An Improved Clustering Method For Text Documents Using Neutrosophic Logic
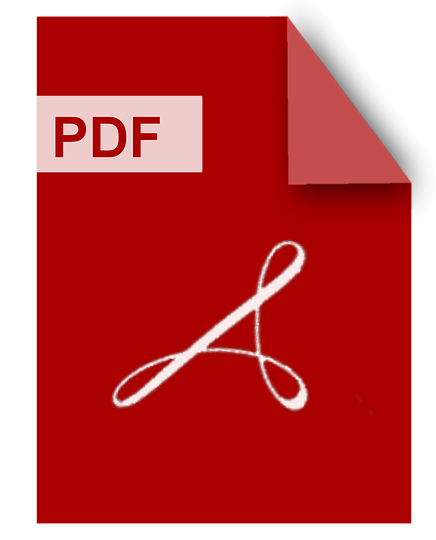
DOWNLOAD
Download An Improved Clustering Method For Text Documents Using Neutrosophic Logic PDF/ePub or read online books in Mobi eBooks. Click Download or Read Online button to get An Improved Clustering Method For Text Documents Using Neutrosophic Logic book now. This website allows unlimited access to, at the time of writing, more than 1.5 million titles, including hundreds of thousands of titles in various foreign languages. If the content not found or just blank you must refresh this page
An Improved Clustering Method For Text Documents Using Neutrosophic Logic
DOWNLOAD
Author : Nadeem Akhtar
language : en
Publisher: Infinite Study
Release Date :
An Improved Clustering Method For Text Documents Using Neutrosophic Logic written by Nadeem Akhtar and has been published by Infinite Study this book supported file pdf, txt, epub, kindle and other format this book has been release on with categories.
As a technique of Information Retrieval, we can consider clustering as an unsupervised learning problem in which we provide a structure to unlabeled and unknown data.
Applications Of Soft Computing For The Web
DOWNLOAD
Author : Rashid Ali
language : en
Publisher: Springer
Release Date : 2018-01-08
Applications Of Soft Computing For The Web written by Rashid Ali and has been published by Springer this book supported file pdf, txt, epub, kindle and other format this book has been release on 2018-01-08 with Computers categories.
This book discusses the applications of different soft computing techniques for the web-based systems and services. The respective chapters highlight recent developments in the field of soft computing applications, from web-based information retrieval to online marketing and online healthcare. In each chapter author endeavor to explain the basic ideas behind the proposed applications in an accessible format for readers who may not possess a background in these fields. This carefully edited book covers a wide range of new applications of soft computing techniques in Web recommender systems, Online documents classification, Online documents summarization, Online document clustering, Online market intelligence, Web usage profiling, Web data extraction, Social network extraction, Question answering systems, Online health care, Web knowledge management, Multimedia information retrieval, Navigation guides, User profiles extraction, Web-based distributed information systems, Web security applications, Internet of Things Applications and so on. The book is aimed for researchers and practitioner who are engaged in developing and applying intelligent systems principles for solving real-life problems. Further, it has been structured so that each chapter can be read independently of the others.
A Novel Framework Using Neutrosophy For Integrated Speech And Text Sentiment Analysis
DOWNLOAD
Author : Kritika Mishra
language : en
Publisher: Infinite Study
Release Date : 2020-10-18
A Novel Framework Using Neutrosophy For Integrated Speech And Text Sentiment Analysis written by Kritika Mishra and has been published by Infinite Study this book supported file pdf, txt, epub, kindle and other format this book has been release on 2020-10-18 with Computers categories.
We have proposed a novel framework that performs sentiment analysis on audio files by calculating their Single-Valued Neutrosophic Sets (SVNS) and clustering them into positive-neutral-negative and combines these results with those obtained by performing sentiment analysis on the text files of those audio.
