A Procedure For Selection Of Explanatory Variables In Generalized Linear Models
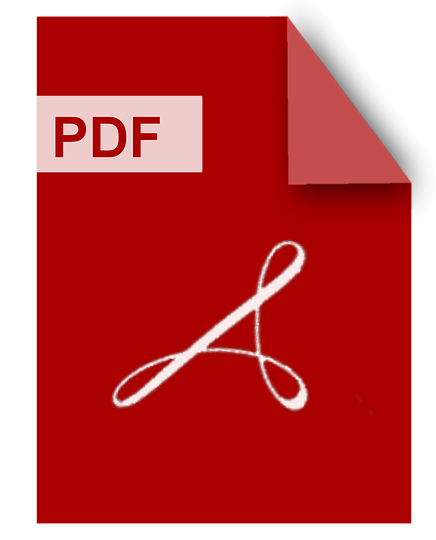
DOWNLOAD
Download A Procedure For Selection Of Explanatory Variables In Generalized Linear Models PDF/ePub or read online books in Mobi eBooks. Click Download or Read Online button to get A Procedure For Selection Of Explanatory Variables In Generalized Linear Models book now. This website allows unlimited access to, at the time of writing, more than 1.5 million titles, including hundreds of thousands of titles in various foreign languages. If the content not found or just blank you must refresh this page
A Procedure For Selection Of Explanatory Variables In Generalized Linear Models
DOWNLOAD
Author : Lennart Nordberg
language : en
Publisher:
Release Date : 1981
A Procedure For Selection Of Explanatory Variables In Generalized Linear Models written by Lennart Nordberg and has been published by this book supported file pdf, txt, epub, kindle and other format this book has been release on 1981 with categories.
Generalized Linear Models
DOWNLOAD
Author : P. McCullagh
language : en
Publisher: Routledge
Release Date : 2019-01-22
Generalized Linear Models written by P. McCullagh and has been published by Routledge this book supported file pdf, txt, epub, kindle and other format this book has been release on 2019-01-22 with Mathematics categories.
The success of the first edition of Generalized Linear Models led to the updated Second Edition, which continues to provide a definitive unified, treatment of methods for the analysis of diverse types of data. Today, it remains popular for its clarity, richness of content and direct relevance to agricultural, biological, health, engineering, and ot
Applying Generalized Linear Models
DOWNLOAD
Author : James K. Lindsey
language : en
Publisher: Springer Science & Business Media
Release Date : 2008-01-15
Applying Generalized Linear Models written by James K. Lindsey and has been published by Springer Science & Business Media this book supported file pdf, txt, epub, kindle and other format this book has been release on 2008-01-15 with Mathematics categories.
This book describes how generalised linear modelling procedures can be used in many different fields, without becoming entangled in problems of statistical inference. The author shows the unity of many of the commonly used models and provides readers with a taste of many different areas, such as survival models, time series, and spatial analysis, and of their unity. As such, this book will appeal to applied statisticians and to scientists having a basic grounding in modern statistics. With many exercises at the end of each chapter, it will equally constitute an excellent text for teaching applied statistics students and non- statistics majors. The reader is assumed to have knowledge of basic statistical principles, whether from a Bayesian, frequentist, or direct likelihood point of view, being familiar at least with the analysis of the simpler normal linear models, regression and ANOVA.
Variable Selection By Regularization Methods For Generalized Mixed Models
DOWNLOAD
Author : Andreas Groll
language : en
Publisher: Cuvillier Verlag
Release Date : 2011-12-13
Variable Selection By Regularization Methods For Generalized Mixed Models written by Andreas Groll and has been published by Cuvillier Verlag this book supported file pdf, txt, epub, kindle and other format this book has been release on 2011-12-13 with Business & Economics categories.
