this book supported file pdf, txt, epub, kindle and other format this book has been release on 2017-10-24 with Computers categories.
The only how-to guide offering a unified, systemic approach to acquiring, cleaning, and managing data in R Every experienced practitioner knows that preparing data for modeling is a painstaking, time-consuming process. Adding to the difficulty is that most modelers learn the steps involved in cleaning and managing data piecemeal, often on the fly, or they develop their own ad hoc methods. This book helps simplify their task by providing a unified, systematic approach to acquiring, modeling, manipulating, cleaning, and maintaining data in R. Starting with the very basics, data scientists Samuel E. Buttrey and Lyn R. Whitaker walk readers through the entire process. From what data looks like and what it should look like, they progress through all the steps involved in getting data ready for modeling. They describe best practices for acquiring data from numerous sources; explore key issues in data handling, including text/regular expressions, big data, parallel processing, merging, matching, and checking for duplicates; and outline highly efficient and reliable techniques for documenting data and recordkeeping, including audit trails, getting data back out of R, and more. The only single-source guide to R data and its preparation, it describes best practices for acquiring, manipulating, cleaning, and maintaining data Begins with the basics and walks readers through all the steps necessary to get data ready for the modeling process Provides expert guidance on how to document the processes described so that they are reproducible Written by seasoned professionals, it provides both introductory and advanced techniques Features case studies with supporting data and R code, hosted on a companion website A Data Scientist's Guide to Acquiring, Cleaning and Managing Data in R is a valuable working resource/bench manual for practitioners who collect and analyze data, lab scientists and research associates of all levels of experience, and graduate-level data mining students.
R For Data Science
DOWNLOAD
Author : Hadley Wickham
language : en
Publisher: "O'Reilly Media, Inc."
Release Date : 2016-12-12
R For Data Science written by Hadley Wickham and has been published by "O'Reilly Media, Inc." this book supported file pdf, txt, epub, kindle and other format this book has been release on 2016-12-12 with Computers categories.
Learn how to use R to turn raw data into insight, knowledge, and understanding. This book introduces you to R, RStudio, and the tidyverse, a collection of R packages designed to work together to make data science fast, fluent, and fun. Suitable for readers with no previous programming experience, R for Data Science is designed to get you doing data science as quickly as possible. Authors Hadley Wickham and Garrett Grolemund guide you through the steps of importing, wrangling, exploring, and modeling your data and communicating the results. You'll get a complete, big-picture understanding of the data science cycle, along with basic tools you need to manage the details. Each section of the book is paired with exercises to help you practice what you've learned along the way. You'll learn how to: Wrangle—transform your datasets into a form convenient for analysis Program—learn powerful R tools for solving data problems with greater clarity and ease Explore—examine your data, generate hypotheses, and quickly test them Model—provide a low-dimensional summary that captures true "signals" in your dataset Communicate—learn R Markdown for integrating prose, code, and results
Statistical Data Cleaning With Applications In R
DOWNLOAD
Author : Mark van der Loo
language : en
Publisher: John Wiley & Sons
Release Date : 2018-04-23
Statistical Data Cleaning With Applications In R written by Mark van der Loo and has been published by John Wiley & Sons this book supported file pdf, txt, epub, kindle and other format this book has been release on 2018-04-23 with Computers categories.
A comprehensive guide to automated statistical data cleaning The production of clean data is a complex and time-consuming process that requires both technical know-how and statistical expertise. Statistical Data Cleaning brings together a wide range of techniques for cleaning textual, numeric or categorical data. This book examines technical data cleaning methods relating to data representation and data structure. A prominent role is given to statistical data validation, data cleaning based on predefined restrictions, and data cleaning strategy. Key features: Focuses on the automation of data cleaning methods, including both theory and applications written in R. Enables the reader to design data cleaning processes for either one-off analytical purposes or for setting up production systems that clean data on a regular basis. Explores statistical techniques for solving issues such as incompleteness, contradictions and outliers, integration of data cleaning components and quality monitoring. Supported by an accompanying website featuring data and R code. This book enables data scientists and statistical analysts working with data to deepen their understanding of data cleaning as well as to upgrade their practical data cleaning skills. It can also be used as material for a course in data cleaning and analyses.
Cleaning Data For Effective Data Science
DOWNLOAD
Author : David Mertz
language : en
Publisher: Packt Publishing Ltd
Release Date : 2021-03-31
Cleaning Data For Effective Data Science written by David Mertz and has been published by Packt Publishing Ltd this book supported file pdf, txt, epub, kindle and other format this book has been release on 2021-03-31 with Mathematics categories.