Cognitive Intelligence With Neutrosophic Statistics In Bioinformatics
DOWNLOAD
Author : Florentin Smarandache
language : en
Publisher: Elsevier
Release Date : 2023-02-11
Cognitive Intelligence With Neutrosophic Statistics In Bioinformatics written by Florentin Smarandache and has been published by Elsevier this book supported file pdf, txt, epub, kindle and other format this book has been release on 2023-02-11 with Mathematics categories.
Cognitive Intelligence with Neutrosophic Statistics in Bioinformatics investigates and presents the many applications that have arisen in the last ten years using neutrosophic statistics in bioinformatics, medicine, agriculture and cognitive science. This book will be very useful to the scientific community, appealing to audiences interested in fuzzy, vague concepts from which uncertain data are collected, including academic researchers, practicing engineers and graduate students. Neutrosophic statistics is a generalization of classical statistics. In classical statistics, the data is known, formed by crisp numbers. In comparison, data in neutrosophic statistics has some indeterminacy. This data may be ambiguous, vague, imprecise, incomplete, and even unknown. Neutrosophic statistics refers to a set of data, such that the data or a part of it are indeterminate in some degree, and to methods used to analyze the data. - Introduces the field of neutrosophic statistics and how it can solve problems working with indeterminate (imprecise, ambiguous, vague, incomplete, unknown) data - Presents various applications of neutrosophic statistics in the fields of bioinformatics, medicine, cognitive science and agriculture - Provides practical examples and definitions of neutrosophic statistics in relation to the various types of indeterminacies
Neutrosophy
DOWNLOAD
Author : Florentin Smarandache
language : en
Publisher:
Release Date : 1998
Neutrosophy written by Florentin Smarandache and has been published by this book supported file pdf, txt, epub, kindle and other format this book has been release on 1998 with Mathematics categories.
Information Retrieval Architecture And Algorithms
DOWNLOAD
Author : Gerald Kowalski
language : en
Publisher: Springer Science & Business Media
Release Date : 2010-12-01
Information Retrieval Architecture And Algorithms written by Gerald Kowalski and has been published by Springer Science & Business Media this book supported file pdf, txt, epub, kindle and other format this book has been release on 2010-12-01 with Computers categories.
This text presents a theoretical and practical examination of the latest developments in Information Retrieval and their application to existing systems. By starting with a functional discussion of what is needed for an information system, the reader can grasp the scope of information retrieval problems and discover the tools to resolve them. The book takes a system approach to explore every functional processing step in a system from ingest of an item to be indexed to displaying results, showing how implementation decisions add to the information retrieval goal, and thus providing the user with the needed outcome, while minimizing their resources to obtain those results. The text stresses the current migration of information retrieval from just textual to multimedia, expounding upon multimedia search, retrieval and display, as well as classic and new textual techniques. It also introduces developments in hardware, and more importantly, search architectures, such as those introduced by Google, in order to approach scalability issues. About this textbook: A first course text for advanced level courses, providing a survey of information retrieval system theory and architecture, complete with challenging exercises Approaches information retrieval from a practical systems view in order for the reader to grasp both scope and solutions Features what is achievable using existing technologies and investigates what deficiencies warrant additional exploration
Fuzzy Sets Fuzzy Logic And Fuzzy Systems
DOWNLOAD
Author : Lotfi Asker Zadeh
language : en
Publisher: World Scientific
Release Date : 1996
Fuzzy Sets Fuzzy Logic And Fuzzy Systems written by Lotfi Asker Zadeh and has been published by World Scientific this book supported file pdf, txt, epub, kindle and other format this book has been release on 1996 with Computers categories.