A regression analysis describes the dependency of random variables in the form of a functional relationship. One distinguishes between the dependent response variable and one or more independent influence variables. There is a variety of model classes and inference methods available, ranging from the conventional linear regression model up to recent non- and semiparametric regression models. The so-called generalized regression models form a methodically consistent framework incorporating many regression approaches with response variables that are not necessarily normally distributed, including the conventional linear regression model based on the normal distribution assumption as a special case. When repeated measurements are modeled in addition to fixed effects also random effects or coefficients can be included. Such models are known as Random Effects Models or Mixed Models. As a consequence, regression procedures are applicable extremely versatile and consider very different problems. In this dissertation regularization techniques for generalized mixed models are developed that are able to perform variable selection. These techniques are especially appropriate when many potential influence variables are present and existing approaches tend to fail. First of all a componentwise boosting technique for generalized linear mixed models is presented which is based on the likelihood function and works by iteratively fitting the residuals using weak learners. The complexity of the resulting estimator is determined by information criteria. For the estimation of variance components two approaches are considered, an estimator resulting from maximizing the profile likelihood, and an estimator which can be calculated using an approximative EM-algorithm. Then the boosting concept is extended to mixed models with ordinal response variables. Two different types of ordered models are considered, the threshold model, also known as cumulative model, and the sequential model. Both are based on the assumption that the observed response variable results from a categorized version of a latent metric variable. In the further course of the thesis the boosting approach is extended to additive predictors. The unknown functions to be estimated are expanded in B-spline basis functions, whose smoothness is controlled by penalty terms. Finally, a suitable L1-regularization technique for generalized linear models is presented, which is based on a combination of Fisher scoring and gradient optimization. Extensive simulation studies and numerous applications illustrate the competitiveness of the methods constructed in this thesis compared to conventional approaches. For the calculation of standard errors bootstrap methods are used.
Variable Selection In Generalized Linear Models
DOWNLOAD
Author : Jan Ulbricht
language : de
Publisher:
Release Date : 2010
Variable Selection In Generalized Linear Models written by Jan Ulbricht and has been published by this book supported file pdf, txt, epub, kindle and other format this book has been release on 2010 with categories.
A Method For Estimation Of Generalized Linear Models When Explanatory Variables Contain Meaurement Error
DOWNLOAD
Author : Roger Mark Sauter
language : en
Publisher:
Release Date : 1989
A Method For Estimation Of Generalized Linear Models When Explanatory Variables Contain Meaurement Error written by Roger Mark Sauter and has been published by this book supported file pdf, txt, epub, kindle and other format this book has been release on 1989 with Linear models (Statistics) categories.
This thesis considers the problem of estimating the linear parameters of generalized linear models (GLM), especially binomial and Poisson regression models, when the explanatory variable is subject to measurement error. In this situation, the dependence of the response variable on the observed explanatory variable cannot typically be modeled as a GLM; in particular, extra variability caused by measurement error cannot be accounted for using the binomial- or Poisson models. One strategy is to use existing methods adapted for extra-variability. The contribution of this thesis is to introduce an estimation method which makes use of Efron's (1986) double exponential family. The proposed method involves the calculation of maximum likelihood estimates from this density when it is used as an approximation to the true density of the response variables given the observed measurements. Efron's family of distributions offers an attractive alternative for approximating the distribution of the response variable given the observed explanatory variable and is closely related to the measurement error in GLM methods suggested by Armstrong (1985) and Prentice (1986). Properties of the proposed method are considered when the double exponential family model is thought to be correct and when it is thought to be an approximation. Special cases and examples are given to illustrate the estimation procedure and how to apply this method. Comparisons are made with other estimation procedures for the measurement error problem, both procedurally and numerically.
Generalized Linear Models For Insurance Data
DOWNLOAD
Author : Piet de Jong
language : en
Publisher: Cambridge University Press
Release Date : 2008-02-28
Generalized Linear Models For Insurance Data written by Piet de Jong and has been published by Cambridge University Press this book supported file pdf, txt, epub, kindle and other format this book has been release on 2008-02-28 with Business & Economics categories.
This is the only book actuaries need to understand generalized linear models (GLMs) for insurance applications. GLMs are used in the insurance industry to support critical decisions. Until now, no text has introduced GLMs in this context or addressed the problems specific to insurance data. Using insurance data sets, this practical, rigorous book treats GLMs, covers all standard exponential family distributions, extends the methodology to correlated data structures, and discusses recent developments which go beyond the GLM. The issues in the book are specific to insurance data, such as model selection in the presence of large data sets and the handling of varying exposure times. Exercises and data-based practicals help readers to consolidate their skills, with solutions and data sets given on the companion website. Although the book is package-independent, SAS code and output examples feature in an appendix and on the website. In addition, R code and output for all the examples are provided on the website.