Think about your data intelligently and ask the right questions Key FeaturesMaster data cleaning techniques necessary to perform real-world data science and machine learning tasksSpot common problems with dirty data and develop flexible solutions from first principlesTest and refine your newly acquired skills through detailed exercises at the end of each chapterBook Description Data cleaning is the all-important first step to successful data science, data analysis, and machine learning. If you work with any kind of data, this book is your go-to resource, arming you with the insights and heuristics experienced data scientists had to learn the hard way. In a light-hearted and engaging exploration of different tools, techniques, and datasets real and fictitious, Python veteran David Mertz teaches you the ins and outs of data preparation and the essential questions you should be asking of every piece of data you work with. Using a mixture of Python, R, and common command-line tools, Cleaning Data for Effective Data Science follows the data cleaning pipeline from start to end, focusing on helping you understand the principles underlying each step of the process. You'll look at data ingestion of a vast range of tabular, hierarchical, and other data formats, impute missing values, detect unreliable data and statistical anomalies, and generate synthetic features. The long-form exercises at the end of each chapter let you get hands-on with the skills you've acquired along the way, also providing a valuable resource for academic courses. What you will learnIngest and work with common data formats like JSON, CSV, SQL and NoSQL databases, PDF, and binary serialized data structuresUnderstand how and why we use tools such as pandas, SciPy, scikit-learn, Tidyverse, and BashApply useful rules and heuristics for assessing data quality and detecting bias, like Benford’s law and the 68-95-99.7 ruleIdentify and handle unreliable data and outliers, examining z-score and other statistical propertiesImpute sensible values into missing data and use sampling to fix imbalancesUse dimensionality reduction, quantization, one-hot encoding, and other feature engineering techniques to draw out patterns in your dataWork carefully with time series data, performing de-trending and interpolationWho this book is for This book is designed to benefit software developers, data scientists, aspiring data scientists, teachers, and students who work with data. If you want to improve your rigor in data hygiene or are looking for a refresher, this book is for you. Basic familiarity with statistics, general concepts in machine learning, knowledge of a programming language (Python or R), and some exposure to data science are helpful.
Best Practices In Data Cleaning
DOWNLOAD
Author : Jason W. Osborne
language : en
Publisher: SAGE
Release Date : 2013
Best Practices In Data Cleaning written by Jason W. Osborne and has been published by SAGE this book supported file pdf, txt, epub, kindle and other format this book has been release on 2013 with Mathematics categories.
Many researchers jump straight from data collection to data analysis without realizing how analyses and hypothesis tests can go profoundly wrong without clean data. This book provides a clear, step-by-step process of examining and cleaning data in order to decrease error rates and increase both the power and replicability of results. Jason W. Osborne, author of Best Practices in Quantitative Methods (SAGE, 2008) provides easily-implemented suggestions that are research-based and will motivate change in practice by empirically demonstrating, for each topic, the benefits of following best practices and the potential consequences of not following these guidelines. If your goal is to do the best research you can do, draw conclusions that are most likely to be accurate representations of the population(s) you wish to speak about, and report results that are most likely to be replicated by other researchers, then this basic guidebook will be indispensible.
Data Wrangling With R
DOWNLOAD
Author : Bradley C. Boehmke, Ph.D.
language : en
Publisher: Springer
Release Date : 2016-11-17
Data Wrangling With R written by Bradley C. Boehmke, Ph.D. and has been published by Springer this book supported file pdf, txt, epub, kindle and other format this book has been release on 2016-11-17 with Computers categories.
This guide for practicing statisticians, data scientists, and R users and programmers will teach the essentials of preprocessing: data leveraging the R programming language to easily and quickly turn noisy data into usable pieces of information. Data wrangling, which is also commonly referred to as data munging, transformation, manipulation, janitor work, etc., can be a painstakingly laborious process. Roughly 80% of data analysis is spent on cleaning and preparing data; however, being a prerequisite to the rest of the data analysis workflow (visualization, analysis, reporting), it is essential that one become fluent and efficient in data wrangling techniques. This book will guide the user through the data wrangling process via a step-by-step tutorial approach and provide a solid foundation for working with data in R. The author's goal is to teach the user how to easily wrangle data in order to spend more time on understanding the content of the data. By the end of the book, the user will have learned: How to work with different types of data such as numerics, characters, regular expressions, factors, and dates The difference between different data structures and how to create, add additional components to, and subset each data structure How to acquire and parse data from locations previously inaccessible How to develop functions and use loop control structures to reduce code redundancy How to use pipe operators to simplify code and make it more readable How to reshape the layout of data and manipulate, summarize, and join data sets
Sas For R Users
DOWNLOAD
Author : Ajay Ohri
language : en
Publisher: John Wiley & Sons
Release Date : 2019-09-24
Sas For R Users written by Ajay Ohri and has been published by John Wiley & Sons this book supported file pdf, txt, epub, kindle and other format this book has been release on 2019-09-24 with Computers categories.
