Additive Logistic Regression
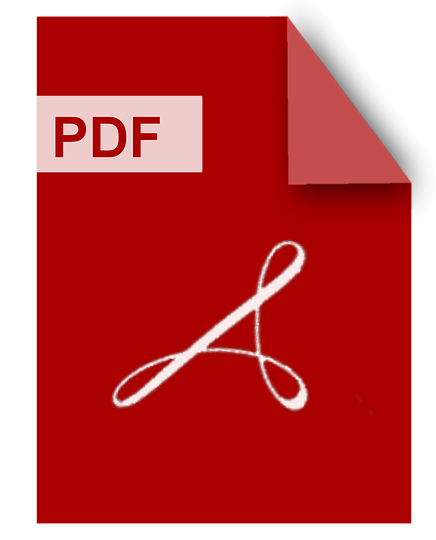
DOWNLOAD eBooks
Download Additive Logistic Regression PDF/ePub or read online books in Mobi eBooks. Click Download or Read Online button to get Additive Logistic Regression book now. This website allows unlimited access to, at the time of writing, more than 1.5 million titles, including hundreds of thousands of titles in various foreign languages. If the content not found or just blank you must refresh this page
Additive Logistic Regression
DOWNLOAD eBooks
Author : Jerome H. Friedman
language : en
Publisher:
Release Date : 1998
Additive Logistic Regression written by Jerome H. Friedman and has been published by this book supported file pdf, txt, epub, kindle and other format this book has been release on 1998 with Regression analysis categories.
Discussion Additive Logistic Regression
DOWNLOAD eBooks
Author :
language : en
Publisher:
Release Date : 2000
Discussion Additive Logistic Regression written by and has been published by this book supported file pdf, txt, epub, kindle and other format this book has been release on 2000 with categories.
Generalized Additive Models
DOWNLOAD eBooks
Author : T.J. Hastie
language : en
Publisher: Routledge
Release Date : 2017-10-19
Generalized Additive Models written by T.J. Hastie and has been published by Routledge this book supported file pdf, txt, epub, kindle and other format this book has been release on 2017-10-19 with Mathematics categories.
This book describes an array of power tools for data analysis that are based on nonparametric regression and smoothing techniques. These methods relax the linear assumption of many standard models and allow analysts to uncover structure in the data that might otherwise have been missed. While McCullagh and Nelder's Generalized Linear Models shows how to extend the usual linear methodology to cover analysis of a range of data types, Generalized Additive Models enhances this methodology even further by incorporating the flexibility of nonparametric regression. Clear prose, exercises in each chapter, and case studies enhance this popular text.
Generalized Additive Models
DOWNLOAD eBooks
Author : Stanford University. Department of Statistics
language : en
Publisher:
Release Date : 1984
Generalized Additive Models written by Stanford University. Department of Statistics and has been published by this book supported file pdf, txt, epub, kindle and other format this book has been release on 1984 with Random walks (Mathematics) categories.
Likelihood based regression models, such as the normal linear regression model and the linear logistic model, assume a linear (or some other parametric) form for the covariate effects. The authors introduce the Local Scoring procedure which is applicable to any likelihood-based regression model: the class of Generalized Linear Models contains many of these. In this class the Local Scoring procedure replaces a linear predictor by a additive predictor; hence the name Generalized Additive Models. Local Scoring can also be applied to non-standard models like Cox's proportional hazards model for survival data.
Vector Generalized Linear And Additive Models
DOWNLOAD eBooks
Author : Thomas W. Yee
language : en
Publisher: Springer
Release Date : 2015-09-11
Vector Generalized Linear And Additive Models written by Thomas W. Yee and has been published by Springer this book supported file pdf, txt, epub, kindle and other format this book has been release on 2015-09-11 with Mathematics categories.
This book presents a greatly enlarged statistical framework compared to generalized linear models (GLMs) with which to approach regression modelling. Comprising of about half-a-dozen major classes of statistical models, and fortified with necessary infrastructure to make the models more fully operable, the framework allows analyses based on many semi-traditional applied statistics models to be performed as a coherent whole. Since their advent in 1972, GLMs have unified important distributions under a single umbrella with enormous implications. However, GLMs are not flexible enough to cope with the demands of practical data analysis. And data-driven GLMs, in the form of generalized additive models (GAMs), are also largely confined to the exponential family. The methodology here and accompanying software (the extensive VGAM R package) are directed at these limitations and are described comprehensively for the first time in one volume. This book treats distributions and classical models as generalized regression models, and the result is a much broader application base for GLMs and GAMs. The book can be used in senior undergraduate or first-year postgraduate courses on GLMs or categorical data analysis and as a methodology resource for VGAM users. In the second part of the book, the R package VGAM allows readers to grasp immediately applications of the methodology. R code is integrated in the text, and datasets are used throughout. Potential applications include ecology, finance, biostatistics, and social sciences. The methodological contribution of this book stands alone and does not require use of the VGAM package.
Interpretable Machine Learning
DOWNLOAD eBooks
Author : Christoph Molnar
language : en
Publisher: Lulu.com
Release Date : 2020
Interpretable Machine Learning written by Christoph Molnar and has been published by Lulu.com this book supported file pdf, txt, epub, kindle and other format this book has been release on 2020 with Artificial intelligence categories.