This book consists of selected papers written by the founder of fuzzy set theory, Lotfi A Zadeh. Since Zadeh is not only the founder of this field, but has also been the principal contributor to its development over the last 30 years, the papers contain virtually all the major ideas in fuzzy set theory, fuzzy logic, and fuzzy systems in their historical context. Many of the ideas presented in the papers are still open to further development. The book is thus an important resource for anyone interested in the areas of fuzzy set theory, fuzzy logic, and fuzzy systems, as well as their applications. Moreover, the book is also intended to play a useful role in higher education, as a rich source of supplementary reading in relevant courses and seminars.The book contains a bibliography of all papers published by Zadeh in the period 1949-1995. It also contains an introduction that traces the development of Zadeh's ideas pertaining to fuzzy sets, fuzzy logic, and fuzzy systems via his papers. The ideas range from his 1965 seminal idea of the concept of a fuzzy set to ideas reflecting his current interest in computing with words ? a computing in which linguistic expressions are used in place of numbers.Places in the papers, where each idea is presented can easily be found by the reader via the Subject Index.
Neutrosophic Set A Generalization Of The Intuitionistic Fuzzy Set
DOWNLOAD
Author : Florentin Smarandache
language : en
Publisher: Infinite Study
Release Date : 2010-08-23
Neutrosophic Set A Generalization Of The Intuitionistic Fuzzy Set written by Florentin Smarandache and has been published by Infinite Study this book supported file pdf, txt, epub, kindle and other format this book has been release on 2010-08-23 with Mathematics categories.
In this paper one generalizes the intuitionistic fuzzy set (IFS), paraconsistent set, and intuitionistic set to the neutrosophic set (NS). Many examples are presented. Distinctions between NS and IFS are underlined.
Algorithms For Single Valued Neutrosophic Decision Making Based On Topsis And Clustering Methods With New Distance Measure
DOWNLOAD
Author : Harish Garg
language : en
Publisher: Infinite Study
Release Date :
Algorithms For Single Valued Neutrosophic Decision Making Based On Topsis And Clustering Methods With New Distance Measure written by Harish Garg and has been published by Infinite Study this book supported file pdf, txt, epub, kindle and other format this book has been release on with Mathematics categories.
Single-valued neutrosophic set (SVNS) is an important contrivance for directing the decision-making queries with unknown and indeterminant data by employing a degree of “acceptance”, “indeterminacy”, and “non-acceptance” in quantitative terms. Under this set, the objective of this paper is to propose some new distance measures to find discrimination between the SVNSs. The basic axioms of the measures have been highlighted and examined their properties. Furthermore, to examine the relevance of proposed measures, an extended TOPSIS (“technique for order preference by similarity to ideal solution”) method is introduced to solve the group decision-making problems. Additionally, a new clustering technique is proposed based on the stated measures to classify the objects. The advantages, comparative analysis as well as superiority analysis is given to shows its influence over existing approaches.
Feature Selection And Enhanced Krill Herd Algorithm For Text Document Clustering
DOWNLOAD
Author : Laith Mohammad Qasim Abualigah
language : en
Publisher: Springer
Release Date : 2018-12-18
Feature Selection And Enhanced Krill Herd Algorithm For Text Document Clustering written by Laith Mohammad Qasim Abualigah and has been published by Springer this book supported file pdf, txt, epub, kindle and other format this book has been release on 2018-12-18 with Technology & Engineering categories.
This book puts forward a new method for solving the text document (TD) clustering problem, which is established in two main stages: (i) A new feature selection method based on a particle swarm optimization algorithm with a novel weighting scheme is proposed, as well as a detailed dimension reduction technique, in order to obtain a new subset of more informative features with low-dimensional space. This new subset is subsequently used to improve the performance of the text clustering (TC) algorithm and reduce its computation time. The k-mean clustering algorithm is used to evaluate the effectiveness of the obtained subsets. (ii) Four krill herd algorithms (KHAs), namely, the (a) basic KHA, (b) modified KHA, (c) hybrid KHA, and (d) multi-objective hybrid KHA, are proposed to solve the TC problem; each algorithm represents an incremental improvement on its predecessor. For the evaluation process, seven benchmark text datasets are used with different characterizations and complexities. Text document (TD) clustering is a new trend in text mining in which the TDs are separated into several coherent clusters, where all documents in the same cluster are similar. The findings presented here confirm that the proposed methods and algorithms delivered the best results in comparison with other, similar methods to be found in the literature.