BRIDGES THE GAP BETWEEN SAS AND R, ALLOWING USERS TRAINED IN ONE LANGUAGE TO EASILY LEARN THE OTHER SAS and R are widely-used, very different software environments. Prized for its statistical and graphical tools, R is an open-source programming language that is popular with statisticians and data miners who develop statistical software and analyze data. SAS (Statistical Analysis System) is the leading corporate software in analytics thanks to its faster data handling and smaller learning curve. SAS for R Users enables entry-level data scientists to take advantage of the best aspects of both tools by providing a cross-functional framework for users who already know R but may need to work with SAS. Those with knowledge of both R and SAS are of far greater value to employers, particularly in corporate settings. Using a clear, step-by-step approach, this book presents an analytics workflow that mirrors that of the everyday data scientist. This up-to-date guide is compatible with the latest R packages as well as SAS University Edition. Useful for anyone seeking employment in data science, this book: Instructs both practitioners and students fluent in one language seeking to learn the other Provides command-by-command translations of R to SAS and SAS to R Offers examples and applications in both R and SAS Presents step-by-step guidance on workflows, color illustrations, sample code, chapter quizzes, and more Includes sections on advanced methods and applications Designed for professionals, researchers, and students, SAS for R Users is a valuable resource for those with some knowledge of coding and basic statistics who wish to enter the realm of data science and business analytics.
R For Political Data Science
DOWNLOAD
Author : Francisco Urdinez
language : en
Publisher: CRC Press
Release Date : 2020-11-17
R For Political Data Science written by Francisco Urdinez and has been published by CRC Press this book supported file pdf, txt, epub, kindle and other format this book has been release on 2020-11-17 with Political Science categories.
R for Political Data Science: A Practical Guide is a handbook for political scientists new to R who want to learn the most useful and common ways to interpret and analyze political data. It was written by political scientists, thinking about the many real-world problems faced in their work. The book has 16 chapters and is organized in three sections. The first, on the use of R, is for those users who are learning R or are migrating from another software. The second section, on econometric models, covers OLS, binary and survival models, panel data, and causal inference. The third section is a data science toolbox of some the most useful tools in the discipline: data imputation, fuzzy merge of large datasets, web mining, quantitative text analysis, network analysis, mapping, spatial cluster analysis, and principal component analysis. Key features: Each chapter has the most up-to-date and simple option available for each task, assuming minimal prerequisites and no previous experience in R Makes extensive use of the Tidyverse, the group of packages that has revolutionized the use of R Provides a step-by-step guide that you can replicate using your own data Includes exercises in every chapter for course use or self-study Focuses on practical-based approaches to statistical inference rather than mathematical formulae Supplemented by an R package, including all data As the title suggests, this book is highly applied in nature, and is designed as a toolbox for the reader. It can be used in methods and data science courses, at both the undergraduate and graduate levels. It will be equally useful for a university student pursuing a PhD, political consultants, or a public official, all of whom need to transform their datasets into substantive and easily interpretable conclusions.
Data Science In Education Using R
DOWNLOAD
Author : Ryan A. Estrellado
language : en
Publisher: Routledge
Release Date : 2020-10-26
Data Science In Education Using R written by Ryan A. Estrellado and has been published by Routledge this book supported file pdf, txt, epub, kindle and other format this book has been release on 2020-10-26 with Education categories.
Data Science in Education Using R is the go-to reference for learning data science in the education field. The book answers questions like: What does a data scientist in education do? How do I get started learning R, the popular open-source statistical programming language? And what does a data analysis project in education look like? If you’re just getting started with R in an education job, this is the book you’ll want with you. This book gets you started with R by teaching the building blocks of programming that you’ll use many times in your career. The book takes a "learn by doing" approach and offers eight analysis walkthroughs that show you a data analysis from start to finish, complete with code for you to practice with. The book finishes with how to get involved in the data science community and how to integrate data science in your education job. This book will be an essential resource for education professionals and researchers looking to increase their data analysis skills as part of their professional and academic development.