This book is about making machine learning models and their decisions interpretable. After exploring the concepts of interpretability, you will learn about simple, interpretable models such as decision trees, decision rules and linear regression. Later chapters focus on general model-agnostic methods for interpreting black box models like feature importance and accumulated local effects and explaining individual predictions with Shapley values and LIME. All interpretation methods are explained in depth and discussed critically. How do they work under the hood? What are their strengths and weaknesses? How can their outputs be interpreted? This book will enable you to select and correctly apply the interpretation method that is most suitable for your machine learning project.
The Elements Of Statistical Learning
DOWNLOAD eBooks
Author : Trevor Hastie
language : en
Publisher: Springer Science & Business Media
Release Date : 2013-11-11
The Elements Of Statistical Learning written by Trevor Hastie and has been published by Springer Science & Business Media this book supported file pdf, txt, epub, kindle and other format this book has been release on 2013-11-11 with Mathematics categories.
During the past decade there has been an explosion in computation and information technology. With it have come vast amounts of data in a variety of fields such as medicine, biology, finance, and marketing. The challenge of understanding these data has led to the development of new tools in the field of statistics, and spawned new areas such as data mining, machine learning, and bioinformatics. Many of these tools have common underpinnings but are often expressed with different terminology. This book describes the important ideas in these areas in a common conceptual framework. While the approach is statistical, the emphasis is on concepts rather than mathematics. Many examples are given, with a liberal use of color graphics. It should be a valuable resource for statisticians and anyone interested in data mining in science or industry. The book’s coverage is broad, from supervised learning (prediction) to unsupervised learning. The many topics include neural networks, support vector machines, classification trees and boosting---the first comprehensive treatment of this topic in any book. This major new edition features many topics not covered in the original, including graphical models, random forests, ensemble methods, least angle regression & path algorithms for the lasso, non-negative matrix factorization, and spectral clustering. There is also a chapter on methods for “wide” data (p bigger than n), including multiple testing and false discovery rates. Trevor Hastie, Robert Tibshirani, and Jerome Friedman are professors of statistics at Stanford University. They are prominent researchers in this area: Hastie and Tibshirani developed generalized additive models and wrote a popular book of that title. Hastie co-developed much of the statistical modeling software and environment in R/S-PLUS and invented principal curves and surfaces. Tibshirani proposed the lasso and is co-author of the very successful An Introduction to the Bootstrap. Friedman is the co-inventor of many data-mining tools including CART, MARS, projection pursuit and gradient boosting.
Generalized Additive Models
DOWNLOAD eBooks
Author : Simon Wood
language : en
Publisher: CRC Press
Release Date : 2006-02-27
Generalized Additive Models written by Simon Wood and has been published by CRC Press this book supported file pdf, txt, epub, kindle and other format this book has been release on 2006-02-27 with Mathematics categories.
Now in widespread use, generalized additive models (GAMs) have evolved into a standard statistical methodology of considerable flexibility. While Hastie and Tibshirani's outstanding 1990 research monograph on GAMs is largely responsible for this, there has been a long-standing need for an accessible introductory treatment of the subject that also emphasizes recent penalized regression spline approaches to GAMs and the mixed model extensions of these models. Generalized Additive Models: An Introduction with R imparts a thorough understanding of the theory and practical applications of GAMs and related advanced models, enabling informed use of these very flexible tools. The author bases his approach on a framework of penalized regression splines, and builds a well-grounded foundation through motivating chapters on linear and generalized linear models. While firmly focused on the practical aspects of GAMs, discussions include fairly full explanations of the theory underlying the methods. Use of the freely available R software helps explain the theory and illustrates the practicalities of linear, generalized linear, and generalized additive models, as well as their mixed effect extensions. The treatment is rich with practical examples, and it includes an entire chapter on the analysis of real data sets using R and the author's add-on package mgcv. Each chapter includes exercises, for which complete solutions are provided in an appendix. Concise, comprehensive, and essentially self-contained, Generalized Additive Models: An Introduction with R prepares readers with the practical skills and the theoretical background needed to use and understand GAMs and to move on to other GAM-related methods and models, such as SS-ANOVA, P-splines, backfitting and Bayesian approaches to smoothing and additive modelling.
Advances In Knowledge Discovery And Data Mining
DOWNLOAD eBooks
Author : Honghua Dai
language : en
Publisher: Springer Science & Business Media
Release Date : 2004-05-11
Advances In Knowledge Discovery And Data Mining written by Honghua Dai and has been published by Springer Science & Business Media this book supported file pdf, txt, epub, kindle and other format this book has been release on 2004-05-11 with Business & Economics categories.
This book constitutes the refereed proceedings of the 8th Pacific-Asia Conference on Knowledge Discovery and Data mining, PAKDD 2004, held in Sydney, Australia in May 2004. The 50 revised full papers and 31 revised short papers presented were carefully reviewed and selected from a total of 238 submissions. The papers are organized in topical sections on classification; clustering; association rules; novel algorithms; event mining, anomaly detection, and intrusion detection; ensemble learning; Bayesian network and graph mining; text mining; multimedia mining; text mining and Web mining; statistical methods, sequential data mining, and time series mining; and biomedical data mining.
Logistic Regression Models
DOWNLOAD eBooks
Author : Joseph M. Hilbe
language : en
Publisher: CRC Press
Release Date : 2009-05-11
Logistic Regression Models written by Joseph M. Hilbe and has been published by CRC Press this book supported file pdf, txt, epub, kindle and other format this book has been release on 2009-05-11 with Mathematics categories.
Logistic Regression Models presents an overview of the full range of logistic models, including binary, proportional, ordered, partially ordered, and unordered categorical response regression procedures. Other topics discussed include panel, survey, skewed, penalized, and exact logistic models. The text illustrates how to apply the various models t