The Estimation And Inference Of Complex Models
DOWNLOAD
Author : Min Zhou
language : en
Publisher:
Release Date : 2017
The Estimation And Inference Of Complex Models written by Min Zhou and has been published by this book supported file pdf, txt, epub, kindle and other format this book has been release on 2017 with Electronic books categories.
In this thesis, we investigate the estimation problem and inference problem for the complex models. Two major categories of complex models are emphasized by us, one is generalized linear models, the other is time series models. For the generalized linear models, we consider one fundamental problem about sure screening for interaction terms in ultra-high dimensional feature space; for time series models, an important model assumption about Markov property is considered by us. The first part of this thesis illustrates the significant interaction pursuit problem for ultra-high dimensional models with two-way interaction effects. We propose a simple sure screening procedure (SSI) to detect significant interactions between the explanatory variables and the response variable in the high or ultra-high dimensional generalized linear regression models. Sure screening method is a simple, but powerful tool for the first step of feature selection or variable selection for ultra-high dimensional data. We investigate the sure screening properties of the proposal method from theoretical insight. Furthermore, we indicate that our proposed method can control the false discovery rate at a reasonable size, so the regularized variable selection methods can be easily applied to get more accurate feature selection in the following model selection procedures. Moreover, from the viewpoint of computational efficiency, we suggest a much more efficient algorithm-discretized SSI (DSSI) to realize our proposed sure screening method in practice. And we also investigate the properties of these two algorithms SSI and DSSI in simulation studies and apply them to some real data analyses for illustration. For the second part, our concern is the testing of the Markov property in time series processes. Markovian assumption plays an extremely important role in time series analysis and is also a fundamental assumption in economic and financial models. However, few existing research mainly focused on how to test the Markov properties for the time series processes. Therefore, for the Markovian assumption, we propose a new test procedure to check if the time series with beta-mixing possesses the Markov property. Our test is based on the Conditional Distance Covariance (CDCov). We investigate the theoretical properties of the proposed method. The asymptotic distribution of the proposed test statistic under the null hypothesis is obtained, and the power of the test procedure under local alternative hypothesizes have been studied. Simulation studies are conducted to demonstrate the finite sample performance of our test.
An Introduction To Generalized Linear Models
DOWNLOAD
Author : George H. Dunteman
language : en
Publisher: SAGE Publications
Release Date : 2005-09-22
An Introduction To Generalized Linear Models written by George H. Dunteman and has been published by SAGE Publications this book supported file pdf, txt, epub, kindle and other format this book has been release on 2005-09-22 with Social Science categories.
Do you have data that is not normally distributed and don′t know how to analyze it using generalized linear models (GLM)? Beginning with a discussion of fundamental statistical modeling concepts in a multiple regression framework, the authors extend these concepts to GLM (including Poisson regression. logistic regression, and proportional hazards models) and demonstrate the similarity of various regression models to GLM. Each procedure is illustrated using real life data sets, and the computer instructions and results will be presented for each example. Throughout the book, there is an emphasis on link functions and error distribution and how the model specifications translate into likelihood functions that can, through maximum likelihood estimation be used to estimate the regression parameters and their associated standard errors. This book provides readers with basic modeling principles that are applicable to a wide variety of situations. Key Features: - Provides an accessible but thorough introduction to GLM, exponential family distribution, and maximum likelihood estimation - Includes discussion on checking model adequacy and description on how to use SAS to fit GLM - Describes the connection between survival analysis and GLM This book is an ideal text for social science researchers who do not have a strong statistical background, but would like to learn more advanced techniques having taken an introductory course covering regression analysis.
Linear Models And Generalizations
DOWNLOAD
Author : C. Radhakrishna Rao
language : en
Publisher: Springer Science & Business Media
Release Date : 2007-10-15
Linear Models And Generalizations written by C. Radhakrishna Rao and has been published by Springer Science & Business Media this book supported file pdf, txt, epub, kindle and other format this book has been release on 2007-10-15 with Mathematics categories.
Revised and updated with the latest results, this Third Edition explores the theory and applications of linear models. The authors present a unified theory of inference from linear models and its generalizations with minimal assumptions. They not only use least squares theory, but also alternative methods of estimation and testing based on convex loss functions and general estimating equations. Highlights of coverage include sensitivity analysis and model selection, an analysis of incomplete data, an analysis of categorical data based on a unified presentation of generalized linear models, and an extensive appendix on matrix theory.